Deploy production AI faster
with leading AI teams
The most scalable way to manage, curate and annotate AI data
Transform petabytes of unstructured multimodal data into high quality data for training, fine-tuning, and aligning AI models, fast.
Trusted by pioneering AI Teams
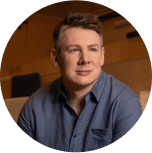
Nick Gillian
Founder & Head of AI
Archetype
“Encord brought together scalability, video-native annotation, clear label visibility, and the flexibility to support other modalities in a single, cohesive platform.”
Read case studyIndex and curate petabytes of data
Securely manage and organize millions of unstructured files with full visibility and traceability of data lineage.
Explore Index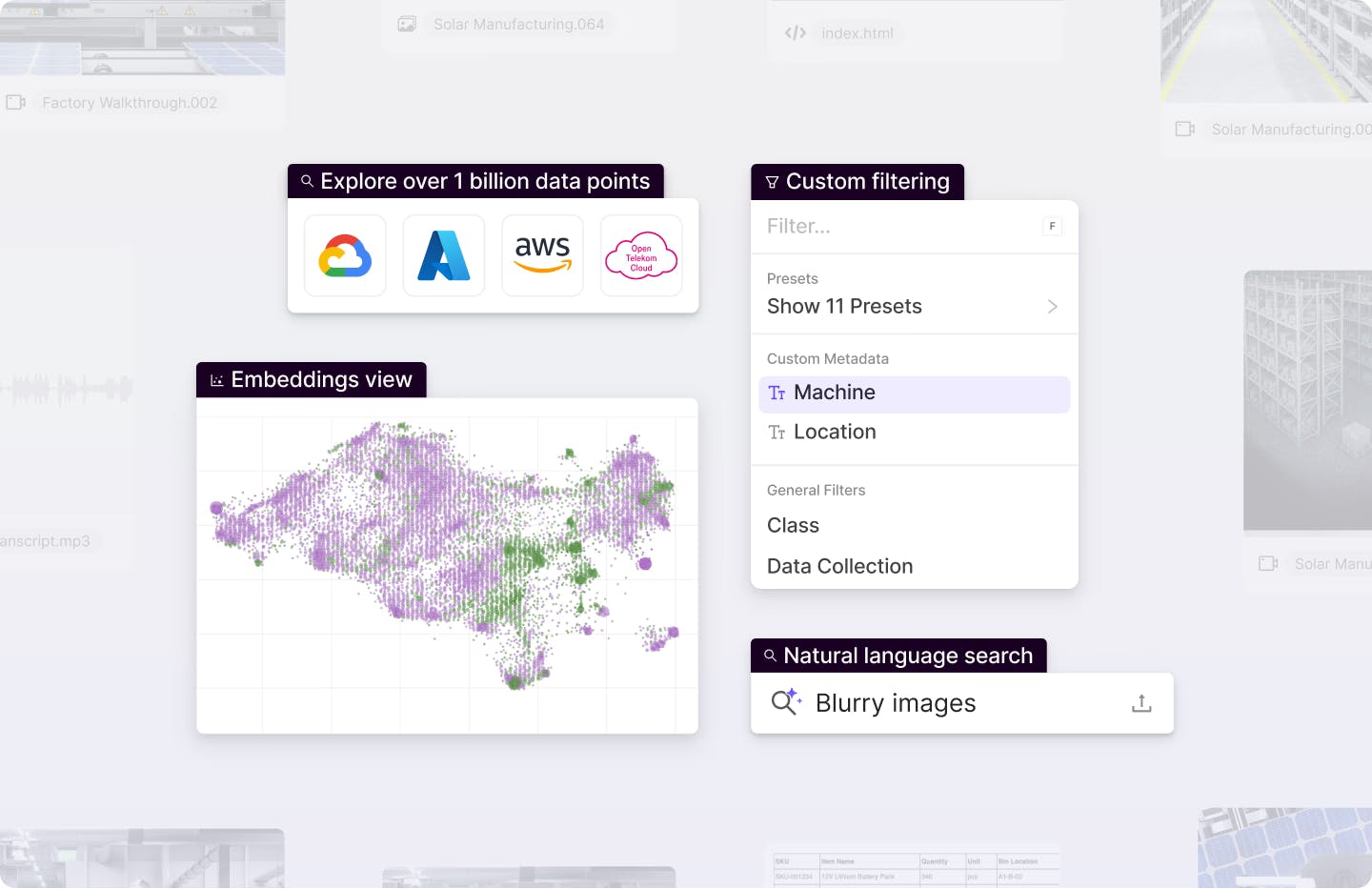
Generate multimodal labels at scale
Integrate AI agents into your project workflow for advanced model and human-in-the-loop labeling use cases.
