Encord Blog
Immerse yourself in vision
Trends, Tech, and beyond
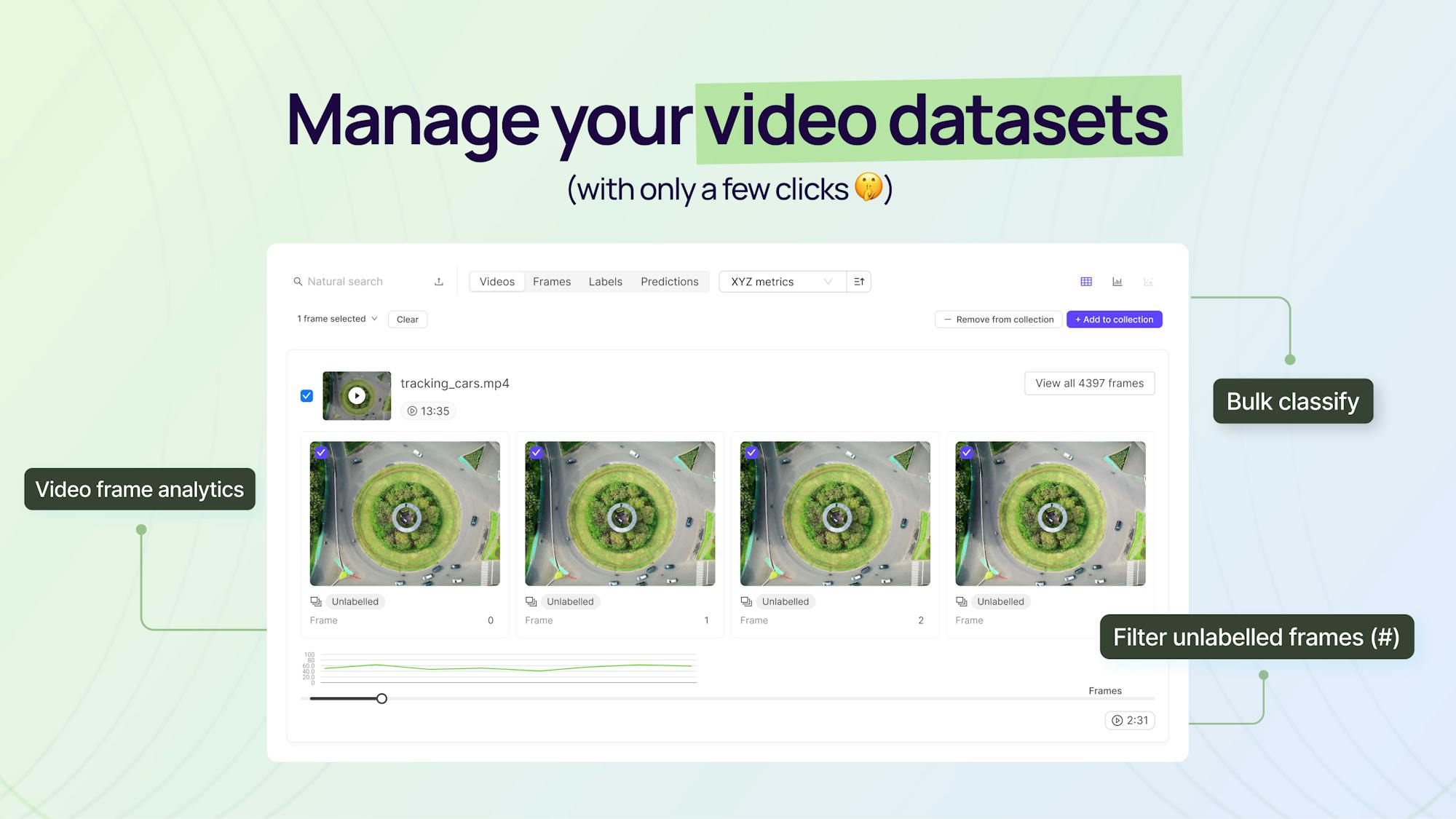
Announcing the launch of Advanced Video Curation
At Encord we continually look for ways to enable our customers to bring their models to market faster. Today, we’re announcing the launch of Video Data Management within the Encord Platform, providing an entirely new way to interact with video data. Gone are the days of searching frame by frame for the relevant clip. Now filter and search across your entire dataset of videos with just a few clicks. What is Advanced Video Curation? In our new video explorer page users can search, filter, and sort entire datasets of videos. Video-level metrics, calculated by taking an average from the frames of a video, allow the user to curate videos based on a range of characteristics, including average brightness, average sharpness, the number of labeled frames, and many more. Users can also curate within individual videos with the new video frame analytics timelines, enabling a temporal view over the entire video. We're thrilled that Video Data Curation in the Encord platform is the first and only platform available to search, query, and curate relevant video clips as part of your data workflows. Support within Encord This is now available for all Encord Active customers. Please see our documentation for more information on activating this tool. For any questions on how to get access to video curation please contact sales@encord.com.
Apr 24 2024
2 m
Trending Articles
1
Announcing the launch of Consensus in Encord Workflows
2
The Step-by-Step Guide to Getting Your AI Models Through FDA Approval
3
Best Image Annotation Tools for Computer Vision [Updated 2024]
4
Top 8 Use Cases of Computer Vision in Manufacturing
5
YOLO Object Detection Explained: Evolution, Algorithm, and Applications
6
Active Learning in Machine Learning: Guide & Strategies [2024]
7
Training, Validation, Test Split for Machine Learning Datasets
Explore our...
Meta’s Llama 3.1 Explained
Meta has released Llama 3.1, an open-source AI model that rivals the best closed-source models like OpenAI’s GPT-4o, Anthropic’s Claude 3, and Google Gemini in flexibility, control, and capabilities. This release marks a pivotal moment in democratizing AI development, offering advanced features like expanded context length and multilingual support. All versions of Llama 3.1 8B, 70B, and 405B are powerful models. With its state-of-the-art capabilities, it can unlock new possibilities in synthetic data generation, model distillation, and beyond. In this blog post, we'll explore the technical advancements, practical applications, and broader implications of Llama 3.1. Overview of Llama 3.1 Llama 3.1 405B is a frontier-level model designed to push the boundaries of what's possible with generative AI. It offers a context length of up to 128K tokens and supports eight languages, making it incredibly versatile. The model's capabilities in general knowledge, math, tool use, and multilingual translation are state-of-the-art, rivaling the best closed-source models available today. Llama 3.1 also introduces significant improvements in synthetic data generation and model distillation, paving the way for more efficient AI development and deployment. The Llama 3.1 collection also includes upgraded variants of the 8B and 70B models, which boast enhanced reasoning capabilities and support for advanced use cases such as long-form text summarization, multilingual conversational agents, and coding assistants. Meta's focus on openness and innovation ensures that these models are available for download and development on various platforms, providing a robust ecosystem for AI advancement. Overview of Previous Llama Models Llama 1 Released in early 2023, Llama 1 was Meta AI’s initial foray into large language models with up to 70 billion parameters. It laid the groundwork for accessible and customizable LLM models, emphasizing transparency and broad usability. Llama 2 Launched later in 2023, Llama 2 improved upon its predecessor with enhanced capabilities and larger models, reaching up to 70 billion parameters. It introduced better performance in natural language understanding and generation, making it a versatile tool for developers and researchers. Read more about it in our Llama 2 explainer blog. Importance of Openness in AI Meta’s latest release, Llama 3.1 405B, underscores the company’s unwavering commitment to open-source AI. In a letter, Mark Zuckerberg highlighted the numerous benefits of open-source AI, emphasizing how it democratizes access to advanced technology and ensures that power is not concentrated in the hands of a few. Advantages of Open-Source Models Unlike closed models, open-source model weights are fully accessible for download, allowing developers to tailor the model to their specific needs. This flexibility extends to training on new datasets, conducting, additional fine-tuning, and developing models invarious environments - whether in the cloud, on-premise, or even locally on laptop- without the need to share the data with the providers. This level of customization allows developers to fully harness the power of generative AI, making it more versatile and impactful. While some argue that closed models are more cost-effective, Llama 3.1 models offer some of the lowest cost per token in the industry, according to testing by Artificial Analysis. Read more about Meta’s commitment to open-source AI in Mark Zuckerberg’s letter Open Source AI is the Path Forward. . Technical Highlights of Llama 3.1 Model Specifications Meta Llama 3.1 is the most advanced open-source AI model to date. With a staggering 405 billion parameters, it is designed to handle complex tasks with remarkable efficiency. The model leverages a standard decoder-only transformer architecture with minor adaptations to maximize training stability and scalability. Trained on over 15 trillion tokens using 16,000 H100 GPUs, Llama 3.1 405B achieves superior performance and versatility. Performance and Capabilities Llama 3.1 405B sets a new benchmark in AI performance. Evaluated on over 150 datasets, it excels in various tasks, including general knowledge, steerability, math, tool use, and multilingual translation. Extensive human evaluations reveal that Llama 3.1 is competitive with leading models like GPT-4, GPT-4o, and Claude 3.5 Sonnet, demonstrating its state-of-the-art capabilities across a range of real-world scenarios. Source Source Multilingual and Extended Context Length One of the standout features of Llama 3.1 is its support for an expanded context length of up to 128K tokens. This significant increase enables the model to handle long-form content, making it ideal for applications such as comprehensive text summarization and in-depth conversations. Llama 3.1 also supports eight languages, enhancing its utility for multilingual applications and making it a powerful tool for global use. Model Architecture and Training Llama 3.1 uses a standard decoder-only transformer model architecture, optimized for large-scale training. The iterative post-training procedure, involving supervised fine-tuning and direct preference optimization, ensures high-quality synthetic data generation and improved performance across capabilities. By enhancing both the quantity and quality of pre- and post-training data, Llama 3.1 achieves superior results, adhering to scaling laws that predict better performance with increased model size. Source To support large-scale production inference, Llama 3.1 models are quantized from 16-bit (BF16) to 8-bit (FP8) numerics, reducing compute requirements and enabling efficient deployment within a single server node. Instruction and Chat Fine-Tuning Llama 3.1 405B excels in detailed instruction-following and chat interactions, thanks to multiple rounds of alignment on top of the pre-trained model. This involves Supervised Fine-Tuning (SFT), Rejection Sampling (RS), and Direct Preference Optimization (DPO), with synthetic data generation playing a key role. The model undergoes rigorous data processing to filter and balance the fine-tuning data, ensuring high-quality responses across all capabilities, even with the extended 128K context window. Read the paper: The Llama 3 Herd of Models. Real-World Applications of Llama 3.1 Llama 3.1’s advanced capabilities make it suitable for a wide range of applications, from real-time and batch inference to supervised fine-tuning and continual pre-training. It supports advanced workflows such as Retrieval-Augmented Generation (RAG) and function calling, offering developers robust tools to create innovative solutions. Some of the possible applications include: Healthcare: Llama 3.1’s multilingual support and extended context length are particularly beneficial in the medical field. AI models built on Llama 3.1 can assist in clinical decision-making by providing detailed analysis and recommendations based on extensive medical literature and patient data. For instance, a healthcare non-profit in Brazil has utilized Llama to streamline patient information management, improving communication and care coordination. Education: In education, Llama 3.1 can serve as an intelligent tutor, offering personalized learning experiences to students. Its ability to understand and generate long-form content makes it perfect for creating comprehensive study guides and providing detailed explanations on complex topics. An AI study buddy built with Llama and integrated into platforms like WhatsApp and Messenger showcases how it can support students in their learning journeys. Customer Service: The model’s enhanced reasoning capabilities and multilingual support can greatly improve customer service interactions. Llama 3.1 can be deployed as a conversational agent that understands and responds to customer inquiries in multiple languages, providing accurate and contextually appropriate responses, thereby enhancing user satisfaction and efficiency. Synthetic Data Generation: One of the standout features of Llama 3.1 is its ability to generate high-quality synthetic data. This can be used to train smaller models, perform simulations, and create datasets for various research purposes. Model Distillation: Llama 3.1 supports advanced model distillation techniques, allowing developers to create smaller, more efficient models without sacrificing performance. This capability is particularly useful for deploying AI on devices with limited computational resources, making high-performance AI accessible in more scenarios. Multilingual Conversational Agents: With support for eight languages and an extended context window, Llama 3.1 is ideal for building