Contents
How is Computer Vision Used in Healthcare?
What are The Benefits of Computer Vision in Healthcare?
What Types of Computer Vision Applications Are Currently in Use in Healthcare?
What's The Future For Computer Vision and Healthcare?
Encord Blog
Future for Computer Vision in Healthcare
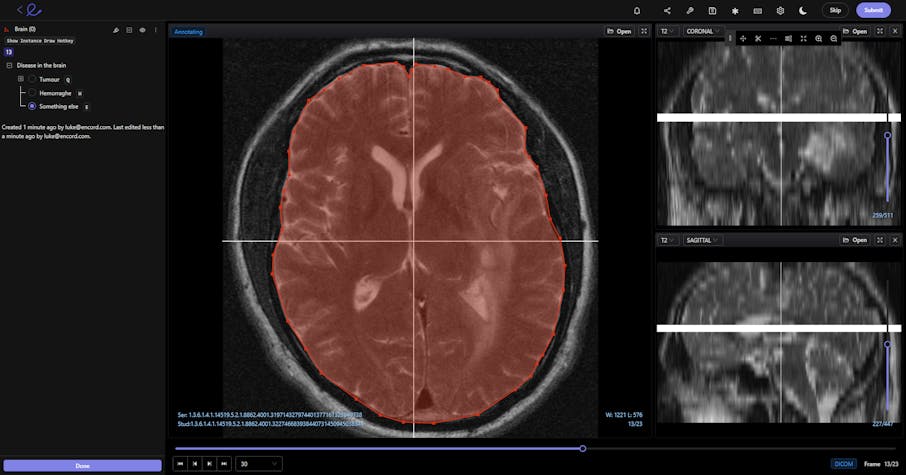
Computer Vision (CV) models and artificial intelligence algorithms (AI) are already playing an important role in numerous healthcare use cases across the medical sector and profession.
The application of computer vision, automation, and other algorithmically-generated models enables medical professionals to more effectively diagnose illnesses, viruses, and tumors, and have numerous other use cases that directly impact patient care.
A lot of work and resources go into training, deploying, and building computer vision models in healthcare to facilitate the application of computer vision in the medical sector. Including automation, that always plays a valuable role in annotation and labeling projects.
To start with, you need the right imaging or video-based datasets, and these need to be annotated and labeled. After that, your machine learning (ML) or data science teams need to train one or more artificial intelligence (AI), CV, or deep learning model until a high accuracy score is achieved, any bias is reduced, and it’s generating the results you need.
Once a CV model is in production, it will generate iterative feedback data from it being used on real-world annotated medical imaging or video-based datasets. This iterative feedback loop will help you train improved and enhanced versions of the model.
In this article, we take a closer look at how computer vision models are used in healthcare, the benefits of using CV in healthcare; alongside several examples of use cases Encord has been involved with, and what we expect for the future of computer vision in healthcare.
How is Computer Vision Used in Healthcare?
Computer vision and deep learning have numerous use cases in the healthcare industry, from medical research through to patient care and surgeries. Every CV and artificial intelligence model starts with datasets of medical images or videos from a wide range of sources, such as radiology, gastroenterology, histology, MRI machines, ultrasounds, and X-rays.
Wherever the images come from, datasets are usually in DICOM or NIfTI formats, and for these, your annotation team needs an annotation platform equipped to handle native medical imaging formats.
Training and deploying a production-ready CV model faster ensures medical professionals and organizations get more effective, efficient, and useful results from CV and deep learning projects. The outcomes of these projects directly impact patients’ treatment plans, medical research, and clinical drug trials. CV models are incredibly useful during the diagnosis process, patient care, and even during clinical operations. The role of IT services for healthcare is instrumental in ensuring these technologies are seamlessly integrated into existing systems, enhancing both the speed and accuracy of diagnoses and treatments.
What are The Benefits of Computer Vision in Healthcare?
Healthcare industry professionals and organizations are handling huge volumes of data. A lot of this data is in the form of images and videos of patients, scans during the diagnosis stage, and the treatment they’re receiving.
These images and videos provide valuable and life-changing insights, pieces of information, and new fields of study that would otherwise be overlooked by the human eye. CV, ML, and AI-based models are useful in numerous ways, as we will soon outline, including robotic surgeries.
What Types of Computer Vision Applications Are Currently in Use in Healthcare?
Healthcare already has hundreds of use cases and applications for computer vision models.
In medical abnormality detection, startups are deploying computer vision models to empower secondary insights from patient medical imaging scans. For example, patients might have had scans taken using an MRI machine with the aim of detecting a particular illness.
A scan comes back clear, at least for the illness a doctor was looking for. But what if there’s something else going on?
With medical image annotations and a production-ready CV model, these images can be processed again to search for other health issues that have been missed and overlooked on the first scan. Using computer topography, and powerful computer vision models, medical teams can benefit from a “second pair of eyes”; in this case, a highly-trained CV model.