Explore Annotate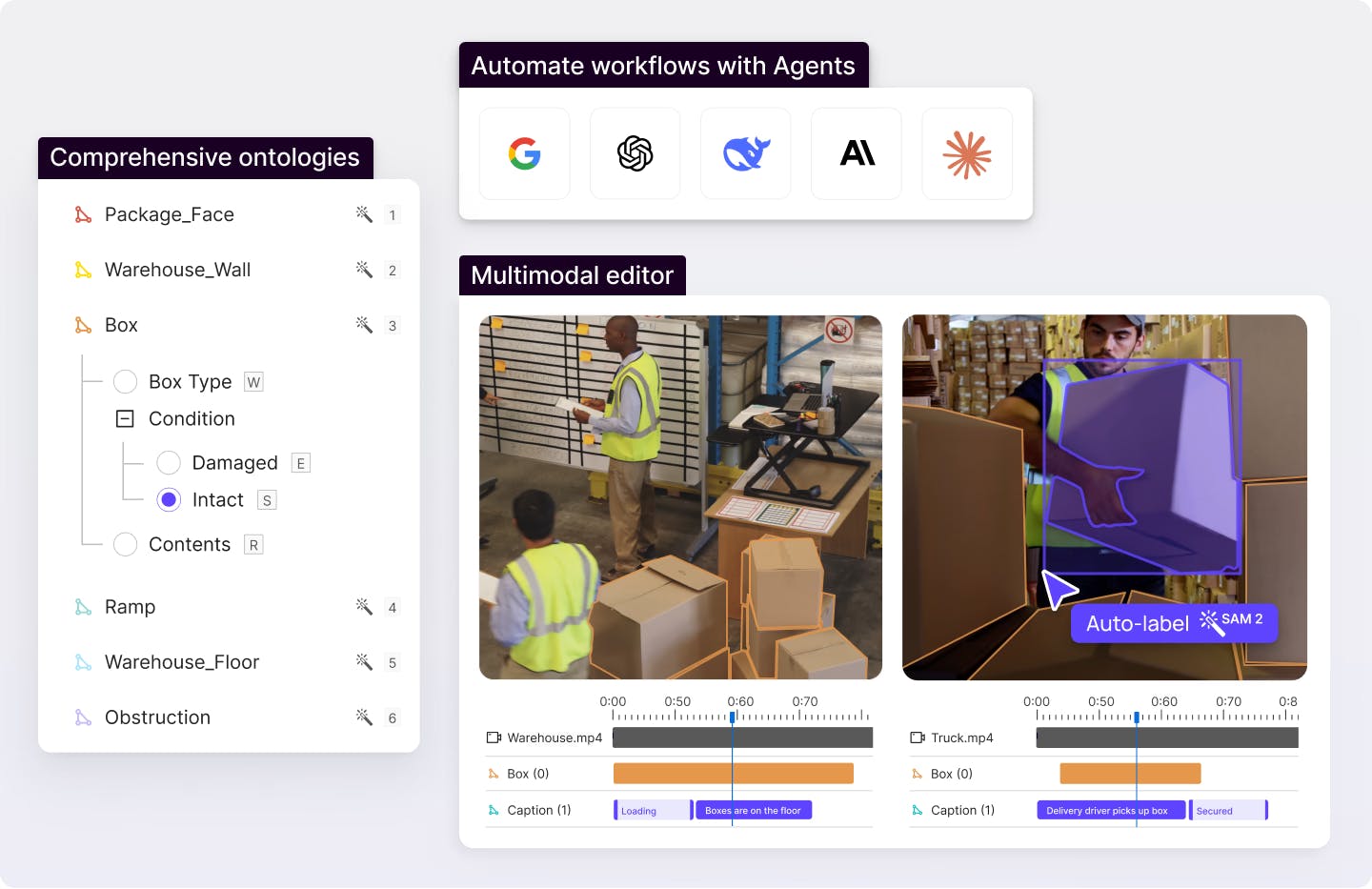
Align your AI models
Validate AI models against your data to surface, curate, and prioritize the most valuable data for training and fine-tuning.
