The fastest way to manage,
curate and annotate AI data
Manage, curate, and label multimodal data such as image, video, audio, document, text and DICOM files – all on one platform. Transform petabytes of unstructured data into high quality data for training, fine-tuning, and aligning AI models, fast.
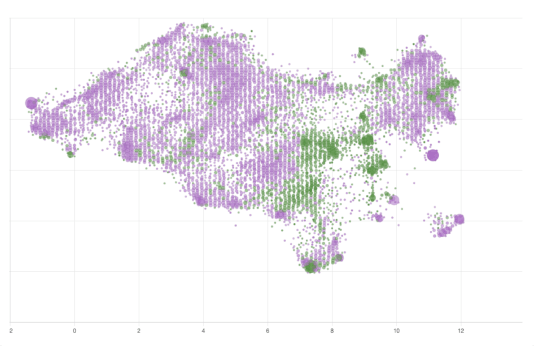
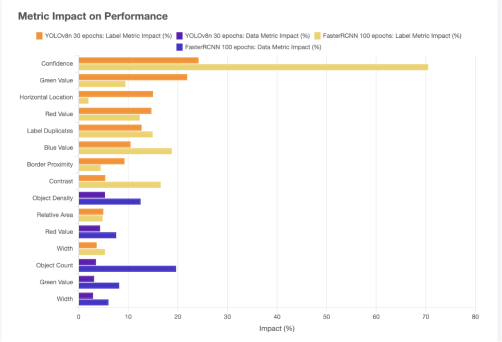
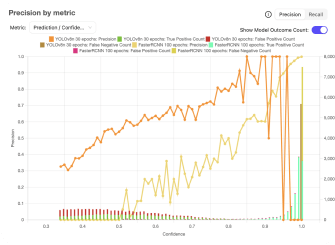