Contents
Computer Vision Applications in Manufacturing
Benefits of Using Computer Vision in Manufacturing
Critical Challenges of Computer Vision in Manufacturing
Computer Vision Applications in Manufacturing: Key Takeaways
Encord Blog
Top 8 Use Cases of Computer Vision in Manufacturing
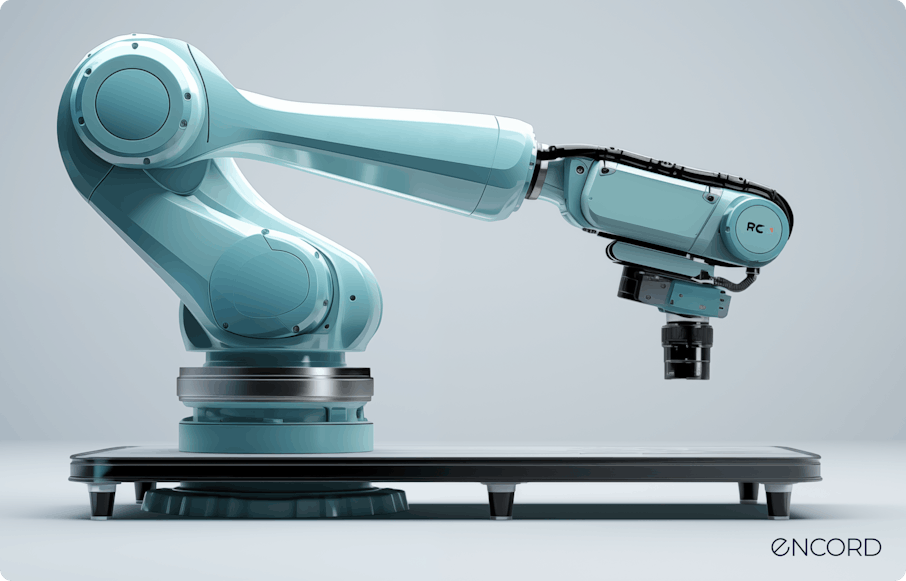
Ford, along with other manufacturing giants, has embraced the next wave of technological evolution—artificial intelligence. They accelerated assembly processes by 15% just by implementing AI and computer vision-enabled robots. Today, computer vision is a cornerstone in modern manufacturing, automating lengthy, repetitive, and potentially hazardous tasks, thereby allowing humans to focus on more complex and meaningful work.
This article explores the diverse applications of computer vision across various manufacturing industries, detailing their benefits and challenges.
Computer Vision Applications in Manufacturing
Computer vision has various applications in the manufacturing industry. The entire manufacturing lifecycle benefits from CV-powered automation and analysis, from product design to logistics and dismantling operations. Let’s discuss some of the use cases of AI in the manufacturing industry below
Product Design and Prototyping
3D design tools like Computer-Aided Design (CAD) are integrating with computer vision models like CLIP-Forge, JoinABLe, and Point2Cyl to make it easier for designers to generate object designs and prototype assemblies. For example:
Automobile design
Hyundai's New Horizons Studio has designed Elevate using CAD integrated with computer vision models for easier prototyping. Elevate is a car with legs for versatile terrain navigation. It's ideal for rescue teams to reach compromised areas with its enhanced lightweight durability. It can also assist people with mobility challenges.
Toys design and prototype
The award-winning toy, Magic Mixies by Moose Toys, was designed and prototyped using Fusion 360, incorporating generative design services. Leveraging Fusion 360, the team swiftly transformed the concept into a functional prototype within 3-4 months. Design and manufacturing for the initial Magic Mixies were accomplished and made available in 18 months.
Footwear and apparel design
Footwear company New Balance has used Gravity Sketch – a 3D design and prototype platform that uses Augmented Reality (AR) to help designers express their ideas in a 3D environment, improve the communication of their design workflow, and quickly reflect on what’s not working.
Industrial design
FARO RevEng Software offers a robust digital design experience, allowing users to create and edit meshes and CAD surfaces from a set of points in a 3D coordinate system. This aids industrial designers in reverse-engineering workflows (generating missing CAD files from legacy or prototype parts) and provides mesh models for additional design or 3D printing.