Explore Active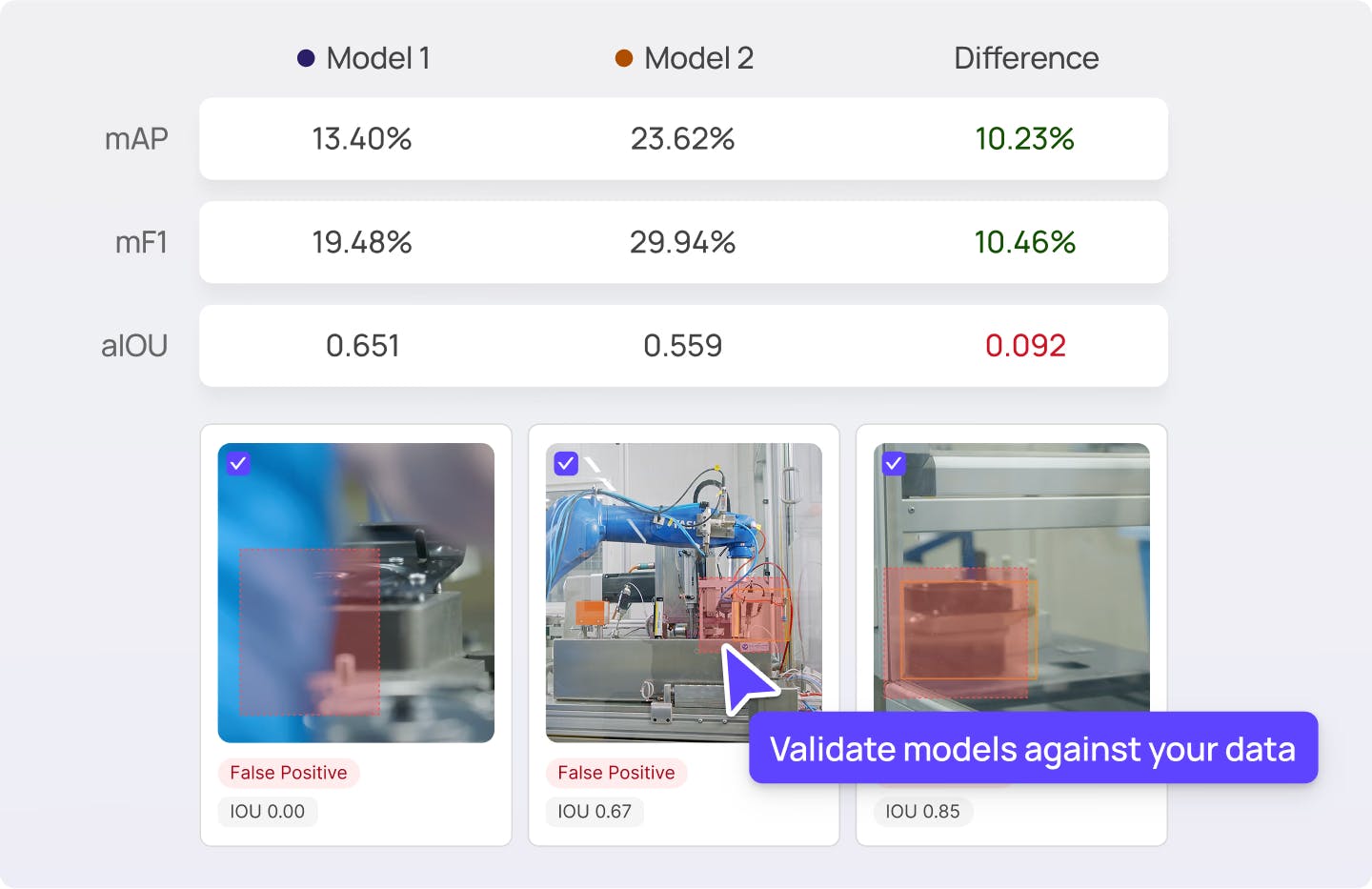
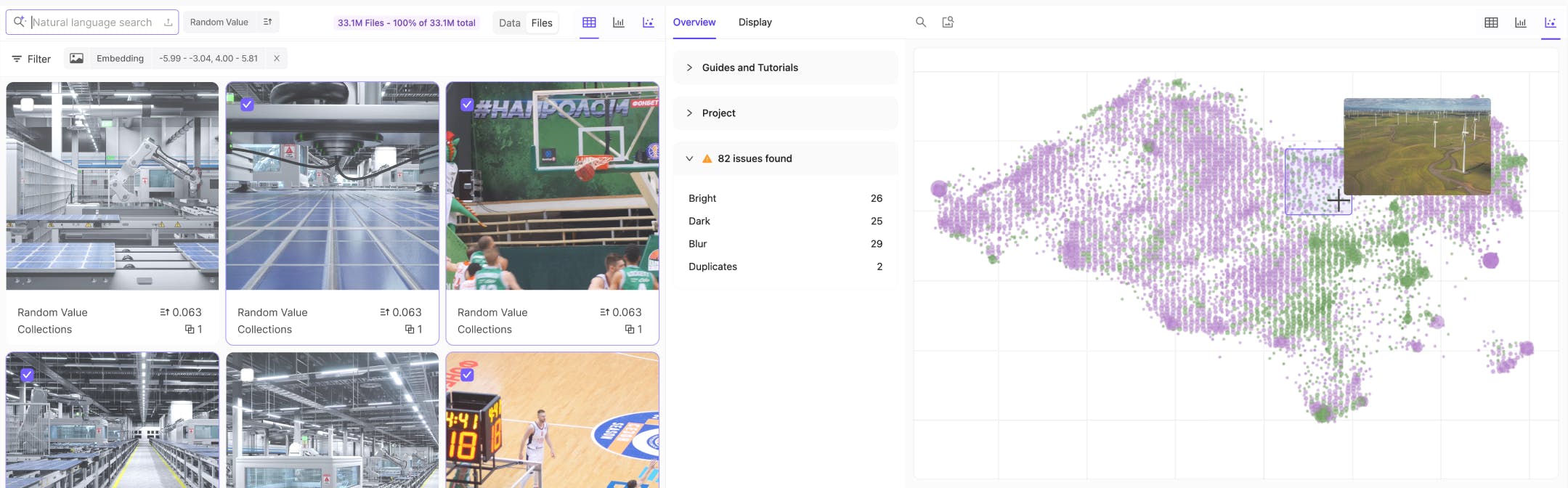
The unified data layer for AI development
Integrate your workflows
securely at </scale>
Connect your cloud storage, MLOps tools, and infrastructure through dedicated integrations. Access projects, datasets, and annotations via our API/SDK. Enterprise-grade security with SOC2, HIPAA, and GDPR compliance plus robust encryption standards.