multilingual conversational agents. These chatbots can handle complex interactions, maintain context over long conversations, and provide accurate translations, making them valuable tools for global businesses and communication platforms. Building with Llama 3.1 Getting Started For developers looking to implement Llama 3.1 right away, Meta provides a comprehensive ecosystem that supports various development workflows. Whether you are looking to implement real-time inference, perform supervised fine-tuning, or generate synthetic data, Llama 3.1 offers the tools and resources needed to get started quickly. Accessibility Llama 3.1 models are available for download on Meta’s platform and Hugging Face, ensuring easy access for developers. Additionally, the models can be run in any environment—cloud, on-premises, or local—without the need to share data with Meta, providing full control over data privacy and security. Read the official documentation for Llama 3.1. You can also find the new Llama in Github and HuggingFace. Partner Ecosystem Meta’s robust partner ecosystem includes AWS, NVIDIA, Databricks, Groq, Dell, Azure, Google Cloud, and Snowflake. These partners offer services and optimizations that help developers leverage the full potential of Llama 3.1, from low-latency inference to turnkey solutions for model distillation and Retrieval-Augmented Generation (RAG). Source Advanced Workflows and Tools Meta’s Llama ecosystem is designed to support advanced AI development workflows, making it easier for developers to create and deploy applications. Synthetic Data Generation: With built-in support for easy-to-use synthetic data generation, developers can quickly produce high-quality data for training and fine-tuning smaller models. This capability accelerates the development process and enhances model performance. Model Distillation: Meta provides clear guidelines and tools for model distillation, enabling developers to create smaller, efficient models from the 405B parameter model. This process helps optimize performance while reducing computational requirements. Retrieval-Augmented Generation (RAG): Llama 3.1 supports RAG workflows, allowing developers to build applications that combine retrieval-based approaches with generative models. This results in more accurate and contextually relevant outputs, enhancing the overall user experience. Function Calling and Real-Time Inference: The model’s capabilities extend to real-time and batch inference, supporting various use cases from interactive applications to large-scale data processing tasks. This flexibility ensures that developers can build applications that meet their specific needs. Community and Support Developers can access resources, tutorials, and community forums to share knowledge and best practices. Community Projects: Meta collaborates with key community projects like vLLM, TensorRT, and PyTorch to ensure that Llama 3.1 is optimized for production deployment. These collaborations help developers get the most out of the model, regardless of their deployment environment. Safety and Security: To promote responsible AI use, Meta has introduced new security and safety tools, including Llama Guard 3 and Prompt Guard. These tools help developers build applications that adhere to best practices in AI safety and ethical considerations. Key Highlights of Llama 3.1 Massive Scale and Advanced Performance: The 405B version boasts 405 billion parameters and was trained on over 15 trillion tokens, delivering top-tier performance across various tasks. Extended Context and Multilingual Capabilities: Supports up to 128K tokens for comprehensive content generation and handles eight languages, enhancing global application versatility. Innovative Features: Enables synthetic data generation and model distillation, allowing for the creation of efficient models and robust training datasets. Comprehensive Ecosystem Support: Available for download on Meta’s platform and Hugging Face, with deployment options across cloud, on-premises, and local environments, supported by key industry partners. Enhanced Safety and Community Collaboration: Includes new safety tools like Llama Guard 3 and Prompt Guard, with active support from community projects for optimized development and deployment.
Jul 25 2024
5 M
The Python Developer's Toolkit for PDF Processing
PDFs (Portable Document Format) are a ubiquitous part of our digital lives, from eBooks and research papers to invoices and contracts. For developers, automating PDF processing can save time and boost productivity. 🔥Fun Fact: While PDFs may appear to contain well-structured text, they do not inherently include paragraphs, sentences, or even words. Instead, a PDF file is only aware of individual characters and their placement on the page.🔥 This characteristic makes extracting meaningful text from PDFs challenging. The characters forming a paragraph are indistinguishable from those in tables, footers, or figure descriptions. Unlike formats such as .txt files or Word documents, PDFs do not contain a continuous stream of text. A PDF document is composed of a collection of objects that collectively describe the appearance of one or more pages. These may include interactive elements and higher-level application data. The file itself contains these objects along with associated structural information, all encapsulated in a single self-contained sequence of bytes. In this comprehensive guide, we’ll explore how to process PDFs in Python using various libraries. We’ll cover tasks such as reading, extracting text and metadata, creating, merging, and splitting PDFs. Prerequisites Before diving into the code, ensure you have the following: Python installed on your system Basic understanding of Python programming Required libraries: PyPDF2, pdfminer.six, ReportLab, and PyMuPDF (fitz) You can install these libraries using pip: pip install PyPDF2 pdfminer.six reportlab PyMuPDF Reading PDFs with PyPDF2 PyPDF2 is a pure-python library used for splitting, merging, cropping, and transforming pages of PDF files. It can also add custom data, viewing options, and passwords to PDF files. Code Example Here we are reading a PDF and extracting text from it: import PyPDF2 def extract_text_from_pdf(file_path): with open(file_path, 'rb') as file: reader = PyPDF2.PdfReader(file) text = '' for page_num in range(len(reader.pages)): text += reader.pages[page_num].extract_text() return text # Usage file_path = 'sample.pdf' print(extract_text_from_pdf(file_path)) Extracting Text and Metadata with pdfminer.six pdfminer.six is a tool for extracting information from PDF documents, focusing on getting and analyzing the text data. Code Example Here’s how to extract text and metadata from a PDF: from pdfminer.high_level import extract_text from pdfminer.pdfparser import PDFParser from pdfminer.pdfdocument import PDFDocument def extract_text_with_pdfminer(file_path): return extract_text(file_path) def extract_metadata(file_path): with open(file_path, 'rb') as file: parser = PDFParser(file) doc = PDFDocument(parser) metadata = doc.info[0] return metadata # Usage file_path = 'sample.pdf' print(extract_text_with_pdfminer(file_path)) print(extract_metadata(file_path)) Creating and Modifying PDFs with ReportLab ReportLab is a robust library for creating PDFs from scratch, allowing for the addition of various elements like text, images, and graphics. Code Example To create a simple PDF: from reportlab.lib.pagesizes import letter from reportlab.pdfgen import canvas def create_pdf(file_path): c = canvas.Canvas(file_path, pagesize=letter) c.drawString(100, 750, "Hello from Encord!") c.save() # Usage create_pdf("test.pdf") To modify an existing PDF, you can use PyPDF2 in conjunction with ReportLab. Manipulating PDFs with PyPDF2 Code Example for Merging PDFs from PyPDF2 import PdfMerger def merge_pdfs(pdf_list, output_path): merger = PdfMerger() for pdf in pdf_list: merger.append(pdf) merger.write(output_path) merger.close() # Usage pdf_list = ['file1.pdf', 'file2.pdf'] merge_pdfs(pdf_list, 'merged.pdf') Code Example for Splitting PDFs from PyPDF2 import PdfReader, PdfWriter def split_pdf(input_path, start_page, end_page, output_path): reader = PdfReader(input_path) writer = PdfWriter() for page_num in range(start_page, end_page): writer.add_page(reader.pages[page_num]) with open(output_path, 'wb') as output_pdf: writer.write(output_pdf) # Usage split_pdf('merged.pdf', 0, 2, 'split_output.pdf') Code Example for Rotating Pages from PyPDF2 import PdfReader, PdfWriter def rotate_pdf(input_path, output_path, rotation_degrees=90): reader = PdfReader(input_path) writer = PdfWriter() for page_num in range(len(reader.pages)): page = reader.pages[page_num] page.rotate(rotation_degrees) writer.add_page(page) with open(output_path, 'wb') as output_pdf: writer.write(output_pdf) # Usage input_path = 'input.pdf' output_path = 'rotated_output.pdf' rotate_pdf(input_path, output_path, 90) Extracting Images from PDFs using PyMuPDF (fitz) PyMuPDF (also known as fitz) allows for advanced operations like extracting images from PDFs. Code Example Here is how to extract images from PDFs: import fitz def extract_images(file_path): pdf_document = fitz.open(file_path) for page_num in range(len(pdf_document)): page = pdf_document.load_page(page_num) images = page.get_images(full=True) for image_index, img in enumerate(images): xref = img[0] base_image = pdf_document.extract_image(xref) image_bytes = base_image["image"] image_ext = base_image["ext"] with open(f"image{page_num+1}_{image_index}.{image_ext}", "wb") as image_file: image_file.write(image_bytes) # Usage extract_images('sample.pdf') If you're extracting images from PDFs to build a dataset for your computer vision model, be sure to explore Encord—a comprehensive data development platform designed for computer vision and multimodal AI teams. Conclusion Python provides a powerful toolkit for PDF processing, enabling developers to perform a wide range of tasks from basic text extraction to complex document manipulation. Libraries like PyPDF2, pdfminer.six, and PyMuPDF offer complementary features that cover most PDF processing needs. When choosing a library, consider the specific requirements of your project. PyPDF2 is great for basic operations, pdfminer.six excels at text extraction, and PyMuPDF offers a comprehensive set of features including image extraction and table detection. As you get deeper into PDF processing with Python, explore the official documentation of these libraries for more advanced features and optimizations (I have linked them in this blog!). Remember to handle exceptions and edge cases, especially when dealing with large or complex PDF files.
