Contents
Overview of SAM 2
Overview of SA-V Dataset
Understanding SAM 2 Data Engine
Creating Your Own Dataset with SAM 2 and Encord
Encord Blog
How SAM 2 and Encord Transforms Video Annotation
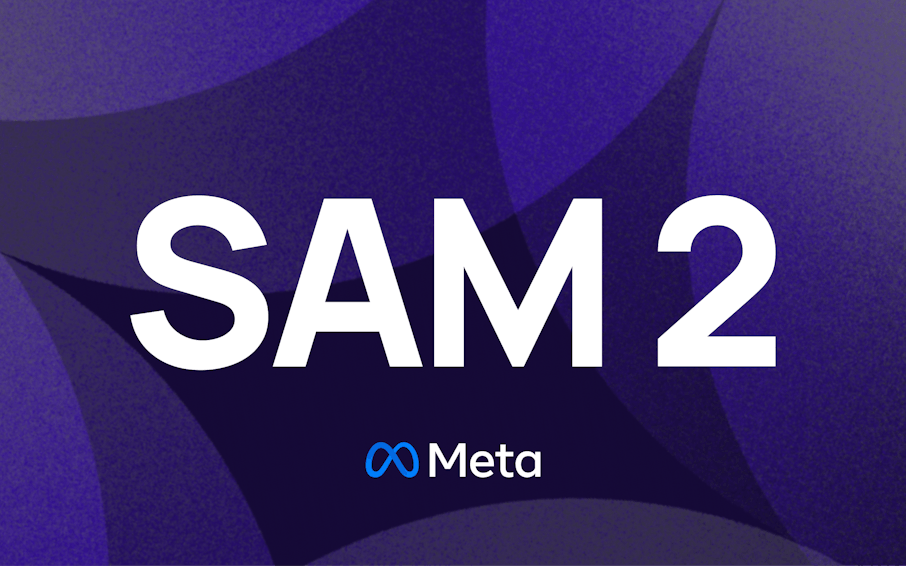
The future of video annotation in here! In this blog, we'll explore how Meta's Segment Anything Model 2 (SAM 2) revolutionizes dataset creation and annotation. Whether you're working on image or video data, SAM 2's enhanced capabilities promise to streamline and elevate your annotation tasks.
We'll dive into the powerful features of SAM 2, the creation of the extensive SA-V dataset, and show you how to harness these tools through Encord to curate your own datasets efficiently. Ready to transform your annotation workflows? Let's get started!
Overview of SAM 2
SAM 2 is Meta’s new foundation model that extends the capabilities of the original Segment Anything Model (SAM) into the video domain. Designed to handle both static images and dynamic video content, SAM 2 integrates advanced segmentation and tracking functionalities within a single, efficient framework. Key features include:
- Real-Time Object Tracking: SAM 2 can track objects consistently across video frames in real-time, ensuring accurate segmentation even when objects temporarily disappear from view.
- Memory Module: The model includes a per-session memory module that maintains and tracks selected objects across all frames of a video. This memory system enables dynamic corrections and adjustments based on supplementary prompts, enhancing the accuracy and reliability of segmentation.
- Improved Performance and Efficiency: Building on the original SAM, SAM 2 offers enhanced performance with faster inference speeds, making it highly accessible and practical for a wide range of applications, from video editing to mixed reality experiences.
Overview of SA-V Dataset
The SA-V dataset is a large and diverse collection of video annotations created using the SAM 2 data engine. This dataset is designed to support the development and evaluation of advanced video segmentation models. Key aspects of the SA-V dataset include:
- Extensive Coverage: The SA-V dataset includes a vast array of objects and object parts, ensuring comprehensive coverage that facilitates the training of models capable of "segmenting anything" in videos.
- High-Quality Annotations: The dataset was created through a meticulous annotation process involving human annotators and the SAM 2 model. This process ensures high-quality, precise segmentations across all frames of the videos.
- Interactive Annotation Workflow: The data engine employs an interactive model-in-the-loop setup, allowing annotators to refine and correct mask predictions dynamically. This approach significantly speeds up the annotation process while maintaining accuracy.
- Verification and Quality Control: To uphold annotation quality, a verification step is incorporated where separate annotators assess and confirm the accuracy of each masklet. Unsatisfactory annotations are refined or rejected to ensure consistency and reliability.
SAM 2: Segment Anything in Images and Videos
Understanding SAM 2 Data Engine
When starting a project that requires comprehensive video segmentation, having a large and diverse dataset is important. However, starting from scratch can be challenging due to initial lack of data needed to train a robust model. That’s why SAM 2 data engine was introduced. It is designed to address this problem by progressively building up a high-quality dataset and improving annotation efficiency over time.
This engine employs an interactive model-in-the-loop setup with human annotators without imposing semantic constraints on the annotated masklets. The focus is on both whole objects (e.g., a person) and parts (e.g., a person’s hat). The data engine operates in three distinct phases, each categorized by the level of model assistance provided to annotators. Here, are the phases and the resulting SA-V dataset:
Phase 1: SAM Per Frame
The initial phase used the image-based interactive SAM to assist human annotation. Annotators were tasked with annotating the mask of a target object in every frame of the video at 6 frames per second (FPS) using SAM, along with pixel-precise manual editing tools such as a “brush” and “eraser.” No tracking model was involved to assist with the temporal propagation of masks to other frames.
