Contents
Criteria for selection of Text Annotation Tools
Overview of Top Text Annotation Tools
#1. SuperAnnotate
#2. Doccano
3. LightTag
#4. Prodigy
#5. Appen
#6. UBIAI
#7. Label Studio
#8. brat (Browser-Based Rapid Annotation Tool)
Future Trends
Key Takeaways: Top Text Annotation Tools in 2024
Encord Blog
Top Text Annotation Tools in 2024: Features, Collaboration, and Industry Applications
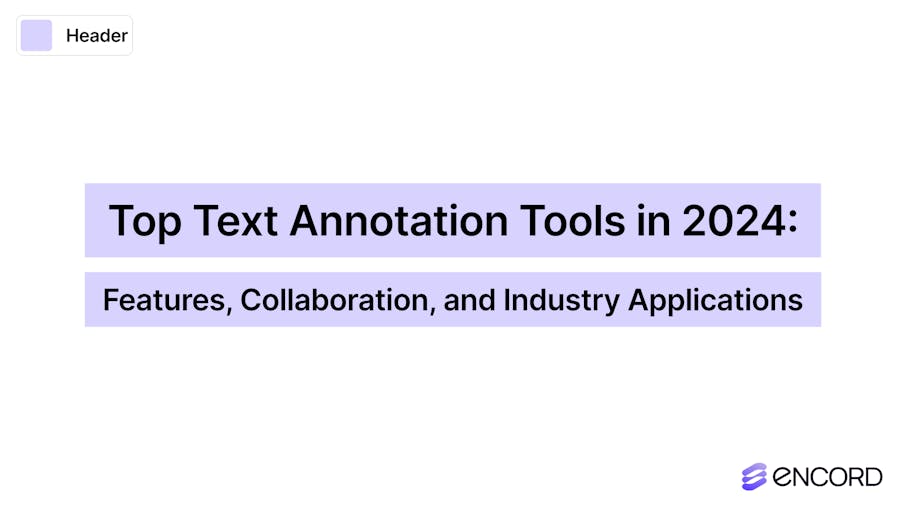
Text annotation is the process of categorizing and labeling textual data to make it understandable and usable by artificial intelligence (AI) systems. Text annotation tools are critical to this process, particularly in natural language processing (NLP).
These tools transform the raw text into structured, labeled datasets that form the foundation for training sophisticated AI models capable of understanding and processing human language.
Text annotation tools enable robust NLP applications in healthcare, finance, and customer service by facilitating named entity recognition, sentiment analysis, and machine translation.
These tools also offer a structured environment for annotation and features like inter-annotator agreement tracking that help keep data quality and consistency high. It is important to avoid training models incorrectly and getting AI results that cannot be trusted.
This article will review some of the most popular text annotation tools, evaluating their features, ease of use, and suitability for different NLP tasks and industries. Let's dive in!
Criteria for selection of Text Annotation Tools
When selecting the top text annotation tools for 2024, keep an eye out for these essential features:
- User Interface: A well-designed and intuitive UI is crucial for annotator efficiency and ease of use. Look for features like customizable workspaces, keyboard shortcuts, and clear visual feedback.
- Collaboration Features: For teams, real-time collaboration, role-based access control, and conflict resolution mechanisms are essential. These features streamline teamwork and ensure consistency across annotations.
- Annotation Formats: The tool should support various annotation formats, such as JSON, XML, or CSV. This versatility ensures compatibility with various AI model architectures and data processing pipelines, allowing seamless integration into existing workflows.
- Scalability: As projects grow, the annotation tool should be able to handle increasing volumes of data without compromising performance. Look for features like distributed annotation capabilities, cloud-based solutions, or on-premise deployment options to ensure the tool can scale with your needs.
- Customization: It is crucial to customize annotation schemas, labeling conventions, and workflows. This flexibility allows the tool to adapt to specific project requirements, unique data characteristics, and domain-specific needs, ensuring the annotated data aligns with the project's goals.
- Model Integration: Advanced annotation tools often offer integration with pre-trained models or active learning capabilities. This feature can significantly speed up the annotation process by providing initial annotations or suggestions, which human annotators can verify or correct. It is particularly useful when annotated data is limited or when dealing with ambiguous or difficult-to-annotate examples.