Jul 17 2024
5 M
PPE Detection Using Computer Vision for Workplace Safety
Employees frequently become victims of fatal occupational accidents due to poor safety standards or protocols. Recent statistics reveal that around 340 million workplace accidents occur annually. The injuries result in severe consequences for the worker and their family while causing significant productivity losses for the company. One strategy to mitigate these incidents is implementing a strict policy of wearing personal protective equipment (PPE) when working in hazardous environments. This equipment can include protective glasses, high-visibility vests, gloves, and helmets to ensure the workers remain safe and productive in the long run. However, manually monitoring PPE compliance is challenging as it involves the daily supervision of an extensive workforce. An alternative is using the latest computer vision (CV)-based solutions and frameworks to determine whether workers wear PPE according to safety protocols. In this article, we will discuss the significance of PPE, compliance issues, the role and benefits of using CV to detect PPE for workplace safety, and a few implementation challenges. What is Personal Protective Equipment (PPE)? Organizations require on-site staff working on the factory floor or on construction sites to wear personal protective equipment (PPE) to enhance workplace safety. Multiple types of PPE include helmets, gloves, safety vests, hard hats, safety glasses, face shields, and respirators. PPE Types Protective gear ensures safe work environments in places where the chances of injuries or illness are high. Proper PPE usage also increases worker productivity in the long term, as workers can perform sensitive tasks safely without fear of accidents. In addition, companies can avoid costs and downtime associated with non-compliance by requiring workers to wear safety gear as part of national and international safety regulations. Issues in PPE Compliance Although complying with PPE standards offers significant benefits, organizations face multiple challenges in ensuring workers follow the stated PPE rules. The following list highlights three major issues in PPE compliance: Human Error and Oversight Workers may forget to wear PPE or deliberately neglect safety protocols due to discomfort. With different PPE items serving different purposes, workers may get confused as they may not know which equipment to use for which task type. This is likely to occur in workplaces with inadequate training on PPE equipment use. Manual Monitoring Limitations Monitoring workers manually to determine whether they use PPE is expensive and error-prone. Organizations must hire and train supervisors who can miss non-compliance instances in large and complex environments. Impact of Non-compliance on Safety and Costs Non-compliance can cause higher injury rates, worker absences, and turnover. Workers’ deteriorating health can also increase operational costs involving medical expenses, legal liabilities, and penalties. Role of Computer Vision in PPE Detection Advancements in artificial intelligence (AI) algorithms allow organizations to quickly implement cutting-edge computer vision technology to automate personal protective equipment detection. Let’s understand how computer vision helps build an efficient PPE detection system. What is Computer Vision? Computer vision techniques allow machines to process and analyze visual datasets such as images and videos, to extract relevant data patterns. It uses AI algorithms to perform multiple tasks, such as image classification, object detection, and segmentation. Organizations in several domains use CV to automate workflows and minimize operational costs. For instance, computer vision algorithms help diagnose medical images in healthcare, detect product anomalies in manufacturing, identify objects on the road for self-driving cars, and optimize inventory management in warehouses. See Also: What Is Computer Vision in Machine Learning. How Computer Vision Enhances PPE? Since CV allows users to analyze visual data, it can improve PPE detection by mitigating the challenges discussed earlier. Here are a few ways CV achieves this: Real-time Monitoring and Detection: CV systems can automatically detect PPE in real-time and ensure workers use it according to safety requirements. Reduction of Human Error: With advanced model training, CV frameworks can work autonomously, reducing the risk of human error and quickly identifying non-complying behavior. Automation of Compliance Checks: CV-based detection can reduce administrative burdens by quickly generating alerts in case of PPE violations and streamlining enforcement workflows. It can send periodic reports and notifications to relevant authorities with relevant data on PPE usage. Technical Components of PPE Detection Systems Before implementing a PPE detection system, organizations must invest in relevant hardware and software components to allow the system to function efficiently. Below are the key requirements for implementing a PPE detection mechanism. Hardware Requirements While precise hardware requirements vary according to the system deployed, critical elements include cameras, sensors, and edge computing devices. Cameras and Sensors: CV systems require high-resolution cameras and sensors to capture images and video feeds from multiple angles and locations. They must also be robust to environmental disturbances and perform in harsh conditions, such as withstand high or low temperatures in factory sites. Edge Computing Devices: While cameras and sensors capture relevant data, edge computing devices help process, analyze, and transfer data to enterprise platforms. NVIDIA Jetson Orin The devices include small but powerful single-board computing units like NVIDIA’s Jetson or Raspberry Pi. Raspberry Pi M.2 Hat+ Users can integrate these modules into cameras and sensors for on-site real-time accelerated computations. See Also: Vision-based Localization: A Guide to VBL Techniques for GPS-Denied Environments. Software Requirements CV systems require advanced deep learning algorithms that can process images with the latest techniques for accurate predictions. Organizations must also invest in platforms that help them smoothly integrate their existing infrastructure with CV frameworks. Deep Learning Models: PPE systems need object detection models such as You Only Look Once (YOLO) or Faster Region-based Convolutional Neural Network (Faster-RCNN) to recognize PPE gear. Image Processing Techniques: The techniques include pipelines that process raw data for use by detection models. Data processing steps may include transformation, normalization, and augmentation. Integration with AI and Machine Learning Frameworks: An efficient detection system requires integrating CV models with machine learning platforms such as TensorFlow, PyTorch, and OpenCV to streamline the development, validation, and deployment process. Implementation of PPE Detection Systems The next step, once you meet the necessary hardware and software requirements, is implementing a PPE detection system. Although implementation can be complex, it usually requires three steps: data collection and annotation, model training and validation, deployment, integration, and continuous operations. Data Collection and Annotation Efficient model training requires users to collect and curate extensive datasets with accurate annotations. Below are a few guidelines to help companies quickly collect and annotate data. Data Collection Organizations must train object detection models on diverse datasets with multiple PPE types. This process ensures that the algorithm recognizes all the varieties of PPE to detect non-compliance accurately. The dataset must also include images and videos with different lighting conditions, backgrounds, angles, locations, and other obstacles. The method will ensure the model recognizes PPE gear in complex and dynamic environments. Users can collect such data using cameras, sensors, and public image repositories. Using generative AI algorithms, they can also generate synthetic data that resembles real images. They can use tools such as Encord Index, which helps register, curate, and manage multiple data types, including metadata. With Index, you can import data from cloud sources or local storage. You can also search, sort, and filter your datasets to: Identify patterns, trends, or anomalies within a subset of the data. Detect duplicates, outliers, and data inconsistencies. Remove irrelevant, noisy, and erroneous data. After filtering, sorting, and searching your data, use Collections with Annotate Projects to streamline your annotation process or send your dataset downstream for processing and training/fine-tuning models. Encord Index For instance, you can filter data according to the amount of blur in images. The filtered dataset will contain blurred images that are unsuitable for model training. You can extract this data subset and process it further to remove blurs and other inconsistencies. See Also: Learn more about object detection in our detailed blog. Data Annotation The next step is data annotation. Annotation involves labeling images and video clips to describe their content. Accurate annotation will help the model recognize which PPE is present in an image or video. Although users can perform annotation manually, this method is tedious and error-prone. A better alternative is to use annotation platforms that contain relevant features and methods to help automate the annotation process. For instance, Encord Annotate is an AI-assissted annotation platform that lets you label images and videos using customizable workflows and collaborative features. It supports all the latest annotation methods, including bounding boxes, polygons, polylines, key points, and segmentation. Encord Annotate Encord Annotate also includes micro-models and automated labeling features to customize annotations to your specific datasets and use cases. Users can train micro-models on relevant datasets and use them to label images across different projects. Automated labeling includes segment anything model (SAM), interpolation, and object tracking methods to speed up annotation by filling in missing labels using information from manual annotations. See Also: The Full Guide to Automated Data Annotation. Data Curation Once the annotation is complete, developers must curate data by organizing the data assets into relevant categories, filtering outliers, and fixing anomalies such as blurred or unclear images. Curation may also involve data anonymization to ensure compliance with privacy regulations. Creating metadata is also helpful, as it can allow new developers and annotators to understand the context and purpose of data items quickly. Data Pre-processing The next is data pre-processing, which may involve image resizing, normalization, noise reduction, and determining the optimal train-test split for model training and validation. Data pre-processing helps in creating a clean dataset that models can readily use to extract meaningful data patterns. Automated pipelines can speed up the process and reduce errors. Feature Engineering After obtaining a clean dataset, the next step is to extract relevant features from the image data to improve model performance and reduce computational complexity. The process involves identifying unique textures that distinguish PPE equipment, analyzing color distributions, assigning key points to help the model recognize corners and edges, and highlighting different backgrounds and environmental conditions. Recommended Read: Mastering Data Cleaning & Data Preprocessing. Model Training and Validation After processing data, the next stage is training and validating a model. Training involves a model learning data patterns to generate accurate predictions, while validation tests model performance on unseen data. Model Training Training involves techniques that feed annotated data into a model to help it learn underlying data patterns for predicting outcomes. In the context of PPE detection, the model will learn to recognize different PPE and predict accurate labels for images containing a particular PPE type. The process requires extensive computing power and expertise to train a model on a large dataset with correct hyperparameters. Modern methods involve fine-tuning foundation models such as YOLO-World, GroundingDINO, Single Shot MultiBox Detector (SSD), and Detection Transformer (DETR) to perform domain-specific detection tasks for better performance. These pre-trained models possess extensive knowledge regarding multiple items, giving them superior generalization ability on new datasets. Experts can repurpose these models for PPE detection use cases, allowing them to reduce training time and cost. Model Validation While training ensures the model accurately predicts image labels from known data, validation checks if the model performs equally well on a new dataset. It involves computing multiple metrics, such as accuracy, precision, recall, and F1-score, to determine whether model predictions are reliable. Although you can compute these metrics manually using custom pipelines, a more systematic approach is to use a platform that automatically computes multiple metrics to assess performance. The approach ensures quick, consistent, and accurate calculations while offering more comprehensive insights into model performance. Encord Active evaluates model performance based on relevant metrics and offers insightful visualizations to help understand how well the model can detect PPE when deployed on sensors or devices in the workplace. Encord Active Encord Active features metrics to measure label, data, video, and model quality. These features help you analyze the problem and debug model quality issues in cases of poor model performance. Deployment and Integration The next stage is deploying the trained models in production and integrating them with safety management systems to track compliance. Deploying Models on Edge and Non-Edge Devices Organizations can deploy models on edge devices for on-site real-time processing. For instance, cameras integrated with NVIDIA Jetson chips can detect PPE use locally and send usage statistics to relevant authorities. The method improves prediction accuracy and speed through parallel processing. Organizations can also integrate these devices with cloud storage solutions for enhanced security and scalability. Alternatively, companies can use on-premises central servers to process data coming from camera feeds in real-time. Such systems offer more computational power than edge devices and allow for more scalability. Also, multiple models can share the server resources, making it more cost-effective. Additionally, a central team can manage upgrades and maintenance routines more efficiently. Overall, the choice of edge or non-edge infrastructure depends on specific use-case. Edge solutions are more effective in cases where privacy is a significant concern and limited finance makes it challenging to invest in extensive on-site infrastructure. Conversely, non-edge solutions (e.g., Cloud platforms) are helpful in cases where the system requires high computational power and latency is not an issue. Integration with Existing Safety Management Systems Integrating these solutions with a safety management platform is necessary for an end-to-end compliance monitoring system. For instance, the organization can define usage thresholds and use automated pipelines that compare actual usage with pre-defined targets. The system can automatically generate alerts that notify management where and when non-compliance occurs. Continuous Operations The last stage, after deployment and integration, concerns continuous operations. The phase involves monitoring performance and implementing regular upgrades to ensure the system’s accuracy and reliability. A few ways to keep the detection system up-to-date and working according to expectations include regular model training on new datasets, installing device software updates, establishing real-time alerts to quickly identify issues, and monitoring key performance indicators (KPIs) against targets to track compliance. Recommended Read: A Guide to Machine Learning Model Observability. Case Study Let’s see how a company can implement an end-to-end detection system to overcome safety and compliance challenges. The Problem A construction company wants to build a PPE detection system to monitor PPE compliance but faces some of the challenges below. Construction sites have dynamic illumination levels, shadows, multiple activities, and several PPE types with different colors and designs. These factors make implementing a PPE detection system challenging. Dynamic illumination levels, for instance, affect the visibility of PPE items at different sites during the day, and shadows from other objects, such as construction equipment, can obscure the primary PPE gear. Similarly, the multitude of activities on construction sites makes it difficult for a PPE detection system to analyze and detect all movements, and the variety of PPE items hinders the detection model’s ability to identify a particular PPE item accurately. The challenges call for a robust detection system that can generalize well to new objects and accurately detect occluded items in crowded environments. The steps below demonstrate how the company can successfully deploy such a detection framework using state-of-the-art equipment and an object detection model. 