Contents
State of FDA Approval for AI algorithms
FDA Artificial Intelligence in Healthcare: How Many AI Algorithms are FDA Approved?
AI/ML Regulatory Landscape: How do you Know if Your AI Healthcare Model Needs FDA Approval?
How to get Your AI Model Through FDA approval: Step-by-Step Guide
FDA AI Approval: Conclusion & Key Takeaways
AI FDA Regulatory Approval FAQs
Encord Blog
The Step-by-Step Guide to Getting Your AI Models Through FDA Approval
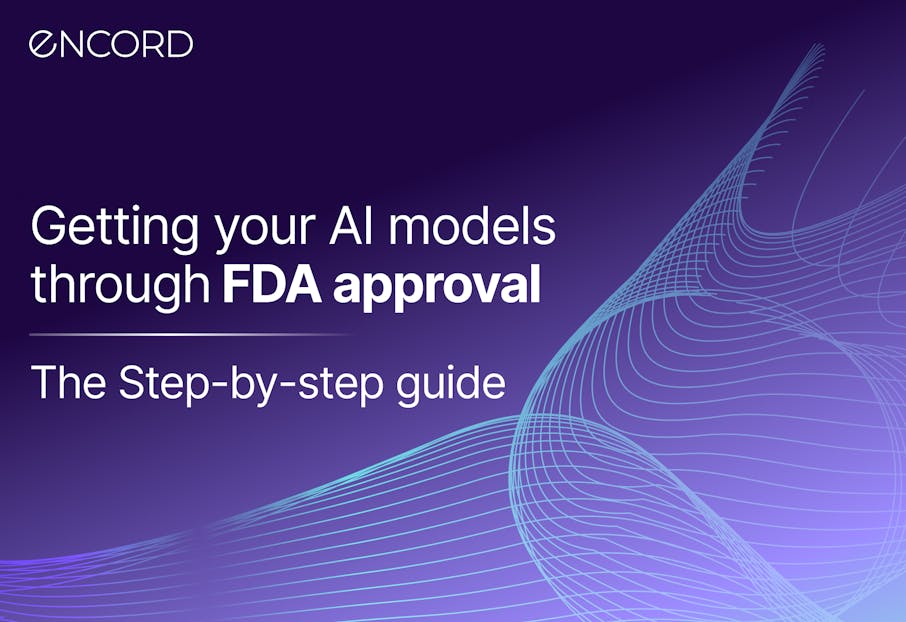
Getting AI models through FDA approval takes time, effort, robust infrastructure, data security, medical expert oversight, and the right AI-based tools to manage data pipelines, quality assurance, and model training.
In this article, we’ve reviewed the US Food & Drug Administration’s (FDA’s) latest thinking and guidelines around AI models (from new software, to devices, to broader healthcare applications). This step-by-step guide is aimed at ensuring you are equipped with the information you need to approach FDA clearance — we will cover the following key steps for getting your AI model through FDA scrutiny:
- Create or source FDA-compliant medical imaging or video-based datasets
- Annotate and label the data (high-quality data and labels are essential)
- Review Medical expert review of labels in medical image/video-based datasets
- A clear and robust FDA-level audit trail
- Quality control and validation studies
- Test your models on the data, figure out what data you need more of/less of to improve your models.
State of FDA Approval for AI algorithms
The number of AI and ML algorithms being approved by the US Food & Drug Administration (FDA) has accelerated dramatically in recent years.
950 AI or machine learning-enabled medical devices have been approved by the FDA as of of August 2024.
By January 2023, the FDA had approved over 520 AI and ML algorithms for medical use. Most of these are related to medical imaging and healthcare image and video analysis, and diagnoses, so in the majority of use cases, these are computer vision (CV) models.
The FDA first approved the use of AI for medical purposes in 1995. Since then, only 50 other algorithms were approved over the next 18 years. And then, between 2019 and 2022, over 300 were approved, with a further 178 granted FDA approval in 2023.
Given the accelerated development of AI, ML, CV, Foundation Models, and Visual Foundation Models (VFMs), the FDA is bracing itself for hundreds of new medical-related models and algorithms seeking approval in the next few years.
See the complete list of FDA-cleared algorithms here.
Algorithms that cleared FDA Approvals
FDA Artificial Intelligence in Healthcare: How Many AI Algorithms are FDA Approved?
Can the FDA handle all of these new approval submissions? Considering the number of AI projects seeking FDA approval, there are naturally concerns about capacity.
Fortunately, just over two years ago, the FDA created its Digital Health Center of Excellence led by Bakul Patel.
