Contents
A Brief Look Back: The Rise of MiniGPT
Core Concepts of MiniGPT-v2
Demo of MiniGPT-v2
Applications of MiniGPT-v2
Comparison with Predecessor, MiniGPT-4
Comparison with SOTA
Conclusion
References
Encord Blog
MiniGPT-v2 Explained
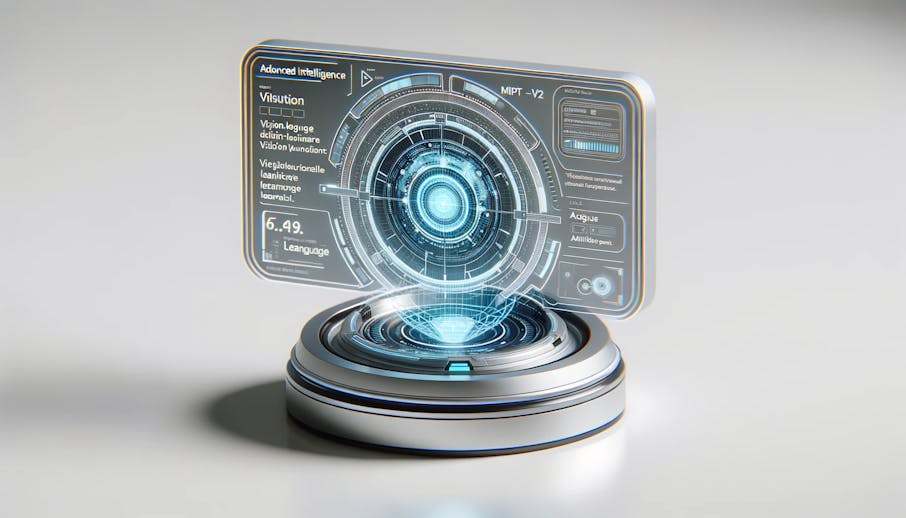
Meta has made an impressive foray into multimodal models through the launch of MiniGPT-v2. This model is capable of efficiently handling various vision-language tasks using straightforward multi-modal instructions. The performance of MiniGPT-v2 is remarkable, demonstrating its prowess across numerous vision-language tasks. The results rival both OpenAI's multimodal GPT-4 and Microsoft’s LLaVA, thereby establishing a new standard in terms of state-of-the-art accuracy, especially when compared to other generalist models in the vision-language domain.
The fusion of natural language processing and computer vision has given rise to a new breed of machine learning models with remarkable capabilities. MiniGPT-v2 is one such model that seeks to serve as a unified interface for a diverse set of vision-language tasks.
In this blog, we'll explore the world of MiniGPT-v2, understanding its architecture, core concepts, applications, and how it compares to its predecessor.
But first, let's take a step back and appreciate the journey of multimodal models like MiniGPT.
A Brief Look Back: The Rise of MiniGPT
MiniGPT-v2 builds upon the success of its predecessor. Earlier versions of GPT (Generative Pre-Trained Transformer) and large language models (LLMs) like BERT laid the foundation for natural language understanding. These models achieved groundbreaking results in various language-related applications. With MiniGPT-v2, the focus shifts to integrating visual information into the mix.
The vision-language multi-task learning landscape poses unique challenges. Imagine a scenario where you ask a model, "Identify the number of pedestrians in the image of a street." Depending on the context, the answer could involve describing the person's spatial location, identifying the bounding box around them, or providing a detailed image caption. The complexities inherented in these tasks require a versatile approach.
In this context, large language models have shown their mettle in various language-related applications, including logical reasoning and common-sense understanding. Their success in natural language processing (NLP) has motivated AI researchers to extend their capabilities to the vision-language tasks, giving rise to models like MiniGPT-v2.
Core Concepts of MiniGPT-v2
MiniGPT-v2: large language model as a unified interface for vision-language multi-task learning
At the heart of MiniGPT-v2 lies a well-thought-out architecture. This model comprises three main components:
Visual Backbone
At the foundation of MiniGPT-v2 is its visual backbone, inspired by the Vision Transformer (ViT). The visual backbone serves as the model's vision encoder. It processes the visual information contained within images. This component is responsible for understanding and encoding the visual context of the input, enabling the model to "see" the content of images.
One distinctive aspect is that the visual backbone is frozen during training. This means that the model's vision encoder doesn't get updated as the model learns from the dataset. It remains constant, allowing the model to focus on refining its language understanding capabilities.
Linear Projection Layer
The linear projection layer in MiniGPT-v2 plays a crucial role in enabling the model to efficiently process high-quality images. As image resolution increases, the number of visual tokens also grows significantly. Handling a large number of tokens can be computationally expensive and resource-intensive. To address this, MiniGPT-v2 employs the linear projection layer as a practical solution.
The key idea here is to concatenate multiple adjacent visual tokens in the embedding space. By grouping these tokens together, they can be projected as a single entity into the same feature space as the large language model. This operation effectively reduces the number of visual input tokens by a significant factor. As a result, MiniGPT-v2 can process high-quality images more efficiently during the training and inference stages.