With this, it’s more time and cost-effective when attempting to detect medical abnormalities in patients, producing more accurate diagnoses and helping to save more lives and deliver better treatment plans.
In gastrointestinal care (GI), computer vision models and ML are now being used in pre-trial screenings of patients for clinical trials and other forms of gastrointestinal care (GI).
GI healthcare providers can use computer vision models to analyze patient and clinical trial imaging datasets to improve the accuracy of the diagnosis process. Abnormalities are easier to highlight when a CV solution is trained to analyze thousands of images faster, more accurately, and in much greater detail than a human team ever could.
Neurovascular and vascular clinical teams are also making extensive use of computer vision models and AI-driven volumetric measurements.
For the human eye, even when using powerful microscopes, detecting life-threatening neurovascular and vascular conditions is a serious challenge. Medical teams need faster, more accurate, and proven approaches to detect, speed up, and improve treatment plans for strokes, aneurysms, and pulmonary embolisms.
Using AI and computer vision models, healthcare companies can empower physicians to make faster, more accurate diagnostic, treatment, and transfer decisions, improving the patient journey every step of the way. Ultimately, this is one of many examples in the healthcare sector where computer vision technology and trained models using annotated datasets are saving lives.
In digital cell morphology, some startups are using a revolutionary technique that is deploying computer vision models to automate the analysis of tens of thousands of cells faster than any human ever could. This is already being used in hematology to analyze and count the cells from smear tests and provide better care plans for patients.
Smear and other hematology tests usually only count a tiny number of cells visible under an electronic microscope. No matter how skilled the person who's looking at these cells or how powerful the microscope is, no one can come close to what a CV model can do when analyzing every single cell at a huge volume.
CV models, in this way, empower doctors to detect and diagnose health problems far earlier. Patients can access the care they need faster, resulting in more life-saving treatment.
In surgical robotics, computer vision models are being used to improve the outcomes of robotic surgery.
Robotic tools are more widely deployed in the healthcare sector. It’s a proven and safe technology, and they’re useful for reducing the invasiveness of surgery, minimizing risk and recovery time, and moving in ways that human surgeons can’t. At the same time, robotic surgeons still need to mimic humans as closely as possible, and that’s where computer vision models play an important role.
Computer vision models are useful for training robotic surgical tools and analyzing thousands of videos from endoscopic surgeries. CV and AI-based models are, therefore part of a robotic surgical tools training system, helping these devices become more precise and accurate, improving patient outcomes from surgery.
Now let’s consider what other future advances and innovations healthcare can expect and drive forward from computer vision models and providers in this field.
What's The Future For Computer Vision and Healthcare?
Future applications and use cases for computer vision in healthcare are only limited by human imagination and resources.
As we are working closely with medical professionals, healthcare data scientists, and numerous companies in this sector, we can already see progress in the following areas:
Deploying computer vision models for augmented reality (AR) treatments and consultations in the consumer market. Integrating ehr software development within computer vision applications in healthcare could enhance the capabilities of AR consultations, offering more precise and personalized medical advice based on real-time data analysis. Imagine having a remote consultation with a doctor using augmented reality. It sounds like something out of science fiction, but it could happen sooner than we might imagine.
Using AR and CV, remote consultations could be just as useful as in-person appointments, if not more so, because doctors could be empowered with CV models to make faster and more accurate diagnoses of patients. Additionally, implementing hospitality iot solutions can enhance the efficiency of these models, offering more precise management of healthcare resources and improving patient care coordination.
In hospitals and treatment centers too, doctors and medical staff could soon be wearing AR/AI-powered medical glasses. Bringing all of the power of AI and CV-based models into the patient experience. Enhanced accuracy would reduce mistakes and improve patient access to the right treatment plans, saving lives, time, and money.
We are also seeing advances in the use of AI-based models in microscopy and more complicated medical subsets, going beyond many of the original radiology use cases. Healthcare providers are also looking at ways to use AI, when combined with data from wearable devices and at-home test kits, to keep patients healthier. Preventative actions rather than treating patients only after they get sick would make a huge difference to the entire sector and the cost of maintaining healthcare for an increasingly aging population.
At the same time, we are seeing a shift towards making more medical datasets public to reduce AI bias in the model training and development stage. Outside of academia, a new generation of startups is building healthcare solutions for specific demographic and ethnic groups, overcoming unintentional bias in a more direct way.
Computer vision models could also be used to segment patient groups based on treatment needs. The segmentation and personalization of healthcare and medicines is a huge growth area for the healthcare sector. Not only would it improve patient outcomes, but it could be valuable for generating more revenue for healthcare providers.
In the hands of the medical profession, computer vision models are already making significant positive impacts on the patient experience. It’s exciting for the entire profession and sector to see what computer vision will do next and what future use cases we can expect over the next decade.
Encord has developed our medical imaging dataset annotation software in close collaboration with medical professionals and healthcare data scientists, giving you a powerful automated image annotation suite, fully auditable data, and powerful labeling protocols.
