Contents
Key Technologies and Trends
Applications of Computer Vision in Logistics and Supply Chain
Case Studies and Examples
Implementation Challenges
Computer Vision in Logistics and Supply Chain: Key Takeaways
Encord Blog
Applications of Computer Vision in Logistics and Supply Chain (2024)
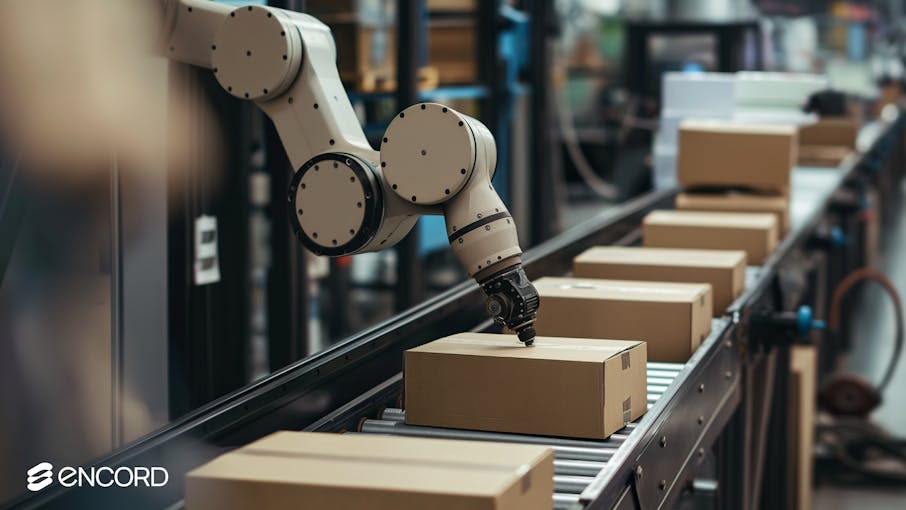
Reports predict artificial intelligence (AI) will be worth $17.5 billion in the supply chain management domain by 2028 as the demand for AI-based solutions increases. Additionally, more than 50% of supply chain organizations will invest in AI systems through 2024 to process extensive datasets and identify areas for improvement.
One key driving element behind the rapid growth of AI in logistics and supply is the emergence of advanced computer vision (CV) frameworks, which enable organizations to automate their supply chain networks and boost productivity.
CV algorithms allow manufacturers to use robots and automated detection systems to streamline manufacturing and supply chain workflows and save significantly on labor costs. A recent survey suggests that using robotics can reduce manufacturing and labor costs by 25% to 30%.
Computer vision is revolutionizing various aspects of logistics and supply chains, from inventory management and warehouse safety to transportation optimization and quality control. CV-powered systems can automatically track inventory levels, monitor worker safety, optimize delivery routes, and detect product defects, among other applications.
In this article, we will discuss the applications of computer vision technology in logistics and supply chains to help you understand how the industry is evolving and how you can improve supply chain operations.
Key Technologies and Trends
As AI and machine learning (ML) evolve in the current digitization era, AI applications in supply chain and logistics will become more prevalent in organizations that want to maintain a competitive edge.
Below are the key technologies and trends revolutionizing the modern supply chain management system.
Deep Learning and AI Integration
The advent of deep learning algorithms allows supply chain managers to analyze extensive data regarding consumers, inventory, and business processes. For instance, advanced CV algorithms can detect inventory levels in real-time and alert relevant team members in case of shortages.
AI and ML models also enable demand forecasting, predictive maintenance, and route optimization, which can lead to greater efficiency and cost savings.
Edge Computing and the Internet of Things (IoT)
Advancements in edge computing allow manufacturers to quickly collect and analyze data regarding floor operations, environmental conditions, and delivery processes using cameras and sensors.
It provides them with real-time information to help them understand operational roadblocks and identify areas for optimization. IoT devices enable end-to-end supply chain visibility and support data-driven decision-making.