Product Manufacturing
Computer vision offers numerous applications in product manufacturing workflows. These include:
Automotive engineering
Ford is one of the leading manufacturers to use Virtual Reality (VR) for collaborative designing. Similarly, innovations in electrical distribution software are pushing boundaries in energy management, streamlining operations for a sustainable future. They use Microsoft HoloLens to enable designers and engineers to work together on car designs seamlessly. In another application, General Electric (GE) scientists are integrating computer vision into 3D printers, enabling machines to inspect large automotive parts as they are built, eliminating the need for time-consuming post-production inspections.
Agricultural efficiency
Singapore-based Singrow provides solutions involving AI-powered robots to guarantee premium-quality vegetables and fruits. The robot is programmed to recognize, identify, and select flowers and ripe strawberries, enhancing pollination efficiency. Singrow's indoor farms are 40% more energy efficient than a conventional strawberry farm and produce a 20% higher yield. Another product, See & Spray by Blue River, uses deep learning and machine vision to distinguish between crops and weeds and then spray chemicals only on the weeds. As a result, Blue River reduces herbicide use by up to 80 percent.
Textile production
Tianyuan Garments is disrupting traditional apparel manufacturing by introducing its latest workforce—sewbots. This technology uses tiny cameras to map soft fabric while robots pass material through sewing machines. Tianyuan Garments aims for a fully operational production process, creating one T-shirt every 22 seconds, with plans to manufacture 800,000 T-shirts daily while cutting operational costs by 33% per T-shirt. To manage such a high volume and technologically advanced operation efficiently, the use of manufacturing project management software is essential.
Steel industry
Cobots—robots that operate with humans to perform repetitive and complex tasks—enable steel manufacturers to perform welding operations. In 2022, Universal Robots (UR) reportedly grew its welding channel application by more than 80%. A steel manufacturer, Raymath, uses four UR robot welders, resulting in 4x productivity.
Moreover, ensuring comprehensive material tracking in production requires the verification of ID codes, serial numbers, or part numbers on steel bars and other products. SLR Engineering has implemented an OCR system capable of reliably identifying codes on the surfaces of steel bars used in tube production with an accuracy of over 99.90%.
Production Line
Production lines in manufacturing have evolved significantly from the pre-industrial period to Industry 4.0. CV-based automation, collaborative robots (cobots), fast installation, and easy programmability have improved the production outcome.
Let’s look at some of the key applications of computer vision in production lines:
Inspecting defects
Foxconn Technology Group, a global leader in smart manufacturing, has introduced FOXCONN NxVAE, which ensures efficiency and accuracy in inspecting defects using CV. It can detect the 13 most common defects in manufacturing production lines without errors. Another example is Volvo's Atlas computer vision system that scans vehicles with 20+ cameras, spotting defects 40% more than manual inspections, taking 5-20 seconds per cycle.
The Automated Production Line Applied for FOXCONN NxVAE
Compressor assembly
Opel, a German automobile manufacturer, uses a UR10 cobot to screw aircon compressors onto engine blocks. It was an ergonomically challenging task for the employees, now handled by a cobot in the production lines.
Automated production
Lion Electric manufactures batteries for electric vehicles. With soaring demand for Lion Electric's products, they have integrated FANUC robots and other automation equipment into their production facility. The team scaled up the system using computer vision to deliver a fully automated solution. This smart system lets Lion smoothly ramp up production while keeping operational costs in check.
Operational Safety and Security
In 2020, 4,764 US workers died on the job. Nearly half of these fatalities occurred in two potentially hazardous occupations:
- Transportation and material moving occupations (1,282 deaths)
- Construction and extraction occupations (976 deaths)
Computer vision enables manufacturers to improve worker safety and security by monitoring high-risk areas, identifying unsafe behaviors, and improving emergency response.
Here are some key areas where computer vision is improving operational safety in manufacturing:
Posture detection
TuMeke has created a CV-powered ergonomic risk assessment platform. Users record smartphone videos of various warehouse activities, such as lifting boxes. The platform then generates a risk summary identifying unsafe postures.