1. Data Collection and Annotation The company can use customized 2D cameras instead of using an off-the-shelf device to ensure the hardware is suitable for dynamic construction sites. Camera set-up Each camera can include a power, processing, and data storage unit. Since construction sites often have dust, sunlight, rain, and heavy equipment, the company can cover the devices with plastic boxes and place them on top of tripods to ensure the cameras capture a wide angle from different locations. The cameras can record 41-second video clips and send the data to a cloud browser. It can use solar power to run the devices, since finding an uninterrupted electrical supply on a construction site is challenging. Finally, the company can use automated data curation and labeling tools to curate and annotate images extracted from video clips. For instance, Encord Index can help organize the images into relevant categories and allow developers to identify data issues such as occulsions, blur, low resolution, and inadequate brightness. Encord Index natively integrates with Encord Annotate to help developers annotate the curated data using automated features to speed up the labeling process. They can also use the latest labeling methods to manually mark key points and draw bounding boxes around PPE equipment. Additionally, Encord’s support for video annotation can streamline annotation more effectively, as developers can directly label relevant objects in video clips for more accurate results. 2. Data Pre-processing and Feature Engineering After annotation, developers can apply relevant data transformation using automated pipelines. The transformations can include image resizing, normalization, and augmentation. Also, they must extract features that differentiate one PPE equipment from the other. Such features may include the equipment’s shape, size, edges, corners, and colors. Lastly, it must determine an appropriate train-test split to train, test, and validate the model. The size of the data will help decide a suitable split. 3. Model Training and Validation The next stage is selecting a suitable model to train and validate. A cost-effective approach is to fine-tune a visual foundation model (VFM) using the data extracted in the previous stage. Once trained, developers can validate the model using relevant metrics, such as identification and recall rates. Encord Active automatically computes multiple metrics to measure performance with intuitive visualizations. See Also: Webinar: Are Visual Foundation Models (VFMs) on par with SOTA? 4. Model Deployment and Integration The company can use multiple frameworks to build, train, and deploy the model. Popular open-source frameworks include TensorFlow, PyTorch, and Scikit-Learn. Additionally, it can integrate the system with a safety management framework to send real-time PPE compliance data to notify and alert authorities in case of anomalies. 5. Continuous Operations Lastly, the company can build real-time alert mechanisms that can quickly identify issues and establish KPIs with achievable targets to measure ongoing performance. Benefits of CV in PPE Detection As the above case study highlights, using CV for PPE detection offers multiple benefits over a manual system. Below are a few key benefits of CV-based PPE detection. Efficiency: A CV-based system captures non-compliance instances more efficiently through real-time monitoring and instant alerts regarding non-complying behavior. Scalability: Organizations can install multiple edge devices to cover many locations and detect PPE compliance. The method allows for monitoring all the employees simultaneously. Data Analytics: CV systems can process and analyze extensive data to help managers track PPE usage statistics. The analysis may reveal actionable insights regarding safety trends and employee pain points. Remote Support: Safety officers can monitor multiple sites from a central location and provide instant support to remote locations. Implementation Challenges Although using CV for PPE detection offers numerous advantages, it is challenging to implement in complex and dynamic environments. The list below highlights a few hurdles and mitigation strategies that organizations may follow during implementation. Technical Challenges The most significant issue is PPE variety, as the PPE detection model must recognize multiple PPE types with varying colors, designs, and shapes. Collecting, storing, and processing a diverse dataset that includes all variations in dynamic environments is challenging. Organizations can address the problem by adopting data augmentation techniques to diversify the dataset. They can also experiment with data generation methods to create synthetic data for better model training. Lastly, they can strategically place cameras in different locations with occlusion and poor lighting conditions to help the model recognize patterns in low-visibility areas. Privacy and Ethical Considerations Real-time PPE detection requires constant monitoring of employees through cameras. The method may result in workers feeling uneasy and having privacy concerns. With rising privacy regulations, implementing visual detection systems becomes more challenging as organizations must ensure they are complying with international standards. Companies can establish strict data privacy guidelines and obtain employee consent before monitoring workers. They can also use anonymization techniques to build training datasets. Scalability and Maintenance Scaling and maintaining PPE detection systems across different environments can be challenging. The issue requires tailored solutions that suit specific locations, evolving safety rules, new PPE types, and changing conditions as the business expands. Businesses can mitigate these concerns by scheduling regular updates and using automated tools to streamline model training with new data. They must also implement systems with modularity to ensure quick upgrades and use APIs to integrate seamlessly with new safety management systems. PPE Detection Using Computer Vision: Key Takeaways Computer vision (CV) offers a more scalable and cost-effective way for PPE detection to ensure workplace safety. Below are a few critical points regarding CV-based PPE detection systems. Technical Components of CV-based PPE Detection System: PPE detection systems require suitable hardware and software components. Appropriate hardware includes cameras, sensors, and edge-computing devices. Software elements include a scalable CV model, image processing pipelines, and solutions for integrating AI systems with deployment platforms. Implementation Steps: The process begins with collecting and annotating diverse data. The next step is model training and validation, with model deployment and integration with the safety management system being the last step. Implementation Tools: Organizations can streamline the implementation process through AI solutions that help with data collection, annotation, and model validation. Encord is an end-to-end data development platform that helps you curate and annotate large datasets. It also supports model validation through an extensive range of data and model quality metrics and AI-assisted model debugging features. So, sign up for Encord now to optimize PPE detection and improve workplace safety.
Jul 16 2024
5 M
Top 10 Multimodal Models
The current era is witnessing a significant revolution as artificial intelligence (AI) capabilities expand beyond straightforward predictions on tabular data. With greater computing power and state-of-the-art (SOTA) deep learning algorithms, AI is approaching a new era where large multimodal models dominate the AI landscape. Reports suggest the multimodal AI market will grow by 35% annually to USD 4.5 billion by 2028 as the demand for analyzing extensive unstructured data increases. These models can comprehend multiple data modalities simultaneously and generate more accurate predictions than their traditional counterparts. In this article, we will discuss what multimodal models are, how they work, the top models in 2024, current challenges, and future trends. What are Multimodal Models? Multimodal models are AI deep-learning models that simultaneously process different modalities, such as text, video, audio, and image, to generate outputs. Multimodal frameworks contain mechanisms to integrate multimodal data collected from multiple sources for more context-specific and comprehensive understanding. In contrast, unimodal models use traditional machine learning (ML) algorithms to process a single data modality simultaneously. For instance, You Only Look Once (YOLO) is a popular object detection model that only understands visual data. Unimodal vs. Multimodal Framework While unimodal models are less complex than multimodal algorithms, multimodal systems offer greater accuracy and enhanced user experience. Due to these benefits, multimodal frameworks are helpful in multiple industrial domains. For instance, manufacturers use autonomous mobile robots that process data from multiple sensors to localize objects. Moreover, healthcare professionals use multimodal models to diagnose diseases using medical images and patient history reports. How Multimodal Models Work? Although multimodal models have varied architectures, most frameworks have a few standard components. A typical architecture includes an encoder, a fusion mechanism, and a decoder. Architecture Encoders Encoders transform raw multimodal data into machine-readable feature vectors or embeddings that models use as input to understand the data’s content. Embeddings Multimodal models often have three types of encoders for each data type - image, text, and audio. Image Encoders: Convolutional neural networks (CNNs) are a popular choice for an image encoder. CNNs can convert image pixels into feature vectors to help the model understand critical image properties. Text Encoders: Text encoders transform text descriptions into embeddings that models can use for further processing. They often use transformer models like those in Generative Pre-Trained Transformer (GPT) frameworks. Audio Encoders: Audio encoders convert raw audio files into usable feature vectors that capture critical audio patterns, including rhythm, tone, and context. Wav2Vec2 is a popular choice for learning audio representations. Fusion Mechanism Strategies Once the encoders transform multiple modalities into embeddings, the next step is to combine them so the model can understand the broader context reflected in all data types. Developers can use various fusion strategies according to the use case. The list below mentions key fusion strategies. Early Fusion: Combines all modalities before passing them to the model for processing. Intermediate Fusion: Projects each modality onto a latent space and fuses the latent representations for further processing. Late Fusion: Processes all modalities in their raw form and fuses the output for each. Hybrid Fusion: Combines early, intermediate, and late fusion strategies at different model processing phases. Fusion Mechanism Methods While the list above mentions the high-level fusion strategies, developers can use multiple methods within each strategy to fuse the relevant modalities. Attention-based Methods Attention-based methods use the transformer architecture to convert embeddings from multiple modalities into a query-key-value structure. The technique emerged from a seminal paper - Attention is All You Need - published in 2017. Researchers initially employed the method for improving language models, as attention networks allowed these models to have longer context windows. However, developers now use attention-based methods in other domains, including computer vision (CV) and generative AI. Attention networks allow models to understand relationships between embeddings for context-aware processing. Cross-modal attention frameworks fuse different modalities in a multimodal context according to the inter-relationships between each data type. For instance, an attention filter will allow the model to understand which parts of a text prompt relate to an image’s visual embeddings, leading to a more efficient fusion output. Concatenation Concatenation is a straightforward fusion technique that merges multiple embeddings into a single feature representation. For instance, the method will concatenate a textual embedding with a visual feature vector to generate a consolidated multimodal feature. The method helps in intermediate fusion strategies by combining the latent representations for each modality. Dot-Product The dot-product method involves element-wise multiplication of feature vectors from different modalities. It helps capture the interactions and correlations between modalities, assisting models to understand the commonalities among different data types. However, it only helps in cases where the feature vectors do not suffer from high dimensionality. Taking dot-products of high-dimensional vectors may require extensive computational power and result in features that only capture common patterns between modalities, disregarding critical nuances. Decoders The last component is a decoder network that processes the feature vectors from different modalities to produce the required output. Decoders can contain cross-modal attention networks to focus on different parts of input data and produce relevant outputs. For instance, translation models often use cross-attention techniques to understand the meanings of sentences in different languages simultaneously. Recurrent neural network (RNN), Convolutional Neural Networks (CNN), and Generative Adversarial Network (GAN) frameworks are popular choices for constructing decoders to perform tasks involving sequential, visual, or generative processes. Learn how multimodal models work in our detailed guide on multimodal learning Multimodal Models - Use Cases With recent advancements in multimodal models, AI systems can perform complex tasks involving the simultaneous integration and interpretation of multiple modalities. The capabilities allow users to implement AI in large-scale environments with extensive and diverse data sources requiring robust processing pipelines. The list below mentions a few of these tasks that multimodal models perform efficiently. Visual Question-Answering (VQA): VQA involves a model answering user queries regarding visual content. For instance, a healthcare professional