Encord Blog
The Critical Role of Computer Vision in Cancer Treatment
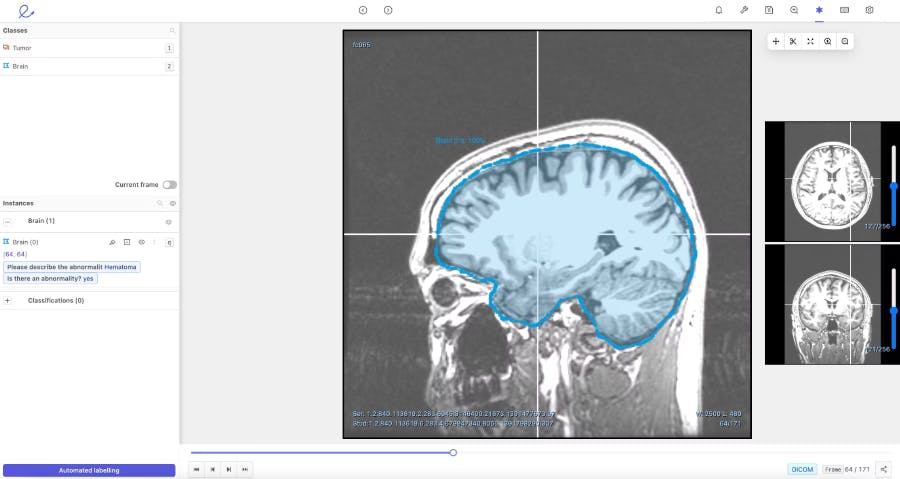
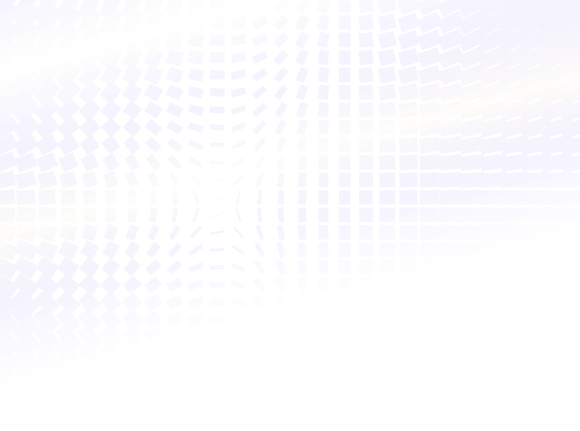
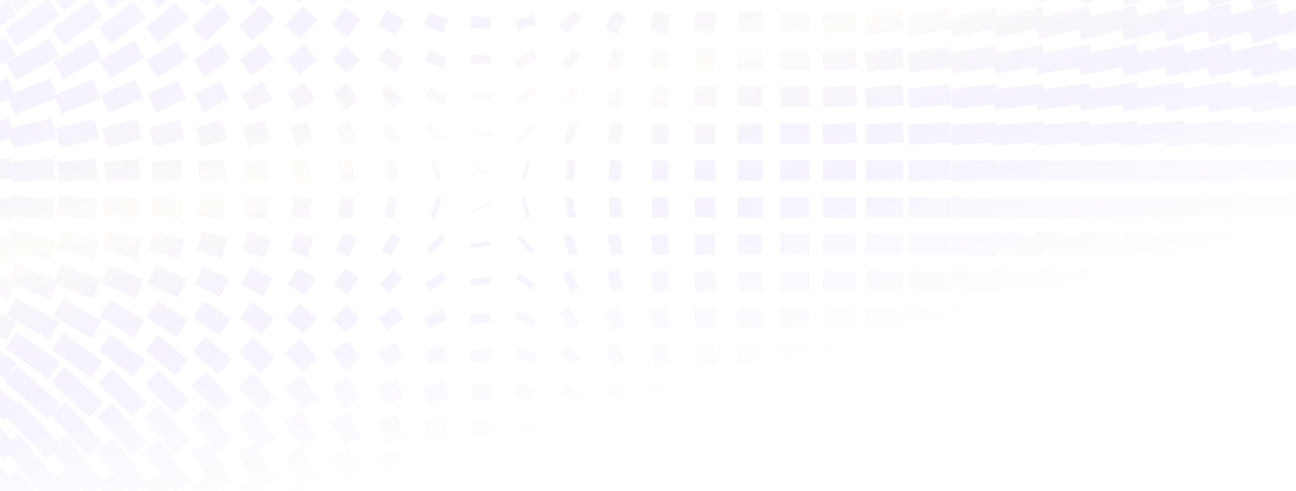
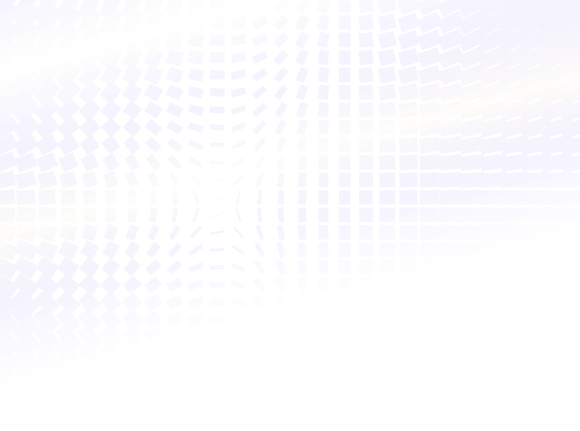
Power your AI models with the right data
Automate your data curation, annotation and label validation workflows.
Get startedWritten by

Eric Landau
This post is about hope–the hope that machine learning and computer vision can bring to physicians treating cancer patients.
Because cancer kills approximately 10 million people each year, I expect most readers to have known someone who died from or experienced the disease. After decades of research and clinical trials, it remains the world’s leading cause of death. It is also an extremely taxing disease to endure, with many experiencing terrible pain.
When it comes to treatment, about 60 percent of patients undergo some form of chemotherapy. Chemotherapy can and does save lives, but it's accompanied by hair loss, fatigue, and vomiting (which films show) as well as swollen limbs, bruising, and peripheral neuropathy (which they don’t). There’s a bleak expression that sums up the chemo experience: “Kill the cancer before the chemo kills you.”
New treatments, such as immunotherapy, might turn out to be game changers for a cancer prognosis, but most doctors will tell you that the best news that a cancer patient can hear are four little words: “We caught it early.”
I know firsthand the power of those words. They caught my father’s cancer early. At a late-stage diagnosis, his odds of a five-year survival would have been less than 30 percent. Caught early, that rate jumped to well over 90 percent.
When doctors catch cancer early, survival rates increase tremendously: at later stages, the cancer has metastasized, spreading throughout the body, which makes effective treatment more difficult.
When it comes to cancer, an ounce of prevention is worth a pound of cure–and that’s where the hope comes in.
Using Machine Learning and Computer Vision to Prevent Late-Stage Cancer Diagnosis
Machine learning and AI technologies are advancing rapidly and bring with them a tremendous amount of hope for early cancer detection and diagnosis.
Physicians already use medical imaging and AI to detect abnormalities in tissue growth. After being trained on large datasets, computer vision models can perform object detection and categorisation tasks to help doctors identify abnormalities in polyps and tissues and to discern whether tumours are malignant or benign.
However, because computer vision gives clinicians an extra pair of eyes, these models can have the potential to catch subtle indications of abnormalities even when doctors aren’t looking for cancer. Doing so can endow doctors with a huge amount of diagnostic power, even outside of their speciality area.
For instance, if a GP scanned a patient for gallstones, she could also feed the scan to a computer vision model that’s running an algorithm to detect abnormalities in the surrounding regions of the body. If the model noticed anything abnormal, the GP could alert the patient, and the patient could see a specialist even though they haven’t yet experienced any external symptoms from the tumour. Such proactive and preventative care has major implications for catching cancer early. For many cancers, and especially for those where there is no regular screening, external symptoms– such as weight loss, pain, and fatigue–often correlate with the progression of the disease, meaning that by the time a patient has cause to see a specialist, it could already be too late for effective treatment.
Because computer vision can enable doctors of any speciality to scan for early signs of cancer, these models also have the potential to democratise healthcare for those living in rural areas and developing nations. The best cancer doctors in the world help to curate, train, and review these algorithms, so the models apply their expertise when looking at patient data. With this technology, hospitals anywhere can provide patients with the expertise of a best-in-class oncologist. While the world has a limited number of high-quality oncologists, these algorithms are infinitely scalable, meaning that the expertise of these doctors will no longer be reserved for patients receiving care from world’s leading hospitals and research institutions.
Building the Medical Imaging and Diagnosis Tools of the Future
Companies across the globe are working to build these diagnostic tools, and, to do so, they need to train their computer vision models to the highest standards.
When building a computer vision model for medical diagnosis, the most important factor is to ensure that the quality of the ground truth is very high. The training data used to train the model must be of the same standard as to what a doctor would have signed off on. The annotations must be accurate and informative, and the distribution of the data must be well-balanced so that the algorithm learns to find its target outcome in examples that represent a variety of real world scenarios. For instance, having demographic variety in the dataset is extremely important. An algorithm trained only on data from college students of a certain ethnic background would not reflect the balance of the real world, so the model wouldn’t be able to make accurate predictions when run on data collected from people of varying demographics.
Building these models also requires a lot of collaboration between doctors and machine learning engineers and between multiple doctors. This collaboration helps ensure that the model is being designed to answer the appropriate questions for the real-world scenario faced by the end-user and that it is learning to make predictions from practising on high-quality training data.
Without the expertise of both data scientists and clinicians during the design and training phases, the resulting model won’t be very effective. Designing clinical grade algorithms requires the input of both sets of stakeholders. Machine learning engineers need to work closely with physicians because physicians are the end-users. By consulting with them, the engineers gain access to a full set of nuanced questions that must be answered for the product to achieve maximum effectiveness in a real world use case.
However, doctors need to be able to work closely with each other, too, so that they can perform thorough workflow reviews. A workflow review contains two parts: the groundtruth review and the model review. For a ground truth review, doctors must check the accuracy of the machine-produced annotations on which the model will be trained. For the model review,doctors check the model’s outputs as a way of measuring its performance and making sure that it’s making predictions accurately. Having multiple doctors of different experience and expertise levels perform workflow reviews and verify the model’s outputs in multiple ways helps ensure the accuracy of its predictions. Often, regulatory bodies like the FDA require that teams building medical models have at least three doctors performing workflow reviews.
Machine learning engineers might be surprised to learn how often doctors disagree with one another when making a diagnosis; however, this difference of opinion is another reason why it’s important for engineers to consult with multiple doctors. If you work with just one doctor when training your model, the algorithm is only fit to that doctor’s opinion. To achieve the highest quality of model, engineers need to accommodate multiple layers of review and multiple opinions and arbitrate across all of them for the best result. Doctors in turn need tools that are catered for them and their workflows, tools that allow them to create precise annotations.
To facilitate the review process and help doctors annotate more efficiently, Encord developed our DICOM annotation tool– the first comprehensive annotation tool with native 3D annotation capabilities designed for medical AI. It is built to handle multiple medical imaging modalities, including CT, X-ray, and MRI. Our DICOM tool combines an approach of training and running automated models, with human supervision to review and refine labels. When it comes to ground truth review, the tool improves efficiency, reduces costs, and increases accuracy– making it an asset for time-pressed doctors and cost conscious hospitals.
When building our DICOM 3D-image annotation tool we consulted with physicians in King’s College, academics at Stanford, and ML experts at AI radiology companies building these types of medical vision models. As a result, we knew that our platform needed to have a flexible interface that enabled collaboration between clinical teams and data science teams. It had to be able to support multiple reviews, facilitate a consensus across different opinions, and perform quality assessments to check the ground truth of the algorithm automating data labelling.
We developed our DICOM annotation tool to augment and replace the manual labelling process that makes AI development expensive, time consuming, and difficult to scale. Currently, most AI development relies on outsourcing data to human labellers, including clinicians. The human error that arises during this process results in doctors having to waste time reviewing and correcting labels. With the DICOM image annotation tool, we hope to save physicians valuable time by giving them the right tools and by reducing their burden of manual labelling.
It’s an upstream approach, but creating our DICOM image annotation tool is our way of contributing to early cancer detection and prevention. Organisations that use the tool can increase the speed at which computer vision models can enter production and become viable for use in a medical environment.
The Future of AI and Medicine
The commercial adoption of medical AI will revolutionise healthcare in ways we can’t yet imagine.
With this technology, we can accelerate medical research by 100x. Think about how medical research was performed in the era before? Doctors and researchers had to write notes in a physical spreadsheet and perform analysis using slide rulers. Each step was immensely time consuming. Computers–though not designed to be a directly relevant technology for medical research– transformed the way research was done because of their power and universal reach.
Computer vision and machine learning will do the same.
Biological research and technology will advance in tandem, and working together, machine learning engineers and clinicians will be able to offer people preventative care rather than forcing them to wait until something egregious arises that warrants treatment.
Prevention of disease is faster, cheaper, and safer than treatment of a disease. We don’t yet have the AI tools to implement this vision at scale, but, with the rise of data-centric AI and advancements in data annotation, machine learning, and computer vision, we will get there.
Using the power of medical AI, we can transform our medical systems from focusing on “sick care” to focusing on “health care”. In doing so, we can spare people from both the life-or-death consequences of receiving a cancer diagnosis “too late” and the human suffering that accompanies current treatment methods.
Like I said, it’s a story of hope. And it’s just beginning.
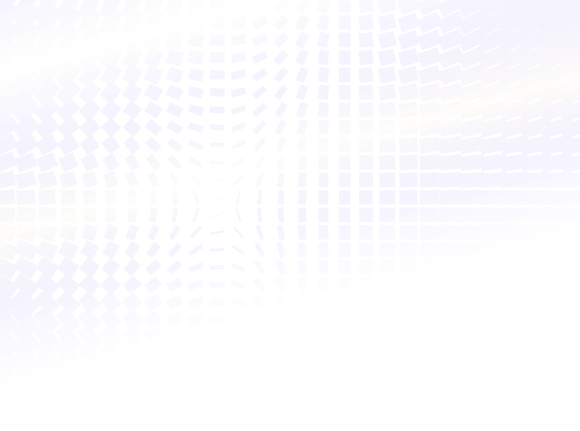
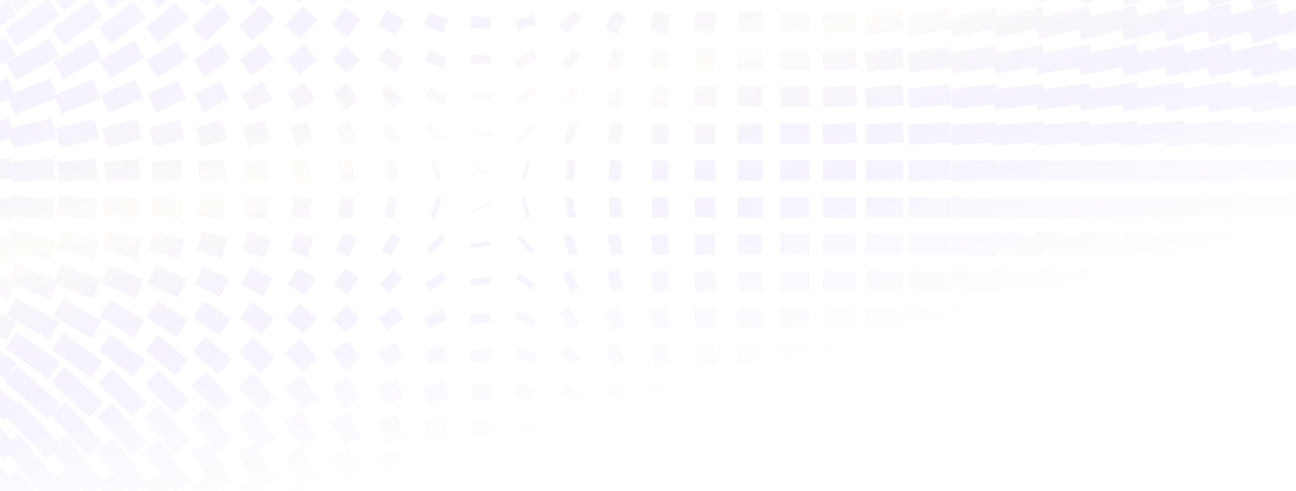
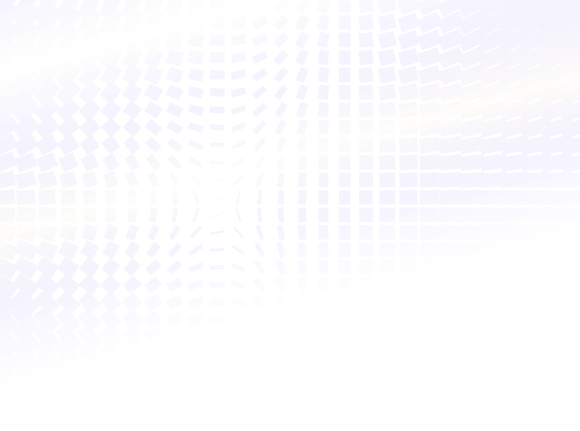
Power your AI models with the right data
Automate your data curation, annotation and label validation workflows.
Get startedWritten by

Eric Landau
Related blogs
The State of AI in Surgical Robotics
In 1985, the PUMA 560 surgical robot made history by assisting the team at Memorial Medical Center during a stereotactic brain biopsy, marking one of the earliest recorded instances of robotic-assisted surgery and astonishing the world. Fast forward to today — surgical robotic systems are supporting surgeons across a growing array of medical interventions, assisting surgeries in ways few people imaged a few decades ago. Over the past eight years alone, the Robotically-Assisted Surgical (RAS) Devices market has expanded from $800 million in 2015 to well over $3 billion today. From prominent healthcare organizations to cutting-edge research institutes, from rapidly growing startups to non-profit initiatives, diverse teams are busy developing innovative surgical robotic systems. Their goal is to enhance surgical efficiency, improve precision and, ultimately, deliver better outcomes for patients. The recent leaps in computer vision have also further spurred this growth, as artificial intelligence is rapidly entering the operating room and enabling these systems to better perceive and interpret visual information in real time and aid surgeons on a wider range of tasks. This article explores the landscape of AI applications in surgical video analysis, some of the key innovators in the space and the role of high-quality training data in the development of AI-assisted surgical systems. AI-Assisted Surgical Robotics Companies like Intuitive Surgical, creator of the Da Vinci Surgical System, led the way in the 1990s: Da Vinci was the first robotics system approved by the FDA, initially for visualization and tissue retraction in 1997 and later for general surgery in 2000. With over 6,000 robots installed worldwide and over $6b in annual revenue, Intuitive has dominated the surgical robotics industry for the better part of the last 20 years, transforming the industry and enabling patient outcomes that were previously impossible. Yet 2019 marked the start of some of its patent expiries, and with that, a wave of new entrants and innovators. The use of AI-assisted techniques in robotics now extends from preoperative planning, to intraoperative guidance and postoperative care, and has advanced significantly thanks to the close collaboration of surgeons, programmers, and scientists. Let’s discuss some of the major real-world applications and teams working in this field — starting with preoperative planning. Preoperative planning Preoperative (pre-op) planning includes a range of workstreams — from visualizing the steps of the operation, to forming a plan to tackle navigation or improve precision. Machine learning and computer vision are being leveraged in pre-op planning in many ways: from rapidly analyzing the tabular and visual data of patients (like medical records or scans), to ensuring precise trajectory planning, optimizing incision sites, and gaining more insights into potential complications. Surgical planning begins with processing and fusing various medical imaging modalities, such as CT scans, MRI scans, and ultrasound scans, to generate a comprehensive 3D model of the patient's anatomy. Computer vision algorithms and deep learning models are then employed to quickly analyze this visual data and surface recommendations and risks with pursuing different surgical steps. Algorithms also enable surgeons to identify and segment specific anatomical structures and regions of interest from the imaging data (like organs, blood vessels, abnormalities, and other critical structures within the 3D model). This segmentation is crucial for surgical planning as it provides a clear visualization of the target area. From here, surgeons can explore different surgical approaches and plan the optimal trajectory for instruments and incisions, assessing the risk factors by quantifying the distance or overlap between the planned surgical path and nearby structures. Pre-op data can also be combined with intraoperative data to achieve surgical outcomes not otherwise possible. One of the most innovative end-to-end platforms is Paradigm™ by Proprio Vision, who just a few days announced the successful completion of the world's first light field-enabled spine surgery. Using an array of advanced sensors and cameras, Paradigm captures high-definition multimodal images during surgery and integrates them with preoperative scans to provide surgeons with real-time mapping of the anatomy. In addition to augmenting navigation capabilities during a procedure, Paradigm also collects large amounts of pre-op and intra-op data to inform future surgical decision-making and improve surgical efficiency and accuracy. You can read more about Proprio's announcement on their website here. Another end-to-end robotic system is Senhance, by Asensus Surgical, which in 2021 was cleared by the FDA for general surgeries. Senhance allows surgeons to create simulations for preoperative planning, while also providing real-time data for intraoperative guidance and generating insightful analytics for postoperative performance assessments and care. Intraoperative guidance A recent report by Bain & Company found that over 50% of surgeons surveyed made use of robotic systems in some capacity during general surgeries. During procedures, where even the slightest hand trembling can risk causing significant harm, image-guided surgery is turning into a requirement. Here, computer vision is often employed for instrument tracking and object recognition, which in turn are leveraged to feed video data to AI models that can monitor the procedure and generate guidance and warnings in case of anomalies, such as excessive bleeding or tissue damage. AI-assisted systems allow surgical robots to locate and follow the movement of surgical instruments, ensuring they are precisely positioned and maneuvered. They can also be used to identify critical structures and masses in the video footage, providing augmented guidance to the surgeon in real time. Model-assisted annotations of polyps in the Encord training data platform General and Minimally Invasive Surgery (MIS) Robotic assisted devices are more and more frequent in Minimally Invasive Surgeries (MIS). The primary objective of MIS is to reduce the trauma to the patient's body; the incision surface area is smaller, and often serves as an entry point, or port, for specialized instruments and a camera, known as a laparoscope, to enter the tissues and feed back real-time video data, which allows surgeons to view internal stuctures on a monitor and be guided through the procedure. MIS employs long, thin instruments with articulating tips that can be maneuvered through the small incisions. Systems like Dexter (by Distalmotion) are currently being used for daily gynecology, urology and general surgery procedures in Europe. “Surgeons can choose to operate entire procedures robotically, or they can leverage the ability to easily switch between the robotic and laparoscopic modalities to perform specialized tasks such as stapling with their preferred and trusted instruments,” Distalmotion CEO Michael Friedrich said in a recent press release announcing their upcoming US expansion. Another promising platform is Maestro (built by Moon Surgical), which sits at the intersection of robotic-assisted surgery and conventional surgery: acting as a robotic surgical assistant, it augments the precision and control of laparoscopic surgery, increasing the dexterity of a surgeon's own hand. Just this month, Moon Surgical announced the successful completion of the first 10 laparoscopic surgeries with its Maestro system in France. The procedures — bariatric and abdominal surgery procedures — were performed by laparoscopic surgeons Dr. Benjamin Cadière and Dr. Georges Debs, who said that the platform provided them with stability and precision that are difficult to match with human assistance. Many different procedure types are benefitting from the innovation in surgical assisted devices. A few examples are: Orthopedic Surgery. Orthopedic surgery is primarily used for the treatment of musculoskeletal conditions and disorders, mostly relating to bones and joints. With deep learning and computer vision, surgeons can build a pre-op model to plan the creation of patient-specific implants and the precise alignment of bones and joints, and then leverage a robotic arm to facilitate the optimal placement during the surgery. Stryker, the creators of the MAKO surgical assistant, are one of the pioneers in this space: MAKO turns a CT scan of a patient's joint into a 3D model, measures soft tissue balance, and, during surgery, ensures the placement is optimized to the patient's anatomy. Ganymed Robotics is another innovator in the space of orthopedic robotics. The Paris-based startup's team of computer vision and deep learning imaging experts have built a tool that leverages multimodal sensors to improve hard tissue surgery, starting with total knee arthroplasty (TKA). Robotic Bronchoscopy. Bronchoscopy helps evaluate and diagnose lung conditions, obtain samples of tissue or fluid, and remove foreign bodies. During a robotic bronchoscopy, the doctor uses a controller to operate a robotic arm, which guides a catheter (a thin, flexible, and maneuverable tube equipped with a camera, light, and shape-sensing technology) through the patient’s airways. Noah Medical received FDA clearance earlier this year for its Galaxy System™: a computer vision powered lung navigation system that improves the visualization and access of robotic brochoscopies. Microsurgery. Microsurgery requires the use of high-powered microscopes and precision instruments to perform intricate procedures on tiny structures within the body, such as blood vessel, nerve and tissue repairs. These kinds of surgeries operate hard-to-see anatomical structures that are often invisible to the human eye, and surgeons performing them need to undergo extensive training to develop exceptional hand-eye coordination. A handful of computer vision powered systems are being built to help improve the outcomes of these delicate surgeries, like MUSA-3, the microsurgery robot by Microsure, which allows surgeons to use a joystick to control instrument positioning during lymphatic surgery. The system is optimized for tremor-filtered movements and high-precision, and uses high-definition on-screen displays to enable real-time image analysis during these exceptionally delicate procedures. The Microsure team raised a €38m Series B earlier this month, as they eye FDA clearance in the US and CE-mark in Europe. Postoperative analysis and training Successful patient outcomes are achieved before, during, and after what happens in the operating room. AI surgical systems are valuable in post-operative analysis, as surgeons can review the process to understand improvement areas, identify potential health risks for the patient, and share insights to align expectations. Video data can also help trained newly formed surgeons, and provide education and knowledge share for the academic surgery community. Annotated surgical videos contain information regarding critical procedures, and can help inform students about effective surgical practices or risks involved with specific techniques. AI systems can also assess surgical performance by monitoring live video feeds and comparing a surgeon’s techniques with those used in similar procedures previously. The system can record custom metrics such as an operation’s total duration, patient satisfaction and post-operative complications, establishing benchmarks and shared understanding. A leader in this space is Orsi Academy, a Belgian training and research community that helps train medical professionals in new AI-driven techniques, such as computer vision for analyzing surgical videos, surgical data science for performance evaluation, and 3D printing, to simulation to help surgeons better understand and view specific body parts and surgical sites. Just a few days ago, Orsi Academy announced that their augmented reality tool (developed by Orsi Innotech) had enabled surgeons at Erasmus Medical Center to perform the world's first robot-assisted lobectomy using augmented reality, marking a huge achievement for the AI-assisted world of surgery. During this surgery, virtual overlay of the tumor, blood vessels and airways were projected over the camera image of the patient’s lung and was rendered with real-time AI-assisted robotic instrument detection. This allows surgeons to find their way inside the patient’s body more safely & effectively. Orsi Academy will be hosting their annual Orsi Event in Belgium, on December 14th and 15th. Details will be available on their website shortly.