3D Modeling and Digital Twins
Digital twins are virtual replicas of physical objects, assets, and processes that allow users to develop accurate simulations. With 3D models and digital twins, manufacturers build realistic scenarios and simulate processes to understand how different interventions will impact operations.
Digital Twin of General Electric’s Gas Turbine
The technology helps them design better floor plans and schedules, coordinate tasks more efficiently, enable predictive maintenance, and enhance resilience planning. Digital twins provide complete supply chain visibility and aid in risk management.
Applications of Computer Vision in Logistics and Supply Chain
With the recent surge in advanced computer vision systems and AI-as-a-Service (AIaaS) providers offering multiple solutions, businesses find it challenging to understand how and where AI helps manufacturers improve operational efficiency.
Modern deep learning architectures, such as Convolutional Neural Networks (CNNs) and YOLO, enable accurate object detection, which forms the foundation for various CV applications in logistics.
The following sections discuss the core and advanced computer vision applications in supply chain and logistics to help you determine how AI can benefit your business.
Core Applications
The CV’s core supply and logistics applications include automated inventory management, quality controls, automated sorting and handling, traceability and tracking, and security and surveillance.
Automated Inventory Management
CV systems can track inventory items on shelves in real-time to avoid shortages. For instance, cameras use optical character recognition (OCR) algorithms to scan barcodes and immediately update inventory levels.
Manufacturers can also link the system to their enterprise resource planning platforms for real-time demand forecasting. Edge computing enables real-time processing and decision-making for inventory management applications.
In addition, AI-based robots help optimize warehousing operations by capturing visual data to track packaged goods for streamlined delivery to distribution centers.
Quality Controls
Cameras and sensors installed on the assembly line and packaging systems allow manufacturers to automate defect detection. The technology helps avoid accidents and improve customer satisfaction as the system flags items with anomalies.
In addition, CV algorithms can detect the quality of documentation on packaged items by comparing it against industry standards.
Relevant documents can include invoices, shipping labels, and customs-related information. The technology prevents items with missing or inadequate documents from reaching the distribution facility.
Automated Sorting and Handling
Autonomous vehicles, such as automated guided vehicles (AGVs) and autonomous mobile robots (AMRs), significantly help sort items and handle materials.
For instance, AMRs equipped with robotic arms can pick items from storage sites and deliver them to docking stations without manual input.
They can adjust material placement according to size and weight and ensure quick order fulfillment by determining the fastest route from source to destination.
Traceability and Tracking
CV systems can trace the movement of products and materials across the warehouse to keep track of their count and locations. This method can improve delivery times and localize objects in real-time.
Object detection models like YOLO can accurately identify and track multiple objects in a scene.
CV algorithms can also detect the number and type of vehicles and containers entering and leaving the warehouse and docking stations. This process will allow manufacturers to record delivered and pending orders accurately.
Security and Surveillance
Logistics facilities can be vulnerable to intrusion and safety hazards as they may store heavy machinery, sensitive equipment, and hazardous materials.
Cameras with embedded CV algorithms can quickly detect anomalous behavior and alert the relevant authorities to prevent accidents. Edge AI enables real-time processing for security applications.
It can also monitor whether staff wear protective equipment when handling dangerous materials to avoid injuries.
The system can also prevent vehicles or people from entering restricted areas by identifying unauthorized access attempts through facial recognition and object detection algorithms.
Advanced Applications
While the above applications help logistics companies implement the core building blocks for warehouse automation, vision solutions also offer advanced functionalities to boost cost savings.
The following sections briefly discuss these advanced use cases to help you understand how you can further improve warehouse management.
Predictive Maintenance
AI algorithms can monitor equipment conditions and determine if it needs maintenance. The algorithms can also predict maintenance schedules and recommend ways to reduce maintenance time and cost.
Vision algorithms can inspect large-scale machinery to identify faults by capturing depth information using 3D cameras. Early detection can help avoid downtime and allow workers to take the necessary precautions when implementing the required fixes.
Route Optimization and Fleet Management
CV systems can collect and analyze extensive data regarding floor layouts, vehicle routes, and incoming obstacles.