may ask a multimodal model regarding the content of an X-ray scan. By combining visual and textual prompts, multimodal models provide relevant and accurate responses to help users perform VQA. Image-to-Text and Text-to-Image Search: Multimodal models help users build powerful search engines that can type natural language queries to search for particular images. They can also build systems that retrieve relevant documents in response to image-based queries. For instance, a user may give an image as input to prompt the system to search for relevant blogs and articles containing the image. Generative AI: Generative AI models help users with text and image generation tasks that require multimodal capabilities. For instance, multimodal models can help users with image captioning, where they ask the model to generate relevant labels for a particular image. They can also use these models for natural language processing (NLP) use cases that involve generating textual descriptions based on video, image, or audio data. Image Segmentation: Image segmentation involves dividing an image into regions to distinguish between different elements within an image. Segmentation Multimodal models can help users perform segmentation more quickly by segmenting areas automatically based on textual prompts. For instance, users can ask the model to segment and label items in the image’s background. Top Multimodal Models Multimodal models are an active research area where experts build state-of-the-art frameworks to address complex issues using AI. The following sections will briefly discuss the latest models to help you understand how multimodal AI is evolving to solve real-world problems in multiple domains. CLIP Contrastive Language-Image Pre-training (CLIP) is a multimodal vision-language model by OpenAI that performs image classification tasks. It pairs descriptions from textual datasets with corresponding images to generate relevant image labels. CLIP Key Features Contrastive Framework: CLIP uses the contrastive loss function to optimize its learning objective. The approach minimizes a distance function by associating relevant text descriptions with related images to help the model understand which text best describes an image’s content. Text and Image Encoders: The architecture uses a transformer-based text encoder and a Vision Transformer (ViT) as an image encoder. Zero-shot Capability: Once CLIP learns to associate text with images, it can quickly generalize to new data and generate relevant captions for new unseen images without task-specific fine-tuning. Use Case Due to CLIP’s versatility, CLIP can help users perform multiple tasks, such as image annotation for creating training data, image retrieval for AI-based search systems, and generation of textual descriptions based on image prompts. Want to learn how to evaluate the CLIP model? Read our blog on evaluating CLIP with Encord Active DALL-E DALL-E is a generative model by Open AI that creates images based on text prompts using a framework similar to GPT-3. It can combine unrelated concepts to produce unique images involving objects, animals, and text. DALL-E Key Features CLIP-based architecture: DALL-E uses the CLIP model as a prior for associated textual descriptions to visual semantics. The method helps DALL-E encode the text prompt into a relevant visual representation in the latent space. A Diffusion Decoder: The decoder module in DALL-E uses the diffusion mechanism to generate images conditioned on textual descriptions. Larger Context Window: DALL-E is a 12-billion parameter model that can process text and image data streams containing up to 1280 tokens. The capability allows the model to generate images from scratch and manipulate existing images. Use Case DALL-E can help generate abstract images and transform existing images. The functionality can allow businesses to visualize new product ideas and help students understand complex visual concepts. LLaVA Large Language and Vision Assistant (LLaVA) is an open-source large multimodal model that combines Vicuna and CLIP to answer queries containing images and text. The model achieves SOTA performance in chat-related tasks with a 92.53% accuracy on the Science QA dataset. LLaVA Key Features Multimodal Instruction-following Data: The model uses instruction-following textual data generated from ChatGPT/GPT-4 to train LLaVA. The data contains questions regarding visual content and responses in the form of conversations, descriptions, and complex reasoning. Language Decoder: LLaVA connects Vicuna as the language decoder with CLIP for model fine-tuning on the instruction-following dataset. Trainable Project Matrix: The model implements a trainable projection matrix to map the visual representations onto the language embedding space. Use Case LLaVA is a robust visual assistant that can help users create advanced chatbots for multiple domains. For instance, LLaVA can help create a chatbot for an e-commerce site where users can provide an item’s image and ask the bot to search for similar items across the website. CogVLM Cognitive Visual Language Model (CogVLM) is an open-source visual language foundation model that uses deep fusion techniques to achieve superior vision and language understanding. The model achieves SOTA performance on seventeen cross-modal benchmarks, including image captioning and VQA datasets. CogVLM Key Features Attention-based Fusion: The model uses a visual expert module that includes attention layers to fuse text and image embeddings. The technique helps retain the performance of the LLM by keeping its layers frozen. ViT Encoder: It uses EVA2-CLIP-E as the visual encoder and a multi-layer perceptron (MLP) adapter to map visual features onto the same space as text features. Pre-trained Large Language Model (LLM): CogVLM 17B uses Vicuna 1.5-7B as the LLM for transforming textual features into word embeddings. Use Case Like LLaVA, CogVLM can help users perform VQA tasks and generate detailed textual descriptions based on visual cues. It can also supplement visual grounding tasks that involve identifying the most relevant objects within an image based on a natural language query. Gen2 Gen2 is a powerful text-to-video and image-to-video model that can generate realistic videos based on textual and visual prompts. It uses diffusion-based models to create context-aware videos using image and text samples as guides. Gen2 Key Features Encoder: Gen2 uses an autoencoder to map input video frames onto a latent space and diffuse them into low-dimensional vectors. Structure and Content: It uses MiDaS, an ML model that estimates the depth of input video frames. It also uses CLIP for image representations by encoding video frames to understand content. Cross-Attention: The model uses a cross-modal attention mechanism to merge the diffused vector with the content and structure representations derived from MiDaS and CLIP. It then performs the reverse diffusion process conditioned on content and structure to generate videos. Use Case Gen2 can help content creators generate video clips using text and image prompts. They can generate stylized videos that map a particular image’s style on an existing video. ImageBind ImageBind is a multimodal model by Meta AI that can combine data from six modalities, including text, video, audio, depth, thermal, and inertial measurement unit (IMU), into a single embedding space. It can then use any modality as input to generate output in any of the mentioned modalities. ImageBind Key Features Output: ImageBind supports audio-to-image, image-to-audio, text-to-image and audio, audio and image-to-image, and audio to generate corresponding images. Image Binding: The model pairs image data with other modalities to train the network. For instance, it finds relevant textual descriptions related to specific images and pairs videos from the web with similar images. Optimization Loss: It uses the InfoNCE loss, where NCE stands for noise-contrastive estimation. The loss function uses contrastive approaches to align non-image modalities with specific images. Use Cases ImageBind’s extensive multimodal capabilities make the model applicable in multiple domains. For instance, users can generate relevant promotional videos with the desired audio by providing a straightforward textual prompt. Read more about it in the blog ImageBind MultiJoint Embedding Model from Meta Explained. Flamingo Flamingo is a vision-language model by DeepMind that can take videos, images, and text as input and generate textual responses regarding the image or video. The model allows for few-shot learning, where users provide a few samples to prompt the model to create relevant responses. Flamingo Key Features Encoders: The model consists of a frozen pre-trained Normalizer-Free ResNet as the vision encoder trained on the contrastive objective. The encoder transforms image and video pixels into 1-dimensional feature vectors. Perceiver Resampler: The perceiver resampler generates a small number of visual tokens for every image and video. This method helps reduce computational complexity in cases of images and videos with an extensive feature set. Cross-Attention Layers: Flamingo incorporates cross-attention layers between the layers of the frozen LLM to fuse visual and textual features. Use Case Flamingo can help in image captioning, classification, and VQA. The user must frame these tasks as task prediction problems conditioned on visual cues. GPT-4o GPT-4 Omni (GPT4o) is a large multimodal model that can take audio, video, text, and image as input and generate any of these modalities as output in real time. The model offers a more interactive user experience as it can respond to prompts with human-level efficiency. GPT-4o Key Features Response Time: The model can respond within 320 milliseconds on average, achieving human-level response time. Multilingual: GPT-4o can understand over fifty languages, including Hindi, Arabic, Urdu, French, and Chinese. Performance: The model achieves GPT-turbo-level performance on multiple benchmarks, including text, reasoning, and coding expertise. Use Case GPT-4o can generate text, video, audio, and image with nuances such as tone, rhythm, and emotion provided in the user prompt. The capability can help users create more engaging and relevant content for marketing purposes. Gemini Google Gemini is a set of multimodal models that can process audio, video, text, and image data. It offers Gemini in three variants: Ultra for complex tasks, Pro for large-scale deployment, and Nano for on-device implementation. Gemini Key Features Larger Context Window: The latest Gemini versions, 1.5 Pro and 1.5 Flash, have long context windows, making it capable of processing long-form videos, text, code, and words. For instance, Gemini 1.5 Pro supports up to two million tokens, and 1.5 Flash supports up to one million tokens, Transformer-based Architecture: Google trained the model on interleaved text, image, video, and audio sequences using a transformer. Using the multimodal input, the model generates images and text as output. Post-training: The model uses supervised fine-tuning and reinforcement learning with human feedback (RLHF) to improve response quality and safety. Use Case The three Gemini model versions allow users to implement Gemini in multiple domains. For instance, Gemini Ultra can help developers generate complex code, Pro can help teachers check students’ hand-written answers, and Nano can help businesses build on-device virtual assistants. Claude 3 Claude 3 is a vision-language model by Anthropic that includes three variants in increasing order of performance: Haiku, Sonnet, and Opus. Opus exhibits SOTA performance across multiple benchmarks, including undergraduate and graduate-level reasoning. Claude Intelligence vs. Cost by Variant Key Features Long Recall: Claude 3 can process input sequences of more than 1 million tokens with powerful recall. Visual Capabilities: The model can understand photos, charts, graphs, and diagrams while processing research papers in less than three seconds. Better Safety: Claude 3 recognizes and responds to harmful prompts with more subtlety, respecting safety protocols while maintaining higher accuracy. Use Case Claude 3 can be a significant educational tool as it comprehends dense data and technical language, including complex diagrams and figures. Challenges and Future Trends While multimodal models offer significant benefits through superior AI capabilities, building and deploying these models is challenging. The list below mentions a few of these challenges to help developers understand possible solutions to overcome these problems. Challenges Data Availability: Although data for each modality exists, aligning these datasets is complex and results in noise during multimodal learning. Helpful mitigation strategies include using pre-trained foundation models, data augmentation techniques, and few-shot learning techniques to train multimodal models. Data Annotation: Annotating multimodal data requires extensive expertise and resources to ensure consistent and accurate labeling across different data types. Developers can address this issue using third-party annotation tools to streamline the annotation process. Mode Complexity: The complex architectural design makes training a multimodal model computationally expensive and prone to overfitting. Strategies such as knowledge distillation, quantization, and regularization can help mitigate these problems and boost generalization performance. Future Trends Despite the challenges, research in multimodal systems is ongoing, leading to productive developments concerning data collection and annotation tools, training methods, and explainable AI. Data Collection and Annotation Tools: Users can invest in end-to-end AI platforms that offer multiple tools to collect, curate, and annotate complex datasets. For instance, Encord is an end-to-end AI solution that offers Encord Index to collect, curate, and organize image and video datasets, and Encord Annotate to label data items using micro-models and automated labeling algorithms. Training Methods: Advancements in training strategies allow users to develop complex models using small data samples. For instance, few-shot, one-shot, and zero-shot learning techniques can help developers train models on small datasets while ensuring high generalization ability to unseen data. Explainable AI (XAI): XAI helps developers understand a model’s decision-making process in more detail. For instance, attention-based networks allow users to visualize which parts of data the model focuses on during inference. Development in XAI methods will enable experts to delve deeper into the causes of potential biases and inconsistencies in model outputs. Multimodal Models: Key Takeaways Multimodal models are revolutionizing human-AI interaction by allowing users and businesses to implement AI in complex environments requiring an advanced understanding of real-world data. Below are a few critical points regarding multimodal models: Multimodal Model Architecture: Multimodal models include an encoder to map raw data from different modalities into feature vectors, a fusion strategy to consolidate data modalities, and a decoder to process the merged embeddings to generate relevant output. Fusion Mechanism: Attention-based methods, concatenation, and dot-product techniques are popular choices for fusing multimodal data. Multimodal Use Cases: Multimodal models help in visual question-answering (VQA), image-to-text and text-to-image search, generative AI, and image segmentation tasks. Top Multimodal Models: CLIP, Dall-E, and LLaVA are popular multimodal models that can process video, image, and textual data. Multimodal Challenges: Building multimodal models involves challenges such as data availability, annotation, and model complexity. However, experts can overcome these problems through modern learning techniques, automated labeling tools, and regularization methods.
