Contents
Understanding Supply Chain Automation
Applications of Supply Chain Automation
Data Challenges in Supply Chain Automation
Improving Data for Effective Supply Chain Automation
Conclusion
Encord Blog
What is Supply Chain Automation?
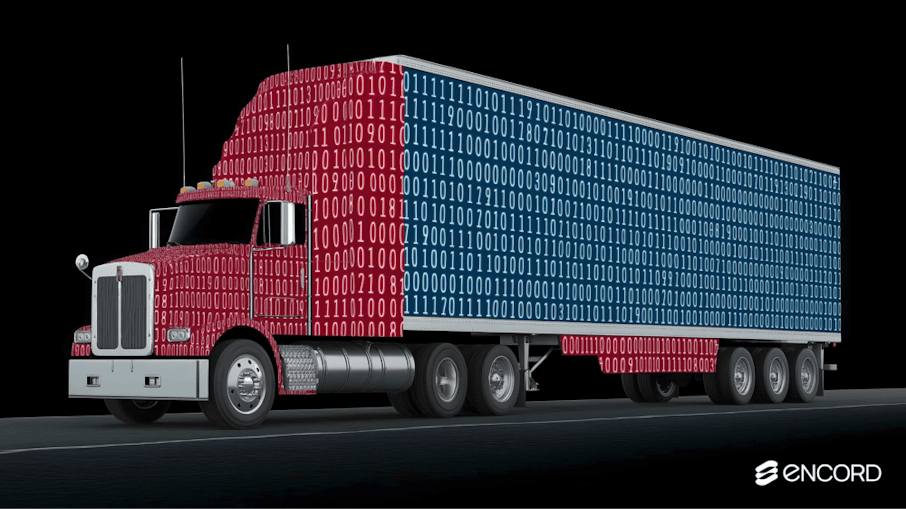
The global supply chain is more complex today than ever, with increasing demand for speed, accuracy, and efficiency. Businesses must move goods faster, while also reducing costs, minimizing errors and optimizing logistics. Traditional supply chain operations mainly rely on manual tasks and legacy systems and therefore, struggle to keep up with increasing demands.
Supply chain automation uses artificial intelligence (AI), robotics and data-driven systems to streamline operations from warehouse management to delivery. As the adoption of automation grows, the companies face new challenges, particularly in handling unstructured data and optimizing AI models for real world applications.
In this blog, we will explore supply chain automation, the data challenges companies face and how physical AI is rapidly transforming a number of industries to become more efficient, cost-effective, and accurate.
Understanding Supply Chain Automation
Supply chain automation refers to the use of AI and robotics to improve the efficiency in logistics, manufacturing, and distribution. By reducing manual intervention, businesses can improve speed, safety, accuracy and cost effectiveness. The automation can span across various stages like from real time inventory tracking to using robots to handle warehouse goods.
How Supply Chain Automation Solution Works?
The automation in the supply chain process generally involves:
- Robotic Process Automation (RPA): Using bots to handle repetitive tasks like data entry, order processing, and invoice management.
- Decision Making: Machine learning models analyze supply and demand patterns, and help businesses make better inventory and logistics decisions.
- Computer Vision & Robotics: Robots sort, pick, and pack goods in warehouses with precision, reducing human labor.
- IoT & Real-Time Tracking: Smart sensors track shipments, monitor warehouse conditions, and provide real-time updates on goods in transit.
- Autonomous Vehicles & Drones: Self-driving trucks and drones transport goods efficiently. It reduces dependency on human drivers.
Key Benefits of Supply Chain Automation
Increased Efficiency & Speed
Automation technologies work 24/7 without fatigue. It ensures faster processing times for tasks like order fulfillment, inventory management, and warehouse operations. Efficient robotic systems also reduce manual errors, leading to smoother logistics operations.
Workforce Optimization
Labor costs in warehousing and logistics are high, and staffing shortages can disrupt operations. Automation reduces reliance on manual labor for repetitive and physically demanding tasks, allowing human workers to focus on higher-value activities such as supervising AI-driven systems or handling exceptions. Also, automation helps businesses ensure safety for the workforce.
Improved Accuracy & Reduced Errors
Human errors in inventory tracking, order fulfillment, and logistics management can cause costly delays and stock discrepancies. AI-powered automation ensures precise data entry, accurate order picking, and real-time tracking, reducing mistakes across the supply chain.
Scalability & Flexibility
Automated systems can scale up or down based on demand fluctuations. For example, during peak seasons like Black Friday or holiday sales, AI-driven fulfillment centers can process higher volumes of orders without requiring additional workforce hiring.
Better Decision Making
With AI-powered analytics, businesses can predict demand, optimize inventory levels, and streamline logistics. This data-driven approach helps companies make faster, smarter decisions, improving overall supply chain management.