AI algorithms can process the data to extract deep insights for optimizing routes. They ensure the vehicles take the shortest path from a particular source to a destination. Digital twins and 3D modeling aid in simulating and optimizing routes.
Vehicles equipped with cameras can use vision systems to detect the correct type of item to carry automatically. This method will help prolong the vehicle’s lifespan by requiring it to carry only items of the weight and size it can support.
Occupancy and Space Utilization
Efficient storage space utilization is crucial for optimizing logistics operations. The process requires monitoring empty spaces and eliminating idle gaps in the warehouse. Moreover, the effective allocation of vehicles is necessary to minimize the time needed to deliver equipment and products. 3D modeling helps optimize warehouse layouts and space utilization.
CV algorithms can determine when and where vehicles will unload items and be ready for another task.
The system can also coordinate vehicle movement across the factory floor to avoid traffic disruptions and detect suitable storage spaces to store idle equipment.
Process Modeling and Simulation
Cameras and sensors installed in the warehouse can send real-time images and video feeds to AI algorithms that process the data for predictive analytics. The analysis can reveal critical insights regarding bottlenecks and delays, allowing the relevant team to resolve issues quickly. Digital twins enable realistic process simulations.
Manufacturers can also use the data to create accurate simulation models. These simulations will allow them to study the effect of different interventions on cost, time, and throughput.
Eventually, the information will be valuable for planning upgrades and maintenance schedules, boosting operational efficiency, and minimizing human error.
Case Studies and Examples
Amazon, Tesla, and DHL’s automated supply chain solutions are prime examples of how robotics with advanced CV capabilities is transforming warehousing and logistics operations.
Amazon’s Digit
Amazon’s warehouses now use a humanoid robot called Digit. This robot picks up yellow bins from a shelf and repeatedly delivers them to a conveyor.
While Digit is a remarkable breakthrough in warehouse automation, Amazon invested heavily in Kiva robots almost a decade ago, which allowed the company to reduce stock retrieval time from 90 to 15 minutes.
These robots can carry loads weighing up to 140 kg and pick up and deliver items to factory workers.
Tesla’s Optimus
Tesla recently revealed its revolutionary humanoid robot, Optimus. The company expects the robot to work in the factory by the end of 2024. Optimus is designed to perform tasks that are unsafe, repetitive, or boring, supporting the company's ambitious automation goals.
Tesla’s Optimus Robots Sorting Batteries
Tesla's aggressive automation strategy began in 2016 with its "Alien Dreadnoughts" initiative, aiming to automate car manufacturing extensively.
The company aimed to build these robots to replace human car manufacturing operators completely.
While the move initially resulted in heavy losses, the company eventually got a significant return on investments (ROI) when its valuation reached a trillion dollars in April 2022.
DHL’s Smart Glasses
DHL's automation strategy involves using mixed-reality smart glasses to boost worker productivity. These glasses use augmented reality (AR) to display task information, enabling hands-free picking and reducing errors.
The smart glasses offer step-by-step instructions to employees, helping them complete tasks more accurately. Experts can also connect with on-site workers via video call to provide remote guidance through annotations and sketches.
DHL Express even offers gamified training simulations using mixed reality, where workers learn to load pallets and containers optimally.
The simulations award bonus points for efficient space utilization and attention to labels, making training more engaging and effective.
Implementation Challenges
The above case studies and examples demonstrate the considerable benefits AI and CV frameworks can offer to logistics and supply chain operations.
While automation is promising, its successful implementation requires businesses to overcome multiple challenges.
Below is a list of some challenges manufacturers must address to ensure their automation strategy works efficiently.
High Initial Costs
As Tesla’s example shows, automation requires hefty initial investments with uncertain returns. Businesses must purchase high-end cameras, sensors, and software solutions and incur costs associated with upgrading the infrastructure.
The high costs can restrict small-to-medium sized enterprises from adopting an automation approach. Higher management of large enterprises may also be reluctant to commit such long-term projects due to uncertainty regarding returns.
