Contents
Why Video Annotation is Crucial for Machine Learning
Challenges With Manual Video Annotation
Steps for Automating Video Annotation
Encord Blog
How to Automate Video Annotation for Machine Learning
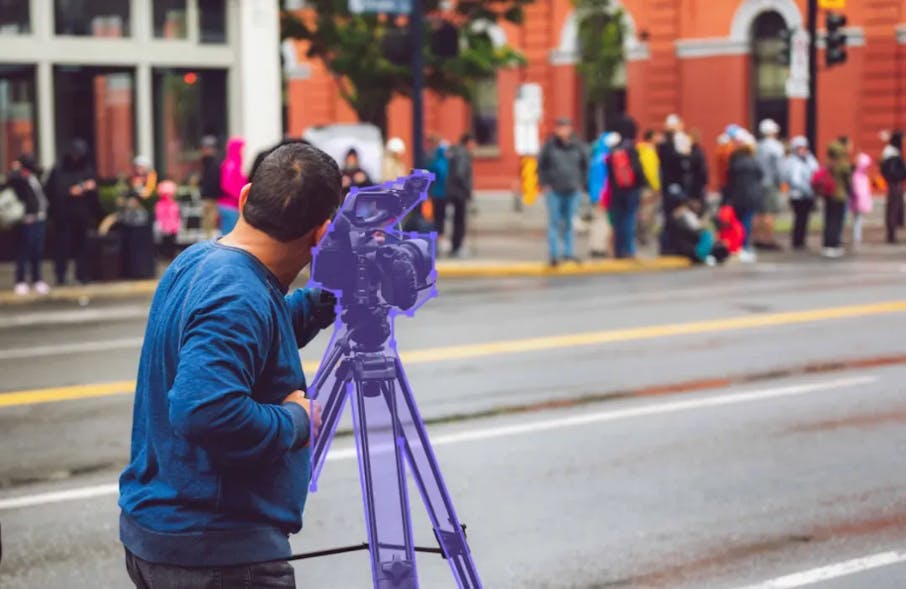
Automating video labeling can significantly speed up the annotation process, reduce costs, and improve consistency and accuracy. In this article, we explore four powerful techniques that can transform your video annotation workflow. Keep reading to learn how these innovations can save you time and money while enhancing the effectiveness of your video labeling projects.
Automated video labeling saves companies a lot of time and money by accelerating the speed and quality of manual video labeling, and eventually taking over the bulk of video annotation work.
Once you start using machine learning and AI-based algorithms for video annotation — using large amounts of labeled videos — and ensuring those videos are accurately labeled is crucial to the success of the project.
Why Video Annotation is Crucial for Machine Learning
Video annotation is a cornerstone of machine learning, especially in applications requiring visual data. As videos become an increasingly important data source in industries such as healthcare, automotive, retail, and security, the ability to extract meaningful insights from them depends heavily on accurate annotations. Here’s why video annotation plays such a critical role:
Training High-Performing Models
Machine learning models require large amounts of labeled data to learn and generalize effectively. In the case of video, annotation provides the structured, labeled datasets that enable models to recognize patterns, detect objects, and understand temporal dynamics. For example, in self-driving cars, video annotation helps systems identify pedestrians, road signs, and other vehicles over time, ensuring safe and reliable navigation.
Enabling Temporal Context
Unlike still images, videos contain temporal information that is crucial for understanding motion and context. Annotating sequences of frames allows machine learning models to grasp changes over time, such as the trajectory of a moving object or the progression of events.
Improving Accuracy Through Contextual Understanding
Video annotation ensures models can interpret the broader context within a scene. For instance, in healthcare applications, annotated surgical videos (DICOM) can teach models to differentiate between tools, tissues, and surgical techniques. The additional contextual cues from videos provide a richer training dataset than isolated images, improving the accuracy of model predictions.