Large Language Model
The main language model in MiniGPT-v2 comes from LLaMA-2 and works as a single interface for different vision language inputs. This pre-trained model acts as the bridge between visual and textual information, enabling MiniGPT-v2 to perform a wide range of vision-language tasks.
The advanced large language model is not specialized for a single task but is designed to handle diverse instructions, questions, and prompts from users. This versatility is achieved using task-specific tokens, a key innovation in MiniGPT-v2. These tokens provide task context to the model, allowing it to understand the image-text pair and the nature of the task at hand.
This adaptability extends to tasks that require spatial understanding, such as visual grounding. For instance, when the model needs to provide the spatial location of an object, it can generate textual representations of bounding boxes to denote the object's spatial position within an image.
The use of task-specific tokens greatly enhances MiniGPT-v2's multi-task understanding during training. By providing a clear context for different tasks, it reduces ambiguity and makes each task easily distinguishable, improving learning efficiency.
Demo of MiniGPT-v2
It's one thing to talk about it, but it's another to see MiniGPT-v2 in action. Here's a sneak peek at how this model handles various vision-language tasks.
Grounding
The MiniGPT-v2 works well on image descriptions. When prompted to “Describe the above image”, the model not only describes the image but performs object detection as well.
Visual Question and Answering (VQA)
When prompted to find a place in the room to hide in the game of hide and seek, MiniGPT-v2 can understand the prompt, assess the image well, and provide a suitable answer.
Detection
In the case of object detection, MiniGPT-v2 can identify large objects. But in case of small objects, it resorts to describing the environment or the image.
Applications of MiniGPT-v2
MiniGPT-v2's versatility shines through in its applications. It's not just about understanding the theory; it's about what it can do in the real world. Here are some of the key applications:
- Image Description: MiniGPT-v2 can generate detailed image descriptions.
- Visual Question Answering: It excels at answering complex visual questions.
- Visual Grounding: The model can pinpoint the locations of objects in images.
- Referring Expression Comprehension: It accurately understands and responds to referring expressions.
- Referring Expression Generation: It can generate referring expressions for objects in images.
- Object Parsing and Grounding: MiniGPT-v2 can extract objects from text and determine their bounding box locations.
Comparison with Predecessor, MiniGPT-4
To gauge MiniGPT-v2's progress, it's important to compare it with its predecessor, MiniGPT-4. The key distinction between the two lies in their performance and capabilities within the domain of vision-language multi-task learning.
MiniGPT-v2, designed as an evolution of MiniGPT-4, surpassed its predecessor in several important aspects:
- Performance: Across a spectrum of visual question-answering (VQA) benchmarks, MiniGPT-v2 consistently outperformed MiniGPT-4. For instance, on QKVQA, MiniGPT-v2 exhibited a remarkable 20.3% increase in top-1 accuracy compared to its predecessor.
- Referring Expression Comprehension: MiniGPT-v2 demonstrated superior performance on referring expression comprehension (REC) benchmarks, including RefCOCO, RefCOCO+, and RefCOCOg.
- Adaptability: MiniGPT-v2, particularly the "chat" variant trained in the third stage, showed higher performance compared to MiniGPT. The third-stage training's focus on improving language skills translated into a substantial 20.7% boost in top-1 accuracy on challenging benchmarks like VizWiz.
Comparison with SOTA
The authors extensively evaluated the performance of the model, setting it against the backdrop of established state-of-the-art (SOTA) vision-language models. They conducted a rigorous series of experiments across diverse tasks, encompassing detailed image/grounded captioning, vision question answering, and visual grounding.
MiniGPT-v2: large language model as a unified interface for vision-language multi-task learning
MiniGPT-v2 showcased consistent performance that firmly established its position at the forefront. In comparison with previous vision-language generalist models such as MiniGPT-4, InstructBLIP, BLIP-2, LLaVA, and Shikra, MiniGPT-v2 undeniably emerges as a stellar performer, setting new standards for excellence in this domain.
Vision Spatial Reasoning (VSR) serves as an exemplary case where MiniGPT-v2 not only outperforms MiniGPT-4 but does so with a substantial 21.3% lead. In the VSR benchmark, MiniGPT-v2 surpasses InstructBLIP by 11.3% and leaves LLaVA trailing by 11.7%. These remarkable achievements underscore MiniGPT-v2's prowess in complex vision-questioning tasks.
Conclusion
The emergence of vision language models like MiniGPT-v2 represents a significant step forward in computer vision and natural language processing. It's a testament to the power of large language models and their adaptability to diverse tasks. As we continue to explore the capabilities of MiniGPT-v2, the possibilities for vision-language tasks are expanding.
References
- Official website: https://minigpt-v2.github.io/
- GitHub Code: https://github.com/Vision-CAIR/MiniGPT-4
- HuggingFace Space: https://huggingface.co/spaces/Vision-CAIR/MiniGPT-v2
- Dataset: https://huggingface.co/datasets/Vision-CAIR/cc_sbu_align
Power your AI models with the right data
Automate your data curation, annotation and label validation workflows.
Get startedWritten by

Akruti Acharya
Explore our products