As this is a per-frame method, all frames required mask annotation from scratch, making the process slow, with an average annotation time of 37.8 seconds per frame. However, this method yielded high-quality spatial annotations per frame. During this phase, 16K masklets were collected across 1.4K videos. This approach was also used to annotate the SA-V validation and test sets to mitigate potential biases of SAM 2 during evaluation.
Phase 2: SAM + SAM 2 Mask
The second phase introduced SAM 2 into the loop, where SAM 2 only accepted masks as prompts, referred to as SAM 2 Mask. Annotators used SAM and other tools as in Phase 1 to generate spatial masks in the first frame, then used SAM 2 Mask to temporally propagate the annotated mask to other frames, creating full spatio-temporal masklets. At any subsequent video frame, annotators could spatially modify the predictions made by SAM 2 Mask by annotating a mask from scratch with SAM, a “brush,” and/or “eraser,” and re-propagate with SAM 2 Mask, repeating this process until the masklet was correct.
SAM 2 Mask was initially trained on Phase 1 data and publicly available datasets. During Phase 2, SAM 2 Mask was re-trained and updated twice using the collected data. This phase resulted in 63.5K masklets, reducing the annotation time to 7.4 seconds per frame, a ~5.1x speedup over Phase 1. However, this decoupled approach still required annotating masks in intermediate frames from scratch, without previous memory.
Phase 3: SAM 2
In the final phase, the fully-featured SAM 2 was utilized, accepting various types of prompts, including points and masks. SAM 2 benefited from memories of objects across the temporal dimension to generate mask predictions, allowing annotators to provide occasional refinement clicks to edit the predicted masklets in intermediate frames, rather than annotating from scratch. SAM 2 was re-trained and updated using the collected annotations five times during this phase. With SAM 2 in the loop, the annotation time per frame decreased to 4.5 seconds, an ~8.4x speedup over Phase 1. This phase produced 197.0K masklets.
Annotation guideline overview. SAM 2: Segment Anything in Images and Videos
Ensuring Quality and Diversity: Verification, Auto Masklet Generation, and Analysis
To maintain high annotation standards, a verification step was introduced. A separate set of annotators assessed each masklet's quality, categorizing them as “satisfactory” (correctly tracking the target object across all frames) or “unsatisfactory” (well-defined boundary but inconsistent tracking). Unsatisfactory masklets were sent back for refinement, while those tracking poorly defined objects were rejected entirely.
To ensure diverse annotations, automatically generated masklets (referred to as “Auto”) were added. SAM 2 was prompted with a grid of points in the first frame to create candidate masklets, which were then verified. Satisfactory auto masklets were included in the SA-V dataset, while unsatisfactory ones were refined by annotators in Phase 3. These masklets covered both prominent central objects and varying background objects.
Comparative analysis across data engine phases showed increased efficiency and maintained quality. Phase 3, using SAM 2, was 8.4x faster than Phase 1, had fewer edited frames per masklet, and required fewer clicks per frame, resulting in better alignment. Performance comparisons of SAM 2, trained on data from each phase, revealed consistent improvements. Evaluations on the SA-V validation set and nine zero-shot benchmarks demonstrated the benefits of iterative data collection and model refinement, with performance measured using the J & F accuracy metric showing significant gains as shown in the table below.
SAM 2: Segment Anything in Images and Videos
Creating Your Own Dataset with SAM 2 and Encord
If you want to use SAM 2 right away to curate your own dataset, you are in luck. Meta’s latest and greatest, SAM 2, is now part of Encord’s automated labeling suite—only one day after its official release! ⚡
Whether you're working on images or videos, SAM 2 promises to enhance both accuracy and efficiency, making your annotation tasks smoother and more effective.
Ready to dive in? Here’s how you can start creating your own dataset using SAM 2 and Encord:
Enable SAM 2
Head over to Encord Labs in your settings and flip the switch to activate SAM 2, as shown in our documentation. Look for the new magic wand icon in the editor—it means you’re all set to use SAM 2’s latest features.
Use Encord Agents for Image or Video Segmentation
Begin your annotation projects with SAM 2 for image or video segmentation. You can use SAM 2 to further enhance video annotation. You’ll also notice a significant boost in speed and precision, streamlining your workflow and improving annotation quality.
Want to master SAM 2? Join our webinar, "How to Fine-Tune SAM 2," for expert tips on customizing and optimizing your model.
Register now to secure your spot and take your SAM 2 skills to the next level!
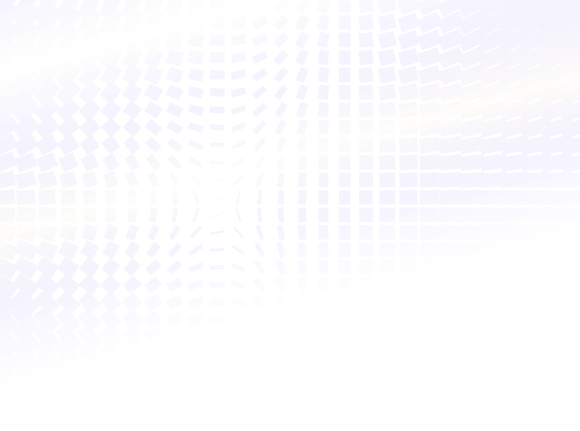
Power your AI models with the right data
Automate your data curation, annotation and label validation workflows.
Book a demoWritten by

Akruti Acharya
Explore our products