Handling Unstructured Data
Videos are inherently unstructured and massive in volume, making raw data challenging to utilize. Annotation transforms this raw data into a structured format that machine learning algorithms can process. This transformation is key to unlocking the potential of video data in solving real-world problems.
Challenges With Manual Video Annotation
Labor-Intensive & Expensive
Manually annotating video data presents a range of significant challenges. First and foremost, the process of generating accurate labels frame by frame is highly labor-intensive. Each video is composed of thousands or even millions of frames, and each frame requires careful analysis and detailed labeling. This sheer volume of work makes manual annotation not only time-consuming but also prohibitively expensive for many organizations, as it often demands the efforts of an entire team of skilled annotators working over long periods.
Outsourced Annotation is Not Always High-Quality
To mitigate these costs, businesses and organizations frequently outsource video annotation tasks to third-party services. While outsourcing might seem like a cost-effective solution at first glance, it introduces its own set of complications. Outsourced teams often face communication barriers, lack of domain-specific expertise, and differing quality standards, all of which can lead to inconsistent or subpar results. Additionally, outsourcing rarely addresses the underlying inefficiency of manual annotation; the process remains slow and prone to human error, especially when handling large-scale datasets.
Solution: Automated Video Annotation Tools
Automated video annotation tools offer a transformative solution to these challenges. By leveraging advanced technologies like machine learning and computer vision, these tools significantly reduce the need for manual inputs. Automation not only accelerates the annotation process but also reduces overall costs while enabling organizations to work with much larger datasets. Perhaps most importantly, automated systems ensure a higher degree of consistency and accuracy in the annotations, helping maintain the quality and reliability of the labeled data.
For organizations aiming to scale their machine learning projects or manage extensive video datasets, automated annotation provides a way to overcome the bottlenecks of manual processes. It enables them to focus resources on deriving insights and developing applications, rather than on the labor-intensive task of labeling data.
Steps for Automating Video Annotation
#1: Multi-Object Tracking (MOT) to Ensure Continuity from Frame to Frame
Tracking objects automatically is a powerful automated video annotation feature. Once you’ve labeled an object, you want to ensure it’s tracked correctly and consistently from one frame to the next, especially if it’s moving and changing direction or speed. Or if the background and light levels change, such as a shift from day to night.
Not only that but if you’ve labeled multiple objects, you need an AI-based video annotation tool capable of tracking every single one of them. The most powerful automated video labeling tool tracks pixels within an annotation from one frame to the next. This shouldn't be a problem even if you are tracking multiple objects with automatic annotation.
Multi-object tracking is especially useful when processing videos through a machine learning automation tool and an asset when analyzing drone footage, surveillance videos, and in the healthcare and manufacturing sectors. Healthcare companies often need to annotate and analyze surgical or gastroenterology videos, whereas manufacturers need clearer, annotated videos of assembly lines.
Automated object tracking for video annotation in Encord
#2: Use Interpolation to Fill in the Gaps
In automated video annotation or labeling, interpolation is the act of propagating labels between two keyframes. Say an annotation team has already manually labeled objects within hundreds of keyframes, using bounding boxes or polygons — at the start and end of a video. Interpolation accelerates the annotation process, filling in the details within the unannotated frames.
However, you must use interpolation carefully, at least when starting out with a video annotation project. There’s always a trade-off between speed and quality. Dependent, of course, on the quality of the labels applied and the complexity of the labeling agents used during the model training stage.
For example, a polygon applied to a complex multi-faceted object that’s moving from one frame to the next might not interpolate as easily as a simple object with a bounding box around it that’s moving slowly. As annotators know, this entirely depends on how much is changing in the video from one frame to the next.
When polygons are drawn on an object in a video, supported by a proprietary algorithm that runs without a representational model, it can tighten the perimeter of the polygon, interpolate, and track the various segments (in this case, clothes) within a moving object, e.g., a person.