Visit our Trust Center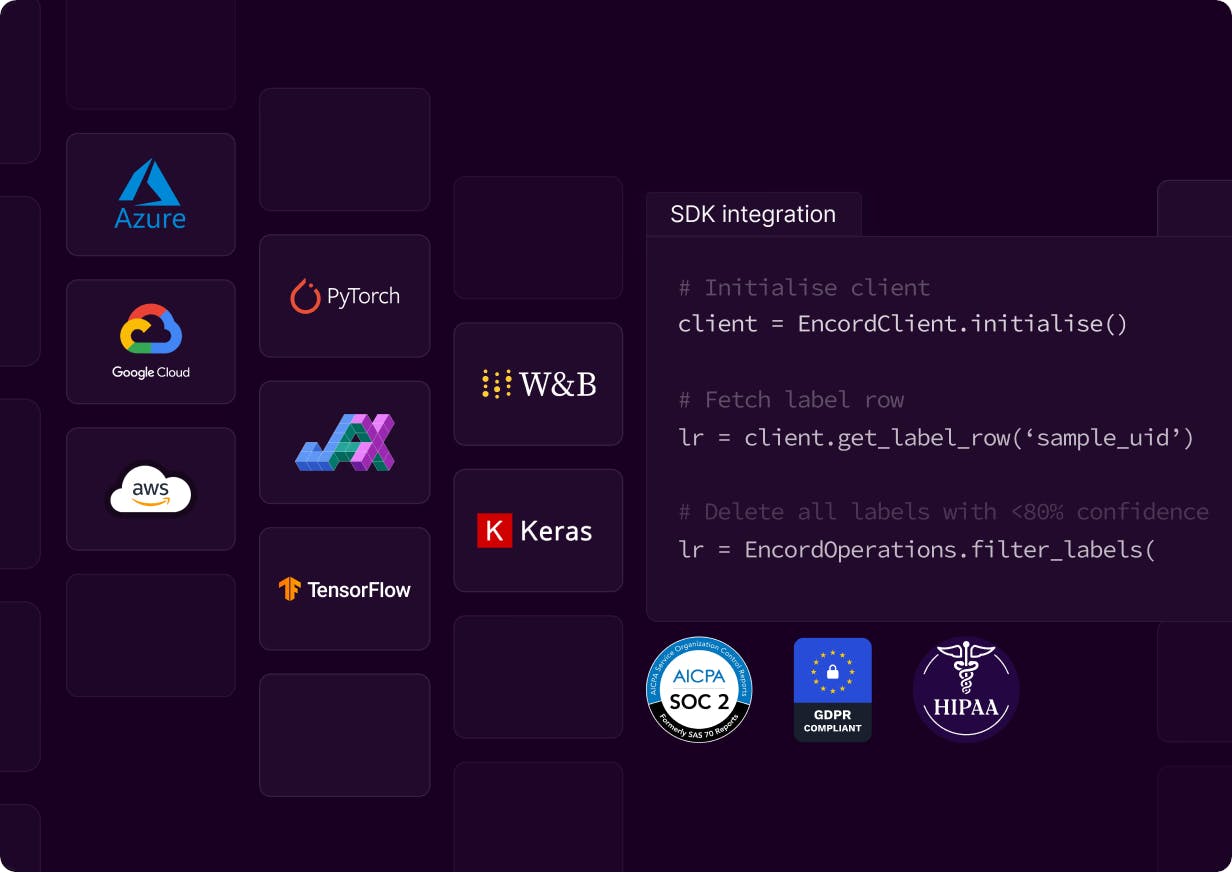
Trusted by 200+ of the world’s top AI teams deploying production AI
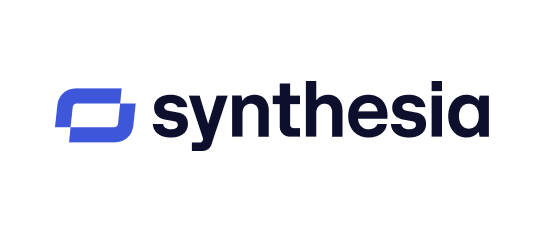
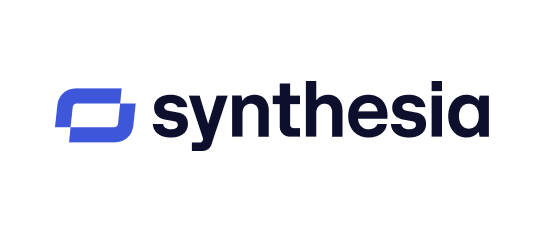
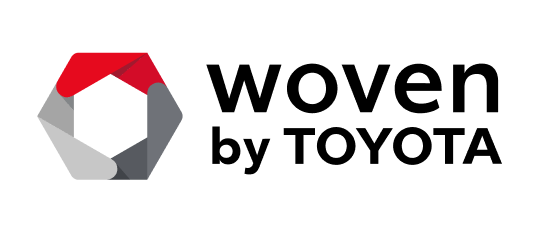
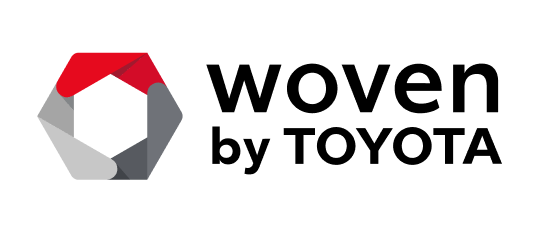
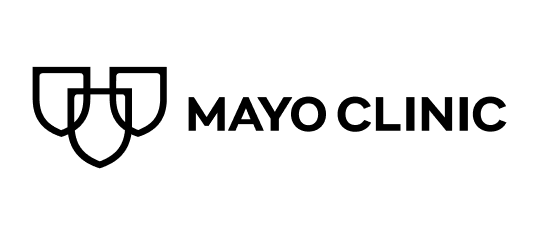
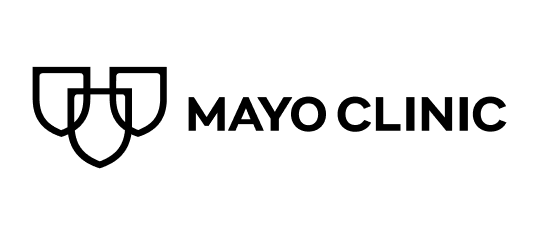
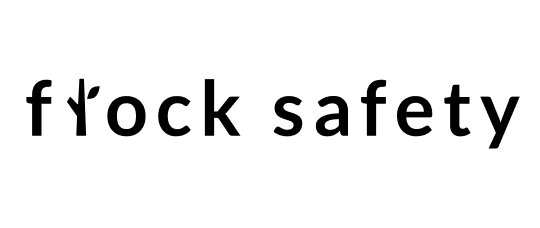