Patel’s since left the FDA. However, his processes have modernized the FDA approval processes for AI models, ensuring they’re equipped for hundreds of new applications.
As a University of Michigan law professor specializing in life science innovation, Nicholson Price, said: “There have been questions about capacity constraints on FDA, whether they have the staff and relevant expertise. They had a plan to increase hiring in this space, and they have in fact hired a bunch more people in the digital health space.”
One of the reasons for this is the vast amount of image-based data that data scientists and ML engineers can use when training models, mainly from imaging and electrocardiograms.
Unfortunately, it’s difficult to assess the number of submitted applications and their outcomes. We know how many are approved. What’s unclear is the number that are rejected or need to be re-submitted.
Here’s where FDA approval for AI gets interesting: “FDA-authorized devices likely are just a fraction of the Artificial intelligence and machine learning -enabled tools that exist in healthcare as most applications of automated learning tools don’t require regulatory review.”
For example, predictive tools (such as artificial intelligence, machine learning, and computer vision models) that use medical records and images don’t require FDA approval.
But . . . that might change under new guidance.
Professor Price says, “My strong impression is that somewhere between the majority and vast majority of ML and AI systems being used in healthcare today have not seen FDA review.”
So, for ML engineers, data science teams, and AI businesses working on AI models for the healthcare sector, the question you need to answer first is: Do we need FDA approval?
AI/ML Regulatory Landscape: How do you Know if Your AI Healthcare Model Needs FDA Approval?
Whether you’re AI healthcare model or an AI model that has healthcare or medical imaging applications needs FDA approval is an important question.
Providing approval isn’t needed, then it will save you hours of time and work. So, we’ve spent time investigating this, and here’s what we’ve found:
Under the 21st Century Cures Act, most software and AI tools are exempt from FDA regulatory approval “as long as the healthcare provider can independently review the basis of the recommendations and doesn’t rely on it to make a diagnostic or treatment decision.”
Risk Classification
For regulatory purposes, AI tools and software fall into the FDA category known as Clinical Decision Support Software (CDS).
- Your software function does NOT acquire, process, or analyze medical images, signals, or patterns.
- Your software function displays analyzes, or prints medical information normally communicated between health care professionals (HCPs).
- Your software function provides recommendations (information/options) to a HCP rather than provide a specific output or directive.
- Your software function provides the basis of the recommendations so that the HCP does not rely primarily on any recommendations to make a decision.
If you aren’t clear whether your AI model falls within FDA regulatory requirements, it’s worth checking the Digital Health Policy Navigator.
Checking Whether your AI Model Falls within FDA Regulatory Requirements
In most cases, AI models themselves don’t need FDA approval.
However, if your company is working with a healthcare, medical imaging, medical device, or any other organization that is going through FDA approval, then any algorithmic models, datasets, and labels being used to train a model need to be compliant with FDA guidelines.
How to get Your AI Model Through FDA approval: Step-by-Step Guide
Here are the steps you need to take when working on an AI, ML, or CV model for healthcare organizations, including MedTech companies, that are using a model for devices or new forms of diagnosing patients or treatments that require FDA approval:
- Create or source FDA-compliant medical imaging or video-based datasets
- Annotate and label the data (high-quality data and labels are essential)
- Review Medical expert review of labels in medical image/video-based datasets
- A clear and robust FDA-level audit trail
- Quality control and validation studies
- Test your models on the data, figure out what data you need more of/less of to improve your models
Here’s how to ensure your AI model will meet FDA approval:
1. FDA-compliant Data: Create or Source FDA-compliant Medical Imaging or Video-based Datasets
Every AI model starts with the data. When working with any company or organization that’s going through the FDA approval process, it’s crucial that the image or video datasets are FDA-compliant.
In practice, this means sourcing (whether open-source or proprietary) high-quality datasets that don’t contain identifiable patient tags and metadata. If files contain specific patient identifiers, then it’s vital annotators and providers cleanse it of anything that could impact the project's development and regulatory approval.
Other factors to consider include:
- Do we have enough data to train a model? Quantity is as important as quality for model training, especially if the project is focused on medical edge cases, and outliers, and addressing any ethnic or gender-based bias.
- How are we storing and transferring this data? Security is crucial, especially if you’re outsourcing the annotation process.
- Can we outsource annotation work? For data security purposes, you need to ensure that transfers, annotation, and labeling is FDA-compliant and adheres to other regulations, such as HIPAA and other relevant data protection laws (e.g., European CE regulations for EU-based projects).
