Contents
Examples of multimodal medical AI data
Key Challenges in Integrating Multimodal Medical Data
The Importance of Labeling in Multimodal Medical AI
How to Label and Analyze Multimodal Medical Files
Key Takeaways
Encord Blog
How to Label and Analyze Multimodal Medical AI Data
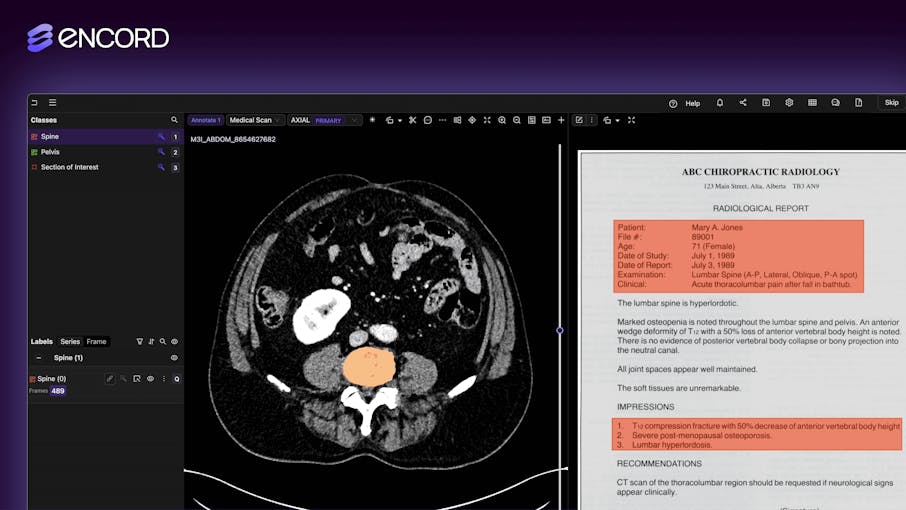
Discover how platforms like Encord are revolutionizing multimodal data labeling, empowering medical AI teams to unlock groundbreaking insights and improve patient outcomes.
Labeling multimodal data is becoming crucial across various fields, especially in the medical industry. Developing sophisticated AI models now demands multimodal datasets, which involve processing audio, video, text, medical imaging, and other data types within a unified, consistent structure.
With the recent addition of robust platform support for document and audio data, alongside the multimodal annotation editor, Encord is now empowering customers to seamlessly manage and label these complex multimodal datasets.
Examples of multimodal medical AI data
What does multimodal mean for the medical industry? Multimodal medical data can take several forms:
DICOM Files and Medical Imaging (CT, MRI, X-rays)
Medical imaging, stored in the DICOM (Digital Imaging and Communications in Medicine) format, forms the backbone of modern healthcare. By labeling these images alongside their corresponding reports, AI systems can be trained to correlate visual features with the descriptive information in the reports, enabling more accurate and insightful analyses.
Electronic Health Records (EHR), Lab Results, and Genomic Data
EHRs consolidate a patient’s medical history, lab results, and treatment records, while genomic data offers insights into genetic predispositions and potential responses to therapies. Together, these multimodal datasets enable personalized medicine, allowing AI to predict disease progression, recommend treatments, and optimize patient outcomes by combining genetic, biochemical, and historical data.
Textual Data from Clinical Notes and Reports
Physicians often document observations, diagnoses, and treatment plans in free-text clinical notes and reports. Natural language processing (NLP) algorithms process these unstructured texts to extract valuable information, such as symptoms, medications, and treatment responses. When integrated with structured data, this enhances AI's ability to provide comprehensive patient assessments and treatment recommendations.
Key Challenges in Integrating Multimodal Medical Data
Integrating multimodal medical data presents several challenges that can hinder the development of effective AI solutions.
Synchronizing Imaging Data
Synchronizing imaging data, like CT or MRI scans, with non-imaging data, such as lab results or genomic information, involves aligning information from fundamentally different modalities, each with unique formats, structures, and temporal contexts. Imaging data is often large, high-dimensional, and requires precise metadata, such as timestamps, acquisition parameters, or patient positioning, to make sense of the images. Non-imaging data, on the other hand, is typically structured as numerical results, textual reports, or categorical labels, which may have been collected at different times, under varying conditions, or in separate systems.
The complexity arises in ensuring that these disparate data types are properly aligned for meaningful analysis. For instance, a lab test result may need to be linked to an MRI scan taken on the same day to establish a correlation, but mismatched timestamps or incomplete metadata can hinder this process.
Without robust synchronization, multimodal datasets risk losing context, making it challenging for AI models to learn accurate relationships between data types. This underscores the importance of tools and platforms that can automate and streamline the process of aligning and synchronizing multimodal data while preserving its clinical integrity.