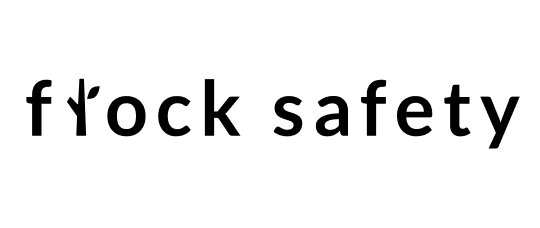
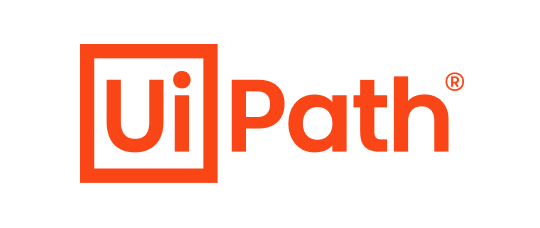
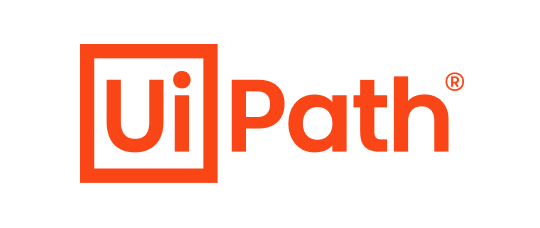
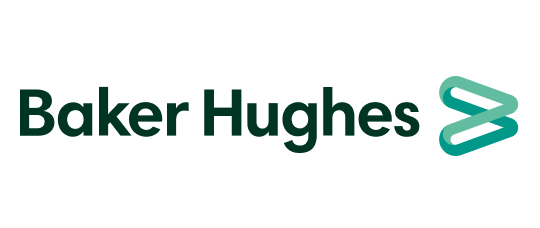
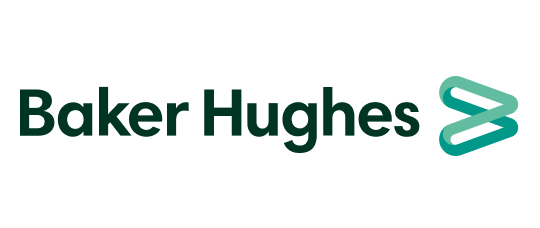
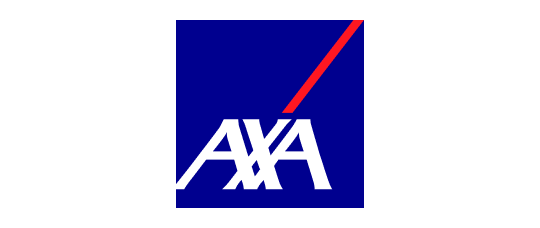
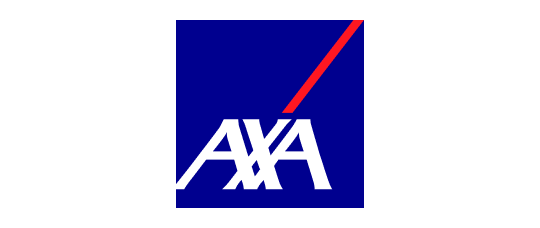
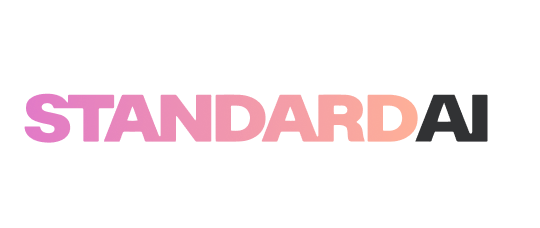
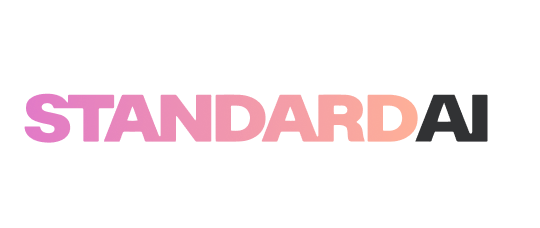
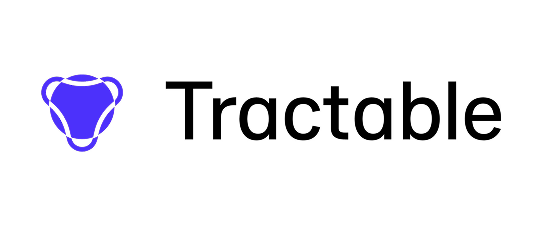