Oct 25 2023
5 M
Best DICOM Labeling Tools [2024 Review]
The FDA has approved over 300 AI algorithms over the last 4 years – the vast majority of which relate to medical imaging. With the increase in medical AI and computer vision applications, healthcare teams are turning to AI models for more accurate and faster diagnosis at scale. A correct or incorrect diagnosis impacts treatment, care plans, and outcomes. And ultimately, computer vision and machine learning applications across medical AI have the potential to materially impact the chances of a positive outcome. And as we know, it all starts with data. Getting a radiology AI product to market – not to mention through FDA or CE clearance – starts with data quality and speed, which in turn relies heavily on accurate annotation and labels, whether the images come from CT, X-ray, PET, ultrasound, or MRI scans. To help you navigate all the DICOM labeling tools and frameworks on the market, we have compiled a list of the most popular annotation tools for annotating DICOM and NIfTI files. 💡Read more: Our Encord DICOM June product updates are out! Whether you are: A data science team at a fast-growing radiology AI startup trying to bring your first products to market or obtain FDA approval A data operations team at a large healthcare organization evaluating medical imaging tools to help your team analyze CT scans and MRI scans ...or a computer vision team at a healthcare provider or vendor delivering high-value machine learning-based solutions for hospitals, doctors, and other medical professionals. This guide will help you compare the top tools to annotate DICOM and NIfTI files and help you find the right one for you. We will compare them across a few key features – collaboration, quality control (QC) and quality assurance (QA), and ease of use for annotators and medical data operations managers. If you’re evaluating NIfTI labeling tools, you can find more about the key features you need to look out for here. So let’s get into it! In this post, we’ll cover six of the most popular AI-based medical image annotation tools: Encord DICOM 3D Slicer Labelbox Kili ITK-Snap MONAI Review of 6 Best Medical AI Annotation Tools for DICOM Encord DICOM Encord is the leading DICOM annotation platform trusted by leading medical AI teams at healthcare institutions. Encord’s AI-based annotation tool was purpose-built in close collaboration with healthcare teams for machine learning and computer vision projects in the medical profession. Encord and Encord Active are designed to handle vast medical image and video-based datasets (e.g. surgical video), alongside DICOM, NIfTI and +25 other data formats. Benefits & Key features: Native DICOM rendering: Render 20,000+ pixel intensities natively in the browser with a PACS-style interface. 3D views: Multiplanar reconstruction (axial, coronal, and sagittal views) and maximum intensity projection (MIP). Windowing support: Preset window settings for numerous modalities and the most common objects that need detecting, identifying, and annotating (e.g., lung, bone, heart, brain, etc.). Hanging protocols support: For Mammography, CT and MRI. Expert review workflows: Collaborative workflows designed for medical teams and scalable data operations. Foundation models support: Generate mask predictions with our AI-based auto-segment tool. Configurable labeling protocols: Create complex medical labels and protocols to train your annotation team with our medical-grade annotation tool. Support for multiple annotation types: Bounding boxes, polygons, segmentation, polylines, keypoints, object primitives, and classification. Best for: Teams rolling out new healthcare AI models, computer vision DataOps teams, annotation providers, ML engineers, and data scientists in medical organizations. Pricing: Free trial model and simple per-user pricing after that. 💡 More insights on labeling DICOM with Encord: Here are some examples of healthcare and medical imaging projects that Encord has been used for: Floy, a radiology AI company that helps radiologists detect critical incidental findings in medical images, reduces CT & MRI annotation time with AI-assisted labeling. RapidAI reduced MRI and CT annotation time by 70% using Encord for AI-assisted labeling. Stanford Medicine cut experiment duration time from 21 to 4 days while processing 3x the number of images in 1 platform rather than 3 Further reading: Best Practice for Annotating DICOM and NIfTI Files The 7 Features to Look Out For When Choosing a DICOM Annotation Tool 3D Slicer 3D Slicer is an open-source software application designed for medical image processing and visualization. It provides a platform for 3D image segmentation and registration. The US The National Institutes of Health (NIH) and other healthcare partners have played an important role in funding 3D Slicer, alongside Harvard Medical School, and dozens of other public and private funding sources. There have been numerous contributors to 3D Slicer, with an active community improving the source code, architecture, building modules, securing funding, and citing 3D slicer in medical computer vision and machine learning model training experiments and development. Benefits & Key features: Easy (& free) to get started labeling DICOM files. Great for manual data annotation — also supports semi-assisted labeling. Robust ground-level annotation capabilities (including classification and object detection) for a broad set of computer vision use cases. Best for: Students, researchers, and academics testing the waters with DICOM annotation (perhaps with a few files or a small open-source medical imaging dataset). Pricing: Free! 💡 More insights on image labeling with 3D Slicer: If your team is looking for a free annotation tool, you should know… 3D Slicer is one of the most popular open-source tools in the space, with over 1.2 million downloads since it was launched in 2011. Other popular free image annotation alternatives to 3D Slicer are CVAT, ITK-Snap, MITK Workbench, HOROS, OsiriX, MONAI and OHIF Viewer. If data security is a requirement for your annotation project… Commercial labeling tools will most likely be a better fit — as key security features like audit trails, encryption, SSO, and generally-required vendor certifications (like SOC2, HIPAA, FDA, and GDPR) are not available in open-source tools. Further reading: Buy vs build for computer vision annotation - what's better? Overview of open-source annotation tools for computer vision Labelbox Labelbox is a US-based data annotation platform founded in 2018, after the founders experienced the difficulties associated with building in-house ML operations tools. Like most of the other platforms mentioned in this guide, Labelbox offers both an image labeling platform, as well as labeling services. Teams can annotate a wide range of data types (PDF, audio, images, videos, and more) using the Labelbox data engine that can be configured for numerous ML, AI, and computer vision use cases. Benefits & Key features: Support for two annotation types – polyline and segmentation – and common imaging modalities – CT, MRI, and ultrasound. SaaS or on-premise workflows with privacy and security built-into the platform. Catalog view to help medical annotation teams label and sift and find patterns within vast multi-format datasets. Best for: Teams wanting to annotate other file formats alongside DICOM, like documents, video, text, audio, and PDFs. Pricing: 10,000 free LBUs to begin with, and custom pricing beyond that. 💡 More insights on labeling DICOM with Labelbox: If your team is looking for on-demand labeling services, you should know… Labelbox can connect your in-house team with outsourcing partners for large ML annotation projects. If data security is a requirement for your annotation project… Labelbox comes with enterprise-grade security as standard for healthcare and AI teams. Further reading: Top 10 Free Healthcare Datasets for Computer Vision 3 ECG Annotation Tools for Machine Learning Kili Kili is a data annotation platform founded in 2018 by a French team who had previously built the AI company, MyElefant, and an AI lab from scratch for BNP Paribas. The platform allows users to create and manage annotation projects, monitor progress, and collaborate with team members in real time. Kili has been used by businesses across various industries, including healthcare, finance, and retail, to accelerate their AI development. Benefits & Key features: Support for multiple annotation types, including text, image, video, and audio. A platform designed to label, find, and fix data annotation issues and simplify DataOps for AI teams of every size. For small-scale projects, DataOps can implement Kili with 5 lines of code to turn a machine learning workflow into a data-centric AI workflow. Best for: ML and DataOps teams across a range of sectors, either with in-house or outsourced teams. Pricing: Free tier for individuals, alongside corporate and enterprise plans for businesses. 💡 More insights on labeling DICOM with Kili: If your team is looking for an easy-to-integrate ML tool, you should know… Kili was designed to embed into ML workflows easily – it doesn’t have as many features as some computer vision SaaS products, but it integrates rapidly in a wide range of data tech stacks. Further reading: How to Annotate DICOM and NIfTI Files Medical Image Segmentation: A Complete Guide ITK-Snap ITK-Snap is a free, open-source, multi-platform software application used for image segmentation. ITK-Snap provides semi-automatic segmentation using active contour methods as well as manual delineation and image navigation. ITK-Snap was originally developed by a team of students at the University of North Carolina led by Guido Gerig (NYU Tanden School of Engineering) in 2004. Since then, it’s evolved considerably, now being overseen by Paul Yushkevich, Jilei Hao, Alison Pouch, Sadhana Ravikumar and other researchers at the Penn Image Computing and Science Laboratory (PICSL) at the University of Pennsylvania. The latest version, ITK-Snap 4.0, was released in 2020, funded by a grant from the Chan-Zuckerberg Initiative. Benefits & Key features: Manual segmentation in three planes. Support for additional 3D and 4D image formats alongside DICOM, like NIfTI. A 3D cut-plane tool for faster processing of image segmentation results and multiple images, including an advanced distributed segmentation service (DSS). Best for: Medical image annotation, students, and research teams. Pricing: Free! Further reading: 9 Best Image Annotation Tools for Computer Vision [2024 Review] The Top 6 Artificial Intelligence Healthcare Trends of 2024 MONAI MONAI is an open-source, PyTorch-based framework designed for deep learning in medical imaging. The project was started in 2019 by NVIDIA, the National Institutes of Health (NIH), and other contributors. The framework provides various tools, including a labeling tool, to assist in the creation of annotated datasets for training deep learning models. MONAI’s labeling tool allows users to annotate images with 2D or 3D bounding boxes, segmentation masks, and points. The annotations can be saved in a variety of formats and easily integrated into the MONAI pipeline for training and evaluation. MONAI has gained popularity due to its ease of use and its ability to accelerate research in medical imaging. Benefits & Key features: Easy (& free) to get started labeling biomedical and healthcare images with the MONAI Label Server. Capabilities for training AI models for healthcare imaging across a range of modalities and medical specialisms with two transformer-based architectures. Convenient integrations through the MONAI Deploy App SDK. Best for: Medical imaging, annotation, and research teams that need an open-source healthcare AI platform. Pricing: Free! 💡 More insights on labeling DICOM with MONAI: If your team is looking for an open-source alternative to commercial tools, you should know… MONAI is designed as an AI-based collaborative platform with a suite of features you can host and deploy in a wide range of medical environments. If data security is a requirement for your annotation project… MONAI is better equipped than most open-source medical imaging projects with layers of enterprise-grade security. Further reading: 7 Ways to Improve Medical Imaging Dataset Guide to Experiments for Medical Imaging in Machine Learning DICOM Annotation Tools: Key Takeaways There you have it! The 6 most popular annotation tools for annotating DICOM. For further reading, you might also want to check out a few honorable mentions, both paid and free annotation tools: Hive: Cloud-based AI tools for organizations that need to apply labels across a wide range of data types Dataloop: Software to train and improve ML and AI models with extensive annotation capabilities Appen: One of the oldest labeling services platforms on the market, launched in 1996 VOTT: An open-source tool with tags and asset export features compatible with Tensorflow and the YOLO format. Ready to improve the accuracy, outputs, and speed to get your healthcare AI models production-ready with DICOM annotations? Sign-up for an Encord Free Trial: The Active Learning Platform for Computer Vision, used by the world’s leading computer vision teams. AI-assisted labeling, model training & diagnostics, find & fix dataset errors and biases, all in one collaborative active learning platform, to get to production AI faster. Try Encord for Free Today. Want to stay updated? Follow us on Twitter and LinkedIn for more content on computer vision, training data, and active learning. Join our Discord channel to chat and connect.
Jul 04 2023
6 M
Medical Image Segmentation: A Complete Guide
Medical image segmentation is used to extract regions of interest (ROIs) from medical images and videos. When training computer vision models for healthcare use cases, you can use image segmentation as a time and cost-effective approach to labeling and annotation to improve accuracy and outputs. Segmentation in medical imaging is a powerful way of identifying objects, segmenting pixels, grouping them, and using this approach to labeling to train computer vision models. In this guide, we’ll explore medical image segmentation, its role in healthcare computer vision projects, applications, and how to implement medical image segmentation. What is Medical Image Segmentation? Computer vision models rely on large training datasets used to train the algorithmic models (CV, AI, ML, etc.) to achieve high-precision medical diagnostics. An integral part of this process is annotating and labeling the images or videos in a dataset. One method for this is image segmentation, which this article will explore in more detail. Medical image segmentation involves the extraction of regions of interest (ROIs) from medical images, such as DICOM and NIfTI images, CT (Computed Tomography) scans, X-Rays, and Magnetic Resonance Imaging (MRI) files. There are numerous ways to approach segmentation, from traditional methods that have been around for decades to new deep-learning techniques. Naturally, everything in the medical profession needs to be implemented with precision, care, and accuracy. Any mistakes in the diagnosis or AI model-building stage could have significant consequences for patients, treatment plans, and healthcare providers. This guide is for medical machine learning (ML), data operations (DataOps), and annotation teams and leaders wanting to learn more about how they can apply image segmentation for their computer vision projects. Read more: Encord’s guide to medical imaging experiments and best practices for machine learning and computer vision. Why is Medical Image Segmentation used In Healthcare Computer Vision Models? Healthcare organizations, medical data operations, and ML teams can use medical image segmentation for dozens of computer vision use cases, including the following: Radiology Radiology is a medical field that generates an enormous amount of images (X-ray, mammography, CT, PET, and MRI), and healthcare organizations are increasingly turning to AI-based models to provide more accurate diagnoses at scale. Training those models to spot what medical professionals can sometimes miss, or identify health issues more accurately, involves labeling and annotating vast datasets. Image segmentation is one way to achieve more accurate labels so that models can go into production faster, producing the results that healthcare organizations need. Gastroenterology We can say the same about gastroenterology (GI) model development. Machine learning and computer vision models can be trained to more accurately identify cancerous polyps, ulcers, IBS, and other conditions at scale. Especially when it comes to outliers and edge cases that even the most skilled doctors and practice specialists can sometimes miss. Histology Medical image annotation is equally useful for histology, especially when AI models can accurately apply widely-used staining protocols (including hematoxylin and eosin stain (H&E), KI67, and HER2). Image segmentation helps medical ML teams train algorithmic models, implement labeling at scale, and generate more accurate histology diagnoses from image-based datasets. Ultrasound Image segmentation can help medical professionals more accurately label ultrasound images to identify gallbladder stones, fetal deformation, and other insights. Cancer Detection When cancerous cells are more difficult to detect, or the results from scans are unclear, computer vision models can play a role in the diagnosis process. Image segmentation techniques can be used to train computer vision models to screen for the most common cancers automatically, medical teams can make improvements in detection and treatment plans. Looking for a dataset to start training a computer vision model on? Here are the top 10 free, open-source healthcare datasets. Different Ways to Apply Medical Image Segmentation In Practice In this section, we’ll briefly cover 8 types of segmentation modes you can use for medical imaging. Here we’ll give you more details on the following types of image segmentation methods: Instance segmentation Semantic segmentation Panoptic segmentation Thresholding Region-based segmentation Edge-based segmentation Clustering segmentation Foundation Model segmentation For more information, check out our in-depth image segmentation guide for computer vision that also includes a number of deep learning techniques and networks. Instance segmentation Similar to object detection, instance segmentation involves detecting, labeling, and segmenting every object in an image. This way, you’re segmenting an object’s boundaries, and whether you’re doing this manually or AI-enabled, overlapping objects can be separated too. It’s a useful approach when individual objects need to be identified and tracked. Semantic Segmentation Semantic segmentation is the act of labeling every pixel in an image. This will provide a densely labeled image, and then an AI-assisted labeling tool can take these inputs and generate a segmentation map where pixel values (0,1,...255) are transformed into class labels (0,1,...n). Panoptic Segmentation Panoptic is a mix of the two approaches outlined above, semantic and instance. Every pixel is applied a class label to identify every object in an image. This method provides an enormous amount of granularity and can be useful in medical imaging for computer vision where attention to detail is mission-critical. Thresholding Segmentation Thresholding is a fairly simple image segmentation method whereby pixels are divided into classes using a histogram intensity that’s aligned to a fixed value or threshold. When images are low-noise, threshold values can stay constant. Whereas in noisy images, a dynamic approach for setting the threshold is more effective. In most cases, a greyscale image is divided into two segments based on their relationship to the threshold value. Two of the most common approaches to thresholding are global and adaptive. Global thresholding for image segmentation divides images into foreground and background regions, with a threshold value to separate the two. Adaptive thresholding divides the foreground and background using locally-applied threshold values that are contingent on image characteristics. Region-based Segmentation Region-based segmentation divides images into regions with similar criteria, such as color, texture, or intensity, using a method that involves grouping pixels. With this data, regions or clusters are then split or merged until a level of segmentation is achieved. Annotators and AI-based tools can do this using a common split and merge technique or graph-based segmentation. Edge-based Segmentation Edge-based segmentation is used to identify and separate the edges of an image from the background. AI tools can be applied to detect changes in intensity or color values and use this to mark the boundaries of objects in images. One method is the Canny edge detection approach, whereby a Gaussian filter is applied, applying non-maximum suppression to thin the edges and using hysteresis thresholding to remove weak edges. Another method, known as Sobel, involves computing the gradient magnitude and direction of an image using a Sobel operator, which is a convolution kernel that extracts horizontal and vertical edge information separately. Clustering Segmentation Clustering is a popular technique that involves grouping pixels into clusters based on similarities, with each cluster representing a segment. Different methods can be used, such as K-mean clustering, mean-shift clustering, hierarchical clustering, and fuzzy clustering. Visual Foundation Model Segmentation: (SAM) Segment Anything Model Meta’s Visual Foundation Model (VFM), called the Segment Anything Model (SAM), is a powerful open-source VFM with auto-segmentation workflows, and it’s live in Encord! It’s considered the first foundation model for image segmentation, developed using the largest image segmentation known, with over 1 billion segmentation masks. Medical image annotation teams can train it to respond with a segmentation mask for any prompt. Prompts can be asking for anything from foreground/background points, a rough box or mask, freeform text, or general information indicating what to segment in an image. Here’s how to use SAM to Automate Data Labeling in Encord. How to Implement Medical Image Segmentation for Healthcare Computer Vision with Encord With an AI-powered annotation platform, such as Encord, you can apply medical image segmentation more effectively, ensuring seamless collaboration between annotation teams, medical professionals, and machine learning engineers. At Encord, we have developed our medical imaging dataset annotation software in collaboration with data operations, machine learning, and AI leaders across the medical industry – this has enabled us to build a powerful, automated image annotation suite, allowing for fully auditable data and powerful labeling protocols. A few of the successes achieved by the medical teams we work with: Stanford Medicine cut experiment duration time from 21 to 4 days while processing 3x the number of images in 1 platform rather than 3 King’s College London achieved a 6.4x average increase in labeling efficiency for GI videos, automating 97% of the labels and allowing their annotators to spend time on value-add tasks Memorial Sloan Kettering Cancer Center built 1000, 100% auditable custom label configurations for its pulmonary thrombosis projects Floy, an AI company that helps radiologists detect critical incidental findings in medical images, reduces CT & MRI Annotation time with AI-assisted labeling RapidAI reduced MRI and CT Annotation time by 70% using Encord for AI-assisted labeling. Ready to automate and improve the quality, speed, and accuracy of your medical imaging segmentation? Sign-up for an Encord Free Trial: The Active Learning Platform for Computer Vision, used by the world’s leading computer vision teams. AI-assisted labeling, model training & diagnostics, find & fix dataset errors and biases, all in one collaborative active learning platform, to get to production AI faster. Try Encord for Free Today. Want to stay updated? Follow us on Twitter and LinkedIn for more content on computer vision, training data, and active learning. Join our Discord channel to chat and connect.