Fatigue detection
To detect signs of fatigue during long-haul truck driving and increase driver safety, Cipia provides CV-based driver monitoring, occupancy monitoring, and sensing solutions like:
- Driver Sense to detect signs of drowsiness and distracted driving
- Cabin Sense ensures passenger safety by detecting posture, seat belts, etc.
Containment leak detection
Dow Chemical reached the goal of zero safety-related incidents using computer vision and Internet of Things (IoT) solutions to detect possible containment leaks within its production environment. They used Azure Video Analyzer, an AI-applied service for detecting objects, people, and keyframes, to address personal protective equipment (PPE) detection and entrance gate monitoring.
Smart construction sites
Komatsu and NVIDIA introduced smart construction sites to improve the operational safety and productivity of job site workers. The drones collect images of the construction site. The CV model runs on the edge, powered by NVIDIA’s Jeston Nano GPU, and then analyzes these images to display site conditions in real-time.
Operational safety
The integration of virtual reality (VR) into Mustang Mach-E technician training by Ford and Bosch improves operational safety. The VR tool simulates high-voltage systems (components of electric vehicles that store and use high voltage), minimizing the necessity for hands-on training and reducing potential risks. Moreover, location-independent training allows technicians to operate in a more secure operational environment.
Quality Control
Quality control is an important process in manufacturing because high-quality products save costs and enhance a brand's reputation, reflecting well-designed processes. Studies suggest that stopped production costs are very high, around 22000$ per minute. Hence, robust inspection of final products ensures that they align with the manufacturer's set standards of quality and consistency. Here’s how computer vision aids quality control in manufacturing:
Pharmaceutical quality control
England-based Pharma Packaging Systems has introduced a CV-based solution for tablet counting and quality inspection. They use CV algorithms to process tablet images for the correct dimension and color and count the tablet occurrences within the frame. Defective tablets are automatically rejected in the production line.
Automobile fabric inspection
Manual inspection of automotive fabric is challenging due to inconsistent inspection levels and limited throughput speed. A UK-based automotive fabric producer addressed the challenge by introducing WebSpector, an automatic textile inspection system. This system integrates various lighting conditions, state-of-the-art cameras, and imaging software to detect and classify subtle defects.
Food volume inspection
3D vision systems can inspect the presence or absence of a part in food packaging. For instance, a 3D vision system like In-Sight 3D from Cognex provides a sense of depth to verify the volume of the package.
Packaging Inspection Using Cognex In-Sight-3D
Automotive quality control
FANUC employs a software program named ZDT (Zero Down Time) that utilizes cameras attached to robots. This system collects images and metadata, sending them to the cloud for processing. It effectively identifies potential issues before they occur. In an 18-month pilot across 38 automotive factories on six continents, applied to 7,000 robots, the solution detected and prevented 72 component failures.
Oil and gas pipeline performance
ExxonMobil collaborated with Microsoft to use the Azure cloud platform for data analytics and computer vision. The advancement of AI in oil and gas underscores the pivotal role of predictive maintenance, enhancing operational efficiency and reducing downtime tracking software in critical infrastructures. Different sensors are installed to capture data regarding infrastructure and equipment to ensure optimal performance and detect potential failures. A crucial tool for companies looking to increase production efficiency is downtime tracking software. But gathering information to demonstrate that the machine has stopped is only one stage in the procedure. Allocating the reason, compiling the facts, and implementing corrective measures to stop or lessen future incidents are the next steps.
Packaging
Companies spend 10-40% of the product's retail price on packaging. Other than visual appeal, packaging offers protection, safety, and usability. However, the likelihood of human error is significant in packaging.
The impact of computer vision in the packaging industry is a game changer, as it provides better accuracy, lowers costs, and frees up resources. Here are examples of companies using computer vision in packaging to build strong customer relationships:
Warehouse automation
Amazon Sparrow, the first robotic system in Amazon warehouses, utilizes computer vision and AI to handle individual products in the inventory. In 2021, global Amazon employees, assisted by Amazon technologies, processed around 5 billion packages. Cardinal, another example, is a robotic workcell using advanced AI and computer vision for efficient package selection, labeling, and placement in a GoCart for the next phase of its journey.