Jul 16 2024
5 M
Meet Shivant - Technical CSM at Encord
For today’s version of “Behind the Enc-urtain”, we sat down with Shivant, Technical CSM at Encord, to learn more about his journey and day-to-day role. Shivant joined the GTM team when it was a little more than a 10 person task force, and has played a pivotal role in our hypergrowth over the last year. In this post, we’ll learn more about the camaraderie he shares with the team, what culture at Encord is like, and the thrill of working on some pretty fascinating projects with some of today’s AI leaders. To start us off - could you introduce yourself to the readers, & share more about your journey to Encord? Of course! I’m originally from South Africa – I studied Business Science and Information Systems, and started my career at one of the leading advisory firms in Cape Town. As a Data Scientist, I worked on everything from technology risk assessments to developing models for lenders around the world. I had a great time - and learned a ton! In 2022 I was presented the opportunity to join a newly-launched program in Analytics at London Business School, one of the best Graduate schools in the world. I decided to pack up my life (quite literally!) and move to London. That year was an insane adventure – and I didn’t know at the time but it prepared me extremely well for what my role post-LBS would be like. It was an extremely diverse and international environment, courses were ever-changing and a good level of challenging, and, as the cliche goes, I met some of my now-best friends! I went to a networking event in the spring, where I met probably two dozen startups that were hiring – I think I walked around basically every booth, and actually missed the Encord one. [NB: it was in a remote corner!] As I was leaving I saw Lavanya [People Associate at Encord] and Nikolaj [Product Manager at Encord] packing up the booth. We started chatting and fast forward to today… here we are! What was something you found surprising about Encord when you joined? How closely everyone works together. I still remember my first day – my desk-neighbors were Justin [Head of Product Engineering], Eric [Co-founder & CEO] and Rad [Head of Engineering]. Coming from a 5,000 employee organization, I already found that insane! Then throughout the day, AEs or BDRs would pass by and chat about a conversation they had just had with a prospect – and engineers sitting nearby would chip in with relevant features they were working on, or ask questions about how prospects were using our product. It all felt quite surreal. I now realize we operate with extremely fast and tight feedback loops and everyone generally gets exposure to every other area of the company – it’s one of the reasons we’ve been able to grow and move as fast as we have. What’s your favorite part of being a Technical CSM at Encord? The incredibly inspiring projects I get to help our customers work on. When most people think about AI today they mostly think about ChatGPT but, beyond LLMs, companies are working on truly incredible products that are improving so many areas of society. To give an example – on any given day, my morning might start with helping the CTO of a generative AI scale-up improve their text-to-video model, be followed by a call with an AI team at a drone startup who is trying to more accurately detect carbon emissions in a forest, and end with meeting a data engineering team at a large healthcare org who’s working on deploying a more automated abnormality-detector for MRI scans. I can’t really think of any other role where I’d be exposed to so much of “the future”. It’s extremely fun. What words would you use to describe the Encord culture? Open and collaborative. We’re one team, and the default for everyone is always to focus on getting to the best outcome for Encord and our customers. Also, agile: the AI space we’re in is moving fast, and we’re able to stay ahead of it all and incorporate cutting-edge technologies into our platform to help our customers – sometimes a few days from it being released by Meta or OpenAI. And then definitely diverse: we’re 60 employees, from 34 different nationalities, which is incredibly cool. I appreciate being surrounded by people from different backgrounds, it helps me see things in ways I wouldn’t otherwise, and has definitely challenged a lot of what I thought was the norm. What are you most excited re. Encord or the CS team this year? There’s a lot to be excited about – this will be a huge year for us. We recently opened our San Francisco office to be closer to many of our customers, so I’m extra excited about having a true Encord base in the Bay area and getting to see everyone more regularly in person. We’re also going to grow the CS team past Fred & I for the first time! We’re looking for both Technical CSMs and Senior CSMs to join the team, both in London and in SF, as well as Customer Support Engineers and ML Solutions Engineers. On the topic of hiring… who do you think Encord would be the right fit for? Who would enjoy Encord the most? In my experience, people who enjoy Encord the most have a strong sense of self-initiative and ambition – they want to achieve big, important outcomes but also realize most of the work to get there is extremely unglamorous and requires no task being “beneath” them. They tend to always approach a problem with the intent of finding a way to get to the solution, and generally get energy from learning and being surrounded by other talented, extremely smart people. Relentlessness is definitely a trait that we all share at Encord. A lot of our team is made up of previous founders, I think that says a lot about our culture. See you at the next episode of “Behind the Enc-urtain”! And as always, you can find our careers page here😉
Jul 10 2024
5 M
How Poor Data is Killing Your Models and How to Fix It
The accuracy and reliability of your AI models hinge on the quality of the data they are trained on. The concept of "Garbage In, Garbage Out" (GIGO) is crucial here—if your data is flawed, your models will be too. This blog will explore how poorly curated data can undermine AI models, examining the cost of poor data quality, and highlighting common pitfalls in data curation. By the end, you'll have a clear understanding of the importance of data quality and actionable steps to enhance it, ensuring your AI projects succeed. Understanding GIGO Principal "Garbage In, Garbage Out" (GIGO) is a foundational concept in computing and data science, emphasizing that the quality of output is determined by the quality of input. Originating from the early days of computing, GIGO underscores that computers, and by extension AI models, will process whatever data they are given. If the input data is flawed—whether due to inaccuracies, incompleteness, or biases—the resulting output will be equally flawed. AI models rely on large datasets for training; these datasets must be accurate, comprehensive, and free of bias to ensure reliable and fair predictions. For example, a study conducted by MIT Media Lab highlighted the consequences of poor data quality in facial recognition systems. The study found that facial recognition software from major tech companies had significantly higher error rates in identifying darker-skinned and female faces compared to lighter-skinned and male faces. This disparity was primarily due to the training datasets lacking diversity, leading to biased and unreliable outcomes. The Cost of Poor Data Quality Impact on Model Accuracy Poor data quality can drastically reduce model accuracy. Inaccurate, incomplete, or inconsistent data can lead to unreliable predictions, rendering the model ineffective. For example, a healthcare AI system trained on erroneous patient records might misdiagnose conditions, leading to harmful treatment recommendations. Business Consequences The financial implications of poor data quality are significant. Companies have lost millions due to flawed AI models making incorrect decisions. For instance, an e-commerce company might lose customers if its recommendation system, based on poor data, suggests irrelevant products. Read the case study on How Automotus increased mAP by 20% by improving their dataset with visual data curation to understand the importance of data quality. Common Pitfalls in Data Curation Incomplete Data Incomplete data is a major issue in CV datasets. Missing or insufficient image data can lead to models that fail to generalize well. For instance, if a dataset meant to train a self-driving car's vision system lacks images of certain weather conditions or types of road signs, the system might perform poorly in real-world scenarios where these missing elements are present. Data Bias Bias in data is another critical issue. If training data reflects existing societal biases, the AI model will perpetuate these biases. For instance, an AI system trained on biased criminal justice data might disproportionately target certain demographics. Outdated Data CV models trained on images that no longer represent the current environment can become obsolete. For example, a model trained on images of cars from the 1990s might struggle to recognize modern vehicles. Regular updates to datasets are necessary to keep the model relevant and accurate. This is particularly important in rapidly evolving fields such as autonomous driving and retail, where the visual environment changes frequently. Inconsistent Data Inconsistent data can arise when images are collected from multiple sources with varying formats, resolutions, and labeling conventions. This inconsistency can confuse the model and lead to poor performance. For example, images labeled with different naming conventions or annotation styles can result in a model that misunderstands or misclassifies objects. Standardizing data collection and annotation processes is key to maintaining consistency across the dataset. Annotation Errors Errors in image annotation, such as incorrect labels or poorly defined bounding boxes, can severely impact model training. Annotations serve as the ground truth for supervised learning, and inaccuracies here can lead to models learning incorrect associations. Rigorous quality control and verification processes are essential to minimize annotation errors. Imbalanced Classes Class imbalance, where some categories are underrepresented in the dataset, is a frequent issue in datasets. For instance, in an object detection dataset, if there are significantly more images of cars than bicycles, the model may become biased towards detecting cars while neglecting bicycles. This imbalance can lead to poor performance on underrepresented classes. Techniques such as data augmentation, oversampling of minority classes, or using class weights during training can help address this issue. Using Encord for Data Curation Encord is a data development platform for computer vision and multimodal AI teams, built to help you manage, clean, and curate your data. With Encord, you can streamline your labeling and workflow management processes, ensuring consistent and high-quality annotations. It also provides robust tools to evaluate model performance, helping you identify and rectify issues early on. With Encord's comprehensive suite of features, you can overcome common pitfalls in data curation and enhance the accuracy and reliability of your AI models. Curious to learn more about how poor data quality can impact your AI models and how Encord can help? Watch the webinar Garbage In Garbage Out: Poorly Curated Data is Killing Your Models for a comprehensive understanding of effective data curation strategies, real-world examples, and practical tips to enhance your model performance
Jul 02 2024
5 M
How to Leverage Computer Vision in Warehouse Automation