Jun 08 2023
4 M
3 ECG Annotation Tools for Machine Learning
Machine learning has made waves within the medical community and healthcare industry. Artificial Intelligence (AI) has proven itself useful in numerous uses across a variety of domains, from Radiology and Gastroenterology to Histology and Surgery. The frontier has now hit Electrocardiography (ECG) as well. With an annotation tool, you can annotate the different waves on your Electrocardiogram diagrams and train machine learning models to recognize patterns in the data. The first open-source frameworks have been developed to build models based on ECG data e.g. Deep-Learning Based ECG Annotation. In this example, the author automated the process of annotating peaks of ECG waveforms using a recurrent neural network in Keras. Even though the model was not 100% performant (it struggles to get the input/output right). It seems to work well on the QT database of PhysioNet. The Authors does mention it fails in some cases that it has never seen. Potential future development of machine learning would be to play with augmenting the ECGs themselves or create synthetic data. The 3 main components of an ECG: the P wave, which represents the depolarization of the atria; the QRS complex represents the depolarization of the ventricles; and the T wave, which represents the repolarization of the ventricles. Source: Wikipedia Another example of how deep learning and machine learning is useful in ECG waveforms can be found in the MathWorks Waveform Segmentation guide. Using a Long Short-Term Memory (LSTM) network, MathWorks achieved impressive results as seen in the confusion matrix below: If you want to get started yourself you can find a lot of open-source ECG datasets, e.g. the QT dataset from PhysioNet. Why are ECG Annotations Important in Medical Research? ECG annotation is an essential aspect of medical research and diagnosis, involving the identification and interpretation of different features in the ECG waveform. It plays a critical role in the accurate diagnosis and treatment of heart conditions and abnormalities, allowing you to detect a wide range of heart conditions, including arrhythmias, ischemia, and hypertrophy. Through the meticulous analysis of the ECG waveform, experts can identify any irregularities in the electrical activity of the heart, accurately determining the underlying cause of a patient's symptoms. The information gleaned from ECG annotation provides vital indicators of heart health, including heart rate, rhythm, and electrical activity. Regular ECG monitoring is invaluable in the management of patients with chronic heart conditions such as atrial fibrillation or heart failure. Here ECG annotation assists experts in identifying changes in heart rhythm or other abnormalities that may indicate a need for treatment adjustment or further diagnostic testing. With regular ECG monitoring and annotation, clinicians can deliver personalized care, tailoring interventions to the unique needs of each patient. How can Machine Learning Support ECG Annotations? Machine learning has significant potential in supporting and automating the analysis of ECG waveforms, providing a powerful tool for clinicians for improving the accuracy and efficiency of ECG interpretation. By utilizing machine learning algorithms, ECG waveforms can be automatically analyzed and annotated, assisting clinicians in detecting and diagnosing heart conditions and abnormalities faster and at higher accuracy. One of the main benefits of machine learning in ECG analysis is the ability to process vast amounts of patient data. By analyzing large datasets, machine learning algorithms can identify patterns and correlations that may be difficult or impossible for humans to detect. This can assist in the identification of complex arrhythmias or other subtle changes in the ECG waveform that may indicate underlying heart conditions. Additionally, machine learning algorithms can help in the detection of abnormalities or changes in the ECG waveform over time, facilitating the early identification of chronic heart conditions. By comparing ECG waveforms from different time points, machine learning algorithms can detect changes in heart rate, rhythm, or other features that may indicate a need for treatment adjustment or further diagnostic testing. Lastly, machine learning models can be trained to recognize patterns in ECG waveforms that may indicate specific heart conditions or abnormalities. For example, an algorithm could be trained to identify patterns that indicate an increased risk of a heart attack or other acute cardiac event. By analyzing ECG waveforms and alerting clinicians to these patterns, it can help in the early identification and treatment of these conditions, potentially saving lives. The three tools we will be reviewing today are: Encord ECG OHIT ECG Viewer WaveformECG Encord ECG Encord is an automated and collaborative annotation platform for medical companies looking at ECG Annotation, DICOM/NIfTI annotation, video annotation, and dataset management. It's the best option for teams that are: Looking for automated, semi-automated or AI-assisted image and video annotation. Annotating all ontologies. Working with other medical modalities such as DICOM and NIfTI. Wanting one place to easily manage annotators, track performance, and create QA/QC workflows. Benefits & Key features: Use-case-centric annotations — from native DICOM & NIfTI annotations for medical imaging to ECG Annotation tool for ECG Waveforms. Allows for point and time interval annotations. Supports the Bioportal Ontology such as PR and QT intervals. Integrated data labeling services. Integrated MLOps workflow for computer vision and machine learning teams. Easy collaboration, annotator management, and QA workflows — to track annotator performance and increase label quality. Robust security functionality — label audit trails, encryption, FDA, CE Compliance, and HIPAA compliance. Advanced Python SDK and API access (+ easy export into JSON and COCO formats). Best for teams who: Are graduating from an in-house solution or open-source tool and need a robust, secure, and collaborative platform to scale their annotation workflows. Haven't found an annotation platform that can actually support their use case as well as they'd like (such as building complex nested ontologies, or rendering ECG waveforms). Team looking to build artificial neural networks for the healthcare industry. AI-focused cardiology start-ups or mature companies looking to expand their machine-learning practices should consider the Encord tool. Pricing: Free trial model, and simple per-user pricing after that. OHIF ECG Viewer The OHIF ECG Viewer provides can be found from Radical Imaging’s Github. The tool provides a streamlined annotation experience and native image rendering with the ability to perform measurements of all relevant ontologies. It is easy to export annotations or create a report for later investigation. The tool does not support any dataset management or collaboration which might be an issue for more sophisticated and mature teams. For a cardiologist just getting started this is a great tool and provides a baseline for comparing to other tools. Benefits & Key features: Leader in open-source software. Renders ECG waveform natively. Easy (& free) to get started labeling images with. Great for manual ECG annotation. Best for: Teams just getting started. Pricing: Free. WaveformECG The WaveformECG tool is a web-based tool for managing and analyzing ECG data. The tool provides a streamlined annotation experience and native image rendering with the ability to perform measurements of all relevant ontologies. It is easy to export annotations or create a report for later investigation. The tool does not support any dataset management or collaboration which might be an issue for more sophisticated and mature teams. So if you're new to the deep learning approach to ECG annotations the WaveformECG tool might be useful but if you’re looking at more advanced artificial neural networks or deep neural networks it might not be the best place. Benefits & Key features: Allows for point and time interval annotations and citations. Supports the Bioportal Ontology and metrics. Annotations are stored with the waveforms, ready for data analysis. Renders ECG waveform natively. Supports scrolling through each ECG waveform. Best for: Researchers and students. Pricing: Free. Conclusion There you have it! The 3 Best ECG annotation Tools for machine learning in 2023. We’re super excited to see the frontier being pushed on ECG waveforms in machine learning and proud to be part of the journey with our customers. If you’re looking into augmenting the ECGs themselves or creating synthetic data get in touch and we can provide you input and help with it!
Mar 14 2023
5 M
Top 10 Free Healthcare Datasets for Computer Vision
As anyone who works with computer vision models knows, the quality of a dataset directly impacts the performance and outcomes from training and production models. If you’re creating a medical imaging model, it’s crucial to get access to accurate and reliable medical imaging datasets. This is especially important for medical imaging start-ups, who may not have the resources to build their own proprietary datasets. Open source medical imaging datasets can give artificial intelligence start-ups the data they need to get their first diagnostic model into production. In this article, we’re sharing information and links on 10 of the best free, open-source datasets for healthcare computer vision models. The Importance of Open-Source Medical Imaging Data Open-source medical imaging datasets are useful because, in most cases, they’re ready to be labeled to create a training dataset. Images have been cleaned and cleansed, rarely contain identifiable patient data, and usually come with a wide range of metadata and other insights that are useful to medical researchers and healthcare providers. Medical images and videos can come from numerous sources, such as microscopy, radiology, CT scans, MRI (magnetic resonance imaging), ultrasound images, X-rays (e.g. chest x-rays), and dozens of others. In most cases, these datasets are focused around a specific medical problem, such as cancer, COVID-19, scar tissue or other healthcare specialisms. Depending on where the images have come from, they can come in a variety of formats. From standard image files (like PNG or JPG) to videos or medical specific file formats such as such as DICOM or NIfTI. Best Practice for Annotating DICOM and NIfTI Files The value of these datasets can’t be understated, especially if you’re training a model for medical image analysis. Depending on the goals of your project, you might be able to use one of these public datasets to complete the project, or you might need access to proprietary medical imaging datasets once a model is trained to a sufficient level of accuracy. Top 10 Free Medical Datasets for Computer Vision Now, let’s take a closer look at the top 10 free, open-source medical imaging datasets for computer vision models. MedPix MedPix is a large-scale, open-source medical imaging dataset containing images from 12,000 patients, covering 9,000 topics and over 59,000 images. The dataset includes metadata from every image, and they’re organized according to where in the body the disease is (organ(s)), pathology, patient demographics, classification, and image captions. Medical professionals can search the database according to symptoms, diagnosis, organs, image modality, description, keywords, and dozens of other choices. MedPix is an open-source medical dataset hosted by The National Library of Medicine (NLM), at the Lister Hill National Centre for Biomedical Communications in Bethesda, MD. Examples of lung images in MedPix The Cancer Imaging Archive (TCIA) Collections Founded in 2011, The Cancer Imaging Archive (TCIA) is a National Institutes of Health (NIH) initiative created to support the cancer research community. The high-quality TCIA open-source dataset includes thousands of “highly curated radiology and histopathology imaging, targeting prioritized research needs and supporting major NIH research programs.” Images include metadata, treatment details, pathologies, and even links to research and expert analysis, whenever available. National COVID-19 Chest Imaging Database (NCCID) Since the outbreak of the COVID-19 pandemic in March 2020, hospitals and health services worldwide have been collecting vast amounts of imaging data on this virus. In the UK, the National Health Service (NHS) has collected a free, open-source database of COVID-19 patient chest images and X-rays. This database includes Chest X-Ray (CXR), Computed Tomography (CT), and Magnetic Resonance Images (MRI) from hospital patients across the UK. Access to this database is approved through a medical board convened to ensure images will be used to improve patient outcomes and contribute to life-saving or life-enhancing treatments. One of the use cases the NHS anticipated is the dataset being used to develop, train, and support computer vision and AI models in the fight against Covid-19. COVID-19 Image Dataset On Kaggle, the open-source imaging dataset platform, you can also access a smaller dataset of Covid-19 patient Chest X-Rays. This dataset includes 137 Covid-19 X-Ray images, plus others to compare against, including Viral Pneumonia and healthy chests/lungs. It contains 317 images, with 3 test directories and 3 training directories. All of the images come from researchers at The University of Montreal. COVID-19 Dataset on Kaggle CT Medical Images Also on Kaggle is an open-source dataset that comes from CT images contained in The Cancer Imaging Archive (TCIA). This dataset is very specific, containing images that come from the middle slice of CT images with the right age, modality, and contrast tags applied. It includes 475 images from 69 different patients. The aim of this dataset “is to identify image textures, statistical patterns, and features correlating strongly with these traits and possibly build simple tools for automatically classifying these images when they have been misclassified.” It will prove useful to those building cancer-related computer vision models and researching this topic. The OASIS Datasets The OASIS (Open Access Series of Imaging Studies) Datasets contain four separate medical imaging datasets of brain scans. It includes thousands of images and patients. These free open-source neuroimaging datasets are designed for medical professionals and medical providers studying a wide variety of brain-related healthcare issues. Making them ideal for training and testing computer vision algorithms that require neuroimaging data and metadata. The OASIS Datasets are supported by National Institutes of Health (NIH) grants, and images come from a number of medical sources, including the Alzheimer’s Association, the James S. McDonnell Foundation, the Mental Illness and Neuroscience Discovery Institute, and the Howard Hughes Medical Institute (HHMI) at Harvard University. Musculoskeletal Radiographs (MURA) MURA (musculoskeletal radiographs) is an open-source musculoskeletal image database that started out as a Stanford University School of Medicine machine learning (ML) competition. Even though the competition is now closed, anyone can request access and download the dataset for the purposes of medical research and training machine learning models. MURA contains 40,561 multi-view radiographic X-Ray images from 14,863 studies and 12,173 patients. Every image was collected from studies and patient scans where the images were labeled either normal or abnormal by board-certified radiologists from Stanford Hospital between 2001 and 2012. re3data re3data (Registry of Research Data Repositories) is a vast open-source library of medical imaging datasets. It’s like a Google or JStor of medical imaging datasets. re3data gives medical researchers and anyone training and testing medical imaging computer vision models access to millions of images and datasets, across dozens of medical specialisms. Funding comes from the German Research Foundation (DFG), with support from other German institutions and libraries, such as the Karlsruhe Institute of Technology (KIT). NIH Deep Lesion Dataset The National Institutes of Health (NIH) Deep Lesion Dataset is an open-source dataset containing thousands of deep lesion images. It was created in 2018, and supported by NIH funding, and anyone can download the images through a simple Box folder. NIH Chest X-Ray Dataset This is a large-scale dataset from NIH, available through Kaggle, and offers a series of over 112,000 chest X-Ray images from more than 30,000 unique patients. It’s the largest known open-source chest X-Ray imaging dataset in the world, with images scoring a 90%+ accuracy, with the majority suitable for weakly-supervised computer vision learning. Every image is supported by Natural Language Processing (NLP) so that labels align with disease classifications supplied by radiological reports. It’s an incredibly valuable resource for anyone conducting machine learning or computer vision modeling that requires chest X-ray images. Medical Image Annotation with Encord For those using a proprietary medical imaging dataset, these aren’t usually labeled images for the purposes of training a model. Equally, for most computer vision models, data scientists will want to label these images according to the goals of their model. In this scenario, which is more common across the medical sector, clinical ops teams will need to get the dataset annotated by trained medical professionals before the dataset is ready to train a computer vision model. Of course, annotators can more effectively deliver their work when they’ve got access to the right tools. Such as an annotation platform like Encord Annotate. Encord streamlines collaboration between medical professionals, machine learning teams, and annotators. With Encord, you can accelerate the process of labeling medical imaging data, produce more accurate datasets and ultimately get your models into production more quickly. Encord Annotate gives annotators access to a range of annotation types (including bounding boxes, human pose estimation, pixel-perfect auto-segmentation and object detection). Encord has developed our medical imaging dataset annotation software in close collaboration with medical professionals and healthcare data science teams, giving you a powerful automated image annotation suite, fully auditable data, and powerful labeling protocols.
Feb 07 2023
6 M
The Top 6 Artificial Intelligence Healthcare Trends of 2024
One of the most exciting things about the end of the year is looking back on the progress that’s been made, and using that progress as a benchmark for making predictions about how far we might come in another year’s time. Every year brings new technologies, new use cases, and exciting AI developments that have real-world impact. When it comes to the use of artificial intelligence in healthcare, 2023 and 2024 saw a steady increase in the number of medical diagnostic models and clinical AI tools making it into production and onto the market. We also saw an increase in the amount and quality of wearable medical devices, a heavier scrutiny of bias in machine learning, and growing privacy concerns about patient data. With many machine learning models now having a positive impact in clinical settings, developments in healthcare AI are set to accelerate rapidly. Here are the six 2024 healthcare AI trends that we’re most excited about. Six 2024 Healthcare AI Trends Healthcare providers will use diagnostic artificial intelligence in fields outside of radiology For the past few years, many AI companies have focused on developing diagnostic models for radiology. Radiology was a logical starting place for companies and researchers looking to build diagnostic models that augment clinicians’ workloads. Because patient screenings are standard practice in radiology, medical professionals collect and have access to a lot of data. I spent much of my career working with breast cancer data. Healthcare providers ask women of a certain age to attend screenings at regular intervals as a preventative measure for breast cancer. At a national level, most countries also have protocols for assessing screenings, so there are standardizations within and between hospitals. Combined, these factors provided machine learning engineers with a good starting point for curating high-quality data, structuring workflows, and building models that support radiologists. Now, machine learning engineers are starting to apply and adapt the lessons they’ve learned from radiology and build models for more complicated medical subsets. The healthcare industry is seeing a lot of AI development in microscopy, which is more challenging than radiology because pathologists have less standardization in the methods they use to count cells. Previously, this lack of standardization made it difficult to collect high-quality training data and develop a labeling protocol for annotators; however, companies such as Paige AI are starting to enter this market with technologies built to augment microscopy practices. Likewise, Rapid AI recently received FDA approval for its stroke detection model, which is an amazing achievement because stroke detection relies on MRI data. MRI does not have standardized units, so machine learning engineers must perform manufacturer specific normalizations when collecting data to train a model. In 2024, we’ll continue to see the use of artificial intelligence expand into different medical specialties. The healthcare industry will focus more on healthcare rather than sick care Rather than use AI only as a means to support a sick patient, healthcare providers will increasingly use AI to help keep patients healthy. At the same time, patients will take more ownership over monitoring their own health. These shifting approaches toward patient care stem from the proliferation of wearable devices, a technology trend that’s been growing over the past few years. Wearables made by third-party companies are enabling users to educate themselves about their own health. Advancements in artificial intelligence are allowing these companies to analyze the data they collect at a much larger scale. As their models improve, the apps and platforms connected to at-home test kits and wearable devices are increasing patients’ ability to reliably monitor their health in real-time before they see a doctor. In a post-pandemic world, more people have become comfortable taking ownership of their health. The use of telehealth and telemedicine, wearables, and at home-testing became increasingly common when stay-at-home orders prevented people from access to on-site healthcare providers. Now, people feel more empowered to address their health concerns because the initial steps of testing and monitoring health no longer necessitate going to a GP, undergoing multiple on-site tests, or obtaining approval from health insurance companies for those tests. At the same time, these companies are continuing to improve their AI’s ability to analyze the data collected, thereby providing better insights from real-time monitoring that can help doctors customize care and treatment plans. With this information, both patients and healthcare professionals can take a more proactive approach to treatment, focusing on staying healthy rather than treating sickness. Researchers will make more datasets public to combat AI bias Many people hope that the development of AI will help eliminate human biases by replacing human subjectivity with data-driven decision-making. However, algorithms and models are still at the mercy of the people who build and train them. Implicit biases and data collection biases can just as easily perpetuate, rather than eliminate, long-standing inequalities in medical care. However, increasing awareness of both the historical bias in medical research and AI bias has resulted in the machine learning community paying increased attention to model bias during training and development. In doing so, ML engineers can help ensure that the machines aren’t biased toward certain demographics because of their ethnicity, age, or gender. In an effort to combat AI bias, there’s going to be an increase in the demand for academia to develop standardized, reproducible systems. More and more journals are requiring that data sets be made public as part of the publication, which means that the research community can verify the results of a system, assess whether the data used to develop the system was balanced, and continue to iterate on and improve the system. Startups will build more demographic-specific healthcare technology While the machine learning community is improving the generalization of models by tackling model bias, AI startups are also tackling these longstanding biases by building demographic-specific models that generalize well for a specific population. Rather than taking a one-size-fits-all approach to designing products, these startups are building technologies capable of generalizing only for a specific population. They have begun to develop personalized health products, segmenting customers by demographics. In doing so, they use AI to better assess and understand patient health based on demographic differences in genes, metabolism, tissue density, and other physiological factors. For instance, some startups have designed healthcare products billed as “for Latinos by Latinos.” Others have created diet-health-focused apps that take into account lifestyle differences that impact the metabolisms of Asian populations. Others have designed skin care monitoring specifically for the LGBTQ+ community. Such products fill an important gap for minority communities who have often suffered from mainstream medical bias in which they receive diagnoses from specialists who have deep expertise in a specific treatment area but not necessarily in diagnosing patients from diverse communities. Differences in biology between populations often mean that a demographic-specific model is needed for patients to obtain the best treatment possible. For example, in Asian populations, women have very dense breasts. Whereas most of the world has a standard for conducting screenings with X-rays, ultrasound works much better when breasts are very dense. When building breast screening models for Asian hospitals, companies should spend more money developing specialized models that have trained on ultrasound images. Advancements in AI are now enabling machine learning engineers to build systems that take demographic differences into account and improve patient outcomes as a result. Rather than rely on technology that caters to the greatest common denominator, new technologies will enable all patients to receive the best possible treatment method, regardless of their background, sex, lifestyle, age, or other factors. The adoption of medical AI will accelerate the democratization of healthcare systems Beyond the at-home monitoring made available by test kits and wearable devices, AI is going to make healthcare more accessible in remote areas as well as developing nations. As the use of healthcare AI becomes more widespread, patients in these areas are becoming increasingly able to get access to preventative screenings via telehealth. For instance, many developing nations and rural areas don’t have enough medical experts to read the scans that they obtain during screenings. A few years ago, the size of medical images hindered the ability to have scans accessed remotely. However, the expansion of computing infrastructure – through the advent of the internet and cloud storage – as well as advances in computer science such as improvements in device memory mean that those medical images that were once too large to send remotely can now be transferred quickly from one location to another, allowing radiologists to look at samples from afar and diagnose patients in areas with limited healthcare resources, improving patient outcomes. As this technology trend continues in the coming years, more people around the world will have access to early screenings, which will increase survival rates in regions that have been historically underserved when it comes to healthcare services. Greater adoption of healthcare AI will increase the tension between data accessibility and data privacy The increase in the adoption of medical AI comes with a Catch-22: we want data to be as easily accessible as possible for expert review at healthcare organizations, and we want to keep it private as possible to protect patients’ identities and other sensitive information. Digital healthcare is becoming the norm, and even the most rigid legacy healthcare providers are undergoing digital transformations or at least using the cloud to store health records. As more patient data is put on servers, we will see an increase in the effort to anonymize data beyond just removing names and identification numbers. Data scientists will begin to think more carefully about anonymization, considering, for instance, whether the combination of information such as a patient’s ethnicity, location, and diagnosis makes the patient identifiable. Standardization in storage is another trend that impacts data privacy. How healthcare organizations store data has a tremendous impact on their security, which is why institutions should no longer focus on producing a solution ideal for their needs but instead focus on storing data in a standardized and secure manner. By providing APIs and software connections that de-identify DICOM images for users, Google Health has pushed the healthcare sector in a positive direction, enabling researchers and ML engineers to use data to build and train AI without exposing a patient’s identity. The trend of combining centralized approaches with the cloud to store data securely is a powerful one. It will continue in 2024, creating positive ripple effects for the AI ecosystem as a whole by empowering researchers to spend less time focused on data privacy tooling and more time focused on creating new methodologies and applications for medical AI. As the healthcare industry continues to embrace artificial intelligence and other new technologies, it can create greater opportunities for patients and clinicians by increasing efficiency, creating pathways for personalized care, and improving access to treatment. At the same time, in the coming years, we’ll see machine learning engineers and healthcare providers put an increasing amount of focus on improving data privacy and reducing AI bias so that as the application of these new technologies benefits all patients. Ready to automate and improve the quality of your medical data? Sign-up for an Encord Free Trial: The Active Learning Platform for Computer Vision, used by the world’s leading computer vision teams. AI-assisted labeling, model training & diagnostics, find & fix dataset errors and biases, all in one collaborative active learning platform, to get to production AI faster. Try Encord for Free Today. Want to stay updated? Follow us on Twitter and LinkedIn for more content on computer vision, training data, and active learning. Join our Discord channel to chat and connect.