- When working with organizations that are obtaining regulatory approval, the company will have to run a clinical study, and this will require using untouched data that has not been seen by the model or anyone working on it. Before annotation work can start, you need to split and partition the dataset, ideally keeping it in a separate physical location to make it easier to demonstrate compliance during the regulatory approval process.
Open-source CT scan image dataset on Kaggle
Once the datasets are ready to use, it’s time to start the annotation and labeling work.
2. Data Annotation and Labeling: High-quality Data and Labels are Essential
Medical image annotation for machine learning models requires accuracy, efficiency, high quality, and security.
As part of this process, it could be worth having medical teams pre-populate labels for greater accuracy before a team of annotators gets started. Highly skilled medical professionals don’t have much time to spare, so getting medical input at the right stages in the project, such as pre-populating labels and during the quality assurance process, is crucial.
Medical imaging annotation projects run smoother when annotators have access to the right tools. For example, you’ll probably need an annotation tool that can support native medical imaging formats, such as DICOM and NIfTI (recent DICOM updates from Encord).
DICOM annotation
Ensure the datasets and labels being used for model development include a wide statistical range quality of images when searching for the ground truth of medical datasets.
Once enough images or videos have been labeled (whether you’re using a self-supervised, semi-supervised, automated, or human-in-the-loop approach), it’s time for a medical expert review. Especially if you’re working with a company that’s going to seek FDA approval for a device or other medical application in which this model will be used.
What is Data Labeling: The Full Guide
5 Strategies To Build Successful Data Labeling Operations
The Full Guide to Automated Data Annotation
7 Ways to Improve Your Medical Imaging Datasets for Your ML Model
3. Medical Expert Review: Medical Expert Review of Labels in Medical Image/Video-based Datasets
Now the first batch of images or videos has been labeled; you need to loop medical experts back into the process. You need to consider that medical professionals and the FDA take different approaches to determining consensus.
Having a variety of approaches built into the platform is especially useful for regulatory approval because different localities will want companies to use different methods to determine consensus.
Make sure this is built into the process, and ensure the medical experts you’re working with have approved the labels annotators have applied before releasing the next batch of data for annotation.
4. FDA Audit Trail: A Clear and Robust FDA-level Audit Trail
Regulatory processes for releasing a model into a clinical setting expect data about intra-rater reliability as well as inter-rater reliability, so it’s important to have this test built into the process and budget from the start.
Alongside this, a robust audit trail for every label created and applied, the ontological structure, and a record of who accessed the data is crucial.
When seeking FDA approval, you can’t leave anything to chance. That’s why medical organizations and companies creating solutions for that sector are turning to Encord for the tools they need for healthcare imaging annotation, labeling, and active learning.
As one AI customer explained about why they’ve signed-up to Encord: “We went through the process of trying out each platform– uploading a test case and labeling a particular pathology,” says Dr. Ryan Mason, a neuroradiologist overseeing annotations at RapidAI.
MRI Mismatch analysis using RapidAI
5. Quality Management System (QMS): Quality Control and Validation Studies
Next comes the rigors of quality control and validation studies. In other words, making sure that the labels that have been applied meet the standards the project needs, especially with FDA approval in mind.
Loop in medical experts as needed while being mindful of the project timeline, and use this data to train the model. Start accelerating the training cycles using iterative learning, or human-in-the-loop strategies, whichever method is the most effective to achieve the required results.
6. FDA Post-Market Surveillance: Continuous AI Model Maintenance and Ongoing Model Updates
Ensure an active data pipeline is established with robust quality assurance built in. And then get the model production-ready once it can accurately analyze and detect the relevant objects in the images in a real-world medical setting. At this stage, you can accelerate the training and testing cycles.
Once the model is production-ready, it can be deployed in the medical device or other healthcare application it’s being built for, and then the organization you’re working with can submit it along with their solution for FDA approval.
Bonus: Obtaining and Maintaining FDA Approval with Open-source or In-house tools
Although there are numerous open-source tools on the market that support medical image datasets, including 3DSlicer, ITK-Snap, MITK Workbench, RIL-Contour, Sefexa, and several others, organizations seeking FDA approval should be cautious about using them.
And the same goes for using in-house tools. There are three main arguments against using in-house or open-source software for annotation and labeling when going through the FDA approval process:
1. Unable to effectively scale your annotation activity
2. Weak data security makes FDA certification harder
3. You can’t effectively monitor your annotators or establish the kind of data audit trails that the FDA will need to see.