Pick and place robots
In collaboration, DHL Parcel and robot integrator AWL created a robotic application designed to pick and place parcels from a randomly arranged pallet onto a sorting installation's conveyor belt. This innovative solution incorporates AI-vision and gripping technology, allowing the robot to handle packages of diverse sizes and weights. With the capacity to lift up to 31.5 kg and process 800 parcels per hour, the robot automates tasks previously performed by humans.
Counting chicken trays
Tyson Foods faced challenges in accurately monitoring packed chicken tray counts, leading to inefficiencies in inventory management. Tyson Foods collaborated with AWS to implement computer vision (CV) technology for counting chicken trays. The solution offered real-time and accurate counting of chicken trays.
Tyson Collaborated With AWS to Build a Counting Solution Using CV
Logistics
In the early 2010s, Amazon pioneered computer vision in logistics, integrating Kiva Systems robots into its fulfillment centers for autonomous navigation and item retrieval. Later on, services like Amazon Rekognition improved quality assurance and operational efficiency in manufacturing, which were influenced by Kiva Systems.
Modern computer vision applications in logistics extend to 24/7 inventory management, automated parcel sorting and packaging, and quality inspection. Some of the real-life examples are given below:
Vision picking
DHL successfully implemented augmented reality (AR) in a warehouse pilot project, utilizing smart glasses for 'vision picking.' In collaboration with Ricoh and Ubimax, staff wore head-mounted displays showing task information, improving picking efficiency by 25%. The warehouse personnel could pick up 20000 items and fulfill 9000 orders.
Package management
WeWork faced significant challenges in package management in its mailrooms. They incorporated OCR and CV solutions from PackageX to read text, QR codes, and barcodes, which reduced the processing time to 85%.
Dismantling
Disassembly involves safely separating a product's components through nondestructive methods, while irreversible separation is termed dismantling or dismounting. Manufacturers must safely take down structures and focus on environmental care, cost reduction, and material treatment. Computer vision is helping manufacturers overcome dismantling challenges.
Here are some examples:
Robotic sorting
AMP Robotics launched an AI-guided system employing two high-performance robots to efficiently sort materials at an impressive speed of 160 pieces per minute. The AMP Neuron platform uses computer vision and machine learning to identify material characteristics such as colors, textures, shapes, sizes, and patterns in precise pick-and-place operations.
Mobile component recycling
Apple's disassembly robot, Daisy, efficiently extracts valuable materials from nine iPhone versions, sorting high-quality components for recycling. Daisy can process up to 200 iPhones
per hour, extracting and sorting components that traditional recycling methods may not recover and doing so with higher quality.
Wood recycling
Europe's third-largest panelboard producer utilizes TOMRA recycling sorting's advanced X-ray transmission and deep learning wood sorting solutions. CV models help them better understand unique object characteristics and how to classify the objects transported on the conveyor belt and scanned by the sensors. This ensures the high-performance and reliable separation of non-processed wood, contributing to the production of wood products with up to 50% recycled content.
Construction waste recycling
Globally, building and infrastructure projects generate a significant volume of bulky Construction and Demolition (C&D) waste, constituting over a third of Europe's total waste. The ZenRobotics Heavy Picker, recognized as the world's strongest recycling robot, efficiently sorts heavy C&D waste, handling materials such as wood, plastics, metals, and inert items weighing up to 30 kilos. This unmanned robot can operate 24/7 for continuous sorting.
Benefits of Using Computer Vision in Manufacturing
According to a Forrester survey, 64% of global business purchase influencers find computer vision crucial, with 58% expressing interest in implementing CV technology at their firm. Some key benefits of implementing CV solutions in manufacturing include:
- Increased productivity: According to Deloitte, adopting computer vision and automation speeds up manufacturing cycles. They increase labor productivity and production output to about 12% and 10%, respectively.
- Enables safer operations for the workforce: Computer vision makes manufacturing environments safe as they monitor workers' conditions, identify abnormalities, and recognize signs of fatigue.