- Industry Adaptability: The tool should support industry-specific data formats and comply with relevant regulations. For example, healthcare applications may require support for HIPAA-compliant data handling, while financial services may need adherence to FINRA guidelines.
- Integration Capabilities: Robust APIs are important for integrating the tool into your existing data ecosystems, machine learning pipelines, and other software stacks.
These features determine a tool's effectiveness and suitability for professional use across various industries.
Overview of Top Text Annotation Tools
In this article, we have handpicked leading text annotation tools, each providing great value in the user interface, collaboration, industry adaptability, and system integration to streamline data labeling for AI and ML advancements. Here are the eight (8) tools we discuss in this article:
- SuperAnnotate
- Doccano
- LightTag
- Prodigy
- Appen
- UBIAI
- Label Studio
- Brat
#1. SuperAnnotate
SuperAnnotate is a versatile data annotation tool designed to create high-quality training data for machine learning models. It supports various data types, including text, images, videos, and audio, and offers advanced features to streamline the annotation process and ensure accuracy.
Figure: SuperAnnotate Tool (Source)
Key Features
- User Interface: SuperAnnotate provides a user-friendly interface that simplifies annotation. The platform is designed to be accessible to both technical and non-technical users.
- Collaboration: The platform supports robust collaboration features, allowing multiple users to work on the same project simultaneously. This includes real-time updates, version control, and role-based access, which enhance team productivity and project management.
- Industry Adaptability:
SuperAnnotate is adaptable to various industries, including healthcare, autonomous driving, retail, and more. Its flexible annotation tools can be customized to meet the specific needs of different sectors. - Integration Capabilities: The platform integrates with popular ML frameworks and tools (TensorFlow, PyTorch, etc.). This includes APIs and SDKs that facilitate data import and export, making incorporating SuperAnnotate into existing workflows easier.
- Other Criteria: SuperAnnotate also features advanced annotation tools such as polygon, polyline, and keypoint annotations, as well as AI-assisted labeling to speed up the annotation process.
Pros
- High Efficiency: The AI-assisted labeling and automation features significantly reduce the time required for manual annotations, increasing overall efficiency.
- Scalability: SuperAnnotate can easily handle large-scale projects, making it suitable for enterprises with extensive data annotation needs.
Cons
- Learning Curve: Despite its user-friendly interface, new users may experience a learning curve when first acquainted with the platform's advanced features.
- Cost: SuperAnnotate offers a range of pricing plans to cater to different user needs. The Starter plan follows request-based pricing. There is also a free plan with limited features and custom pricing for enterprise plans. For detailed pricing information, it is recommended to visit the SuperAnnotate website.
#2. Doccano
Doccano is an open-source, web-based text annotation tool designed for creating labeled datasets for machine learning. It supports text classification, sequence labeling, and sequence-to-sequence tasks, making it ideal for sentiment analysis, named entity recognition, and text summarization.
Figure: Doccano Tool (Source)
Key Features
- User Interface: Doccano features a simple web-based interface, which makes it accessible even for beginners. The UI is designed to streamline the annotation process, allowing users to easily upload datasets and start annotating without extensive setup or configuration.
- Collaboration: Doccano supports team collaboration, enabling multiple users to work concurrently on the same annotation project. Features include sharing annotations across users.
- Industry Adaptability: Doccano is highly adaptable and can be used across various industries for tasks such as sentiment analysis, named entity recognition, and text summarization. Its flexibility makes it suitable for different sectors requiring text annotation.
- Integration Capabilities: The platform's REST APIs allow integration with other tools and machine learning frameworks. This allows users to automate parts of the annotation process and integrate Doccano into their existing workflows.
- Other Criteria: Doccano supports multiple export formats, including CSV and JSON, which facilitates the integration of annotated data into various machine-learning pipelines. It also provides features for defining annotation guidelines and tracking labeling statistics.
Pros
- Open Source and Customizable: Doccano is open-source, allowing users to modify and customize the tool according to their needs. This flexibility is particularly beneficial for organizations with unique annotation requirements.
Cons
- Performance Issues: In self-hosted environments, Doccano may experience performance issues such as lagginess and shuffling the order of annotations. These issues can affect the efficiency of the annotation process.
3. LightTag
LightTag is a data annotation platform designed to help teams label text data efficiently. Its user-friendly interface and robust collaboration features make it suitable for various industries.