Interpolation to support video annotation in Encord
#3: Use Micro-Models to Accelerate AI-assisted Video Annotation
In most cases, machine learning (ML) models and AI-based algorithms need vast amounts of data before they can produce meaningful results. Not only that, but the data going in should be clean and consistent. Otherwise, you risk the whole project taking much longer than anticipated or having to start over again.
Automated video labeling and annotation are complicated. This method is also known as model-assisted labeling (MAL), or AI-assisted labeling (AAL). This type of labeling is far more complex than annotating static images or applying ML to vast Excel spreadsheets and other data sources.
Conversely, micro-models are powerful, tightly-scoped approaches that over-fit data models to bootstrap your video annotation tasks. Training machine learning algorithms using micro-models is an iterative process that requires manual annotation and labeling at the start. However, you don’t need nearly as much manual work or time spent training the model as you would with other video annotation platforms.
In some cases, you can train micro-models on as few as five labeled frames. As we outline in another post, “micro-models are annotation-specific models that are overtrained to a particular task or particular piece of data.”
Micro-models are best applied to a narrow domain, e.g., automatically annotating particular objects throughout a long video, and the training data required is minimal. It can take minutes to train a micro-model and only minutes or hours to run through the development cycle. Micro-models save vast amounts of time and money for organizations in the healthcare, manufacturing, or research sectors, especially when annotating complex moving objects.
#4: Auto Object Segmentation to Improve the Quality of Object Segments
Auto-segmentation is drawing an outline around an object and then using an algorithm to automatically “snap” to the contours of the object, making the outline tighter and more accurately aligned with the object and label being tracked from one frame to the next.
Annotators can do this using polygons. You might, for example, need to segment clothes a person is wearing in a surveillance video so that you can see when a suspect takes off an item of clothing to put something else on.
With the right video annotation tool, auto object segmentation is applicable for almost any use case across dozens of sectors. It works on arbitrary shapes, and interpolation can track object segments across thousands of frames. In most cases, the outcome is a massive time and cost saving throughout a video annotation project, resulting in much faster and higher quality segmentations.
Automated object segmentation in Encord
The power of automated video annotation
In our experience, there are very few cases where automatic video annotation can’t play a useful role during video annotation projects. Automation empowers annotators to work faster, more effectively, and deliver higher-quality project outputs.
Experience Encord in action. Try out our automated video annotation features (including our proprietary micro-model approach).
AI-assisted labeling, model training & diagnostics, find & fix dataset errors and biases, all in one collaborative active learning platform, to get to production AI faster. Try Encord for Free Today.
Want to stay updated?
Follow us on Twitter and LinkedIn for more content on computer vision, training data, and active learning.
Better Data, Better AI
Enhance your AI with automated data curation, annotation, and validation.
Try it todayWritten by

Alexandre Bonnet
- Yes, modern automated video labeling tools can handle complex scenarios like fast-moving objects or lighting changes. Features such as multi-object tracking and auto-segmentation ensure labels stay accurate even as conditions change from frame to frame.
- Micro-models are specialized machine learning models that are trained for specific tasks with minimal labeled data. They allow video annotation projects to be sped up significantly by applying narrow, focused algorithms to specific video content, like annotating particular objects across multiple frames, with minimal manual input required.
- Yes, automated video labeling is highly scalable. Once a model is trained, it can quickly process large amounts of video data, applying consistent annotations across thousands of frames without the need for human intervention at each step. This saves time and reduces the cost of annotating large datasets.
- Yes, modern automated video annotation tools can handle a wide range of objects, including people, vehicles, animals, and even complex items like clothing or machinery. These tools are adaptable across different sectors, from healthcare to surveillance to manufacturing, making them versatile for various annotation needs.
- Automated video annotation is particularly beneficial for projects involving large datasets, fast-paced video footage, or those requiring high consistency, such as surveillance footage analysis, drone video processing, and healthcare applications like annotating surgical procedures or gastroenterology videos. It is also effective in manufacturing environments where assembly line videos need annotation.
Explore our products