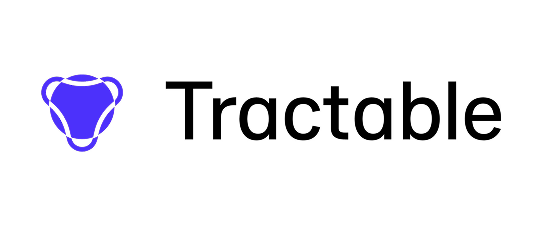
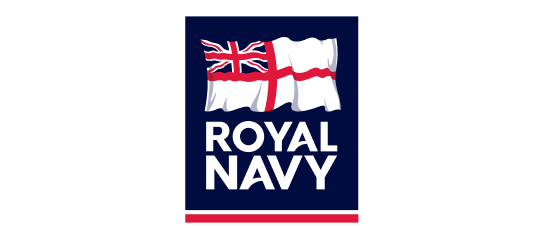
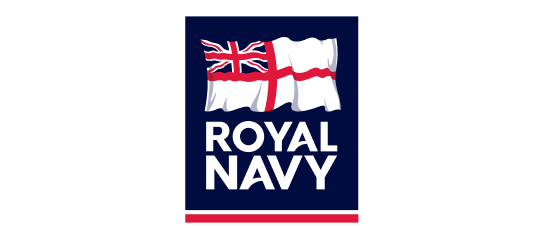
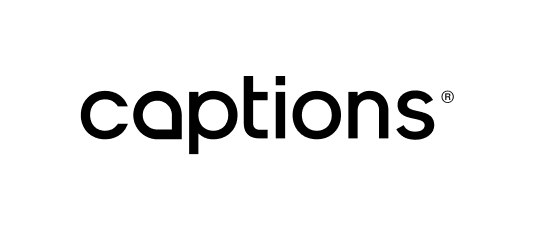
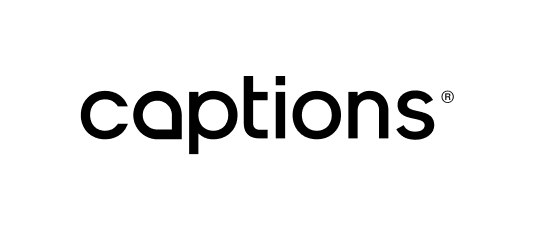
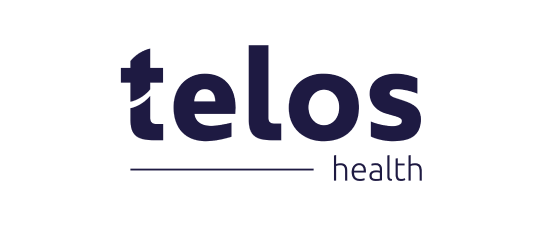
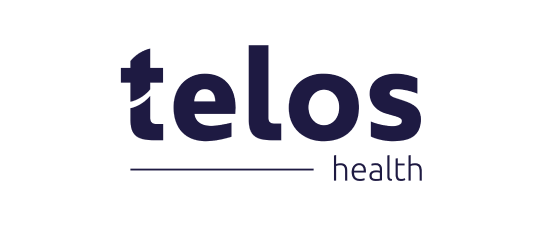
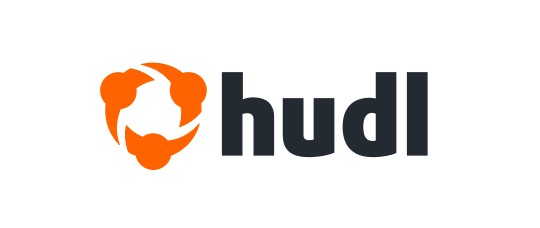
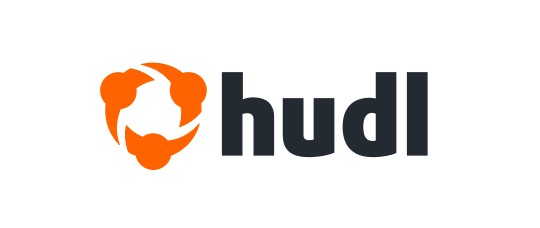
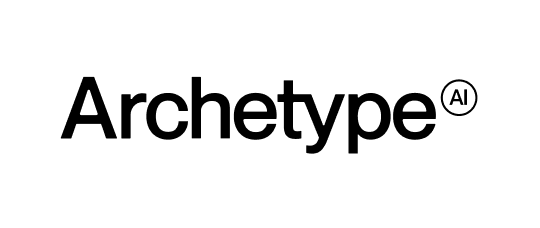
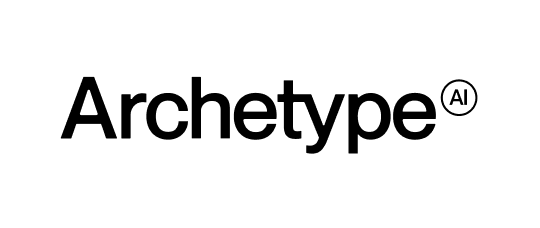