The platform is particularly useful for machine learning projects that require high-quality labeled data.
Figure: LightTag Tool (Source)
Key Features
- User Interface: LightTag provides an intuitive and easy-to-navigate interface, allowing users to get started with text annotation tasks quickly.
- Collaboration: The platform supports team collaboration, allowing multiple users to work on the same project simultaneously for annotation consistency.
- Quality Control: LightTag includes features for quality control, such as inter-annotator agreement metrics, to ensure the accuracy and reliability of the annotated data.
- Industry Adaptability: LightTag is adaptable to various industries, including healthcare, finance, and e-commerce, thanks to its compliance with stringent data protection regulations like HIPAA. This compliance ensures that LightTag can handle sensitive data securely, making it suitable for industries with strict privacy and security requirements.
- Integration Capabilities: LightTag integrates smoothly with other tools and platforms through its API, which supports the automation of workflows and machine-in-the-loop annotations. This integration capability allows easy data import and export processes, enhancing workflow efficiency.
Pros
- Efficient Collaboration: LightTag's collaboration features enhance team productivity and ensure consistent annotations across projects.
Cons
- Limited Free Tier: The free tier of LightTag may have limitations in features and the number of annotations, which could be a constraint for larger projects.
- Learning Curve: While the interface is user-friendly, there may still be a learning curve for users unfamiliar with data annotation tools.
#4. Prodigy
Prodigy is a scriptable annotation tool designed to create training data for machine learning models. Developed by Explosion, the spaCy maker Prodigy integrates active learning to make the annotation process more efficient by involving the model in the loop.
This allows the model to learn from the annotations in real time and prioritize the most informative examples for annotation.
Figure: Prodigy Tool (Source)
Key Features
- User Interface: Prodigy offers a web-based interface that supports various annotation tasks, including text classification, named entity recognition, and image annotation. The user-friendly and efficient interface allows annotators to focus on one decision at a time.
- Collaboration: Prodigy supports collaboration through its web application, allowing multiple annotators to work on the same project. However, it is primarily designed for small teams and individual data scientists who can manage the annotation process.
- Industry Adaptability: Prodigy is highly adaptable and can be used across various industries, including healthcare, finance, and e-commerce, for tasks such as sentiment analysis, entity recognition, and image classification. Its flexibility makes it suitable for different sectors requiring annotated data.
- Integration Capabilities: The platform integrates seamlessly with Python-based data science workflows and popular machine learning frameworks like spaCy, TensorFlow, and PyTorch. It also supports custom recipes, allowing users to tailor the tool to their needs.
- Other Criteria: Prodigy includes built-in recipes for various annotation tasks and supports active learning, which helps in collecting high-quality training data efficiently. It also offers extensive documentation and community support to help users get the most out of the tool.
Pros
- Efficiency: Prodigy's active learning approach significantly reduces the time and effort required for manual annotation by prioritizing the most informative examples.
- Scriptability: The tool is fully scriptable, allowing users to seamlessly create custom workflows and integrate Prodigy into their existing data science pipelines.
Cons
- Cost: Prodigy is a paid tool, which may not be suitable for all budgets, especially for small businesses or individual users.
- Limited Large-Scale Collaboration: While Prodigy supports collaboration, it is primarily designed for small teams and may not offer the advanced collaborative features required for large-scale projects.
#5. Appen
Appen is a leading provider of high-quality training data for machine learning and artificial intelligence. The company uses a global crowd of over one million skilled contractors to annotate and curate data, which is then used by some of the world's largest technology companies to improve their AI systems.
Figure: Appen Tool (Source)
Key Features
- User Interface: Appen uses Appen Connect to manage project-related information, including recruiting, onboarding, and task management. The interface is designed to be user-friendly, allowing contractors to navigate and complete tasks easily.
- Collaboration: Appen supports collaboration through its global crowd workforce, enabling multiple contractors to work on various projects simultaneously. This distributed model allows efficient data collection and annotation across different time zones and languages.
- Industry Adaptability: Appen's services are adaptable to various industries, including technology, automotive, financial services, retail, healthcare, and government. The company provides data for various AI applications, such as speech recognition, computer vision, and natural language processing.