- Eliminating errors: Tasks handled by computer vision eliminate human error because of their precise nature. Reducing errors close to zero ultimately improves the quality of the product.
- Reduced operating costs: Improved efficiency and reduced machine downtime, achieved through automation and computer vision-based maintenance (potentially up to 50%, according to McKinsey), lead to overall operating cost reduction.
Critical Challenges of Computer Vision in Manufacturing
The manufacturing industry has witnessed improved productivity due to computer vision. However, there are many challenges to employing computer vision in real-world use cases. Key challenges include:
- Inadequate hardware: Most computer vision solutions combine high-end hardware requirements and optimized software. Generally, CV solutions require high-resolution cameras, sensors, and bots. These gadgets and infrastructure components are costly and require special care.
- Lack of high-quality data: Computer vision works best with high-quality training data. The absence of quality data leads to project failure. For instance, lack of quality data is one of the reasons many AI tools failed to perform as expected during COVID-19.
- Issues with technological integration: Integration of computer vision into existing manufacturing setup faces compatibility issues due to diverse machinery, the need for continuous monitoring, and the requirement of skillful personnel.
- High costs: Computer vision in manufacturing faces significant cost challenges. Cloud-based processing has dynamic costs and latency issues, while edge deployment requires careful hardware consideration. For instance, CCD cameras can range from $30 to $3,500, and different cloud providers offer different pricing tiers amounting to thousands. Algorithm complexity is another issue—lightweight models offer cost-effective scaling compared to large models. To save costs, manufacturers should also avoid using proprietary data, which can backfire, leading to deteriorating results.
Computer Vision Applications in Manufacturing: Key Takeaways
- Computer vision enables manufacturers to interpret visual data like humans and automate repetitive tasks.
- Key computer vision techniques include object detection and recognition, image classification, and segmentation.
- Computer vision applications in manufacturing cover many areas, including designing, production, quality assurance, packaging, logistics, and dismantling.
- CV facilitates many industries with visual inspection, predictive maintenance, intelligent processes, error detection, automated assembly lines, and robotic collaboration.
- Computer vision benefits manufacturing processes with high productivity, more safety, and less human error.
- Challenges for CV in manufacturing include expensive hardware, a lack of skilled professionals, and high-quality data.
Power your AI models with the right data
Automate your data curation, annotation and label validation workflows.
Get startedWritten by

Haziqa Sajid
- Computer vision is currently applied in agriculture, healthcare, and logistics, with its utilization extending to various other industries.
- The role of computer vision in manufacturing is to enhance efficiency, automate tasks, and improve safety.
- Ford’s AI- and computer vision-driven assembly lines are excellent examples of improving its speed by 15%.
- The computer vision market reached $15 billion in 2022 and is expected to reach an estimated $82.1 billion by 2032.
- The main advantages include increased productivity, enhanced safety, and reduced errors.
- Computer vision contributes to quality control by identifying defects, inspecting quality, and minimizing human oversight.
- Some of the anticipated trends in manufacturing include growth in predictive maintenance, augmented reality applications, and human-machine collaboration.
- Computer vision is fueling the manufacturing industry to its fourth industrial revolution. Examples like bin picking, machine tending, predictive maintenance, and detecting defects are some of its applications.
- The reliability of computer vision systems has seen an incredible spike over the past few years. With companies like Ford, DHL, and Amazon, continuous usage of CV serves as compelling evidence of its effectiveness.
- Vision systems in manufacturing are automated technologies that use cameras and image processing to inspect, measure, and guide the production process.
- Machine vision allows machines to perceive their environment using cameras and other optic sensors, while computer vision trains computers to interpret and understand the visual world.
- Computer vision is a subset of artificial intelligence (AI) to allow computers to see. Machine learning is used within computer vision to improve recognition and analysis.
- The four basic types of machine vision systems include 1D vision systems, 2D vision systems, line or area scans, and 3D vision systems.
- No, deep learning is not mandatory for computer vision, but it is commonly used as it excels in tasks like image recognition and object detection.
- The three R's of computer vision are Recognition, Reconstruction, and Reorganization.
Explore our products