Why Supply Chain Automation is Critical Today?
The global supply chain has faced many unexpected challenges in recent years like pandemic-related disruptions, labor shortages, increasing e-commerce demand, and rising logistics costs. Companies that fail to automate risk falling behind competitors that use the efficiency of automation.
By implementing automation, businesses can future-proof their supply chains, ensuring agility, reliability, and scalability in an increasingly complex global market.
Applications of Supply Chain Automation
This is really transforming the industries by optimizing operations across warehousing, logistics, transportation, and fulfilment. Here are some of the key applications:
Automated Logistics
Warehouses nowadays are becoming fully automated environments where robotic systems handle tasks that require significant labor. This includes:
- Automated Picking & Sorting: Automated conveyor systems manage inventory movement, increasing the speed of fulfillment.
- Inventory Tracking: IoT sensors, RFID tags, and computer vision continuously track stock levels in real-time, reducing errors.
- Automated Storage & Retrieval Systems (AS/RS): These systems use robotic shuttles and cranes to optimize space utilization and ensure fast, efficient retrieval of items.
- Dynamic Order Processing: AI algorithms prioritize orders based on urgency, demand, and supply chain constraints.
Example
Massive fulfilment centers like Amazon use robotic arms to sort, pick and package millions of products daily. It reduces the need for manual labor and increases efficiency.
Autonomous Freight and Delivery
The transportation and logistics sector is integrating AI to improve efficiency, reduce delivery times, and minimize operational costs. This includes:
- Autonomous Vehicles & Drones: Self-driving trucks and delivery drones are being deployed for delivering products to customers, reducing dependence on human drivers.
- Route Optimization: Machine learning algorithms analyze traffic, weather, and delivery schedules to optimize routes. This helps in cutting fuel costs and improving on-time deliveries.
- Smart Freight Tracking: GPS and IoT sensors provide real-time shipment tracking, improving transparency and security in logistics.
Example
FedEx and UPS are testing autonomous delivery vehicles and AI route planning to speed up shipments and optimize delivery networks.
Quality Control and Inspection
Given the volume of the products handled by businesses, using AI models for quality control and inspection of the products at least the first line of inspection can be helpful.
- Defect Detection: Computer vision systems inspect goods in real-time, and identify defects or damages before they reach customers.
- Automated Sorting & Rejection: Robotics handle product sorting, and make sure defective items are removed from the supply chain before shipment.
- Predictive Maintenance for Equipment: AI systems monitor warehouse machinery and fleet vehicles, detecting potential failures before they occur.
Example
The Tesla factories use real time defect detection systems during the manufacturing and packaging process.
Demand Forecasting
Predictive analytics is helping businesses make better and data driven decisions by utilixing the huge amounts of supply chain data. Some of the applications are:
- Predicting Demand Spikes: Machine learning models analyze historical data, seasonal trends, and market conditions to optimize stock levels.
- Preventing Stock Shortages and Overstocking: Automated inventory systems adjust product procurement according to real-time visibility based on demand forecasts.
- Dynamic Pricing Adjustments: Data driven insights allow businesses to adjust pricing dynamically based on supply and demand fluctuations.
Example
Walmart uses forecasting models for inventory management across its global supply chain. It also analyses local demographics and purchasing patterns for cost savings associated with excess inventory, prevent stockouts and in general improve customer satisfaction.
Warehouse Automation
This makes the warehouse operations faster, safer and more efficient by automating one of the most physically demanding tasks in the supply chain businesses.
Source
Some of the applications are:
- Automated Unloading and Loading: Traditional trailer unloading is labor-intensive and slow. The robots automate the process, increasing speed while reducing physical strain on workers.
- Labor Optimization: By automating repetitive tasks, warehouse workers can shift to supervisory and higher-value roles, improving overall operational efficiency.
- Robotic Picking & Sorting: The robots can handle package sorting and placement with CV and ML models to minimize errors and maximize efficiency.
Example
Pickle Robot uses robotic arms to automate trailer unloading and package sorting. The robots are able to handle various package sizes with precision ensuring safety for workers and the products equally.
Watch our full webinar with Pickle Robot:
Data Challenges in Supply Chain Automation
Supply chain automation relies heavily on AI, robotics, and real-time data processing to optimize operations. However, managing and utilizing supply chain data presents several challenges. From unstructured data inefficiencies to fragmented systems, these issues can slow down automation efforts and impact the decision making process.
Unstructured Data Issues
Supply chain data comes from various sources like video feeds, IoT sensors, GPS tracking, and robotic systems. Unlike structured databases, this data is unorganized, complex, and difficult to process using existing systems.
But the AI models require structured, labeled datasets to function effectively, but supply chain environments generate raw, unstructured data that must be cleaned, annotated, and processed before use.