Dec 21 2022
7 M
6 Best Open Source DICOM Annotation Tools
Viewing and annotating medical data, images, and videos is a crucial, and frequent, task for many practitioners in the healthcare industry. A starting point for many when evaluating how to go about this task, will be to start with open-source medical imaging annotation tools – these tools are a popular choice in the medical sector and can be a smart way to save money when getting started on an image or video dataset annotation project. In this article, we will cover the handful of key data annotation tools that our team often discusses with leaders from Data Operations and Machine Learning teams (as well as radiologists, clinicians, and the broader annotation community) as they are getting started in their ‘data annotation’ journey. We will mainly cover a handful of tools designed to solve specific DICOM annotation pain points and problems, such as MITK Workbench, ITK-Snap, 3D Slicer, and several others, rather than broader-ranging computer vision annotation tools. As we all know, there are pros and cons to using open-source tools for DICOM annotation projects. When conducting your own evaluation, it’s worth comparing what is on the market with what your own requirements are – based on your specific use cases and forward-looking plans. In this article, we will cover several of the most popular open-source tools for DICOM annotation, including the key use cases, benefits, and downsides of these tools – we will also look ahead to what’s next after getting started with these tools, and considerations that teams make as they go forward in their data annotation journey. What are Open-Source Annotation Tools? Open-source annotation tools are software programs whose source code is freely available for anyone to use. When we think of annotation platforms, what we mean by open-source annotation tools are tools that help teams with the broad annotation and labeling process (including use cases like image classification, image segmentation, data labeling, and object detection). They will be aimed at supporting almost any image or video annotation purpose (unless the license specifically prohibits a certain type of use). Open-source tools are usually built collaboratively, with numerous — sometimes hundreds or thousands — of developers contributing to the source code. Tools are often tested using publicly available medical imaging datasets, and are usually financially supported by a charitable foundation, public/users donations, or one or more tech company sponsors. What are The Advantages of Using Open-Source Annotation Tools for Medical Imaging? The key advantages of open-source annotation tools for medical imaging are: They are free to use They are available for commercial use and can be built upon and customized They typically support community and academic use cases alike In most cases, they support an array of medical image file formats (including DICOM and NIfTI medical image file formats). Now let’s take a closer look at some of the most popular open-source annotation tools on the market. What are Some of the Most Popular Medical Imaging Open-Source Annotation Tools? A wide range of open-source tools support, and were specifically created to, manage annotation projects for medical image datasets. In this article, we will focus on several of the most popular, including MITK Workbench, ITK-Snap, 3D Slicer, HOROS, OsiriX, and the OHIF viewer. MITK Workbench MITK Workbench is a free open-source software for medical image processing, annotation, and segmentation. It’s based on The Medical Imaging Interaction Toolkit (MITK), “open-source software for the development of interactive medical image processing software.” The source code is stored in GitHub and there is MTIK Workbench software that anyone can download and use for Windows, Linux, and Mac (macOS). Here’s more information about how you can use the MTIK Workbench for medical imaging annotation and segmentation projects. MTIK, and the subsequent open-source workbench tool, were originally developed for and by PhD students and researchers in the Division of Medical and Biological Informatics (MBI) of the German Cancer Research Center. ITK-Snap ITK-Snap is another open-source medical imaging annotation tool – unlike some of the others we will cover in this article, it is focused exclusively on one step of the broader data annotation process: the segmentation task. It was created as the result of a long-term collaboration between researchers at PICSL at the University of Pennsylvania, and the Scientific Computing and Imaging Institute (SCI) at the University of Utah, and as a result has a heavy academic following. ITK-Snap’s main offering is manual segmentation tools (eg. brush and paint); it also provides a basic set of semi-automatic tools (mainly the ‘Snake Interaction Mode’) and complementary tools to the segmentation process (mainly the interpolation feature). It is a perfect fit and a very popular option for practitioner teams just starting out, and it supports DICOM and NIfTI medical image file formats. 3D Slicer 3D Slicer was created for the “visualization, processing, segmentation, registration, and analysis of medical, biomedical, and other 3D images and meshes.” It comes with downloadable desktop software, can be used commercially, and enables access to an extensive development platform, and an active network of users. 3D Slicer helps medical imaging operations and data teams implement segmentation on multi-layered medical images, including 2D and 3D segmentation – tools available for segmentation include manual ones (eg. brush, drawing tool, and eraser) as well as a larger set of semi-automatic ones compared to ITK-Snap (eg. thresholding and level tracking). 3D Slicer also allows for basic tasks complementary to segmentation, including basic interpolation between slices, and filters. The main downside that teams often lament when using 3D Slicer for annotating images and files is the functionality set (which is often reported as being quite convoluted) and the steeper learning curve compared to other tools mentioned in this article. For over 10 years, the US National Institutes of Health (NIH) has been a key contributor and supporter, and 3D Slicer has had over 1 million downloads since it was launched. HOROS HOROS is another free open-source medical imaging viewer and annotation software project. It is often a preferred tool when annotating with Apple computers and, not by coincidence, its stated goal is “to develop a fully functional, 64-bit medical image viewer for macOS”. Annotation and ML teams can use the HOROS viewer and annotation tools to annotate medical images and videos, store them in the cloud, and create reports to document an annotation project collaboratively. Several other medical and healthcare-related projects have contributed and are a part of HOROS, including OsiriX, OpenJPEG, OpenGL, VTK, ITK, DCMTK, GDCM, Grok, and Horos Cloud. HOROS works with and is supported by technology partners in the healthcare sector as well as by donations from users. OsiriX Interconnected to, and a supporter of HOROS, OsiriX is another option that many teams opt for when looking for a labeling tool to get started with. Initially fully open-sourced, OsiriX now offers either a ‘Lite’ version, which is available for free as a demo application, or OsiriX MD, which is a commercial version that you can use from $69.99 per month. Similar to most open-source tools, OsiriX Lite is often leveraged by early-stage startups, proof-of-concept (POC) projects, and research work. Based on our many conversations with teams, a few key features are worth digging into when evaluating a tool like OsiriX Lite against others; specifically, its capabilities in regards to 3D rendering, as well as DICOM and collaboration features (which, depending on the use case, teams often cite as being limited). On the other hand, one of the main benefits of OsiriX MD is to make up for the issue of security, which is one of the main challenges with open-source annotation tools (and which we’ll go through in a second more in-depth). OsiriX MD is an FDA-cleared and CE II labeled tool, and this increased level of security and safety makes it a better option for teams annotating professionally (or undergoing or eyeing the FDA approval). OHIF Viewer The OHIF Viewer was developed by and is supported by the Open Health Imaging Foundation (OHIF), at the Massachusetts General Hospital (MGH), and is open-source software under an MIT license. The OHIF Viewer is a tool for creating “custom workflows with user-friendly interfaces. Review cases and report results quickly, zero installation required.” It includes advanced visualization tools, and an easy-to-use annotation suite, and is compliant with DICOMWeb and OpenID Connect standards. OHIF is an open-source annotation tool that comes close to commercial options, as it supports multi-modal image fusion, multiplanar reformatting, and more. It also comes with a cloud-based interface, making it easier to manage collaborative annotation projects. Despite the various benefits of using open-source annotation tools for medical imaging projects, there are several downsides too. What are the Downsides of Using Open-Source Tools for DICOM Annotation? As with any DICOM annotation tool, the ultimate goal of the labeling process is to provide high-quality data to the next step in the process; annotation is simply one stage, albeit a crucial one – in the case of building machine learning applications, once the datasets are labeled and annotated, you will be putting them to test by feeding them into a model (often a broader machine learning (ML) or computer vision (CV) model), then training and iterating on the training, until you are ready to launch a production-ready model and finally solve the critical objective you set out to achieve. Similarly, a hospitality software development company can apply such structured data processing and iterative enhancements to optimize guest experiences and operational efficiencies in travel and hospitality industries. Open-source tools are often a great starting point when going from 0 to 1 with the annotation of medical imaging and video datasets, but, as we all know, they are inherently limited in their ability to achieve some of the more critical and powerful outcomes that teams face as they progress through the journey. Throughout conversations with thousands of practitioners and leaders across the Medical AI community, we recurrently hear about numerous key downsides of open-source annotation software – these are often useful to consider ahead of time, in order for you to effectively plan out what your next steps in your data journey will be. Below, we’ll dig deeper into the three main ones – scale, security, and collaboration – which you can also read more about in our blog here. These are: Scaling annotation activity with open-source tools is a big challenge. Open-source tools often come with a basic set of features (and hence are a perfect fit for many teams as they get started), but lack the wider set of needs that companies start to require at scale. For example, whereas it’s often a sensible setup for teams to collaborate on the annotation process over an open-source tool and back-and-forth emails, as the number of annotators and volume of data increases, in-app and real-time collaboration capabilities like tagging become key. Teams we work with often start to feel this pain point as they start to scale, and that’s when a more solid commercial option can help save resources, speed up the process, and also avoid errors and inaccuracy. Open-source tools inherently fall behind on security requirements. In most cases, open-source tools don’t benefit from the rigorous compliance standards of commercial tools, and by nature don’t include features like auditing, which more established companies require in order to achieve milestones like FDA approval. Many don’t have auditable data trails that can be monitored, tracked, and reported on, making it more difficult to achieve compliance with the FDA and HIPAA, or GDPR and CE certification in Europe. Free doesn’t always mean cost-effective. As the volume of annotations and annotators increases, the hidden costs of managing the process start to grow exponentially. At this stage, project leaders often find themselves needing to start being able to quantify, manage and measure their process and work; they need to be able to monitor and gain clear insight into the process and streamline operations on multiple spectrums. This is where limitations of open-source tools start to heavily affect their ability to achieve their objectives; two examples we hear often at this stage are frustrations around needing to write off a large percentage of time on non-value add tasks, as well as not being able to track how each annotator is performing, leading to poor process and output. After getting started with open-source annotation software for their medical imaging dataset annotation projects, most teams tend to graduate to a commercial, proprietary tool that’s purpose-built to help take their project from 1 onwards. Tools like these are often easy to collaborate across, have best-practice security standards, and allow data operations and machine learning teams to scale their project cost-effectively (as well as help attain milestones like FDA clearance). Encord is the leading annotation platform in medical AI, with a platform built to easily manage the annotation process, while allowing for the most complex of annotation tasks. At Encord, we have developed our medical imaging dataset annotation software in collaboration with data operations, machine learning, and AI leaders across the medical industry – this has enabled us to build a powerful, automated DICOM annotation suite, allowing for fully auditable data, and powerful labeling protocols. A few of the successes achieved by the medical teams we work with: Stanford Medicine cut experiment duration time from 21 to 4 days while processing 3x the number of images in 1 platform rather than 3 King’s College London achieved a 6.4x average increase in labeling efficiency for GI videos, automating 97% of the labels and allowing their annotators to spend time on value-add tasks Memorial Sloan Kettering Cancer Center built 1000, 100% auditable custom label configurations for its pulmonary thrombosis projects Experience Encord in action. Sign-up for an Encord Free Trial: The Active Learning Platform for Computer Vision, used by the world’s leading computer vision teams. AI-assisted labeling, model training & diagnostics, find & fix dataset errors and biases, all in one collaborative active learning platform, to get to production AI faster. Try Encord for Free Today. Want to stay updated? Follow us on Twitter and LinkedIn for more content on computer vision, training data, and active learning. Join our Discord channel to chat and connect.
Dec 19 2022
10 M
Future for Computer Vision in Healthcare
Computer Vision (CV) models and artificial intelligence algorithms (AI) are already playing an important role in numerous healthcare use cases across the medical sector and profession. The application of computer vision, automation, and other algorithmically-generated models enables medical professionals to more effectively diagnose illnesses, viruses, and tumors, and have numerous other use cases that directly impact patient care. A lot of work and resources go into training, deploying, and building computer vision models in healthcare to facilitate the application of computer vision in the medical sector. Including automation, that always plays a valuable role in annotation and labeling projects. To start with, you need the right imaging or video-based datasets, and these need to be annotated and labeled. After that, your machine learning (ML) or data science teams need to train one or more artificial intelligence (AI), CV, or deep learning model until a high accuracy score is achieved, any bias is reduced, and it’s generating the results you need. Once a CV model is in production, it will generate iterative feedback data from it being used on real-world annotated medical imaging or video-based datasets. This iterative feedback loop will help you train improved and enhanced versions of the model. In this article, we take a closer look at how computer vision models are used in healthcare, the benefits of using CV in healthcare; alongside several examples of use cases Encord has been involved with, and what we expect for the future of computer vision in healthcare. How is Computer Vision Used in Healthcare? Computer vision and deep learning have numerous use cases in the healthcare industry, from medical research through to patient care and surgeries. Every CV and artificial intelligence model starts with datasets of medical images or videos from a wide range of sources, such as radiology, gastroenterology, histology, MRI machines, ultrasounds, and X-rays. Wherever the images come from, datasets are usually in DICOM or NIfTI formats, and for these, your annotation team needs an annotation platform equipped to handle native medical imaging formats. Training and deploying a production-ready CV model faster ensures medical professionals and organizations get more effective, efficient, and useful results from CV and deep learning projects. The outcomes of these projects directly impact patients’ treatment plans, medical research, and clinical drug trials. CV models are incredibly useful during the diagnosis process, patient care, and even during clinical operations. The role of IT services for healthcare is instrumental in ensuring these technologies are seamlessly integrated into existing systems, enhancing both the speed and accuracy of diagnoses and treatments. What are The Benefits of Computer Vision in Healthcare? Healthcare industry professionals and organizations are handling huge volumes of data. A lot of this data is in the form of images and videos of patients, scans during the diagnosis stage, and the treatment they’re receiving. These images and videos provide valuable and life-changing insights, pieces of information, and new fields of study that would otherwise be overlooked by the human eye. CV, ML, and AI-based models are useful in numerous ways, as we will soon outline, including robotic surgeries. What Types of Computer Vision Applications Are Currently in Use in Healthcare? Healthcare already has hundreds of use cases and applications for computer vision models. In medical abnormality detection, startups are deploying computer vision models to empower secondary insights from patient medical imaging scans. For example, patients might have had scans taken using an MRI machine with the aim of detecting a particular illness. A scan comes back clear, at least for the illness a doctor was looking for. But what if there’s something else going on? With medical image annotations and a production-ready CV model, these images can be processed again to search for other health issues that have been missed and overlooked on the first scan. Using computer topography, and powerful computer vision models, medical teams can benefit from a “second pair of eyes”; in this case, a highly-trained CV model. With this, it’s more time and cost-effective when attempting to detect medical abnormalities in patients, producing more accurate diagnoses and helping to save more lives and deliver better treatment plans. In gastrointestinal care (GI), computer vision models and ML are now being used in pre-trial screenings of patients for clinical trials and other forms of gastrointestinal care (GI). GI healthcare providers can use computer vision models to analyze patient and clinical trial imaging datasets to improve the accuracy of the diagnosis process. Abnormalities are easier to highlight when a CV solution is trained to analyze thousands of images faster, more accurately, and in much greater detail than a human team ever could. Neurovascular and vascular clinical teams are also making extensive use of computer vision models and AI-driven volumetric measurements. For the human eye, even when using powerful microscopes, detecting life-threatening neurovascular and vascular conditions is a serious challenge. Medical teams need faster, more accurate, and proven approaches to detect, speed up, and improve treatment plans for strokes, aneurysms, and pulmonary embolisms. Using AI and computer vision models, healthcare companies can empower physicians to make faster, more accurate diagnostic, treatment, and transfer decisions, improving the patient journey every step of the way. Ultimately, this is one of many examples in the healthcare sector where computer vision technology and trained models using annotated datasets are saving lives. In digital cell morphology, some startups are using a revolutionary technique that is deploying computer vision models to automate the analysis of tens of thousands of cells faster than any human ever could. This is already being used in hematology to analyze and count the cells from smear tests and provide better care plans for patients. Smear and other hematology tests usually only count a tiny number of cells visible under an electronic microscope. No matter how skilled the person who's looking at these cells or how powerful the microscope is, no one can come close to what a CV model can do when analyzing every single cell at a huge volume. CV models, in this way, empower doctors to detect and diagnose health problems far earlier. Patients can access the care they need faster, resulting in more life-saving treatment. In surgical robotics, computer vision models are being used to improve the outcomes of robotic surgery. Robotic tools are more widely deployed in the healthcare sector. It’s a proven and safe technology, and they’re useful for reducing the invasiveness of surgery, minimizing risk and recovery time, and moving in ways that human surgeons can’t. At the same time, robotic surgeons still need to mimic humans as closely as possible, and that’s where computer vision models play an important role. Computer vision models are useful for training robotic surgical tools and analyzing thousands of videos from endoscopic surgeries. CV and AI-based models are, therefore part of a robotic surgical tools training system, helping these devices become more precise and accurate, improving patient outcomes from surgery. Now let’s consider what other future advances and innovations healthcare can expect and drive forward from computer vision models and providers in this field. What's The Future For Computer Vision and Healthcare? Future applications and use cases for computer vision in healthcare are only limited by human imagination and resources. As we are working closely with medical professionals, healthcare data scientists, and numerous companies in this sector, we can already see progress in the following areas: Deploying computer vision models for augmented reality (AR) treatments and consultations in the consumer market. Integrating ehr software development within computer vision applications in healthcare could enhance the capabilities of AR consultations, offering more precise and personalized medical advice based on real-time data analysis. Imagine having a remote consultation with a doctor using augmented reality. It sounds like something out of science fiction, but it could happen sooner than we might imagine. Using AR and CV, remote consultations could be just as useful as in-person appointments, if not more so, because doctors could be empowered with CV models to make faster and more accurate diagnoses of patients. Additionally, implementing hospitality iot solutions can enhance the efficiency of these models, offering more precise management of healthcare resources and improving patient care coordination. In hospitals and treatment centers too, doctors and medical staff could soon be wearing AR/AI-powered medical glasses. Bringing all of the power of AI and CV-based models into the patient experience. Enhanced accuracy would reduce mistakes and improve patient access to the right treatment plans, saving lives, time, and money. We are also seeing advances in the use of AI-based models in microscopy and more complicated medical subsets, going beyond many of the original radiology use cases. Healthcare providers are also looking at ways to use AI, when combined with data from wearable devices and at-home test kits, to keep patients healthier. Preventative actions rather than treating patients only after they get sick would make a huge difference to the entire sector and the cost of maintaining healthcare for an increasingly aging population. At the same time, we are seeing a shift towards making more medical datasets public to reduce AI bias in the model training and development stage. Outside of academia, a new generation of startups is building healthcare solutions for specific demographic and ethnic groups, overcoming unintentional bias in a more direct way. Computer vision models could also be used to segment patient groups based on treatment needs. The segmentation and personalization of healthcare and medicines is a huge growth area for the healthcare sector. Not only would it improve patient outcomes, but it could be valuable for generating more revenue for healthcare providers. In the hands of the medical profession, computer vision models are already making significant positive impacts on the patient experience. It’s exciting for the entire profession and sector to see what computer vision will do next and what future use cases we can expect over the next decade. Encord has developed our medical imaging dataset annotation software in close collaboration with medical professionals and healthcare data scientists, giving you a powerful automated image annotation suite, fully auditable data, and powerful labeling protocols. Experience Encord in action. Dramatically reduce manual video annotation tasks, generating massive savings and efficiencies. Try it for Free Today.