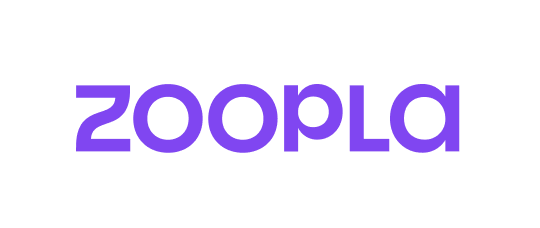
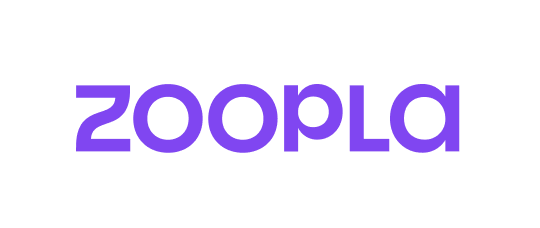
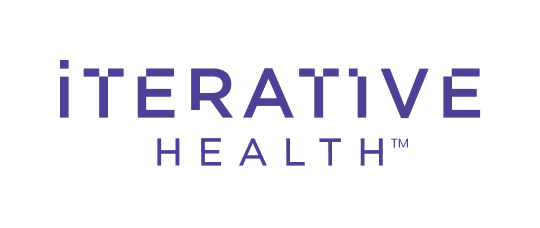
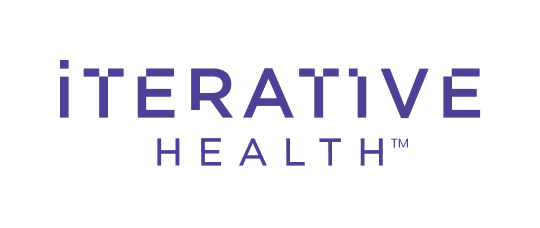
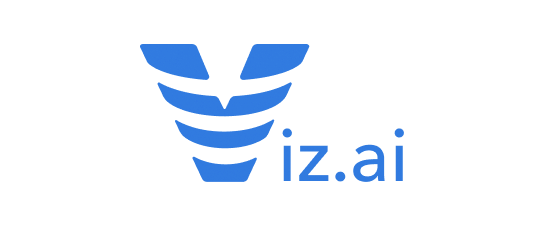
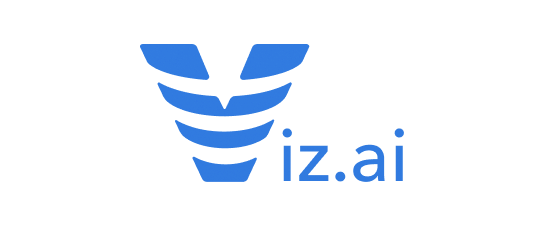
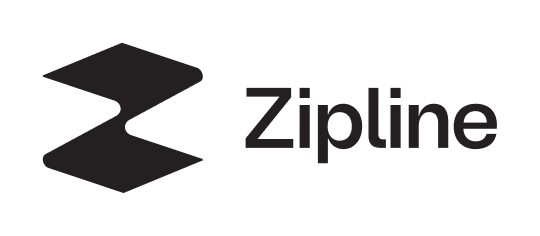
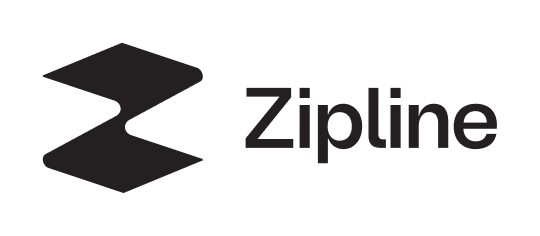
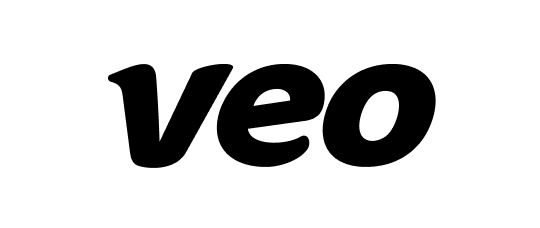
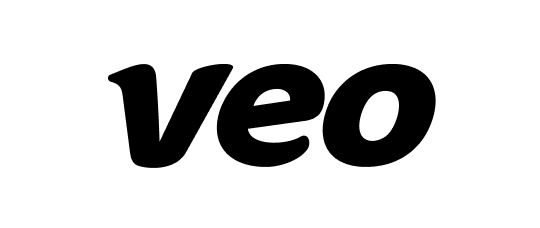