For more information, here’s why open-source tools could negatively impact medical data annotation projects.
FDA AI Approval: Conclusion & Key Takeaways
Going through the FDA approval process, as several of our clients have⏤including Viz AI and RapidAI⏤is time-consuming and requires higher levels of data security, quality assurance, and traceability of how medical datasets move through the annotation and model training pipeline.
When building and training a model, you need to take the following steps:
- Create or source FDA-compliant medical imaging or video-based datasets;
- Annotate and label the data (high-quality data and labels are essential);
- Review Medical expert review of labels in medical image/video-based datasets;
- A clear and robust FDA-level audit trail;
- Quality control and validation studies;
- Test your models on the data, and figure out what data you need more of/less of to improve your models.
Encord has developed our medical imaging dataset annotation software in close collaboration with medical professionals and healthcare data scientists, giving you a powerful automated image annotation suite, fully auditable data, and powerful labeling protocols.
AI FDA Regulatory Approval FAQs
For more information, here are a couple of FAQs on FDA approval for AI models and software or devices that use artificial intelligence.
What’s the FDA's current thinking on approving AI?
For product owners, AI software developers, and anyone wondering whether they need FDA approval, it’s also worth referring to the following published guideline documents and reports:
- Policy for Device Software Functions and Mobile Medical Applications
- General Wellness: Policy for Low Risk Devices
- Changes to Existing Medical Software Policies Resulting from Section 3060 of the 21st Century Cures Act
- Medical Device Data Systems, Medical Image Storage Devices, and Medical Image Communications Devices
- Clinical Decision Support Software
What’s the FDA’s role in regulating AI algorithms?
The FDA does play a role in regulating AI algorithms. However, that’s only if your algorithm requires regulatory approval.
In the majority of cases, providing it falls under the category of being a non-device CDS and is within the framework of the 21st Century Cures Act, then FDA approval isn’t needed.
Make sure to check the FDA’s Digital Health Policy Navigator or contact them for clarification:
Division of Industry and Consumer Education (DICE) at 1-800-638-2041 or DICE@fda.hhs.gov.
Contact The Digital Health Center of Excellence at DigitalHealth@fda.hhs.gov.
📌 Try Encord Free – The active learning platform trusted by industry leaders.
Join the world’s leading computer vision teams and bring your AI projects to production faster.
Want to stay updated?
- Follow us on Twitter and LinkedIn for more content on computer vision, training data, and active learning.
- Join our Discord Channel to chat and connect.
Data infrastructure for multimodal AI
Click around the platform to see the product in action.
Explore the platformWritten by
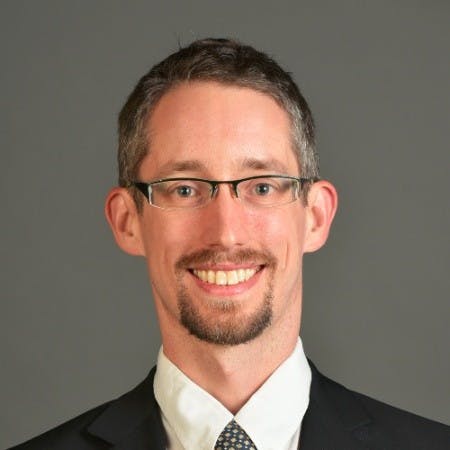
Dr. Andreas Heindl
Explore our products
- FDA approval ensures that AI models used in healthcare meet stringent standards for safety, efficacy, and data security. This is crucial when algorithms are used in clinical decision-making, diagnostics, or treatment processes.
- No. Many AI models, especially those providing general guidance or support, are exempt under the 21st Century Cures Act, as long as healthcare providers can independently review the model’s recommendations and it doesn’t directly influence diagnostic or treatment decisions.
- AI models fall into the Clinical Decision Support Software (CDS) category. To qualify as a non-device CDS (and avoid FDA regulation), your software must: - Not acquire or process medical images, signals, or patterns. - Present data that healthcare professionals typically exchange. - Provide recommendations rather than specific actions. - Allow healthcare professionals to independently review the recommendations. Refer to the FDA Digital Health Policy Navigator for clarification.
- The FDA is focused on algorithms in radiology (e.g., image-based diagnostics) and cardiology, as these areas benefit most from existing datasets and medical imaging advances. The agency also emphasizes clear audit trails, robust validation, and real-world evidence for approval.
- If your AI model does not meet FDA standards, you can revise it based on the agency’s feedback. Common issues include insufficient data quality, lack of audit trails, or inadequate validation studies.