Dec 12 2022
7 M
Guide to Experiments for Medical Imaging in Machine Learning
In the scientific, and especially data science community, the word “experiment” means to test a hypothesis until empirical data agrees or conflicts with an experiment's desired outcomes. Machine learning medical imaging experiments need to be rigorous. In medical imaging machine learning experiments, this involves testing dozens of datasets using machine learning models to achieve higher levels of accuracy, until the artificial intelligence model can be put into production. Running medical imaging dataset experiment is an essential part of building a stable, robust, and reliable computer vision model (such as a tool for use in oncology). The outcomes of these experiments are even more important when building models for healthcare; you have to be even more confident in the accuracy of the results, as this could influence a life-or-death decision for patients. However, running multiple experiments can quickly become a massive challenge. Managing the models, datasets, annotators, and experiment results is a full-time job. An inefficient workflow for managing these experiments can make these problems much worse. In this article, we will look at how to increase the efficiency and effectiveness of your medical imaging dataset experiments to create state-of-the-art models. Why Do You Need to Run Experiments For Deep Learning Models? Running experiments for machine learning and computer vision models is crucial to the process of creating a viable and accurate production model. At the experimental stage, you need to figure out which approach will work and which won’t. Once you’ve got a working model and a source of ground truth (dataset), then you can scale and replicate this approach during the production stage to achieve the project outcomes and objectives. Reaching this goal means going through dozens of experiments. It’s a time-consuming task, and running experiments is a full-time job. You need a team of annotators, a large volume of high-quality data (medical imaging datasets of tumors or lesions, for example), and the right tools to make this work easier. At every stage, the results should gradually improve, until you’ve got a viable and accurate model and process. Before starting any experiment cycle, it’s important to know the key parameters you want to track. For example, hyperparameters, model architectures, accuracy scores, loss measures, weighting, bias, gradients, dependencies, and other model metrics. Once the experiment outcomes, goals, and metrics are clear, then you can start running machine learning imaging dataset experiments. Why is it More Important to Create Experiments For Medical Imaging Datasets? In the healthcare sector, medical image machine learning and computer vision models play an integral role in patient diagnosis, our understanding of diseases, and numerous other medical fields. Medical images come from numerous sources (including Magnetic Resonance Imaging (MRI), X-rays, and Computed Tomography (CT images)) for a range of conditions, such as Alzheimer's disease, lung cancer, or breast cancer. Unlike other datasets in other sectors, medical images come in more complex formats, including DICOM and NIfTI. These widely-used medical image file formats have several layers of data, such as patient information, connections to other databases, and appointment details. Even when patient training data is anonymized, the layers and formats make medical imaging datasets more detailed and involved than you will find in other sectors. An example of DICOM annotation in Encord Alongside these complications, project leaders have to weigh the necessity of gaining regulatory approval for working models, clear audit trails, and enhanced data security. Remember, the ultimate outcome of any medical machine learning model could directly impact patient healthcare treatment outcomes. Accuracy and keeping bias as low as possible are essential. For example, a slight inaccuracy when analyzing music preference data isn’t going to hurt anyone. Whereas, with medical imaging datasets, the accuracy and results can have serious, life-changing outcomes for patients worldwide. Hence the need to test as much data as possible. Not only is this important to ensure a robust model for primary use cases; but you also need to assess datasets and models against a wider range of edge and corner cases. What Does The Ideal Experiment Workflow Look Like? Machine learning medical imaging dataset experiments have tried and tested workflows that improve efficiency. Before starting ML-based experiments, you need to ensure you’ve got the right components to start running experiments. Components of a machine learning experiment workflow need to include: Dataset(s): in this case, medical imaging datasets from the right medical fields, specialisms (such as radiology), image sources, and file formats; A hypothesis with a range of hyperparameters and variables to test; Project outcomes and goals, including the relevant benchmarking and accuracy targets; Experiment iteration cycle frameworks, e.g. the number of experiments you’ve got the resources and time to run; Other relevant experiment components, such as the metadata needed and model architecture. Once these components are ready, you can start running machine-learning experiments on medical imaging datasets. An ideal workflow should involve the following: Outline the experiment hypothesis, parameters, and variables; Source the data (either open-source datasets or in-house data); Ensure the right annotations and labels are applied to a series of segments within these datasets. Not the entire dataset, because at this stage you simply need enough images to run small-scale experiments. You can use automated image annotation tools and software, such as Encord, to accelerate this phase in the project. Once the annotated datasets are ready, and the machine learning or computer vision algorithms in place to run these experiments, they can begin. Each experiment could take one or two weeks. Running a whole series of experiments and iterating on the results, reducing bias and increasing accuracy could take anything from 1 to 6 months before the experiment outcomes and datasets are ready to go into production. Experiment results determine when it’s possible to put a machine-learning model into production. Ongoing monitoring of these experiments, the outcomes, and audit trails are equally crucial. Especially in the healthcare sector. Project leaders need a 360 overview (with a few clicks of a mouse) of the entire experiment lifecycle and every iteration, right down to the granular level, including detailed oversight of the work of the annotation teams. Once the ideal outcome has been achieved, you need to ensure the configuration of the machine learning model that produced that outcome is the one used for the production model. Make sure the annotations and labels used in the most successful iteration of the experiment are carried over and replicated across the entire medical imaging datasets. How Does Collecting More Data Improve Experiment Outcomes? With machine learning experiments, or any computer vision or AI-based experiments, the more data you have the better. Especially when it comes to medical imaging ML model experiments for most use cases. However, it’s important to remember that quality and diversity are as important as the volume of data. Medical imaging data should include the most relevant clinical practice data possible for the experiments. Such as having enough images with positive and negative cases, different ethnic groups, and either including or excluding the relevant edge cases; e.g. patients who have or haven’t received treatment. Example of a DICOM image ontology in Encord Getting a high volume of data is crucial. But the quality and diversity of the datasets you’ve got available matter too. As does the quality and accuracy of the annotations and labels applied and reviewed by skilled radiologists and clinicians. What Happens If You Get The Wrong Machine Learning Experiments Outcomes? During machine learning experiments, most go wrong or fail in some way. That’s not unusual. As most data scientists and clinical ops managers know, this is normal. You might have 100 experiments running and only 10 to 15 produce outcomes close to what you need. A failure isn’t a setback. In fact, following the scientific methodology, failures simply get you closer to successful outcomes that validate a hypothesis. Even if a hypothesis is invalidated, that’s a positive too, as it will help you refocus efforts on the right perimeters and valuators to test a new theory. Or in some cases, a negative outcome could be the goal behind an ML-based experiment. So, it’s useful to never see failure as a negative but to learn from the experiments that fail and move forward with the learnings from those that have achieved the desired outcomes. Only this way can you successfully put a machine learning model into production. How Can The Right Experiment Workflow Improve Experiment Efficiency? With the right tools, processes, and systems, project and clinical ops managers can create efficient medical imaging machine learning project workflows. Open-source tools can be a great starting point but can make it harder to develop the scope of your projects. For example, open-source tools can reduce efficiency, make scaling difficult, weaken data security, and monitoring or audit annotators’ work is almost impossible. Instead, medical image dataset, annotation, and machine-learning teams benefit from using proprietary automated image annotation tools to improve experiment efficiency. Encord has developed our medical imaging dataset annotation software in close collaboration with medical professionals and healthcare data scientists, giving you a powerful automated image annotation suite, fully auditable data, and powerful labeling protocols. Ready to automate and improve the quality of your medical data annotations? Sign-up for an Encord Free Trial: The Active Learning Platform for Computer Vision, used by the world’s leading computer vision teams. AI-assisted labeling, model training & diagnostics, find & fix dataset errors and biases, all in one collaborative active learning platform, to get to production AI faster. Try Encord for Free Today. Want to stay updated? Follow us on Twitter and LinkedIn for more content on computer vision, training data, and active learning.
Nov 25 2022
5 M
The Full Guide to Open Source Annotation Tools for Medical Imaging
Open-source software and tools are widely available for computer vision and medical imaging machine-learning projects. In some cases, it can be advantageous to use open-source tools when testing and training a machine-learning model on medical imaging datasets. You can save money, and several tools — such as 3DSlicer and ITK-Snap — are designed specifically for medical image annotation and training ML models on healthcare datasets. In the healthcare sector, the quality of a dataset and the efficiency of the tools you use to annotate and train machine learning models is crucial. It could be a matter of life and death for patients, as medical specialists and doctors need the most accurate outputs from computer vision and ML models to diagnose patients. As clinical and data operations teams know, the complexity, formats, and layers of data within medical images are complex and detailed. You need the right tools for the job. Using the wrong tool, such as an open-source annotation application could negatively impact model development. In this article, we cover the main open-source tools for medical image annotation, the use cases for these tools, and how they’re holding your annotation projects back. We also outline what you need to look for in an annotation tool that will help you overcome these challenges, including the features that will give you the results you need. What Are the Main Open-source Tools for Medical Image Annotation? There are numerous open-source tools on the market that support medical image datasets, including 3DSlicer, ITK-Snap, MITK Workbench, RIL-Contour, Sefexa, and several others. For this article, we will focus on two of the most popular open-source medical image annotation tools: 3DSlicer and ITKSnap. Although, the ways open-source tools can hold medical image annotation projects back aren’t limited to 3DSlicer and ITK-Snap. What is 3D Slicer? 3D Slicer is a free open-source image computing platform. It was designed for the “visualization, processing, segmentation, registration, and analysis of medical, biomedical, and other 3D images and meshes.” 3D Slicer comes with downloadable desktop software, access to a development platform, and an active community of users and developers working on similar problems. It’s designed to work with some of the most popular and widely-used medical imaging formats, including DICOM and NIfTI. 3D Slicer supports 2D, 3D, and 4D segmentations, AI-based segmentation, tools for ground truth training data generation, and extensions for Deep Learning, Tensorflow, and MONAI compatibility. It also comes with surgical guidance and planning tools, and a whole load more. The US National Institutes of Health (NIH) has been a key contributor and supporter over the last 10 years, and 3D Slicer has had over 1 million downloads since it was first launched. Despite extensive support and an active community, the user interface is somewhat complex and takes time to learn. What is ITK-Snap? It supports DICOM and NIfTI medical image file formats, with the core functionalities focusing on “semi-automatic segmentation using active contour methods, as well as manual delineation and image navigation.” Improving medical image segmentation during the annotation of datasets is the main reason this tool was created, aiming to achieve a better, more intuitive user interface than other open-source software on the market. ITK-Snap is the result of a decade-long collaboration between researchers at PICSL at the University of Pennsylvania, and the Scientific Computing and Imaging Institute (SCI) at the University of Utah. What Are The Main Use Cases for Open-source Medical Image Annotation Tools? Open-source annotation tools are used in numerous ways when annotators are working on medical image datasets. Images and videos come from dozens of sources (whether the datasets are open-source or in-house), such as MRI machines, X-rays and CT scans. Particular use cases depend on the objectives and desired outcomes — the problem(s) that needs solving — of a machine-learning or computer vision-based medical imaging project. A consistent end goal is to solve a medical problem. Such as improving the percentage of patients accurately diagnosed, or using ML and AI-based models to more effectively identify diseases, illnesses, and tumors. The more data you’ve got the better. It improves the chances of accurate outcomes when an ML model has more data to work with. However, high levels of accuracy are only possible if annotation and labeling are implemented accurately and efficiently, and for that, you need the right tools. Open-source tools aren’t bad tools, as such. The ones we’ve mentioned in this article were created with medical imaging datasets and medical image formats and use cases specifically in mind. Many were created with the help of medical professionals, organizations, and data scientists. However, there are several limitations, and there is a risk these limitations could hold annotation and computer vision projects back.. 3 Ways Open-source Tools Are Holding Your Annotation Projects Back 1. Unable to effectively scale your annotation activity One of the main challenges is scaling annotation activity. When you use cloud-based tools and platforms, an annotation team can work collaboratively in real-time across several time zones, and work directly with data and medical ops teams in another country. However, the tools mentioned in this article are desktop-based. It’s a seriously limiting feature when annotation teams need to work together on large imaging datasets, and teams need to receive quick feedback from medical imaging specialists when training ML models with new datasets. If an annotation team is using open-source software, the only way to share images and receive feedback is through email and cloud-storage platforms, such as Dropbox. This can make it particularly difficult to scale annotation projects, especially when you’ve got large imaging datasets to work through and strict data security compliance requirements to follow. 2. Weak data security makes FDA and CE certification harder Data security is absolutely crucial in the healthcare sector. In the US, medical data compliance is governed by the FDA and HIPAA. In the UK and Europe, CE certification and GDPR are always front-of-mind for any teams handling data, whether or not medical images have been stripped of identifiable patient information. When you are using open-source tools, there’s no audit trail, and this could prove a costly mistake in the healthcare sector. Without an audit trail and timestamps, there’s no way to show who’s worked on which image and who made edits, annotations, labels, or any changes. It’s much harder to adhere to medical data security regulations when medical imaging data isn’t fully auditable. It’s also easier for annotators to download copies of images onto personal computers and devices, causing a security risk, especially if images still have identifiable patient information on them. 3. You can’t monitor your annotators Open-source annotation tools are free, but that doesn’t mean they’re cost-effective. In most cases, free tools aren’t as efficient as premium options on the market. Because open-source tools aren’t cloud-based, collaboration is more difficult and annotation, data ops, and medical project managers have no way of monitoring the progress of annotators. Unlike premium solutions, these tools don’t come with performance and analytical dashboards. If a manager can’t oversee the work of annotators effectively then projects are more difficult to manage and the efficiency of annotations will be negatively impacted. As a result, annotation projects will take longer, and if re-annotation is needed, or accuracy is low, then it will take even more time to generate accurate training data. What Should You Look For in a Medical Annotation Tool to Overcome These Challenges? Considering the challenges associated with open-source medical image annotation tools, it’s understandable that many project leaders and medical ops managers look for premium solutions. To achieve the results you need from medical image annotation projects, you need a tool with the following features: An easy-to-use, cloud-based, collaborative interface It might sound basic, but it’s so important that the interface annotators are using is intuitive and collaborative. You need to know that annotators in different countries, or on different shifts can work together on the same medical imaging datasets, and those datasets are accessible to data and healthcare ops teams in another country, as required. A cloud-based interface is the most effective way to ensure this. Designed for and by medical imaging professionals and healthcare data scientists Similar to the open-source tools, you need annotation software that’s been designed with the support and close collaboration of medical image and data professionals. Medical image annotation is more complex and involved than in other sectors. With the right tool, such as Encord, you can be confident it’s been designed with your needs and project goals in mind. Native DICOM and NIfTI file support It’s essential that the right tool comes with native DICOM and NIfTI file support. You need one that includes features that are specifically designed to annotate and label DICOM and other medical image files and formats. A medical image annotation tool should allow you to see images in 2D orthogonal planes (coronal, sagittal, axial), viewing medical metadata and make window width (WW) and window level (WL) adjustments. 3D and 2D annotation, and powerful automation features Automation features can save annotation teams a massive amount of time. One of the most powerful automation features is interpolation that can match pixel data, and allows annotators to draw the interpolation labels in arbitrary directions. Project dashboard and quality control Having a project dashboard and built-in quality control features is essential for the smooth running of any medical image annotation project. As a project manager, this is something open-source tools can’t provide, and this can make the difference between success or a costly failure. Audit trails, and SOC 2 and HIPAA compliance Having easily-accessible audit trails is mission-critical for medical and data ops teams and managers. Achieving FDA, CE, SOC 2 (Systems and Organizational Control 2) or HIPAA (Health Insurance Portability and Accountability Act) compliance is impossible without an auditable data trail. It’s an essential feature to have in any medical image annotation tool. Encord has developed our medical imaging dataset annotation software in close collaboration with medical professionals and healthcare data scientists, giving you a powerful automated image annotation suite, fully auditable data, and powerful labeling protocols. Experience Encord in action. Dramatically reduce manual video annotation tasks, generating massive savings and efficiencies. Try it for Free Today.
Nov 15 2022
7 M
7 Ways to Improve Medical Imaging Dataset
The quality of a medical imaging dataset — as is the case for imaging datasets in any sector — directly impacts the performance of a machine learning model. In the healthcare sector, this is even more important, where the quality of large-scale medical imaging datasets for diagnostic and medical AI (artificial intelligence) or deep learning models, could be a matter of life and death for patients. As clinical operations teams know, the complexity, formats, and layers of information are greater and more involved than non-medical images and videos. Hence the need for artificial intelligence, machine learning (ML), and deep learning algorithms to understand, interpret, and learn from annotated medical imaging datasets. In this article, we will outline the challenges of creating training datasets from medical images and videos (especially radiology modalities), and share best practice advice for creating the highest-quality training datasets. What is a Medical Imaging Dataset? A medical imaging dataset can include a wide range of medical images or videos. Medical images and videos come from numerous sources, including microscopy, radiology, CT scans, MRI (magnetic resonance imaging), ultrasound images, X-rays (e.g. chest X-rays), and several others. Medical images also come in several different formats, such as DICOM, NIfTI, and PACS. For more information on medical imaging dataset file formats: Best Practice for Annotating DICOM and NIfTI Files What's the difference between DICOM and NIfTI Files? Medical image analysis is a complex field. It involves taking training data and applying ML, artificial intelligence, or deep learning algorithms to understand the content and context of images, videos, and health information to spot patterns and contribute to healthcare providers’ understanding of diseases and health conditions. Images and videos from magnetic resonance imaging (MRI) machines and radiologists are some of the most common sources of medical imaging data. It all starts with creating accurate training data from large-scale medical imaging datasets, and for that, you need a large enough sample size. ML model performance correlates directly to the quality and statistically relevant quantity of annotated images or videos an algorithm is trained on. How Are Medical Imaging Datasets Used in Machine Learning? A medical imaging dataset is created, annotated, labeled, and fed into machine learning (ML) models and other AI-based algorithms to help medical professionals solve problems. The end goal is to solve medical problems, using datasets and ML models to help clinical operations teams, nurses, doctors, and other medical specialists to make more accurate diagnoses of medical illnesses. To achieve that end goal, it’s often useful to have more than one dataset to train an ML model and a large enough sample size. For example, a dataset of patients who potentially have health problems and illnesses, such as cancer, and a healthy set, without any illnesses. ML and AI-based models are more effective when they can be trained to identify diseases, illnesses, and tumors. It’s especially useful when annotating and labeling large-scale medical imaging datasets, to have images that come with metadata as well as clinical reports. The more information you can feed into an ML model, the more accurately it can solve problems. Of course, this means that medical imaging datasets are data-intensive, and ML models are data-hungry. Why is it Important to Have High-Quality Medical Imaging Datasets for Machine Learning? Annotation and labeling work takes time, and there’s pressure on clinical operations teams to source the highest quality datasets possible. Quality control is integral to this process, especially when project outcome and model accuracy is so important High-quality data should ideally come from multiple devices and platforms, covering images or videos of as many ethnic groups as possible, to reduce the risk of bias. Datasets should include images or videos of healthy and unhealthy patients. Quality directly impacts machine learning model outcomes. So, the more accurate and widespread a range of images, and annotations applied, the more likely a model will train to a level of effectiveness and efficiency to make the project a worthwhile investment. Annotators can create more accurate training data when they have the right tools, such as an AI-based tool that helps leading medical institutions and companies address some of the hardest challenges in computer vision for healthcare. Clinical operations teams need a platform that streamlines collaboration between annotation teams, medical professionals, and machine learning engineers, such as Encord. What are the Consequences if a ‘Bad’ Dataset is Fed Into a Machine Learning Model? Feeding a poor quality, poorly cleaned (cleansing the raw data is integral to this process), and inaccurately labeled and annotated dataset into a machine learning model is a waste of time. It will negatively impact a model’s outcomes and outputs, potentially rendering the entire project worthless. Forcing clinical operations teams to either start again or re-do large parts of the project, costs more time and money, especially when handling large datasets. The quality of a large dataset makes a huge difference. A poor-quality dataset could cause a model to fail, not to learn anything from the data because there’s insufficient viable material it can learn from. Or if a model does train on an insufficiently diverse medical dataset it will produce a biased outcome. A model could be biased in numerous ways. It could be biased for or against men or women. Or biased for or against certain ethnic groups. A model could also inaccurately identify sick people as healthy, and healthy people as being sick. Hence the importance of a statistically large enough sample size within a dataset. ‘Bad’ data comes in many forms. It’s the role of annotation and labeling teams and providers to ensure clinical operations and ML teams have the highest quality data possible, with accurate annotations and labels, and strict quality control. What are the Common Problems with Medical Imaging Datasets? Common problems include imaging datasets that aren’t readable to machine learning models. Hospitals sell large datasets for medical imaging research and ML-based projects. When this happens, images could be delivered without the diversity a model requires or stripped of vital clinical metadata, such as biopsy reports. Or hospitals will simply sell datasets in large quantities, without having the technical capability of filtering for the right images and videos. However, an equally common problem is that medical data still includes identifiable personal patient information, such as names, insurance details, or addresses. Due to healthcare regulatory requirements and data protection laws (e.g. the FDA and European CE regulations), every image annotation project needs to be especially careful that datasets are cleansed of anything that could identify patients and breach confidentiality. Other problems include using data from older models of medical devices, resulting in lower-resolution images and videos. What are the Common Challenges in Creating a Medical Imaging Dataset? Creating and starting to annotate and label a medical image dataset involves overcoming some of these common challenges: Where are you getting the data from? Will it come from in-house sources (e.g. a hospital or medical provider using its own imaging datasets), from public sources, or will you buy it from hospitals? Who is going to annotate and label the dataset; e.g. in-house or external providers? Remember, a radiologist's or other specialist’s time is far too valuable. You need a reliable provider for this work. Where and how will you store the medical imaging data? How will the raw data be extracted for annotation and labeling? How will the medical imaging data be transported? Usually. datasets contain hundreds of thousands of images, videos, and medical metadata. It’s not as easy as simply storing it in cloud-based servers. This isn’t something you can attach as a Zip folder and send in an email. Medical data needs high levels of encryption, and in some cases, armed guards. Are you getting all of the data you need to train a model? Remember that you need a wide enough range of images to avoid inaccuracies and bias. How will you validate this and sift through vast quantities of images, videos, and medical imaging data? How long can you keep the data? Regulators may limit this to three years. If data is being annotated and labeled in a developing country; what are their data protection laws, and can you legally do this, and how can you ensure the data is shipped and stored securely? How can you effectively implement quality control throughout the annotation process to ensure the model receives the highest quality and most accurate data possible? All of these questions need to be considered and answered before starting a medical image dataset annotation project. And only once images or videos have been annotated and labeled can you start training a machine-learning model to solve the particular problems and challenges of the project. Now here are 7 ways clinical operations teams can improve the quality and accuracy of medical imaging datasets. Key Takeaways: 7 Ways Clinical Operations Teams Can Improve Medical Imaging Datasets #1: Getting the right data and getting enough data Before embarking on any computer vision project, you need to get the right data and it needs to be of a high enough quality and quantity for statistical weighting purposes. As we’ve mentioned, quality is so important, it can have a direct positive or negative impact on the outcomes of ML-based models. Project leaders need to coordinate with machine learning, data science, and clinical teams before ordering medical imaging datasets. Doing this should help you overcome some of the challenges of getting ‘bad’ data or annotation teams having to sift through thousands of irrelevant or poor quality images and videos when annotating training data, and the associated cost/time impacts. #2: Addressing regulatory concerns and compliance when annotating medical imaging datasets Regulatory and compliance questions need to be addressed before buying or extracting medical image datasets, either from in-house sources, or external suppliers, such as hospitals. Project leaders and ML teams need to ensure the medical imaging datasets comply with the relevant FDA, European CE regulations, HIPAA, or any other data protection laws. Regulatory compliance concerns need to cover how data is stored, accessed, and transported, the time a project will take, and ensuring the images or videos are sufficiently anonymous (without any specific patient identifiers). Otherwise, you risk breaking laws that come with hefty fines, and even the risk of data breaches, especially when working with third-party annotation providers. #3: Give annotation teams powerful AI-based tools that specialize in medical image datasets Medical image annotation for machine learning models requires accuracy, efficiency, a high level of quality, and security. With powerful AI-based image annotation tools, medical annotators and professionals can save hours of work and generate more accurately labeled medical images. Ensure your annotation teams have the tools they need to turn medical imaging datasets into training data that AI, ML, or deep learning models can use and learn from. #4: Ensure medical imaging datasets are easy to transfer and use in machine-learning models Clinical data needs to be delivered and transferred in an easily parsable format, easy to annotate, portable, and once annotated, fast and efficient to feed into an ML model. Having the right tools helps too, as annotators and ML teams can annotate images and videos in a native format, such as DICOM and NIfTI. When searching for the ground truth of medical datasets, imaging modalities, and medical image segmentation all play a role. Giving deep learning algorithms a statistical range and quality of images alongside anonymized health information, dimensionality (in the case of DICOM images), and biomedical imaging data can produce the outcomes that ML teams and project leaders are looking for. #5: Ensuring clinical operations and ML teams have the viewing capacity for large volumes of imaging data Viewing capacity is a concern that project leaders need to factor in when there are large volumes of images or videos within a medical imaging dataset. Do your annotation and ML teams have enough devices to view this data on? Can you increase resources to ensure viewing capacity doesn’t cause a blockage in the project? #6: Overcoming storage and transfer challenges As we’ve mentioned before, storage and transfer challenges also have to be overcome. Medical imaging datasets are often many hundreds or thousands of terabytes. You can’t simply email a Zip folder to an annotation provider. Project leaders need to ensure the buying or medical raw data extraction, cleansing, storage, and transfer process is secure and efficient from end to end. #7: Apply automation and other tools during the annotation process When annotating thousands of medical images or videos, you need automation and other tools to support annotation teams. Make sure they’ve got the right tools, equipped to handle medical imaging datasets, so that whatever the quality and quantity they need to handle, you can be confident it will be managed efficiently and cost-effectively. Encord has developed our medical imaging dataset annotation software in close collaboration with medical professionals and healthcare data scientists, giving you a powerful automated image annotation suite, fully auditable data, and powerful labeling protocols. Ready to automate and improve the quality of your medical data annotations? Sign-up for an Encord Free Trial: The Active Learning Platform for Computer Vision, used by the world’s leading computer vision teams. AI-assisted labeling, model training & diagnostics, find & fix dataset errors and biases, all in one collaborative active learning platform, to get to production AI faster. Try Encord for Free Today. Want to stay updated? Follow us on Twitter and LinkedIn for more content on computer vision, training data, and active learning. Join our Discord channel to chat and connect.