Experience Encord in action. Dramatically reduce manual video annotation tasks, generating massive savings and efficiencies. Try it for Free Today.
Data infrastructure for multimodal AI
Click around the platform to see the product in action.
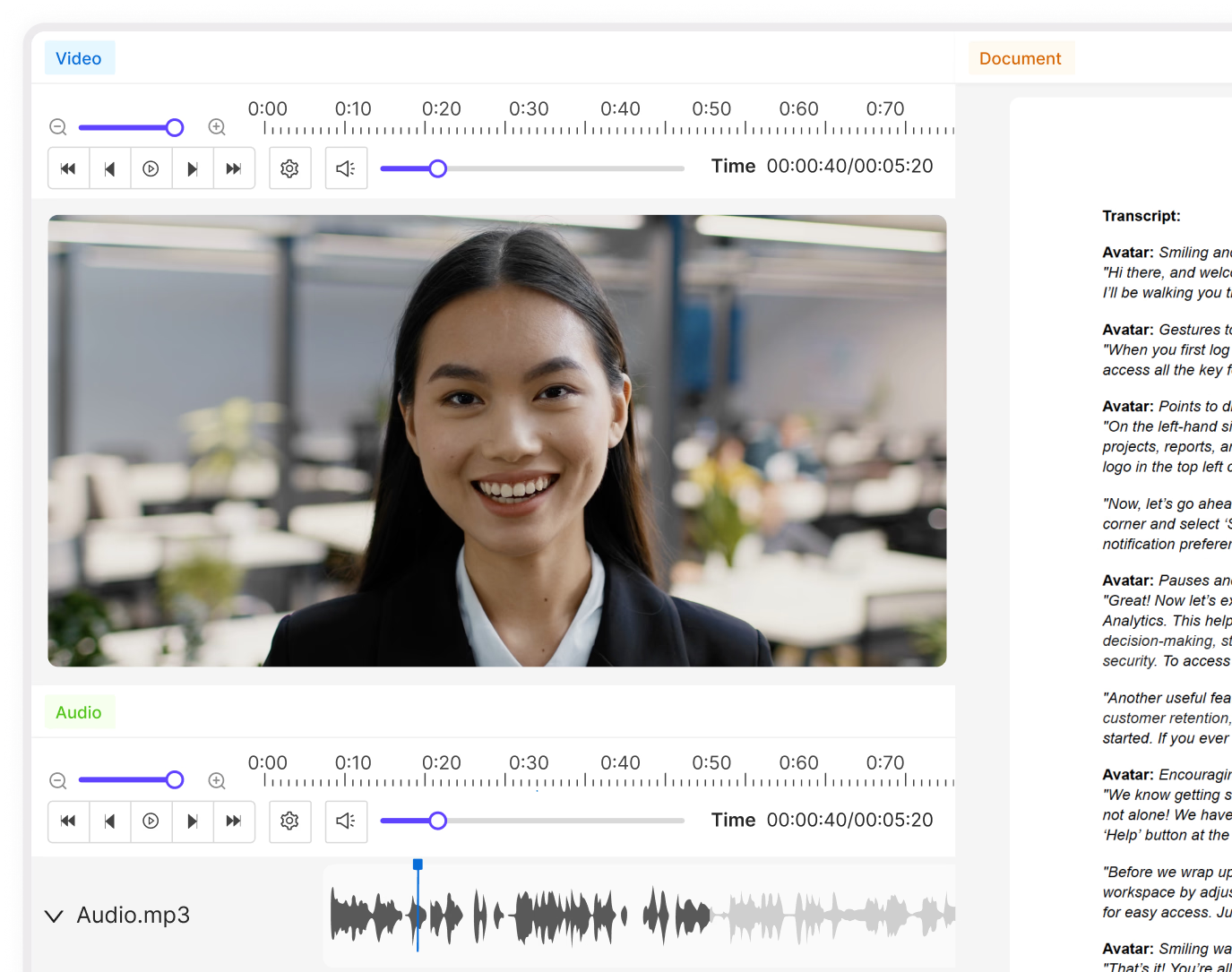
Written by
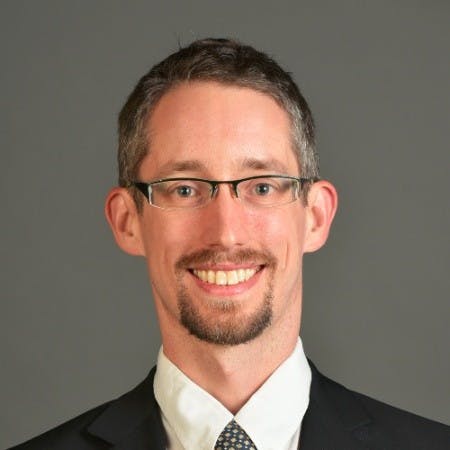
Dr. Andreas Heindl
Explore our products