However, businesses can mitigate these challenges by beginning with a phased approach. The method involves experimenting with automation at a small scale, observing the returns, and identifying low-level problems.
Gradually, organizations can fine-tune and expand their experiments to implement a full-scale automation pipeline to achieve the desired targets.
Integration with Existing Systems
Integrating modern CV frameworks with legacy infrastructure can pose numerous problems due to incompatibility issues and a lack of staff expertise. Without an integrated pipeline, automation can result in extensive financial losses and damage a company’s relationship with investors.
Organizations can address integration issues by investing in modular CV systems and relevant APIs. These investments can result in seamless communication between software and hardware platforms. Additionally, businesses can minimize downtime by incrementally integrating the new solution with existing infrastructure instead of doing everything in one go.
Designing effective training strategies can also help staff upgrade their skills and familiarize themselves with the new technology.
Data Management and Privacy
Effective use of CV technology requires extensive on-site data collection and processing. However, the rising concerns around data privacy make it challenging for businesses to implement data-intensive solutions for efficient automation.
For instance, implementing CV systems for security and surveillance purposes can make workers more cautious and uneasy about their actions on the factory floor.
Building a robust data governance framework and creating a strong policy adherence culture are ways to maintain data privacy. The governance framework must address all aspects of the data lifecycle, from collection to disposal.
Transparent communication with employees about data collection practices and implementing privacy measures can help build trust and acceptance.
Lastly, organizations must develop strong encryption protocols to prevent data breaches and comply with data protection regulations like GDPR and CCPA.
Computer Vision in Logistics and Supply Chain: Key Takeaways
CV helps businesses boost profitability by automating logistics and supply chain workflows. However, the returns can be uncertain and require firm commitments from relevant stakeholders.
Below are a few critical points regarding CV implementation in the supply chain and logistics.
- Basic CV Applications in Supply Chain and Logistics: Automated inventory management, quality control and inspection, automated sorting and handling, traceability and tracking, and security and surveillance are core CV use cases in logistics and supply chain.
- Advanced CV Applications in Supply Chain and Logistics: CV systems offer advanced functionalities to automate logistics workflows, including predictive maintenance, route optimization, fleet management, occupancy and space utilization, and process modeling and simulation.
- Implementation Challenges: A few challenges in implementing CV systems include high initial costs, integration issues, and data privacy concerns. However, phased implementation, modular CV platforms, and robust data governance frameworks can mitigate these issues.
So, get a suitable computer vision data platform to begin your automation journey now!
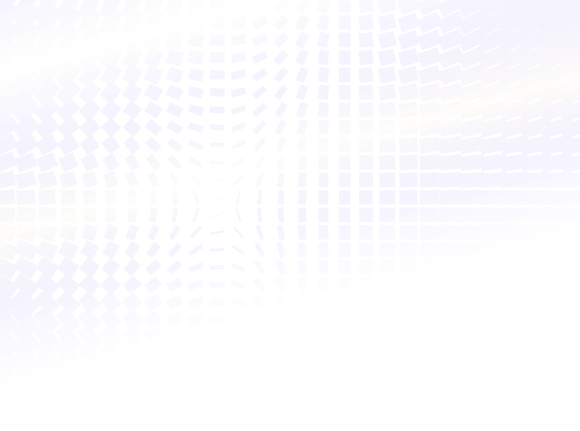
Power your AI models with the right data
Automate your data curation, annotation and label validation workflows.
Book a demoWritten by

Haziqa Sajid
- CV applications include: Automated inventory management, Quality control, Automated sorting and handling, Traceability and surveillance. Predictive maintenance, Route optimization, Fleet management, Space utilization and Process simulation.
- CV systems can track inventory levels in real-time by monitoring shelves in retail stores and storage fasciitis in the warehouse.
- CV systems use object detection and segmentation algorithms to detect product anomalies.
- Manufacturing, healthcare, and retail are three domains where CV is helping streamline operations.
- Autonomous vehicles can perform repetitive delivery tasks independently and reduce labor costs.
Explore our products