Nov 11 2022
7 M
What’s the Difference Between DICOM and NIfTI?
Imaging standards and file formats play an essential role in medical imaging annotation. This article assesses the difference between DICOM and NIfTI, two of the most common medical imaging data formats. One of the most significant advances in medical image annotation is the application of machine learning to evaluate images for a more precise, faster, and more accurate medical diagnosis. Before machine learning (ML), artificial intelligence (AI), or any other diagnostic algorithms can be applied, you need to know that annotation software can handle the two most common medical and healthcare image file formats, including DICOM and NIfTI. With medical images, the data type makes a huge difference. Unlike other image file formats (e.g., JPEG, PNG), healthcare professionals need to see A LOT more detail, so the raw data needs to be in a format that will reveal layers of the human body, organs, and the brain. Hence the need for 3D images or layers of 2D slices so that when it gets to the image processing stage, 3D visualizations, and rotations can be applied to get a much clearer understanding of the medical issue a doctor is trying to diagnose. Computer vision and deep learning are playing an increasingly important role in the medical diagnosis and analysis process. Hence the advantage of specialist annotation and labeling tools that can handle DICOM data and NIfTI files so that segmentation and other labeling methods can be applied effectively. Interested in annotating DICOM or NIfTI data? Learn more about our collaborative DICOM and NIfTI annotation platform for Healthcare AI here. Let’s review the difference between the DICOM and NIfTI, including who uses them and what they’re most commonly used for. What is the DICOM standard? The DICOM standard — Digital Imaging and Communications in Medicine (DICOM) — is used to exchange images and information and has been around for over over 20 years. The use of the DICOM format started to take off in the mid-nineties. DICOM comes with several support layers, allowing image senders and receivers to exchange information about the analyzed images. Today, almost every imaging device used in radiology — including CT, MRI, Ultrasound, and RF — is equipped to support the DICOM standard. According to the standard facilitator, DICOM “enables the transfer of medical images in a multi-vendor environment and facilitates the development and expansion of picture archiving and communication systems.” Imaging devices for other medical areas of expertise, including pathology, dermatology, endoscopy, neuroscience, and ophthalmology, are starting to use the DICOM standard. Other layers of support include database connections and the ability for users to retrieve medical image header information, patient data, and metadata. Medical imaging devices can identify where images have been stored, making real-time retrieval and analysis easier. The third support layer includes image management, quality, storage, security, and patient scheduling information. One of the most important and valuable fundamentals of DICOM is the information model built into the standard. One of the most widely accepted definitions of this is: “DICOM information objects are definitions of the information to be exchanged. One can think of them as templates that are reused over and over again when a modality generates a new image or other DICOM object. Each image type, and therefore information object, has specific characteristics.” For example, “a CT image requires different descriptors in the image header than an ultrasound image or an ophthalmology image.” Information objects are also known as Service Object Pairs (SOP) Classes. Each template, or SOP, comes with unique identifiers and a template so that when data is exchanged — whether that’s an annotated image or patient scheduling information — the two devices participating in the exchange transfer everything in as much detail as the respective users need. Guidelines for the DICOM standard are overseen by the National Electrical Manufacturers Association (NEMA), known as the standard facilitator. Now let’s compare DICOM with another widely used medical and research imaging standard, NIfTI. What is the NIfTI standard? The Neuroimaging Informatics Technology Initiative (NIfTI) was established to work with medical and research device users and manufacturers to address some of the problems and shortfalls of other imaging standards. The NIfTI standard was specifically designed to address these challenges in the neuroimaging field, focusing on functional magnetic resonance imaging (fMRI). One of the most significant challenges neurosurgeons faced with older image formats, specifically the Analyze 7.5 file format, was the lack of information about the orientation of image objects. Orientation was ambiguous and unclear, forcing anyone attempting to analyze an image to add detailed notes about the orientation of objects within images. In particular, there was often confusion as to which side of the brain a doctor was looking at, a significant problem that needed a solution. NIfTI solved this problem in the following way: “In the NIfTI format, the first three dimensions are reserved to define the three spatial dimensions — x, y and z —, while the fourth dimension is reserved to define the time points — t.” Other dimensions store “voxel-specific distributional parameters [and] vector-based data.” According to NIfTI, “the primary goal of NIfTI is to provide coordinated and targeted service, training, and research to speed the development and enhance the utility of informatics tools related to neuroimaging.” NIfTI has two standards, NIfTI-1 and NIfTI-2, with the second operating as a 64-bit improvement on the original. Not replacing it, but running alongside NIfTI-1, and supported by a wide range of neuroimaging medical devices and operating software systems. NIfTI is sponsored and overseen by The National Institute of Mental Health and the National Institute of Neurological Disorders and Stroke. Now let’s review the differences between DICOM and NIfTI. What are the differences between DICOM and NIfTI? #1: Less metadata with NIfTI files With a NIfTI file, you don’t need to populate the same number of tags (integer pairs) that DICOM image files required. There’s a lot less metadata to sift through and analyze; although in some respects, that’s a downside, as DICOM gives medical users layers of data about the image and patient. Metadata overlay being toggled in Encord #2: DICOM files are often more cumbersome DICOM is described as a “robust” standard, although that can cause difficulties for users. Strict formatting requirements govern DICOM transfers to ensure the receiving device supports SOP Classes and Transfer Syntaxes, e.g., the file format and encryption used to transfer data. One device negotiates with another when DICOM files are being transferred. If one device can’t handle the information another device is attempting to send, “it will inform the requester so that the sender can either fall back to a different object (e.g., a previous version) or send the information to a different destination.” Consequently, the handling, transfer, reading, and writing of NIfTI files are usually easier and quicker than DICOM image files. It’s like comparing a text file (NIfTI) with a Word file (DICOM). One has more detail, whereas the other is simpler, smaller, and easier to use. In many cases, the extra layers of data prove invaluable for image analysis across various medical fields. #3: More information can be stored on DICOM files As mentioned above, DICOM files allow medical professionals to store more information across multiple layers. You can create structured reports and even freeze an image so that other clinicians and healthcare data scientists can clearly see what an opinion/recommendation is based on. So, although DICOM files are sometimes harder to handle, the information stored is more sophisticated and applicable across a wider range of medical use cases. Read more: Encord’s guide to medical imaging experiments and best practices for machine learning and computer vision. #4: DICOM works with 2D layers, whereas NIfTI can display 3D detail With NIfTI files, images and other data is stored in a 3D format. It’s specifically designed this way to overcome spatial orientation challenges of other medical image file formats. On the other hand, DICOM image files and the associated data is made up of 2D layers. This allows you to view different slices of an image, which is especially useful when analyzing the human body and various organs. 2D Multiplanar Reconstruction (MPR) in Encord #5: NIfTI can take longer to load; DICOM enables users to display one layer at a time Although the transfer of DICOM files often takes longer, NIfTI can take longer to load because the image data is stored in a 3D format. Depending on the software or image viewer being used, everything attempts to load at the same time. Whereas, once a DICOM file is received, users can display data one image at a time or simply load a single image and the associated metadata, such as the medial notes connected to that image. The 2D layering of DICOM files means it’s quicker and easier to apply medical image annotation software or to send an image and notes to the printer in a medical workplace. #6: You can convert DICOM into NIfTI And finally — not a difference, but it’s useful to know healthcare professionals and data scientists can convert DICOM into NIfTI files without losing image quality. A NIfTI conversion is helpful when you need the data in a different format. Several open-source and proprietary software packages will help you do this, as the main challenge to overcome is combining the layers into a single image without losing any annotations, labels, or metadata. Need to know more about annotating DICOM into NIfTI files? Check out: How to Annotate DICOM and NIfTI Files Who uses DICOM and what’s it used for? DICOM is a more widely used image format in the healthcare profession, with a wide range of specialist fields deploying it, including radiology (for CT, X-Ray, MRI, Ultrasound, and RF), pathology, dermatology, endoscopy, and ophthalmology. Hospitals worldwide use DICOM not only for the ability to share images and annotations but for the easy transfer and link to patient medical records, scheduling, and other valuable medical metadata objects. DICOM is especially useful when trying to detect cancers. When DICOM files are labeled with DICOM-compatible medical image annotation tools, annotators can train models to spot cancers faster and more effectively, making drastic improvements in early detection. Who uses NIfTI and what’s it used for? NIfTI was originally created to solve a serious spatial orientation problem in neuroimaging, focusing on functional magnetic resonance imaging (fMRI). With NIfTI, neurosurgeons can quickly identify image objects, such as the right or left side of the brain, in 3D. It’s an invaluable asset when analyzing human brain images, a notoriously difficult organ to assess and annotate. Radiologists are also using NIfTI file formats, as are image-based computer vision research teams and AI startups in the medical sector seeking FDA approval. AI-based Medical Image Annotation for DICOM and NIfTI Labeling Both medical image file formats are incredibly useful and powerful. Both have advantages and disadvantages. The good news is, whether you use DICOM or NIfTI, or both, Encord’s medical imaging annotation suite supports both file formats and standards. Our imaging software is the first purpose-built 3D annotation tool for healthcare AI Encord has developed this software in close collaboration with medical professionals and healthcare data scientists, giving you a powerful automated image annotation suite with precise, 3D annotation built-in, fully auditable images, and unparalleled efficiency. Accelerate your DICOM and annotation, labeling, and quality assurance with Encord Active: the open-source active learning toolkit for computer vision. Ready to automate and improve the quality of your medical imaging datasets, including DICOM and NIfTI image annotation? Sign-up for an Encord Free Trial: The Active Learning Platform for Computer Vision, used by the world’s leading computer vision teams. AI-assisted labeling, model training & diagnostics, find & fix dataset errors and biases, all in one collaborative active learning platform, to get to production AI faster. Try Encord for Free Today. Want to stay updated? Follow us on Twitter and LinkedIn for more content on computer vision, training data, and active learning. Join our Discord channel to chat and connect. Here are some examples of healthcare and medical imaging projects involving Encord: Floy, an AI company that helps radiologists detect critical incidental findings in medical images, reduced CT & MRI Annotation time with AI-assisted labeling RapidAI reduced MRI and CT Annotation time by 70% using Encord for AI-assisted labeling. Stanford Medicine cut experiment duration time from 21 to 4 days while processing 3x the number of images in 1 platform rather than 3 Further Reading: Healthcare and Medical Imaging Annotation Medical Image Segmentation: A Complete Guide 3 ECG Annotation Tools for Machine Learning Top 10 Free Healthcare Datasets for Computer Vision The Top 6 Artificial Intelligence Healthcare Trends of 2023
Nov 11 2022
5 M
The Comprehensive Guide to Medical Annotations
Medical annotations are more complicated than applying annotations and labels to non-medical images. In most cases, file sizes, formats, modalities, and the sheer volume of data is larger and more complicated than other image-based datasets. Medical annotations must be more accurate for training algorithmic models, patient outcomes, and healthcare plans. Mistakes are costly in the medical profession. Patient healthcare plans, treatments, and outcomes depend on an accurate diagnosis. Medical image annotations are part of labeling anything from X-Rays to CT scans. When applied to image or video-based datasets that are used to train computer vision, machine learning, and artificial intelligence (CV, ML, AI, etc.) models, medical annotations are integral to new treatment innovations across the healthcare sector. In this article, we provide more detail about the medical image annotation process, including healthcare use cases, best practice guidelines, and considerations medical ML teams need to factor in when annotating images and videos. What are Medical Image Annotations? Encord's DICOM annotation tool Computer vision models and other algorithmic models, such as artificial intelligence (AI), and machine learning (ML), rely on accurately annotated and labeled datasets to train them. In a medical environment, the annotations and labels need to be even more precise to produce accurate outcomes, such as diagnosing patients. Accurate annotated examples of medical images are crucial for training and making a model production-ready. Annotations usually are initially provided by experts in the relevant medical specialism. Then annotation teams and AI-powered tools normally take over to annotate vast datasets based on the labels created. The best example of this is a radiologist who uses an annotation platform to note down their opinion of a scan, which in turn trains the neural network accordingly. Companies can build their own labeling platform or take advantage of third-party medical imaging labeling tools (take a look at this blog for the pros and cons of each approach). Whatever you decide to do, the better your approach to labeling your DICOM or NIfTI images, the better your model will perform. How does Medical Image Annotation Compare With Regular Image Annotation? Medical image annotation is more complex than annotating datasets filled with (non-medical) images, such as JPGs or PNG files. Medical data ops and annotation teams have much more to consider, such as regulatory compliance, layered file types, 2D, 3D, and even 4D formats, windowing control settings, and much more. Here’s a table to explain some of the challenges of medical image annotation: Now let’s take a look at medical annotation use cases and the numerous ways annotations and labels can be applied for computer vision models in the healthcare sector. Medical Image Annotation Use Cases There are hundreds of use cases for medical annotations and labeling across dozens of specialisms and healthcare practices, including the following: Pathology For the vast majority of diseases, most of the diagnostic capabilities come from various scans and images that are taken by highly specialized medical equipment. By labeling these scans accurately, we can train machine learning models to pick up those diseases themselves, reducing the need for human involvement. Radiology Radiology is one of the most common use cases for medical image annotations. Mainly because of the vast number of images this medical field generates, with dozens of radiology modalities, including X-ray, mammography, CT, PET, and MRI. With the right annotation tool, medical data ops and annotation teams can benefit from a PACS-style interface to make native DICOM and NIfTI image rendering possible. Plus, annotation tools should come with customizable hotkeys and other features for multiplanar reconstruction (MPR) and maximum intensity projection (MIP). Gastroenterology You can improve video yields and accelerate GI model development with the right annotation tool. For gastroenterology model development, you need an annotation tool to support native video uploads of any size or length. This proves especially useful for annotation and computer vision work designed to detect cancerous polyps, ulcers, IBS, and other conditions. Histology Medical image annotation is equally useful for histology, giving annotators and medical ops teams the ability and tools to Increase micro and macroscopic data labeling protocols and training datasets. With the right annotation tools, you should have inbuilt support for the most common and widely-used staining protocols (including hematoxylin and eosin stain (H&E), KI67, and HER2). Plus, it’s time and cost-saving to use an annotation platform compared to booking out expensive microscopy workstation time. Surgical Surgical AI models benefit from faster, AI-powered automated annotations. For this, medical data operations teams need a medical-grade video annotation and clinical operations platform designed for surgical intelligence use cases. Cancer Detection Cancers are notoriously challenging to diagnose, so medical image annotation can help us train models that spot them earlier and more accurately than humans can – which can make a huge difference to patient outcomes. When computer vision models are used to screen for the most common cancers automatically, we can drastically improve early detection and treatment plans and outcomes. Ultrasound By annotating ultrasound images, we can use artificial intelligence (AI) to pick up higher levels of granularity for things like gallbladder stones, fetal deformation, and other diagnostic insights. The quicker we understand what we’re dealing with, the better the care will be. Microscopy In medical research, we rely a lot on what we can examine under a microscope to understand what’s happening at the lowest level of abstraction. By labeling these images and applying them as a training dataset, we can push medical research forward and scale our impact as a result. The beauty of machine learning is that there are many more use cases to discover as we start to work with this data and let the algorithms do their thing. This is at the forefront of the future of medicine, and the quality of the annotations will be a significant factor in how things evolve. Read more about the top 6 AI trends in healthcare. Windowing preset feature on the Encord medical image annotation tool Essential Considerations When Preparing Healthcare Imaging Data for Computer Vision Medical image annotation relies on high levels of precision because of the complexity involved and the stakes under which these models will be used. In order to pass FDA approval and make it to production, the data must be of the highest quality possible – to adhere to regulatory guidelines and create a stronger and more effective machine learning model. To do this at scale, organizations need to make it as easy and intuitive as possible for annotators to capture the required information. The time of these experts is costly, so the more efficient and frictionless the annotation process, the better quality data you’ll get and the more you can control costs. Four key considerations should be prioritized when you’re tasked with annotating medical imaging data, or managing an annotation team: Dataset volumes, sizes, and file types As with any machine learning project, the more data you have to train with, the better the model will perform. This assumes a certain level of data quality, of course, but wherever you can – you should try to increase the size of the training set as much as possible. Data Distribution & Diversity In the medical field, there is tremendous diversity in terms of human bodies, and that needs to be reflected in your data. This diversity is crucial if you want your model to be effective in the real world. It would be best to ensure sufficient distribution across demographic factors like age, gender, geography, hospitals, previously diagnosed conditions, and other relevant details. Read more: Encord’s guide to medical imaging experiments and best practices for machine learning and computer vision. Data Formats Medical images can come in various formats, including DICOM and NIfTI images, CT (Computed Tomography) scans, X-Rays, and Magnetic Resonance Imaging (MRI) files. Your annotation process needs to handle these formats natively so that you don’t lose any detail or information along the way. This ensures that you’re getting the most out of your workflow and know it can fit into the existing medical system in which you want to innovate. Data Visualization When images are delivered in 3D, you need to ensure you view the files in the same format to get the full picture of whatever medical image is annotated. You want to provide the annotator with everything they need to provide an accurate evaluation, which means that you need to be thoughtful and intentional about how you present the images to them. It’s equally important to consider the different views and volumes of medical images, such as 2D and 3D. In most cases, you need both to assess what’s happening in the images accurately. Image types such as MRI, CT, and OCT scans can be viewed in a number of ways, such as using the sagittal, axial, or coronal planes. However, for ML purposes, it’s more time and cost-effective to pick and annotate those to train a model. This list is not exhaustive, but it should give you a sense of what to consider when building a medical image annotation workflow. Brush selection tool for annotating DICOM and NIfTI images in Encord How to Implement Medical Image Annotations? Here are the steps you need to take to implement medical image annotations for a computer vision project. Sourcing Data Medical image or video datasets can be sourced in numerous ways. There are dozens of open-source medical datasets. Healthcare organizations might have their own in-house data sources they can tap into and access. Or you will have to buy datasets from hospitals and healthcare providers. It depends on how much data you need (images, videos, etc.), and the health issues you’re investigating and training an algorithmic model on. Budgets, timescales, and the resources you invest in annotating and labeling datasets also play a role. As do the tools you’re going to use, as automated tools accelerate the medical image annotation process. Need to source medical imaging data quickly and for free? Here are the top 10 free healthcare datasets. Preparing Medical Image Datasets Once you’ve sourced these datasets, the images and videos within need to be cleaned and prepared for the annotation process. If your AI model is being trained as part of a commercial project aiming for FDA approval, then it’s essential that patient identifiers are removed from tags and metadata. You need to split and partition the dataset, ideally keeping it in a separate physical location to ensure FDA approval is easier to achieve. Create the Annotations and Labels Next, the annotations and labels need to be created. In most cases, when a project is for a medical use case, healthcare professionals should create the labels. Especially if the annotation and labeling work is being outsourced to non-experts; otherwise, you risk the entire project by hoping annotators know what they’re looking at. Once the labels are ready, and ideally pre-populated in a small sample of the dataset, then the annotation work can begin. Whether in-house or outsourced, starting this with smaller selections from the overall dataset makes sense. This way, especially initially, annotator outputs can be more effectively measured against data labeling key performance indicators (KPIs). A quality assurance loop can be cycled through a few iterations until enough annotations and labels have been applied to validate and test a dataset sample, to start training the model. Validate and Test A Dataset Sample Medical imaging computer vision model training involves a continuous series of experiments. How much data scientists are involved depends on the way a model is being trained (e.g., unsupervised, semi-supervised, self-supervised, human-in-the-loop, etc.), and the outcomes of the validation and test dataset samples. Here are 7 ways to improve medical imaging experiments. In some cases, you might need to re-do the labels and annotations until they’re more accurate. Or very least, a percentage of them might need to be improved and enhanced until the dataset is ready to be fed into the ML model. Data Security & Regulatory Compliance (HIPPA, FDA, CE) for Medical Image Annotation Tools Alongside all the considerations about the quality of the data being captured and the efficiency of the process, we also need to think carefully about the security of the images that you’re annotating. The labeling tool that you’re using needs to conform to the most up-to-date security best practices to reassure your stakeholders and customers that you’re taking medical data security seriously. There are two key regulatory frameworks that you should be aware of here if you’re looking for an external medical image annotation tool: SOC 2 defines criteria and benchmarks for managing customer data and is measured through an external audit that evaluates data security practices across the board. HIPAA (the Health Insurance Portability and Accountability Act) is a U.S. Federal law that protects sensitive patient health information. This is a non-negotiable for whoever is providing your data labeling tool. Depending on why an algorithmic model is being developed, you may also need to consider US Food & Drug Administration (FDA) compliance. We’ve got a guide for getting AI models through the FDA approval process. For AI companies or healthcare organizations operating in Europe, there are also GDPR and European Union (EU) CE regulations to think about. Although they operate in similar ways to US data protection and healthcare-specific laws, it’s important to ensure data handling, processing, security, and audit trails are compliant with the relevant legislation. As well as the data security credentials of your annotation tool provider, you also need to control the permissions available to your annotators carefully. You want to have very granular access controls so that they only see the absolute minimum that they need in order to do their job. All of this should be tied up in a product where you retain the rights to your data and models – which simultaneously protects your IP and makes it easier to ensure high-quality data protection from the source all the way to final outputs. Different Types of Tools for Medical Image Annotations & Labels Medical imaging, healthcare, and annotation teams have three main options for selecting medical annotation tools: Open-source annotation tools; In-house annotation tools, Or powerful third-party annotation tools and platforms. The first two, open-source and in-house, have several limitations, especially when it comes to data compliance, scalability, collaborative workflows, and in some cases, audit trails or the lack thereof. In-house tools are also notoriously expensive and time-consuming to build and maintain. On the other hand, with a powerful third-party annotation tool, you can be up and running quickly, have complete data auditability, and compliance, and benefit from powerful medical annotation and collaborative workflow features. Including quality control and quality assurance. Here are three ECG annotation tools you can use. Medical Image Annotations With Encord At Encord, we have developed our medical imaging dataset annotation software in collaboration with data operations, machine learning, and AI leaders across the medical industry – this has enabled us to build a powerful, automated image annotation suite, allowing for fully auditable data, and powerful labeling protocols. Encord comes complete with Encord Active, Encord’s Annotator training module, and an extensive range of features specifically for medical image annotations, labeling, AI-assisted labeling, quality assurance, and workflows. Case studies from projects that Encord has been deployed in for medical annotations include the following: Floy, an AI company that helps radiologists detect critical incidental findings in medical images, reduced CT & MRI Annotation time with AI-assisted labeling RapidAI reduced MRI and CT Annotation time by 70% using Encord for AI-assisted labeling. Stanford Medicine cut experiment duration time from 21 to 4 days while processing 3x the number of images in 1 platform rather than 3 Ready to automate and improve the quality of your data labeling? Sign-up for an Encord Free Trial: The Active Learning Platform for Computer Vision, used by the world’s leading computer vision teams. AI-assisted labeling, model training & diagnostics, find & fix dataset errors and biases, all in one collaborative active learning platform, to get to production AI faster. Try Encord for Free Today. Want to stay updated? Follow us on Twitter and LinkedIn for more content on computer vision, training data, and active learning. Join our Discord channel to chat and connect.