- Integration Capabilities: The platform integrates seamlessly with clients' existing AI and machine learning workflows. The platform supports various data formats and can be customized to meet specific project requirements, ensuring smooth integration with different systems.
- Exhaustive Services: Appen offers a comprehensive suite of data annotation tools and services, including text, image, video, and audio annotation. The platform also provides quality assurance and project management support to ensure high-quality data delivery.
Pros
- Global Reach: Appen's extensive network of over one million contractors in more than 170 countries allows for diverse and scalable data collection and annotation.
- Expertise: With over 25 years of experience in the industry, Appen brings unparalleled expertise to every project, ensuring high-quality and reliable data for AI training.
Cons
- Cost: The services provided by Appen can be expensive and may not be suitable for small businesses or individual users with limited budgets.
- Worker Conditions: There have been concerns about the working conditions and compensation of Appen's contractors, which may affect the overall perception of the company.
#6. UBIAI
UBIAI is a powerful text annotation tool designed to help businesses label and fine-tune training data for Natural Language Processing (NLP) models.
It supports many document formats, including PDFs, images, and handwritten texts, and offers advanced features like Optical Character Recognition (OCR) and multilingual support.
Figure: UBIAI Tool (Source)
Key Features
- User Interface: UBIAI provides an intuitive and user-friendly interface, making it accessible for users with different technical expertise.
- Collaboration: The platform supports team collaboration, allowing multiple annotators to work on the same project simultaneously, ensuring consistency and efficiency.
- Industry Adaptability: UBIAI is versatile and can be used across various industries, including healthcare, finance, and legal sectors, to meet specific annotation needs.
- Integration Capabilities: It integrates seamlessly with other tools and platforms, facilitating smooth data import and export processes.
- OCR Technology: UBIAI's advanced OCR technology allows for accurate text extraction from PDFs and images, ensuring no detail is missed.
Pros
- Efficient Data Extraction: UBIAI's OCR technology and multilingual support make it highly efficient in extracting data from diverse document types.
Cons
- Limited Free Tier: The free tier of UBIAI may have limitations in terms of features and the number of annotations, which could be a constraint for larger projects.
- Learning Curve: While the interface is user-friendly, there may still be a learning curve for users unfamiliar with data annotation tools.
#7. Label Studio
Label Studio is an open-source data labelling tool that supports multiple projects, users, and data types on a single platform. It allows users to perform various labelling tasks, integrate with ML models for pre-labelling, and utilize active learning to enhance the efficiency of the annotation process.
Label Studio is also an Enterprise cloud service with enhanced security, team management features, data discovery, analytics, and support SLAs.
Figure: Label Studio Tool (Source)
Key Features
- User Interface: Label Studio offers a user-friendly web-based interface that supports various annotation tasks, including text, image, audio, and video labelling. The interface is designed to be intuitive, allowing users to easily set up projects, import data, and start annotating.
- Collaboration: The platform supports collaboration among multiple annotators, enabling team members to work on the same project simultaneously. Tasks are locked while annotated to prevent overwriting, and the platform supports role-based access control (RBAC) for managing user permissions.
- Industry Adaptability: Label Studio is highly adaptable and can be used across various industries, including healthcare, finance, and e-commerce, for tasks such as sentiment analysis, named entity recognition, and image classification. Its flexibility makes it suitable for different sectors requiring annotated data.
- Integration Capabilities: The platform integrates seamlessly with machine learning frameworks like TensorFlow, PyTorch, and spaCy. It also supports REST APIs, webhooks, and a Python SDK, allowing users to integrate Label Studio into their existing workflows and automate parts of the annotation process.
- Other Criteria: Label Studio provides advanced features such as ML-assisted labelling, which uses model predictions to assist annotators, and a comprehensive data management system that includes cloud storage integration, data versioning, and export options in various formats.
Pros
- Open Source and Customizable: Label Studio is open-source, allowing users to modify and customize the tool according to their needs. This flexibility is particularly beneficial for organizations with unique annotation requirements.
Cons
- Learning Curve: Despite its user-friendly interface, new users may experience a learning curve when first getting acquainted with the platform's advanced features and customization options.
- Performance Issues: Label Studio may experience performance issues such as lagginess in self-hosted environments, especially when handling large datasets or complex annotation tasks.