In today's fast-paced supply chain environment, warehouses must operate efficiently and accurately to keep up. Manual processes often cause errors, inefficiencies, and safety risks, making it hard for warehouses to meet modern logistics demands. A key challenge is keeping accurate inventory levels and ensuring the proper handling and shipment of goods. Warehouse automation addresses these challenges by using technology to perform tasks and processes with minimal human intervention. This includes deploying robotics, automated storage and retrieval systems (AS/RS), automated guided vehicles (AGVs), conveyor systems, and software solutions like warehouse management systems (WMS). The goal is to streamline operations, enhance efficiency, reduce errors, and improve overall productivity. Computer vision (CV) plays a pivotal role in revolutionizing warehouse automation. It enables machines to interpret and understand visual data using cameras, automating tasks traditionally performed by human workers. In this article, you will learn about the impact of CV on warehouse automation. You will explore key applications using CV in warehouse automation. After reading this article, you will understand how CV improves warehouse efficiency, accuracy, and safety and how to use it to optimize logistics processes. Role of Computer Vision in Warehouse Automation Computer vision (CV) is essential to improving warehouse automation by enabling systems to interpret and act on visual data. It transforms warehouse operations, from inventory management to quality control, significantly improving efficiency, accuracy, and safety. Computer Vision powered Robotic Arm (Source) Here’s a detailed look at the role of computer vision in warehouse automation: Automated Data Capture CV systems continuously capture visual data from the warehouse environment, converting it into actionable insights. This automated data capture reduces the need for manual data entry and ensures real-time updates. Object Detection and Tracking CV algorithms detect and track objects, such as products, pallets, and equipment, within the warehouse. This capability is essential for maintaining accurate inventory records, monitoring the movement of goods, and optimizing storage layouts. See Also: Object Detection: Models, Use Cases, Examples. Quality Assurance By analyzing visual data, CV systems can identify defects, damages, or non-compliance with quality standards. This role is crucial for maintaining high product quality and minimizing errors. Spatial Awareness and Navigation CV provides spatial awareness to robotic systems, enabling them to navigate the warehouse environment safely and efficiently. This includes avoiding obstacles, finding optimal paths, and coordinating movements with other robots or humans. Environmental Monitoring Vision systems monitor environmental conditions such as lighting, temperature, and humidity. This role ensures that products are stored under optimal conditions and helps in maintaining the integrity of sensitive goods. Security and Safety CV enhances warehouse security by monitoring for unauthorized access, theft, or other security breaches. Additionally, it plays a vital role in ensuring workplace safety by detecting hazardous conditions and ensuring compliance with safety protocols. Key Applications of Computer Vision in Warehouse Automation In this section, you will learn key examples of CV applications in warehouse automation. These real-world examples illustrate how computer vision revolutionizes warehouse operations, enhancing efficiency, accuracy, and safety in warehouse processes. Automated Inventory Management Automated inventory management using computer vision involves cameras, sensors, and CV algorithms and models (such as YOLO, SSD, R-CNN, etc.) to monitor and manage inventory levels in real-time. This enables the automated identification, tracking, and counting of items, automatically updating inventory records, and triggering reordering processes when stock levels are low within a warehouse. High-resolution cameras and sensors are strategically placed throughout the warehouse. These devices continuously capture images and video of the inventory, shelves, and storage areas. Automated inventory system KoiVision (Source: NVIDIA Blogs) The captured visual data is processed using CV algorithms. These algorithms can detect and recognize items, read barcodes or QR codes, and assess the quantity and condition of the inventory. The systems provide real-time updates on inventory levels. The system automatically adjusts the inventory records to reflect these changes as items are added or removed. The CV system is integrated with the warehouse management system, enabling real-time synchronization of inventory data. For example, PepsiCo uses computer vision technologies from KoiReader to inspect labels for efficient inventory management and distribution operations. Order Picking and Packing Order picking and packing using CV uses object detection and recognition to automate and optimize the selection and packaging of items for shipment in a warehouse. Robotic systems equipped with CV identify, pick, and pack items for shipment. Vision systems ensure the correct items are picked and packed, minimizing errors. Cameras and sensors are installed throughout the picking and packing areas or on robotic arms. These devices continuously capture images and video of items, shelves, and packaging stations. Computer vision algorithms analyze the visual data to identify items by recognizing shapes, sizes, barcodes, QR codes, and other visual markers. The system determines the exact location of each item. In the packing area, computer vision systems assist in selecting the appropriate packaging materials and methods for each item. For example, Ocado, the UK-based online supermarket, uses robotic arms guided by computer vision to pick up groceries. The vision systems help the robots identify products, pick them up without damaging them, and efficiently place them into customer orders. Ocado robotic arms guided by Computer Vision (Source: BBC) Autonomous Mobile Robots (AMRs) AMRs are robots capable of navigating complex and dynamic environments without physical guidance. They use sophisticated sensors and algorithms to understand and interpret their surroundings. AMRs use computer vision, LiDAR, and other sensors to create and continuously update maps of their environment to navigate the warehouse environment, transport goods, and avoid obstacles. Vision systems provide real-time feedback for route optimization and collision avoidance, enabling AMRs to dynamically plan and adjust their paths based on real-time environmental data. Computer vision allows AMRs to recognize objects, thus helping them interact with them, such as picking items from shelves or placing them in designated areas. Fetch Robotics deploys AMRs equipped with computer vision for warehouse material handling tasks. These robots can navigate complex environments, transport goods between locations, and work alongside human workers. Fetch Robotics’ Freight1500 has zero blind spots and 360° robot vision. (Source: Fetch Robotics.) Sorting and Categorizing Sorting robots are stationary (e.g., XYZ Gantry Robots, Robotic Arms) and mobile variants designed to swiftly and effectively organize goods and parcels based on their destination and categorization criteria. These robots use sensors, cameras, actuators, and mechanical components to detect, identify, and classify objects accurately before sorting them into designated locations. This automated process enhances logistics and distribution operations efficiency, ensuring precise handling and timely delivery of items to their intended destinations. For example, Unbox Robotics’ Elevated Mobile Robots (EMRs) are specifically engineered for automated material handling and sorting tasks within warehouses and distribution centers. See this article to learn more. These robots often have elevated platforms or track systems, enabling them to navigate above and around warehouse machinery and obstacles. Using different sensors and CVs, EMRs can accurately identify and categorize items based on various attributes, such as size, shape, weight, and other distinguishing features. This capability allows them to efficiently manage sorting tasks, contributing to streamlined warehouse operations and enhanced logistical efficiency. Unbox Robotics’ AMRs for sorting (Source: Unbox Robotics) Safety and Security Monitoring Computer vision is critical to enhancing safety and security by continuously monitoring the environment, detecting potential hazards, and preventing unauthorized access. These systems analyze the visual data to detect potential safety hazards. This includes identifying spills, obstacles, unsafe stacking of goods, and malfunctioning equipment. When a hazard is detected, the system can immediately alert warehouse personnel to address the issue. Using computer vision, worker behavior can be monitored to ensure compliance with safety protocols. For example, it can detect whether employees wear required personal protective equipment (PPE), such as helmets, gloves, and safety vests. It can also monitor for unsafe behaviors, such as workers entering restricted areas or operating machinery improperly. CV can be integrated with access control systems to prevent unauthorized entry. By using facial recognition and other biometric data, the system ensures that only authorized personnel can access sensitive areas. For example, Protex AI, a CV startup, collaborated with DHL on a proof-of-concept project utilizing their AI-based unsafe event capture solution. Workplace safety monitoring at DHL warehouse (Source: DHL) Palletizing and Depalletizing Palletizing and depalletizing are critical processes in warehouse operations. Palletizing involves arranging products onto a pallet for storage or transportation, while depalletizing involves removing products. Using CV in these processes enhances efficiency, accuracy, and safety. In this process, cameras and sensors capture images of products, determining their size, shape, orientation, and position. This data is crucial for creating an optimal arrangement on the pallet. The visual data is then analyzed to identify and classify the items. This step ensures the system understands what items must be palletized and how they should be arranged. The system calculates how to arrange items on a pallet to maximize space and stability. This involves determining the most efficient stacking pattern and orientation for each item. For example, Mech-Mind’s AI+3D industrial robot solution uses computer vision in warehouses to palletize and depalletize cartons. The 3D vision system, which uses Mech-Eye DEEP 3D vision camera, generates precise point cloud data while AI algorithms position suction cups correctly for accurate grabbing. Quickly recognizing new cartons and handling various random pallet patterns optimize consistency and efficiency. Palletizing and depalletizing cartons in Warehouse (Source: Mech-Mind) Label Verification Label verification is critical in warehouse operations to ensure that products are correctly identified, tracked, and processed. CV enhances the accuracy and efficiency of label verification by automating the reading and verification of product labels. CV algorithms analyze the images on products to read text, barcodes, QR codes, and other label information. This enables the system to extract crucial details such as product codes, descriptions, batch numbers, and expiration dates. The extracted label information is compared against data in the warehouse management system (WMS). The system verifies that the labels match the expected data for each product, ensuring accuracy in identification and inventory management. Label verification for quality control (Source: OpenCV AI) Recommended: Vision-based Localization: A Guide to VBL Techniques for GPS-Denied Environments. Computer Vision Implementation Strategies in Warehouse Automation Implementing computer vision in warehouse automation involves careful planning and execution to ensure successful integration and maximized benefits. Image Source: baslerweb.com Here are key implementation strategies: Needs Assessment and Goal Setting Conduct a thorough assessment of the warehouse operations to identify specific areas where computer vision can add value. Define clear objectives such as improving accuracy, reducing labor costs, enhancing safety, or increasing operational efficiency. Following are the action steps that must be taken: Engage stakeholders to understand pain points. Map out current processes and identify bottlenecks. Set measurable goals for the computer vision implementation. Choosing the Right Technology and Vendors Select appropriate computer vision technologies and tools that align with your objectives. Consider factors such as accuracy, speed, scalability, and ease of integration. Following are the action steps that must be taken: Evaluate different computer vision algorithms (e.g., CNNs, YOLO, SSD). Consider hardware requirements, including cameras, sensors, and computational resources. Choose software platforms and libraries (e.g., OpenCV, TensorFlow, and PyTorch) that support your needs. Develop and Train Models Create and train models customized to your warehouse's specific tasks, such as object detection, classification, or segmentation. Following are the action steps that must be taken: Collect and annotate a diverse set of training data representative of the warehouse environment. Train models using supervised learning techniques and validate their performance on test datasets. Optimize models for accuracy and efficiency, using transfer learning to leverage pre-trained models. Integrate with Existing Systems Ensure seamless integration of computer vision systems with existing warehouse management systems (WMS), enterprise resource planning (ERP) systems, and other relevant software. The following are the action steps that must be taken: Use APIs and middleware to connect computer vision systems with WMS and ERP systems. Ensure data compatibility and synchronization between systems. Develop custom integration solutions if necessary. Maintenance and Continuous Improvement Implement a maintenance plan to ensure the computer vision systems remain operational and effective. To put in place a robust maintenance plan, you must consider the following action steps: Establish regular maintenance schedules for hardware components such as cameras and sensors. Monitor system performance and conduct periodic reviews to identify areas for improvement. Update software and models to incorporate new features or address emerging challenges. Continuously