Nov 11 2022
10 M
7 Features to Look for in a DICOM Annotation Tool
If you’re trying to create training data for a medical AI model, you might have used free and open-source tools like ITK SNAP to label medical images. And they’re great as a starting point but do lack a number of features for annotating efficiently and effectively. So if you’ve come to the conclusion that you need a better solution for labeling medical images (especially in DICOM or NIfTI formats), you’ll be looking around at image labeling tools. But even when looking at paid for tools, there is an element of risk. Not all image annotation tools are created equal, especially when it comes to the specific needs of the computer vision and healthcare space. So to help you find the right platform, we’ve created a guide to the seven features you need to look for when choosing tools for annotating and labeling DICOM images. Native DICOM support It might seem obvious, but a fundamental consideration is whether the annotation tool you’re looking at can support DICOM files natively (it also helps if it can natively support other file formats, like NIfTI). What that means is whether the tool can open and view DICOM files without having to convert them to some other format (such as a video file). When a file is converted from DICOM to something else, it increases the chances of data being lost (such as DICOM metadata) or the converted file is corrupted in some way. Converted files are also not displayed using Hounsfield units, because they have to be shifted to a grayscale range that is compatible with the file format they've been converted to. Ultimately this results in lower-quality annotations as your annotators are losing vital data from the images they’re looking at. And given the importance of having high-quality training data for medical AI models, it makes sense to ensure you’re not losing anything when you add your image files to your data labeling tool. DICOM support in Encord Native 3D annotation Another key feature is the ability to view and annotate images in 3D, natively within the annotation platform. This makes it easier to identify objects within the scans (such as cancerous tumors) and also allows for volumetric annotations, where you’re labeling something in three dimensions. Having the ability to annotate radiology images in 3D means you can create better annotations and ultimately create better data to train your model on Easy to use interface While this might seem like a basic point, it is something that needs a lot of consideration. There are many labeling tools out there that can be used to annotate medical images, but they won’t have been designed with this specific task in mind. Annotating a chest X-Ray or brain MRI is a very different task from labeling road signs or fruit, and the tool you use has to reflect that. Some key usability features you need to look for when choosing a DICOM annotation tool include: The ability to render images in the full range of the Hounsfield scale Multiplanar reconstruction showing images in 2D orthogonal planes (coronal, sagittal, axial) so you can better visualize, analyze, and annotate the images Window width (WW) and window level (WL) adjustments with the option to save custom preset, which can save your annotators time A distance measurement tool for measuring the accurate, real-world distance between any two points in an image View metadata as an overlay so your annotators can easily see the metadata when they need it. Window width and window level adjustment in Encord Automated annotation of DICOM images Given the expense of using highly skilled medical annotators, any feature that can make them more efficient is vital. This is why your DICOM annotation tool needs to have annotation automation functionality. There are a number of ways automation can be achieved, but one of the most powerful is interpolation. But not all interpolation features are the same. You’ll want one that: Doesn’t need matching pixel information in neighboring frames in order to function Doesn’t require a matching number of vertices between objects in set keyframes Allows you to draw object vertices in arbitrary directions, and not have to follow a predefined direction Quality control features Maintaining the quality of your labeled data is vital for ensuring your models have the best ground truth to learn from. And being able to put rigorous quality control measures in place can make this much easier and more efficient. What you need to be looking for in your DICOM annotation platform is two things. The first is the ability to set granular parameters for your quality control workflows. This should include the following: The percentage of labels that are to be manually reviewed Rules for distribution of review tasks Common rejection reasons that can be used to identify and systematize errors in your labels Reviewer to class and annotator mapping (e.g. label X with class Y should always be reviewed by reviewer Z) Assign tasks that are rejected after a specific number of review cycles for expert reviews. Then the second quality control feature to look for is being able to dynamically change the sampling rate applied to submitted annotation tasks. This means the project administrator can set a higher proportion of submitted labels the reviewer should look at, increasing the overall quality of the labeled data. It also helps if this can be tweaked further to set sampling rates by annotator and annotation type so that more complex images get a more thorough review. Data labeling quality control panel in Encord Audit Trails The penultimate feature you need to consider for your DICOM annotation tool is around creating an audit trail. To get FDA or CE approval, one of the requirements is to be able to provide a full audit trail of the data that your medical diagnostic model is trained on. With this in mind, your labeling tool needs to have the ability to show a full audit trail for every label produced and the review process behind that label. SOC2 and HIPAA compliance And finally, whatever medical image labeling tool you end up using, if you’re going to be using it to handle sensitive patient data, it needs to comply with a couple of critical frameworks. The first of these is SOC 2 (Systems and Organizational Control 2), which demonstrates that an organization’s business process, information technology, and risk management controls are properly designed. The second of these is compliance with HIPAA (Health Insurance Portability and Accountability Act), showing that the data labeling platform looks after patient data in accordance with the rules of HIPAA. So there you have it - seven features that you should be looking at when choosing your DICOM annotation platform. Taken together, these features can make your medical image labeling much more efficient while also resulting in better labeled data and reduced risk. Looking for a DICOM annotation tool (which also supports NIfTI and a range of other file formats)? Sign-up for an Encord Free Trial: The Active Learning Platform for Computer Vision, used by the world’s leading computer vision teams. AI-assisted labeling, model training & diagnostics, find & fix dataset errors and biases, all in one collaborative active learning platform, to get to production AI faster. Try Encord for Free Today. Want to stay updated? Follow us on Twitter and LinkedIn for more content on computer vision, training data, and active learning. Join our Discord channel to chat and connect.
Nov 11 2022
6 M
How to Annotate DICOM and NIfTI Files
In medical image annotation and computer vision models, the datasets and tools used have highly-specialized requirements. In this article, we will outline the best practice for using DICOM and NIfTI files in computer vision models. Medical imaging and annotation is a specialized field. Perhaps more so than any others, accuracy is crucial. When we consider the end-users, such as healthcare professionals, and the ultimate outcomes, the impact on patients, we can see why accuracy is crucial. A correct or incorrect diagnosis impacts treatment, care plans, and outcomes. Accurate computer vision models and machine learning-powered annotation of videos and images can make the difference between life and death for some patients. Before diving into four best practices to follow when annotating DICOM and NIfTI images, let’s take a moment to consider data security, and clarify how those imaging formats differ from others used, such as PACS and JPEG. Data Security in Medical Image Labeling and Computer Vision Models Healthcare providers have strict regulatory oversights worldwide. In every country, government agencies (e.g. the FDA and European CE regulations) and watchdogs carefully monitor compliance of patients’ rights, data protection, and the accuracy of patients’ records. In the US, for example, HIPAA (the Health Insurance Portability and Accountability Act) is non-negotiable for handling and processing sensitive patient healthcare data, including images, labeling, annotation, and computer vision analysis. SOC 2 is another benchmark for handling consumer and patient data. It includes an external audit that evaluates data security for the entire end-to-end data process, including computer vision and medical image annotation software. Data security best practices are mission-critical in the healthcare sector. Medical Imaging Standards Used in Computer Vision Models: DICOM & NIfTI In most cases, healthcare providers use the DICOM and NIfTI imaging standards. Medical images play a role in that, as 3D and 2D scans — regardless of the imaging standard — are integral to the diagnosis doctors give patients. Accuracy, especially when labeling medical images, is crucial. Consequently, the software healthcare providers use to label, annotate, and analyze images plays a key role in healthcare decision-making and patient treatment plans. Computer vision models are powerful Artificial Intelligence and Machine Learning-based (AI/ML) software tools for analyzing images. Healthcare organizations’ commitment to accuracy, excellence, and cost-effective treatment is one of the main drivers behind adopting computer vision models in image and video analysis. In a previous article, we explained what’s the difference between the DICOM and NIfTI healthcare imaging standards? Have a read to find out more. What is the difference between the DICOM format and JPEG? One of the most common imaging formats is JPEG (Joint Photographic Experts Group), and although widely used the world over, it’s not practical or useful in a medical setting. DICOM files contain layers and layers of images, associated metadata, and links to databases and other medical systems. On the other hand, JPEG files are single-layer 2D images. Medical images in a JPEG format wouldn’t be detailed nor useful enough for medical purposes. Although you can convert DICOM and other files into JPEGs, this is usually convenient when explaining something in simple terms to a patient. DICOM image being annotated in Encord What is the difference between DICOM and PACS? In most healthcare workplaces, doctors and specialists also use the Picture Archiving and Communication System, or PACS, alongside other imaging formats. PACS is used as a medical image storage and archive system, with images being fed into by radiologists and other medical specialists. Images usually come from X-ray machines and MRI scanners. On the other hand, the DICOM format is an international communication standard for storing, communicating and transmitting medical images with layers of metadata. Medical professionals can use both, with one format supporting the other to ensure every stakeholder involved in patient care has the necessary information. Now let’s dive into our overview of the four best practices healthcare annotators and medical professionals should apply when using DICOM and NIfTI files in Computer Vision Models. 4 Best Practices for Using DICOM and NIfTI File Format in Computer Vision Models #1: Display the data correctly to allow for pixel-perfect annotations When annotators and data scientists talk about displaying the data “correctly”, we mean in a native format. So that when images or videos are uploaded to an AI-based computer vision annotation tool — whether in DICOM format or NIfTI format — nothing is lost. In the best video annotation and computer vision tools, DICOM or NIfTI formatting is displayed natively. Videos of any length can be displayed and processed. And crucially, DICOM files come with layers of patient information, such as database connections, image analysis and doctor notes, and even scheduling data for appointments. None of this information must be lost during AI-based computer vision analysis of those images. For example, DICOM images are used in CT, MRI, Ultrasound, and RF. Displaying these images in a native format means that annotators and healthcare professionals can accurately measure the size of tumors and other medical problems, and this information can be fed into computer vision models. Another example is that displaying gastro and other videos natively means that videos of any length (timescale) can be uploaded, allowing for faster loading, no data loss, and more accurate analysis in computer vision models. #2: Ensure high levels of medical image annotation quality for computer vision models Computer vision models need the highest quality of labels and annotation to ensure AI-based algorithms analyze images as accurately as possible. With the right medical imaging tools and workflows, this is achieved two ways: Consensus benchmarks. Having a team of annotators assess and annotate the same images, supported by a computer vision modeling tool, makes achieving a benchmark for expert review easier. Medical imaging is sometimes difficult to assess and not clear-cut, so leveraging multiple experts' consensus helps ensure quality is as high as possible. Granular expert review workflows. Medical professionals don’t have time to annotate and label medical images manually. Annotators make the manual inputs; in most cases, those images are processed through computer vision models and tools. After that, annotated images are passed onto an expert, such as a senior radiologist or the company's “annotation gold standard” expert. Any mistakes or inaccuracies are sent back to be re-annotated before the images can be reviewed by healthcare professionals making decisions on patient cases. #3: Make data audits granular: Mission-critical for healthcare regulatory compliance Regulatory compliance is mission-critical in healthcare. In the US, healthcare providers have HIPAA, SOC 2, and other data protection laws to consider. In Europe, similar laws and regulations exist, alongside patient and consumer watchdogs and GDPR. Healthcare providers need the ability to fully audit computer vision model training data, down to the granular level. You should also be able to export data granularly. Complete control and data auditability ensure no delays or unexpected and unwelcome surprises in the regulatory approval process. This reduces workloads for annotators, admin staff, and departmental heads. #4: Improve image and video annotation efficiency with automation, to save radiologists valuable time Annotation work needs to be done as efficiently as possible. Radiologists' and other senior medical professionals’ time is valuable and expensive. Here’s a few ways images can be annotated, labeled and processed efficiently: A medical imaging tool with an intuitive user-interface. Radiologists are used to working in certain ways, with specific tools and systems (e.g., the Picture Archiving and Communication System, or PACS), and imaging formats, such as DICOM and NIfTI. To ensure radiologists and other medical professionals, any medical imaging tool introduced should have an intuitive interface that’s quick and easy to learn. Automation features. Manually annotating dozens or hundreds of slides is time-consuming, especially when there’s layers of slides for every DICOM image file. Automation features, such as pre-processing saves hours of manual annotation work, and here’s how this benefits medical professionals and organizations: Pre-processing ensures that medical professionals only look at and adjust images that have already been annotated and labeled. Automation and machine learning models ensure a higher standard of accuracy, quality, and consistency. In microscopy, annotators can manually label cells of interest, and then use automation features to annotate much larger datasets with much greater speed and accuracy. Encord’s platform is already in use in several healthcare organizations, making positive real-word impacts to patient care. At Stanford Medicine, the Division of Nephrology reduced experiment duration by 80% while processing 3x more images. Encord has also been deployed in medical image annotation projects by King’s College London and Memorial Sloan Kettering Cancer Center (MSK). Take a look at other case studies here. Key Takeaways Before applying computer vision models to DICOM and NIfTI medical images, annotators need to implement several best practice steps to get the best results possible, such as: Display the data correctly to allow for pixel-perfect annotations; e.g. using tools that display DICOM and NIfTI in a native format Ensure high levels of medical image annotation quality for computer vision models. Manual labeling at the start of the process, and automation tools, generate higher levels of annotation quality and accuracy when images are fed into computer vision models Make data audits granular: Mission-critical for healthcare regulatory compliance. With the right tools, you don’t need to worry about this because data granularity and audit-friendly features come as standard Improve image and video annotation efficiency with automation, to save radiologists valuable time. Healthcare professionals' time is valuable and expensive. Annotators can save stakeholders and themselves time and money when using automation features, such as pre-processing tools. Medical image annotation for computer vision models requires accuracy, efficiency, and a high level of quality. With powerful AI-based image annotation tools, medical annotators and professionals can save hours of work and generate more accurately labeled medical images. Experience Encord in action. Dramatically reduce manual video annotation tasks, generating massive savings and efficiencies. Try it for free today.
Nov 11 2022
5 M
How To Obtain CE Approval for Medical Diagnostic Models
When it comes to medical AI, obtaining regulatory approval to sell a product within the EU is a long and complicated process. This article provides an overview of the steps required to obtain CE approval under the EU MDR, including working with a Notified Body, developing a QMS that aligns with best practices for ISO 13485 certification, establishing the Intended Use and device classification for medical technology, and compiling the documents required for the Technical Files audit for CE approval. Medical devices date back to early civilization. In 6000 BCE, Neolithic groups fashioned knives, saws, and drills out of stones for use in surgery, amputation, and dental work. Historical records show that by 500 CE, the Greeks and Romans had documented the widespread use of medical instruments. And in the mid-1800s, scientific advancement led to a proliferation of medical devices being used to treat soldiers’ wounds and the ailments of wealthy families. Regulating the use of medical devices, however, is a much newer concept, which largely came about after World War II when an explosion of technological progress and manufacturing capacity resulted in a surge of devices being sold to hospitals and doctors. In the years since, when incidents involving medical devices have occurred, governments have reacted accordingly, changing existing regulations and implementing new ones. At their core, these regulations– which vary from country to country–exist to protect patients. While regulators have traditionally focused on physical products (such as scalpels, thermometers, and prosthetics), the acceleration of technology and expansion of engineering has resulted in the increased use of AI and machine learning within the medical field. When used for medical purposes, machine learning models and the algorithms used to build them are considered medical devices and, for regulatory approval purposes, often considered components of healthcare software. Unfortunately, technology evolves much more quickly than regulatory guidelines. Often, the medical device guidelines are somewhat generic, designed for generalisation and not for the nuances of building deep learning algorithms. As a result, AI companies may experience tension between complying with regulations and developing medical machine learning models designed for continuous improvement. Nonetheless, meeting the essential requirements of the regulations for healthcare software in your intended market is paramount for commercializing your medical diagnostic model. Below is a high-level overview of the current (2022) process for meeting regulatory approval to deploy a medical model in the European Union. Understanding European Regulatory Approval: The Difference Between the CE Mark and the MDR To sell any product in Europe, a company must obtain a CE marking for that specific good. CE stands for “Conformité Européene,” and the mark indicates that the manufacturer has confirmed the product conforms to European health, safety, and environmental protection standards. Once a product has CE approval, it can be sold throughout the European Economic Area, regardless of where it’s made. The essential requirements for products vary depending on the product type. Different product types must comply with the standards of different Directives set by the European Commission. Medical devices, including healthcare models, must meet the standards laid out in the EU Medical Device Regulation (MDR). To receive a CE marking for a diagnostic model, an AI company must show that its technology complies with the essential requirements outlined in the MDR. However, AI companies should think from a software perspective rather than a model-only perspective. Because a model is part of the overall software, a manufacturer needs to ensure that all components of software intended for diagnostic support work well. If a model predicts correctly, but the software has a bug that causes an incomprehensible output, then the AI company won’t receive CE approval for its product. To obtain CE approval, the manufacturer must determine the appropriate medical device classification level for their healthcare software and complete the conformity assessment for that classification level. Who Approves the CE Marking for Medical AI? The European Commission doesn’t actually judge firsthand whether the medical product fulfills the essential requirements of the Directive. Instead, they rely on private, for-profit, external organizations known as Notified Bodies to perform the conformity assessment. The EU has approved approximately 25 Notified Bodies to carry out these assessments for medical software, and the company can work with whichever one they like. However, the company is also responsible for paying the Notified Body to conduct the audit. For all companies, but especially startups with more limited resources, the cost and time required to navigate these complex regulations can create barriers to getting a product to market. Many larger AI companies will opt to work with consultants to ensure that the process is successful on the first attempt. However, since getting a product through regulation alone can cost tens of thousands of dollars, for many startups, hiring external expertise isn’t a viable option. In this case, startups in the medical AI ecosystem should consider working together, sharing information about similar technologies that have received regulatory approval, and imitating the path previously taken. When building medical AI, companies shouldn’t underestimate the need to think about regulatory approval from the get-go. If you don't document your processes and design your healthcare model with these regulations in mind, you run the risk of accumulating a lot of technical debt or being unable to sell your product to consumers. Obtaining CE Approval for Healthcare AI The first step in securing a CE mark for your AI is declaring its Intended Use, which is exactly what it sounds like you must declare what your product does, including the medical purpose and product description (e.g., how it works), who should use it, and how they should use it. The Intended Use is the foundation of the conformity assessment. It is a lengthy description that provides a detailed level of documentation outlining both the Intended Use and how and why the company concluded that this was the best and most appropriate use of the technology. Within this section, you’ll need to include a lot of information, such as a description of the AI, its purpose and use environment, intended users and patient populations, anticipated risks, and other details. The Intended Use document describes what the software should do, not what it could do. As you develop and evaluate the technology, you’ll work to prove that it achieves this purpose via software tests, user tests, clinical validation, and more. Start-ups operate at a fast pace, and their ability to adapt as they gain new information is often beneficial for product performance. However, for higher-risk devices (Class II and above), the MDR prevents any “significant changes” to the design (such as introducing a new machine learning algorithm) or the product’s Intended Use (such as diagnosing a different disease or treating a different part of the body) because these changes could affect the product’s safety and performance. When it comes to algorithms and models, there’s still some debate about what qualifies as a “significant” change. The European Commission has provided some guidance, but ultimately, the Notified Body will decide on a case-by-case basis whether a change is significant. The best strategy for AI companies seeking regulatory approval quickly is to make as few changes as possible to the model once you’ve declared your Intended Use. While doing so may hinder technological innovation, it prevents an AI company from encountering the long review timelines and additional costs that often accompany obtaining approval for a significant change. The Intended Use will function as a roadmap for regulators. After you outline the purpose of your technology and patient population that it will serve, the Notified Body will evaluate whether the technology actually achieves that purpose for that population in a safe and effective manner. That’s why if you attempt to change this description later on, you’ll have to start the entire conformity assessment again. When establishing the Intended Use, you’ll also need to determine the medical device classification of your software. The EU ranks devices from Class I to Class III based on the risks they pose to patients. Class I devices, such as bandages and crutches, have low risks to patients and do not include a measuring function. Class II devices, which pose moderate risks, are broken down into two subsets. Class II.a. carries less risk, such as blood pressure cuffs and syringes, while Class II.b. poses a slightly higher risk and often has direct contact with the human body, such as anesthesia machines and ventilators. Class III are high-risk devices, like pacemakers and stents. The higher the class of the device, the more thorough the conformity assessment required and the more intense the regulatory scrutiny. Because the consequence of a malfunctioning device rises with the class level, so does the manufacturer’s burden to prove that the device is both safe for patients and beneficial to patients or medical professionals. Because medical machine learning models are usually considered a part of healthcare software, they mostly fall under Class IIa or Class IIb. The more autonomous the AI, the more likely it is to fall into the higher class. For a Class II Medical Device, the conformity assessment includes a two-part audit by the Notified Body. Part one of the audit requires that a company show that the Quality Management System (QMS) used for its software development complies with International Standards of Organizations regulations for medical devices (ISO 13485) – regulation which is independent of, but connected with, the MDR. Part two requires that the company produce specific technical documentation (known as Technical Files) that details the development and testing of its technology. Compiling the Documentation Required for European Regulatory Approval To pass the QMS audit, a company must provide the Notified Body with a collection of documents that details the processes and procedures used to develop the healthcare software. Expect to provide hundreds of pages documenting dozens of standard operating procedures. In general, the documents provided for the QMS must show the operating procedures that the company used when developing its software, including the medical model. You’ll need to show the processes you used to collect, anonymize, label, and store patient data. Keep in mind that your data processes must be compliant with data protection and privacy laws, which means navigating an inherent tension between providing evidence that your healthcare model works for the intended population (a requirement for CE approval) and adhering to GDPR regulations. For instance, let’s say that to prove the benefit of your technology; you need to show that your diagnostic model performs exceptionally well on men aged 40 to 60. In doing so, you’ll also have to think about protecting the identity of patients. When you begin collecting patient data, you’ll want to structure the data so that it meets both requirements. Rather than list the patient’s age, consider bucketing the data into age ranges of 10 years to help ensure privacy. Modern machine learning algorithms must be trained on vast amounts of annotated data, and you’ll need to complete an audit trail about where the data came from, who annotated it, and how. Having the correct technology will make producing this trail much simpler; for instance, having a tool like Encord that natively supports DICOM and logs the history of how annotations were created, including time stamps and sequential recording, will help you keep an audit trail for each individual label. In addition to achieving an ISO 13485 certification for its QMS, the company must provide the Notified Body with its Technical Files to achieve CE approval under the MDR. The Technical Files is a collection of documents (also hundreds of pages long) designed to show, via real-world evidence, that a medical device meets the necessary safety and performance requirements. It contains all testing reports dating back to the conception of the technology. The Technical Files also contains marketing material, such as website copy, product descriptions, and manuals, but the majority of its documents are related to the performance of the healthcare software, including the medical model. You must show that the machine learning model performs its intended use at a certain threshold for the intended population and support these claims with real-world evidence. Within these technical documents, you’ll also need to show records of how you built your models and performed your testing. As much as possible, you’ll want to be sure these processes and procedures were in place, documented, and followed from the beginning of AI development. Interestingly, the Technical Files don’t actually contain the codebase used to write algorithms, nor does it contain the datasets used to train, validate, and test model performance. It’s a document log that details how the AI was developed rather than a collection of the material that went into making the AI. You’ll need to include design specifications that detail acceptable functionality as well as the measures taken to ensure the device works as intended. You’ll also need to detail the system architecture, including how this AI interacts with other systems that might be found in its intended use environment. When it comes to devise performance, you’ll have to show that the model achieves its intended purpose by reporting on metrics such as accuracy, sensitivity, specificity, and recall rate. The Technical Files also contain the Clinical Evaluation Report. This report details all the performance testing done during research, development, and pre-market release to show that your healthcare software adds additional clinical benefits, such as improving the speed or accuracy of diagnoses. To determine and justify the threshold for the performance of the model, you need to include a literature review in which you perform a meta-analysis about equivalent technology and medical practices. In this review, you’ll establish the performance level for state-of-the-art technology and provide context for showing that your technology goes beyond the existing state-of-the-art options and for demonstrating that the medical benefits of doing so outweigh any potential risks of using AI. You’ll prove these claims with a clinical investigation that reports and explains software results, including the results of the model’s performance. It’s worth noting that the report requires a study containing clinical data but not the conduction of clinical trials. An AI company can partner with research hospitals to conduct trials, but for most companies, a retrospective analysis is faster, cheaper, and more practical. In a retrospective analysis, your company can obtain previously annotated medical data and compare the notes and predictions made by medical professionals against the predictions made by your diagnostic model. You should round out the report with a benefit-risk-analysis conclusion– which references any anticipated risks laid out in the Technical Files and Intended Use– and then make a recommendation for using the healthcare software. Taken as a whole, the clinical evaluation report ultimately serves to summarise the technology’s required performance benchmarks, risks, and benefits, clinical performance, compliance with general safety requirements, and limitations. In addition to the above documentation, the audit process also requires that the head of the company submit a signed Declaration of Conformity in which they assert that all aspects of production and testing conform to EU mandates. The Impact of European Regulatory Approval on Company Employees Remember that undergoing these audits can be a stressful time for your employees. As part of the QMS audit, Notified Body wants to ensure that your company is “living the QMS.” Because standard operating procedures aim to reduce errors, the auditor needs to ensure that a company hasn’t just written these procedures but that their employees actually follow them on the day-to-day. The Notified Body will send representatives to the company to ask questions about the information contained in the submitted documents. The company must declare which person is responsible for each task, and the representatives will individually interview these employees, asking questions like “How has this model been handed to production? How has this process been documented? Who had access to the data?” While the Notified Body officials won’t necessarily require complicated answers, they do expect that your employees will be able to provide commentary on the document trail, including information about who was involved in the product, where the data came from, and where it was stored, the type of tests that were conducted, and various other aspects of the technological development. The Notified Body is looking to ensure there are no deviations, known as “nonconformities,” between what is done in practice and how it should be done according to your standard operating procedure. When it comes to the QMS, companies should aim to “do what they document, and document what they do.” The End of the CE Approval Process Once the Notified Body confirms that the software passes the essential requirements of the Medical Regulation Directive, your company will receive a Declaration of Conformity for the product. You will have to display the CE mark on your product before you can release it on the market. Make sure to follow the guidelines specific to your product type. Congratulations! You can now market and sell your medical diagnostic model throughout the EU! Conclusion: A Quick Summary of the EU MDR CE Approval Process for Healthcare AI Develop procedures for data collection and model development that align with QMS best practices. Ensure that other aspects of quality management needed for ISO 13485 certification– employee qualifications, supplier evaluations, and external software tests– are in place. (If possible, obtain validation reports from suppliers to lighten the internal workload required.) Consider working with a regulatory approval consultant or connecting with other startups in the medical AI ecosystem to build regulatory and audit know-how. Establish the intended use for your medical device Determine the device classification for your healthcare software Select an approved Notified Body to perform the conformity assessment Prepare the documentation for the QMS audit and prepare employees for onsite QMS checks. Undergo onsite visits and QMS audits. Receive ISO 13485 certification Prepare the documentation for the Technical File, including the Clinical Evaluation Report, and submit it Sign and submit the Declaration of Conformity Undergo Technical Files audit by Notified Body Following approval, adhere to the requirements for affixing the CE mark to your device. Special thanks to Leander Maerkisch, Founder and Managing Director of Floy, for providing insight and expertise to this article. Encord is a medical imaging annotation tool and active learning platform for computer vision. It is fully auditable and features SDK and API access, making it the perfect annotation tool for building CE-compliant diagnostic models. Get a demo of the platform to understand more about how Encord can help you.