Also, since the supply chain data sources vary so much, the data modalities also vary. Hence, a reliable data processing platform is essential which can handle different modalities.
Example
Surveillance cameras in warehouses capture footage of package movements, but extracting meaningful insights such as detecting misplaced items or predicting equipment failures requires advanced models trained on well annotated video data.
Edge Cases & Variability
Warehouses and logistics hubs are highly dynamic environments where AI systems must handle unexpected conditions, such as:
- Irregular package sizes and shapes that may not fit standard sorting models.
- Unstructured warehouse layouts where items are moved manually, making tracking difficult.
- Environmental factors like poor lighting, dust, or obstructions that can impact AI vision systems.
Example
A robotic arm needs to be trained to pick all different shapes and sizes of boxes. Otherwise the arms would pick uniformly shaped boxes and may struggle when faced with irregular or damaged packages, leading to errors and delays.
Lack of High-Quality Labeled Data
Training AI models for supply chain automation requires large volumes of accurately labeled data. A process that is both time-consuming and expensive.
Data annotation for robotics and computer vision requires human expertise to label objects in warehouse environments e.g., differentiating between package types, identifying conveyor belt anomalies, or classifying damaged goods.Without high-quality annotated datasets, AI models struggle with real-world deployment due to poor generalization.
Example
A self-driving forklift needs detailed labeled data of warehouse pathways, obstacles, and human movement patterns to navigate safely—without this, its performance remains unreliable.
Data Silos and Fragmentation
Supply chain data is often stored in disconnected systems across different departments, vendors, and third-party logistics providers, making it difficult to get a unified view of operations.
Example
A warehouse may use one system for inventory tracking, another for shipment logistics, and a separate platform for robotic operations. Without integrating and connecting all of these systems, AI models cannot make real-time, data-driven decisions across the entire supply chain.
Improving Data for Effective Supply Chain Automation
High quality data helps build reliable AI models which is essential in supply chain automation. From unstructured data processing to better annotation workflows and system integration, improving data quality can significantly improve AI logistics.
Structuring Unstructured Data
The data in the supply chain pipeline comes from various sources and in large amounts. It is mainly unstructured and needs to be processed, annotated and in general converted into a usable format so that AI models can be trained on it. This will help the AI models to make accurate and automate the process.
Comprehensive data platforms like Encord help organize, label and extract valuable insights from video or sensor data.
Handling Edge Cases
AI models must adapt to unexpected warehouse conditions such as damaged packages, irregular stacking, or poor lighting. During data curation for building automated supply chain models, it is essential to curate a diverse and well balanced dataset. Annotation tools allow the teams to label complex scenarios and also visualize the whole dataset and help curate a balanced training data.
Efficient Data Annotation
AI models for supply chain automation need large, high-quality labeled datasets, but manual annotation is slow and costly. AI-assisted annotation speeds up labeling while ensuring accuracy. Data platforms like Encord help identify, label, and visualize warehouse data, enabling teams to curate balanced training datasets for improved AI performance.
Conclusion
Supply chain automation is revolutionizing how businesses manage logistics, warehouses, and transportation. AI, robotics, and real-time data analytics are improving the customer experience. However, bottlenecks such as unstructured data, edge cases, and fragmented systems must be addressed to access the automation’s full potential.
High-quality, structured data is essential for training reliable AI models. Advanced annotation tools and intelligent data management solutions streamline data labeling, improve model accuracy, and ensure seamless system integration. With the use of these data platforms like Encord, business processes can build smarter, more scalable automation tools for supply chains.
As automation adoption continues to grow, companies that effectively manage their data and AI workflows will gain a competitive edge. Future-ready supply chains will not only optimize efficiency but also enhance resilience, adaptability, and overall decision-making.
Power your AI models with the right data
Automate your data curation, annotation and label validation workflows.
Get startedWritten by

Alexandre Bonnet
- Supply chain automation refers to the use of AI, robotics, and data-driven systems to optimize logistics, warehousing, and transportation processes. It reduces manual labor, improves efficiency, and enhances decision-making in supply chain operations.
- AI helps by analyzing supply and demand patterns, optimizing inventory management, improving route planning, and automating quality control through computer vision and predictive analytics.
- Some major benefits include increased efficiency, reduced operational costs, improved accuracy, workforce optimization, better decision-making, and enhanced scalability to handle demand fluctuations.
- Companies face challenges such as handling unstructured data, managing edge cases, integrating fragmented systems, and obtaining high-quality labeled data for AI model training.
- Robots assist in picking, sorting, and packing goods, reducing errors and increasing processing speed. Automated storage and retrieval systems (AS/RS) further enhance warehouse efficiency.
- IoT sensors monitor inventory levels, track shipments, and provide real-time updates, ensuring transparency and reducing losses due to misplaced or delayed goods.
Explore our products