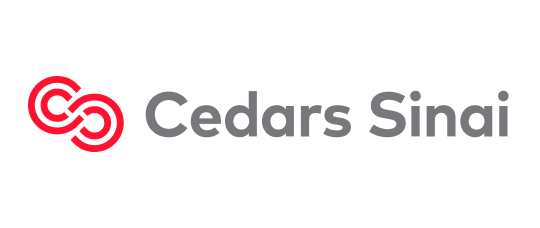
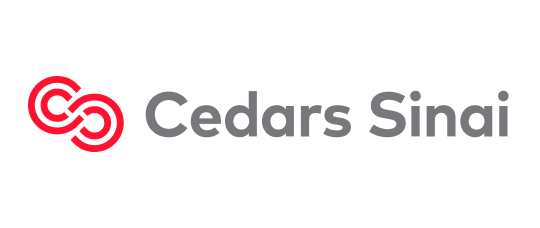
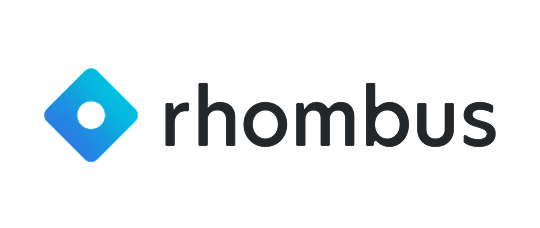
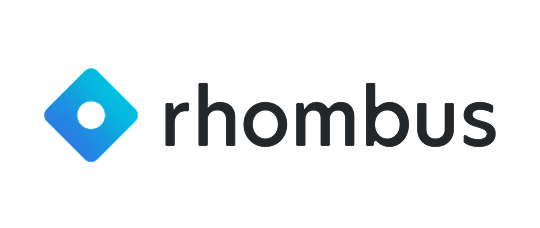
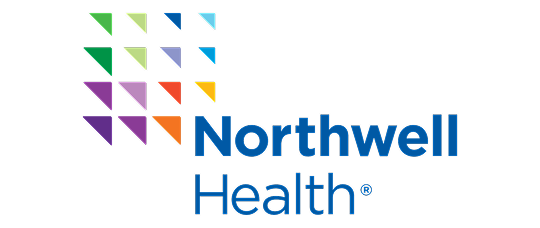
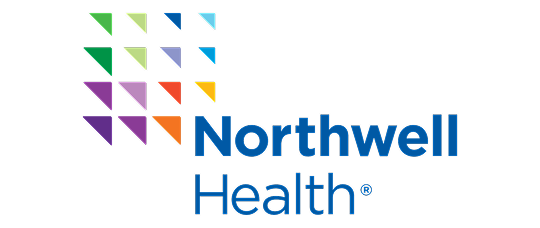
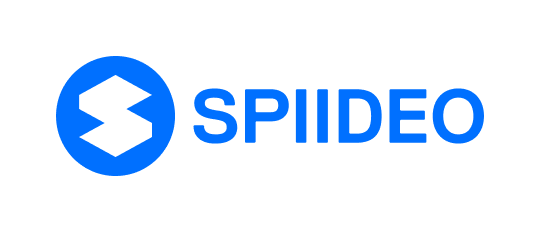
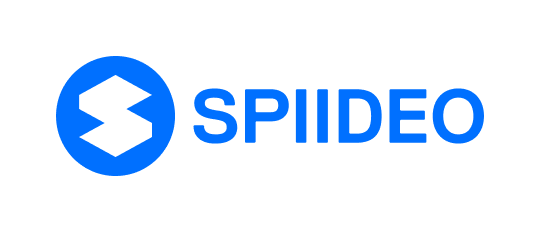
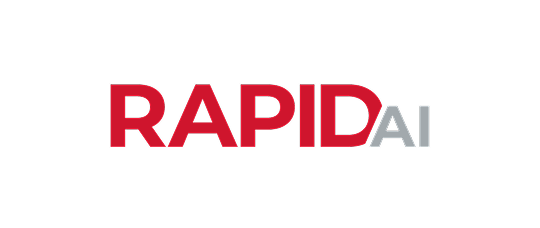
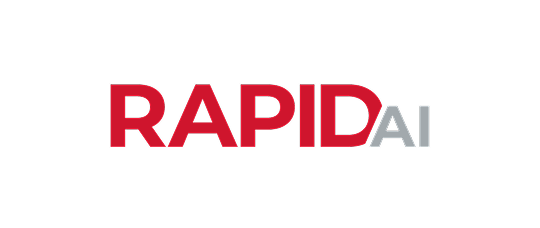