#8. brat (Browser-Based Rapid Annotation Tool)
brat is a web-based tool designed for rapid text annotation, particularly for Natural Language Processing (NLP) tasks. It integrates NLP technology and provides an intuitive interface for structured annotation to enhance annotator productivity.
Figure: brat Tool (Source)
Key Features
- User Interface: brat offers an intuitive and user-friendly interface that allows annotators to mark and link text spans easily using familiar mouse-based gestures.
- Collaboration: The tool supports collaborative annotation efforts, enabling multiple users to work on the same project simultaneously.
- Industry Adaptability: brat is versatile and can be applied to a wide range of text annotation tasks, including entity mention annotation, chunking, binary relation annotation, and more.
- Integration Capabilities: It integrates with external resources like Wikipedia and supports automatic text annotation tools, enhancing its functionality.
- Annotation Visualization: brat provides high-quality visualization of annotations, making it easier to understand complex annotations and relationships between text spans.
Pros
- Rich Feature Set: brat supports various annotation types, including typed text spans, binary relations, and n-ary associations, making it suitable for diverse annotation tasks.
- Open Source: The tool is freely available under the MIT license, allowing users to customize and extend its functionalities.
Cons
- Complex Setup: Setting up brat can be complex, requiring some technical knowledge to configure and maintain the server and data.
- Limited Input Formats: brat primarily accepts text files as input documents, which may not be suitable for annotating structured documents like PDFs.
Future Trends
The landscape of text annotation tools is rapidly evolving due to AI and machine learning advancements. Key trends shaping the future include:
- Recognition and Filtering of AI-Generated Content: Tools will be needed to identify and filter AI-generated content to ensure authenticity, requiring large-scale corpora and specialized recognition models.
- Increased Specialization and Refinement: Annotation tasks will demand domain-specific knowledge, necessitating more fine-grained processes in healthcare, legal, and finance.
- Integration of Advanced AI Techniques: Techniques such as few-shot and active learning will reduce costs, improve efficiency, and allow models to adapt quickly to new data.
- Automated and Semi-Automated Annotation: Enhanced use of machine learning for pre-annotation will accelerate processes and reduce manual intervention while maintaining high accuracy.
- Privacy Protection and Data Security: Robust privacy measures and compliance with regulations will be essential for handling sensitive data securely.
- Real-Time and Multimodal Data Annotation: There will be a demand for tools capable of efficiently handling and annotating diverse data types like text, images, and videos in real-time.
Key Takeaways: Top Text Annotation Tools in 2024
Choosing the right text annotation tool is essential for effective data management and AI training. The right tool enhances annotation accuracy and efficiency, leading to better-performing AI models.
Key considerations include scalability and integration, ensuring the tool grows with your project and fits seamlessly with existing systems. User-friendliness and customization are crucial for engagement, while robust security features and compliance with privacy regulations protect sensitive data.
Additionally, considering the total cost of ownership and leveraging AI to automate tasks can reduce costs and improve efficiency. High-quality annotations are vital, so tools with built-in quality assurance mechanisms are preferred.
In summary, the evolution of text annotation tools will focus on specialization, efficiency, and security, enabling organizations to enhance data management and achieve superior AI project outcomes.
Power your AI models with the right data
Automate your data curation, annotation and label validation workflows.
Get startedWritten by
Nikolaj Buhl
- Text annotation involves labelling or tagging data, such as text, images, or audio, to create training datasets for machine learning models. It helps algorithms understand and process the text data.
- Manual annotation involves human annotators adding labels to data, ensuring high precision by capturing subtle nuances. Automatic annotation uses AI to label data quickly and at scale, though it may lack the accuracy of human annotation.
- Important features include user-friendly interfaces, collaboration capabilities, integration with machine learning pipelines, scalability, and robust security measures. Tools should also support various data types and offer advanced AI techniques like active learning to improve efficiency.
- Quality can be maintained through clear annotation guidelines, multiple annotators to verify data, iterative feedback processes, and built-in quality control mechanisms. Measuring inter-annotator agreement and regular validation of annotations also help ensure data reliability.
- Data privacy is crucial to protect sensitive information during the annotation process. Tools must comply with regulations like GDPR and HIPAA and include features like encryption, access controls, and secure data handling practices to maintain user trust and meet legal requirements.
Explore our products