Inconsistency Across Different Data Types
Another significant challenge is the inconsistency in formats and metadata across different data types—such as DICOM files, EHRs, and textual clinical notes. These variations make it difficult to establish a unified structure for data analysis and processing, as each data type comes with its own standards, organization, and level of detail. Overcoming these discrepancies is critical to ensure seamless integration and meaningful insights.
The Importance of Labeling in Multimodal Medical AI
Labeling is at the heart of developing successful multimodal medical AI systems. High-quality labels allow models to identify patterns, make accurate predictions, and generate reliable insights, which is especially critical in healthcare where precision is paramount.
Labels for DICOM files, for example, must accurately reflect the clinical context and imaging features to guide model predictions. Annotating medical images and text also presents unique difficulties due to the complexity and diversity of data, requiring experts to handle subtle differences and ambiguous cases. The role of medical experts in this process cannot be overstated, as their domain knowledge ensures that annotations are both precise and clinically relevant. Accurate labeling is not just a technical requirement—it is a cornerstone of building AI systems that can deliver impactful, life-saving solutions in the medical field.
How to Label and Analyze Multimodal Medical Files
Encord offers a powerful solution for labeling multimodal medical data. In this guide, we’ll demonstrate its capabilities using an example that combines a CT scan with its corresponding medical report.
Create a multimodal dataset
First you must create a dataset in Encord that contains your multimodal medical files. Upload your files to Encord, and add them to a dataset. See our documentation for more information on uploading files and creating datasets.
Ensure that you add custom metadata to your files so that they can be displayed correctly in the label editor. Documentation on how to add custom metadata can be found here.
Create an ontology to label your files
Next you must create an ontology to define what labels you can apply to files in your dataset. For our CT scan & medical report example the ontology contains bitmask objects for `Spine` and `Pelvis` to label the CT scan, as well as a bounding box for labeling a `Section of interest` in the PDF file. See our documentation here for more information on creating ontologies in Encord.
Create a project
Once your dataset and ontology are ready, the next step is to create a project. Choose or create a workflow to suit your needs, then attach your dataset and ontology to the project to get started. For more information on creating projects, see our documentation here.
Set a custom editor layout
In this example, we aim to label the CT scan alongside its corresponding medical report. To achieve this, we need to design a custom editor layout that displays the files side-by-side in the label editor. This layout leverages the custom metadata added to each file either during or after the upload process in Encord.
For more information on creating custom editor layouts and attaching them to a project, see the end-to-end example in our documentation here.
Label your data
With everything ready, it’s time to start labeling your data. Open a DICOM file from the task queue to view it in the label editor alongside its corresponding medical report.
Apply labels to the currently selected file. To add labels to the related file, click Annotate this tile before proceeding. Once both files are labeled, click Submit to finalize and submit them. Both files will then move to the review queue for further processing. See our documentation on how to label for more information.
Key Takeaways
- Multimodal medical AI data is diverse and complex: From DICOM files and imaging data to electronic health records and clinical notes, integrating and analyzing these diverse modalities is critical for advancing healthcare AI.
- Data labeling is foundational for success: Structured and accurately labeled datasets are essential for training high-performing AI models, especially in the medical field, where precision is paramount.
- Challenges of multimodal data require robust solutions: Issues like inconsistent formats, metadata mismatches, and synchronizing imaging with non-imaging data add complexity, but advanced platforms such as Encord can streamline these processes.
- High-quality annotations demand expertise and tools: Creating reliable labels for medical data often requires collaboration with domain experts and specialized tools, ensuring datasets are both accurate and clinically relevant.
- Platforms like Encord simplify multimodal data workflows: By supporting multiple data modalities, synchronizing disparate data types, and offering integrated annotation tools, Encord helps medical AI teams accelerate development and improve model performance.
Better Data, Better AI
Enhance your AI with automated data curation, annotation, and validation.
Try it todayWritten by

David Babuschkin
- Multimodal medical data refers to diverse data types used in healthcare AI, including medical imaging (CT, MRI, X-rays), Electronic Health Records (EHRs), genomic data, and textual data from clinical notes and reports. These different types of data must be integrated and labeled for AI models to analyze and make predictions.
- Multimodal data provides a more comprehensive understanding of a patient's health by combining different types of information, such as images, clinical notes, and lab results. This integration helps AI models offer more accurate predictions, enhance diagnoses, and personalize treatment plans.
- DICOM Files & Medical Imaging: CT scans, MRIs, and X-rays stored in DICOM format. Electronic Health Records (EHRs): Patient history, lab results, and treatment records. Genomic Data: Insights into genetic predispositions and therapy responses. Clinical Notes: Textual data from physicians documenting observations and treatment plans.
- Labeling multimodal medical data often requires collaboration with domain experts, such as radiologists and physicians, who ensure that labels are clinically accurate. Specialized tools, like Encord, also help facilitate this process by offering advanced features and automating parts of the workflow.
Explore our products