improve the system based on performance data and feedback. How Encord Helps with the Continuous Improvement of Warehouse Automation Systems You can improve your warehouse automation and maintenance strategies with a platform like Encord, which offers three products: Encord Annotate to help label the visual datasets you collect from the warehouse and sites through vision-based sensors, Encord Index to help curate and manage the scale of the visual dataset, If you train computer vision models at scale, Encord Active can help you test and evaluate the model's performance before you deploy it to the system. Companies like Voxel, a global leader in workplace safety, empower worksites by providing the data they need to protect workers and gain insight into workplace activities. See how Encord helps them solve critical problems with automation systems. Benefits of Computer Vision in Warehouse Automation Implementing computer vision (CV) in warehouse automation brings numerous advantages that significantly enhance operational efficiency, accuracy, and safety. Warehouse automation using robots and AMRs (Source: roboticstomorrow.com) The following are some key benefits: Increased Efficiency CV automates tasks such as inventory management, sorting, and quality control, which speeds up processes that would otherwise be time-consuming if done manually. It results in: Faster order processing and fulfillment. Reduced cycle times for inventory counting and verification. Improved throughput in sorting and packing operations. Enhanced Accuracy CV systems provide precise and consistent analysis of visual data, reducing human error in tasks such as counting, sorting, and inspecting goods, resulting in: More accurate inventory records and reduced stock discrepancies. Fewer picking and packing errors, leading to improved customer satisfaction. Precise defect detection and quality control, ensuring higher product quality. Improved Safety Computer vision systems can continuously monitor the warehouse environment for potential safety hazards, such as obstacles, spills, or unsafe behavior, and alert staff to take preventive measures, resulting in: Reduced risk of accidents and injuries. Enhanced compliance with safety regulations. Real-time alerts and interventions to prevent hazardous situations. Enhanced Quality Control CV systems can inspect products for defects, damages, or non-compliance with quality standards, ensuring that only high-quality products are shipped to customers. It results in: Improved product quality and consistency. Reduction in returns and customer complaints. Increased customer satisfaction and brand reputation. Challenges of Using Computer Vision in Warehouse Automation Image Source: DHL High Initial Investment: Implementing computer vision systems can be costly due to the need for high-quality cameras, computing resources, and networking infrastructure. Integration with Existing Systems: Integrating new computer vision technologies with existing warehouse management systems (WMS) and processes can be complex and time-consuming. Data Privacy and Security Concerns: Capturing and storing visual data can raise privacy issues and expose the system to cybersecurity threats. Accuracy and Reliability of Vision Systems: Computer vision systems can sometimes struggle with accuracy due to varying lighting conditions, occlusions, or environmental changes. Complexity of Implementation: Setting up and configuring computer vision systems requires technical expertise and can be complex. Scalability Issues: As the volume of data increases, the system needs to scale accordingly, which can be challenging. Technical Limitations: Computer vision technology is still evolving, and there may be limitations in the algorithms' ability to recognize and interpret certain objects or scenarios. Conclusion We can reduce manual labor and associated errors by automating various tasks, such as inventory management, sorting, quality control, and safety monitoring, leading to faster and more reliable warehouse operations. Implementing computer vision involves strategic planning, including assessing needs, selecting appropriate technologies, training machine learning models, integrating systems, and conducting pilot tests. These steps ensure that the systems are scalable, flexible, and capable of adapting to dynamic warehouse environments. Key benefits of computer vision in warehouse automation include increased efficiency, improved accuracy, enhanced safety, real-time data and insights, cost savings, scalability, quality control, predictive maintenance, and improved security. These advantages collectively contribute to a more streamlined and effective warehouse operation, supporting businesses in meeting customer demands and maintaining a competitive edge. Planners must carefully plan, implement robust security measures, and continuously maintain the system to address challenges such as high initial setup costs, integration complexities, and data privacy concerns. The future of computer vision in warehouses looks promising, with advancements in AI algorithms, IoT integration, and more efficient hardware solutions expected to further enhance its capabilities.
Jul 01 2024
7 M
Encord Monthly Wrap: June Industry Newsletter
Hi there, Welcome to the Computer Vision Monthly Wrap for June 2024! Here’s what you should expect: 🎁 Automatic Data Curation for Self-Supervised Learning: A Clustering-Based Approach 📽️ Top CVPR 2024 papers, including the poster sessions ⚒️ Developer resources to use for your next vision AI application 🤝 New model releases in the computer vision and multimodal AI world Let’s go! 🚀 Encord released TTI-Eval, an open-source library to evaluate the performance of fine-tuned CLIP, domain-specific ones like BioCLIP models, and other VLMs on your dataset! Check out the getting started blog post. 📐 📜 Top Picks for Computer Vision Papers This Month Automatic Data Curation for Self-Supervised Learning: A Clustering-Based Approach Researchers at Meta AI released a paper introducing an automatic data curation method for self-supervised learning that can create large, diverse, and balanced datasets without manual effort. The approach in the paper uses hierarchical k-means clustering and balanced sampling to curate high-quality datasets from raw data. The method addresses imbalanced data representation in web-based collections, ensuring a more uniform distribution of diverse concepts. What’s impressive? 🤯 The approach enables training self-supervised models on automatically curated datasets, which alleviates the need for costly manual labeling and curation Hierarchical k-means clustering obtains uniform data clusters representing different concepts Balanced sampling from the clusters ensures the curated dataset has an even distribution of concepts Experiments on images, satellite data, and text show features trained on the auto-curated datasets match or exceed the performance of features from manually curated data How can you apply it? ⚒️ Curate your own high-quality datasets from large raw data sources for self-supervised pre-training Scale up model training by avoiding the bottleneck of manual data curation Improve model robustness and generalization by training on diverse and balanced datasets Apply the technique to various domains like computer vision, earth observation (remote-sensing), and natural language processing Frederik Hvilshøj, Lead ML Engineer at Encord, spoke to the paper's first author and distilled (yes, I don’t excuse the pun 😁) insights from the paper and conversations. Watch the video on LinkedIn. 📜 Read the publication. Top Papers and Poster Sessions from CVPR 2024 CVPR 2024 was quite an experience for many researchers, stakeholders, and engineers working on computer vision and multimodal AI problems. At Encord, we even released a fun game to get you battling it out with AI to win amazing prizes! 😎. This article reviews the top papers presented at CVPR 2024, including the research highlights. Frederik also reviewed some of the papers that were presented during the poster session: YOLO-World: Real-Time Open-Vocabulary Object Detection Putting the Object Back into Video Object Segmentation Panda-70M: Captioning 70M Videos with Multiple Cross- modality Teachers InternVL: Scaling up Vision Foundation models and Aligning for Generic Visual-Linguistic Tasks 🧑💻 Developer Resources You’d Find Useful Building Multi-Modal RAG Systems → Frederik Hvilshøj shared insights on a new model that could be just what you need to integrate multimodal RAGs in your apps. [WATCH] Interactive Tutorial On Using Gemini in Data Pipelines → Learn how to use Gemini 1.5 Pro to extract structured data from visual content with hands-on examples in this Colab notebook. Notebook for Fine-tune Florence-2 → The Hugging Face team and community members shared a notebook, HF Space, and a walkthrough blog on fine-tuning Florence-2 on DocVQA dataset. Check them out. New to Florence-2 from Microsoft? See this explainer blog post. How to Pre-Label Your Data with GPT-4o → Multimodal AI models are increasingly useful for bulk classification and pre-labeling datasets. That blog walks you through the principles behind this and shows you how to set up your own AI Agents to automate labeling 📰 Computer Vision In the News DeepMind’s new AI generates soundtracks and dialogue for videos → A new video-to-audio model that DeepMind developed can use a video and a soundtrack description (such as "jellyfish pulsating underwater, marine life, ocean") to produce audio that matches the video's mood, characters, and plot. Sensor Perception with NVIDIA’s Omniverse Cloud Sensor RTX at CVPR 2024 → At CVPR, NVIDIA showcased Omniverse microservices, including techniques and algorithms to simulate perception-based activities in realistic virtual environments before real-world deployment. From TechCrunch → All the rage has been about Runway’s new video-generating AI, Gen-3, which offers improved controls and more high-fidelity video generation results. Till next month, have a super-sparkly time!
Jul 01 2024
7 M
Automate Text Labeling for Your Image Dataset: A Step-by-Step Guide
Building a high-quality image dataset can be a daunting task, especially when it involves extensive manual labeling. Fortunately, with the Encord Agents, you can automate the process of text labeling, making your workflow more efficient and accurate. In this blog, we'll walk you through how to set up and use Encord Agents to perform OCR, streamlining your image annotation tasks. Why Use OCR for Text Labeling? OCR enables the extraction of text from images, transforming it into editable and searchable data. This can be incredibly useful for labeling datasets that contain images with embedded text, such as street signs, documents, product labels, and more. By automating this process with Encord Agents, you can save time and ensure consistency in your annotations. Using Encord to Automate Text Labeling Uploading Data The first step of any data labeling process is data curation. We will upload our data to Encord Index which streamlines this process by enabling data collection, versioning, and quality assurance. Here, you have the option to upload your data directly or seamlessly integrate with leading cloud storage providers such as AWS S3, Azure Blob Storage, Google Cloud Storage, and Open TelekomCloud OSS. Set Up Encord Agent Define Task First, determine the specific task you want your Encord Agent to perform. For this example, we'll focus on using OCR to extract and label text from images. Set Up a Server You'll need a server to run your code. This could be an AWS Lambda function, a Google Cloud function, or any server that supports HTTPS endpoints. Register the Agent in Encord Next, you'll need to register your OCR Agent in Encord. Encord will send a payload that includes necessary details like project hash, data hash, and image URL. In Encord Apollo, navigate to the Agents section and select Register Agents. Here, enter the name, description, and endpoint of the agent to complete the registration process. Test the Agent After registration, test your Agent before using it in Label Editor. Let’s start labeling! Automated Data Labeling Start your Annotation Project. In this example, we are annotating road signs. Trigger the Agent in the Label Editor of Encord Annotate to get the OCR text to add to the label. By automating text extraction from images, it saves time and ensures consistency in labeling. This automation reduces manual effort, allowing annotators to focus more on refining annotations rather than repetitive data entry tasks. Encord Agents are crucial in automating data labeling processes. By integrating technologies like GPT-4o, Gemini, BERT, T5, and other state-of-the-art models, Encord Agents allows users to achieve better accuracy and productivity in data annotation workflows. Whether you're annotating images, documents, or videos, these agents streamline the labeling process, allowing annotators to focus on refining annotations rather than repetitive tasks. This integration not only enhances workflow efficiency but also ensures consistent and high-quality annotations throughout your projects.
Jun 28 2024
5 M
Explore our products