Nov 11 2022
8 M
How to Structure QA Workflows for Medical Images
When building any computer vision model, machine learning teams need high-quality datasets with high-quality annotations to ensure that the model performs well across a variety of metrics. However, when it comes to building artificial intelligence models for healthcare use cases, the stakes are even higher. These models will directly affect individual lives. They need to train on data annotated by highly skilled medical professionals who don’t have much time to spare. They’ll also be held to a high scientific and regulatory standard, so to get a model out of development and into production, ML teams need to train it on the best possible data with the best possible annotations. That’s why every computer vision company–but especially those building medical diagnostic models– should have a quality assurance workflow for medical data annotation. Structuring a quality assurance workflow for image annotation requires putting processes in place to ensure that your labeled images are of highest possible quality. When it comes to medical image annotation (whether for radiology modalities or any other use case) , there are a few additional factors to consider when structuring a QA workflow. If you take these considerations into account when building your workflow and have the framework for your workflow in place before you begin the annotation process, then you’ll save time at the later stages of model development. Because medical image annotation requires medical professionals, annotation can be a costly part of building medical AI models. Having a QA workflow for image annotation before beginning model development can help a company to budget accordingly and reduce the risk of wasting an annotator’s time at the company’s expense. Step 1: Select and Divide the Datasets Medical models need to train on vast amounts of data. Your company will need to source high-quality training data while carefully considering the amount and types of data required for the model to perform well on its designated task. For instance, some tumors are rarer than others. However, the model needs to be able to classify rare tumors should it come across them “in the wild,” so the sourced data must contain enough examples of these tumors to learn to classify them accurately. Before you begin building your QA workflow, a portion of the data needs to be separated from the total data collected. This fraction becomes the test data– the never-before-seen data that you’ll use after the training and validation stages to determine if your model meets the performance threshold required to be released into clinical settings. This data should not be physically accessible to anyone on the machine learning or data engineering teams because when the time comes to obtain regulatory approval, the company will have to run a clinical study, and doing so will require using untouched data that has not been seen by the model or anyone working on it. Ideally, this test data will be copied onto a separate hard drive and kept in a separate physical location so as to ease the burden of showing compliance during the regulatory approval process. When building medical imaging models, you’ll also need to think carefully about the amount and types of annotations required to train the model. For instance, for those rare tumors, you need to decide how many examples need to be labeled, how often annotators will label them, and how annotators will categorize them. Your company might source millions of mammograms or CT scans, but, in reality, medical professionals won’t have enough time to label all those pieces of data, so you’ll have to make a decision about how to schedule the annotation process.. To do so, you’ll have to decide on an amount of representative data and split that data into a training set and a validation set. However, before you split the data, you’ll also need to decide how many times each piece of data will be labeled. By computing a consensus, you ensure that you are not modeling a single annotator. Step 2: Prepare to Annotate with Multiple Blinds In medical imaging, single labeling is not sufficient. The images need multiple labels by different labellers just like scans need to be read by multiple doctors during clinical practice. In most European and North American countries, double reading is standard practice: each medical image is read by at least two radiologists. At a minimum, your validation set will need to be double labeled. That means that different annotators will need to label the same piece of data. Furthermore, you may want to let annotators label the same data multiple times. By doing so, you can compute the inter and intra reader agreement. Of course, having multiple annotators is costly because these annotators are medical professionals– usually radiologists– with a significant amount of experience. The majority of the data, say 80 percent, will fall into the training data set. The cost and time constraints associated with medical image annotation generally mean that the training data is often only single labeled, which enables the model to begin training more quickly at less cost. However, the remaining data, which makes up the validation set, will be used to evaluate the model's performance after it completes its training. Most companies should aim to secure additional labels for the validation data. Having five or so annotators labeling each image will provide enough opinions to ensure that the predictions of the model are correct. The more opinions you have, the less the model will be biased towards a specific radiologist’s opinion and generalize better on unseen data. This division of labor should be determined when setting up the annotation pipeline. The annotators don’t know how many times a piece of data is being labeled, and ideally the labeling is always completed blind. They shouldn’t talk to one another or discuss it. When working in a hospital setting, this confidentiality isn’t always guaranteed, but when working with a distributed group of radiologists, the double blind remains intact and uncompromised. Step 3: Establish the Image Annotation Protocol Now that you’ve collected and divided the data, you’ll need to establish the labeling protocol for the radiologists. The labeling protocol provides guidelines for annotating “structures of interest”– tumors, calcifications, lymph nodes, etc.– in the images. The correct method for labeling these structures isn’t always straightforward, and there is often a trade-off between what is done in clinical routine and what is needed for training a machine learning. For instance, let’s say you have a mass– a dense region is the breast for example. This mass can be round but it can also be star shaped. The annotator needs to know whether they should circle the mass or closely follow the outline. That decision depends on what the AI system will need to do in a clinical setting. If you’re training a system that only has to detect whether a mass is present, then a loose annotation might be sufficient. However, if the system is trying to discriminate between masses of different shapes, then you’ll likely need to segment it very carefully by following its exact outline. Often, irregular shaped masses tend to be signs of more aggressive cancers, so the machine will definitely need to be able to identify them. Another example is calcification in medical imaging, which looks like salt and pepper noise on a medical image. How should that be annotated? A box around all the grains? A circle around each grain? A large bounding box means a compromise for the machine’s learning because it contains both calcifications and normal tissue, but it’s also unreasonable to ask doctors to annotate hundreds of small dots. You’ll need to detail what annotators should do in this situation in the labeling protocol. The same goes for encountering other objects– such as pacemakers and breast implants– in a scan. If annotators need to label these objects, then you must instruct them to do so. A member of the machine learning team and someone with a clinical background should produce the labeling protocol together because different subject-matter experts think about these things differently. Remember that doctors don’t think about distinguishing a pacemaker from a tumor. They have years of experience and the ability to think critically, so to them it seems ridiculous that someone might mistake a pacemaker for a cancerous tumor. However, models can’t reason: they’ll only learn what the labels specifically point out to them in the medical images. Often, machine learning teams need to explain that to the radiologists. Otherwise, doctors might not understand why it matters if they leave a pacemaker unlabelled or circle an irregular shaped mass in one image and outline it in the next. Be as explicit and exhaustive as possible. Annotation is a tedious and time consuming task, so labelers will understandably look for ways to cut corners unless you instruct them not to. Provide them with a labeling protocol manual that is precise but not overly lengthy. Include some pictures of good annotations and poor annotations as examples of what to do and what not to do. Then onboard them with a webinar session in which you share examples and demo the annotation platform so the labellers know what to expect and how to annotate within the platform. Without a detailed labeling protocol, labellers may produce inconsistent labels. A common mistake is mixing up left and right when asked to annotate a specific structure, e.g. “label the left lung.” And loose annotations– circling rather than following the outline– often occur simply out of habit. Step 4: Practice Medical Image Annotation on a Handful of Samples DICOM images contain a wealth of information that enables the best possible diagnosis of a patient. However, labeling volumetric images, such as CT or MRI scans, is challenging. Encord’s DICOM Annotation Tool was designed in close collaboration with medical professionals, so unlike other existing DICOM viewers, it allows for seamless annotations and the building of a data pipeline to ensure data quality. Most existing data pipeline tools can’t represent the pixel intensities required for CT and MRI scans whereas our platform provides accurate and truthful displays of DICOMs. While some of our competitors convert DICOMs to other formats (e.g. PNGs, videos), we allow users to work directly on DICOMs, so that nothing is lost in conversion. By providing annotators with functions like custom windowing and maximum intensity projection, we’re enabling them to work similar to what they are used to in clinical practice, so that they can accurately assess images without the interference of shifting data quality. DICOM metadata in Encord Volumetric images contains many slices and take a lot of time to investigate. Encord’s tool also supports maximum intensity projection in which users can collapse multiple slices into one plane layer, providing them with the opportunity to review the image from a different perspective– a perspective that might reveal findings otherwise missed. All of these features and more should help annotators better master the labeling protocol and produce high-quality medical image annotations more efficiently. Providing the right tools will help your annotators do the best possible job in the least amount of time. However, regardless of the tools used, before deploying the training data for annotation, you should provide each radiologist a handful of samples to annotate and then have a meeting with them, either as a group or individually, to discuss how they think it went. Work with a clinical expert to review that handful of samples to determine whether the labels achieve the high quality needed for training the machine learning models and algorithms. Compare the data samples to one another to determine whether one annotator performs significantly better or worse than the others. Consider questions like: Does one annotator label fewer structures than the others? Does another draw loose bounding boxes? Some variation is expected, but if one annotator differs significantly from the others, you may have to meet with them privately to align expectations. Even with a labeling protocol, closely reviewing these handful of samples is essential for catching misalignments in thinking before releasing too much of the data. Remember, doctors think like doctors. If a patient's scan reveals 13 cancerous tumors in the liver, a doctor might only circle seven, because, in a clinical setting, that would be enough to know the patient has cancer and needs treatment. However, machine learning teams need to make sure that the doctor labels all 13 because the model will encounter those additional six and become penalized by the missing label. Missed annotations will make estimating the true model performance impossible, so machine learning teams need to help doctors understand why they need to perform exhaustive labeling, which is more time-consuming and differs from their routine clinical work. Different annotators will have different thresholds for what they think should be annotated, so you’ll need the input of a clinical partner to determine what should have been annotated. Uncertainty always exists in medical image evaluation, so you’ll need to calibrate the annotators, telling them to be more or less sensitive in their thresholds. Step 5: Release the First Batch of Images for Labeling In Your Annotation Tool Once all the radiologists understand the labelling expectations, it’s time to release a first batch of images for annotation. For the first batch, release about a third of the data that needs to be annotated. Set a timeline for completing the annotations. The timeline will depend on your company’s time constraints. For instance, if you are working towards attending a conference, you’ll need to train the model sooner rather than later, and you’ll want to shorten the timeline for annotations. Someone from the machine learning team and the clinical partner should oversee and review the annotation process. That means you need to build time in for quality control. Reviewing annotations takes time, and, ideally, you’ll keep a record of each annotator’s labelling quality, so that you have monthly or weekly statistics that show how well each radiologist labelled an image compared to the ground truth or to a consensus. When it comes to medical image annotation, establishing a ground truth requires finding information about the patients’ outcomes, which can be tricky. For instance, if three doctors read an image of a mass as non-cancerous, but a biopsy later revealed that the mass was cancerous, then the ground truth for that image is actually “cancerous.” Ideally, when you collect your data, you’ll receive clinical data along with the DICOM image that provides information about the patient’s treatment and outcomes post-scan, allowing for the establishment of a ground truth based on real-world outcomes. Because Encord platform supports DICOM metadata, if the clinician and radiographer have collected this metadata, using Encord will enable you to seamlessly access important information about the patient’s medical condition, history, and outcomes. In the case that no such clinical information is available, a consensus derived from annotations will have to serve as a proxy for the ground truth. A consensus occurs when a group of radiologists read the same scan, and arrive at an agreement, usually via a majority voting, about the finding. That finding then serves as the ground truth for the data. However, in a clinical setting, doctors take different approaches to determining consensus. That’s why Encord’s platform provides a variety of features to help compute a consensus. It includes templates for maturity voting for any amount of annotators. It also features weighting so that a more experienced medical professional’s annotations would be given greater consideration than a more junior-level one. When disagreements about an image arise, Encord’s platform enables an arbitration panel in which the image is sent to an additional, more experienced professional to decide the consensus. Having a variety of approaches built into the platform is especially useful for regulatory approval because different localities will want companies to use different methods to determine consensus. Within this part of the QA workflow, you should also build in a test of intra-rater reliability, in which each reviewer receives a set of data which contains the same image multiple times. The goal is to ensure that the rater performs consistently across time. This continuous monitoring of the raters answers important questions such as: Does the reviewer perform as well in the morning as in the evening? Does the reviewer perform poorly on the weekend compared to the weekdays? Regulatory processes for releasing a model into a clinical setting expect data about intra-rater reliability as well as inter-rater reliability, so it’s important to have this test built into the process and budget from the start. Step 6: Release the Rest of the Data for Annotation and Implement Continuous Labeling Monitoring If the review of the first batch of annotations went well, then it’s time to release the rest of the data to the annotators. In general, if there’s a strict timeline or a concrete amount of data, a company will release the rest of the data in distinct batches and provide deadlines for labelling each batch. Otherwise, most companies will implement a continuous labeling stream. When a company has access to an ongoing flow of data from different manufacturers, a continuous labeling stream is the best strategy. Continuous labeling streams require continuous labeling monitoring, and continuous labeling monitoring is great because it provides interesting and important insights about the labels and the data itself. Encord’s DICOM annotation tool provides machine learning teams with access to important metadata that may have an impact on annotations and machine performance. DICOM data contains information about the state of the machine– its electrical current, X-ray exposure, angle relevant to the patient, surrounding temperature, and more.The team can also break the data broken down by country, costs, and manufacturer. All of this information is important because it contributes to the appearance of the image, which means that the metadata has an impact on model performance. For instance, if labellers consistently mislabel images from a certain manufacturer or hospital’s set of data, then machine learning teams might realize that the image quality from that device isn’t as good as the image quality from other sources or that images taken on a particular day suffered from an odd device setting. An image from one manufacturer could look very different from the image of another. If they have only 10 percent of images from Siemen devices, they know that they’ll need to gather more Siemen images to ensure that the model can predict well on images captured on that brand of device. The same goes for medical images captured with newer models of devices vs. old ones. Geographic regions matter, too. Manufacturers tune their devices based on where they’ll be deployed; for instance, contrast settings differ between the US and Europe. Using images from a variety of geographies and manufacturers can help you work against imbuing the machine learning model with bias and to ensure that it generalizes properly. With the onset of continuous labeling and continuous monitoring, we’ve reached the end of the steps for building a quality assurance workflow for medical image data labeling. The workflow might seem granular in its detail, but a surprising amount of common mistakes occur in medical image annotation when there isn’t a strong workflow in place. Building a Tool for Medical Annotations Means Understanding Medical Professionals There are six, equally important steps to structuring a quality assurance workflow for medical image annotation: Select and divide the dataset Prepare to annotate with multiple blinds Establish the labeling protocol Practice medical image annotation on a handful of samples Release the first batch of images for annotation Release the rest of the data for annotation and implement continuous labeling monitoring However, having the right tools that fit seamlessly into the day-to-day lives of medical professionals is an equally important part of structuring a quality assurance workflow for image annotation. Encord’s DICOM annotation tool was built in collaboration with clinicians, so it enables medical professionals to navigate and interact with images in the same way that they do in their clinical workflow. We recognize that radiologists and other medical professionals are busy people who have spent years building certain domain expertise and skills. Our annotation tool mimics and integrates with the clinical experience. Radiologists spend most of their days in dark rooms, using high-resolution grayscale monitors to look at digital images. That’s why our tool supports darkmode, preventing radiologists from encountering a green or white interface during their clinical routine. We also designed a viewer that supports the same method and handling for looking through a volume of image slices, so that they can rely on the muscle memory that they’ve developed through years of using clinical tools. That’s why we also support hanging protocol. After years of hanging scans up on walls, radiologists are used to seeing them displayed in a certain way. For instance, when reading a mammography, they want to see both breasts at the same time to compare for symmetry and features intrinsic to that particular patient. Rather than ask the radiologist to change for the digital age, we’ve changed the tool to orient images in the way that makes the most sense for their profession. Our platform, interface, and mouse gestures (including windowing!) were all designed with the clinical experience in mind. Interested in learning more about Encord’s DICOM Annotation Tool? Reach out for a demo today.
Nov 11 2022
12 M
Pain Relief for Doctors Labelling Data
I started academically in physics, but dropped out(sorry, took a leave of absence) of my PhD in the first year and did a long stint in quantitative finance. So out of all the possible topics for my first peer-reviewed published paper: portfolio optimisation, dark matter signatures, density functional theory, I ended up with the topic of…drawing rectangles on a colonoscopy video. I didn’t think it would come to this, but here we are. In reality though, drawing boxes on a colonoscopy video is one of the most interesting problems I have worked on. The purpose of this post is to review the paper we (including my cofounder Ulrik at Encord) recently published on this topic: “Novel artificial intelligence-driven software significantly shortens the time required for annotation in computer vision projects”. The paper, in the journal Endoscopy International Open, can be found here. This was cowritten with the deft and patient assistance of our collaborators at King’s College London, Dr Bu Hayee and Dr Mehul Patel. To convince you to keep reading after already having seen the words “annotation” and “colonoscopy”, we can for starters state that the field of gastroenterology is immensely important for human wellbeing. This is both in terms of cancer incidence and everyday chronic ailments. From cancer.org: In the United States, colorectal cancer is the third leading cause of cancer-related deaths in men and in women, and the second most common cause of cancer deaths when men and women are combined. It’s expected to cause about 52,980 deaths during 2021. Even more prevalent is inflammatory bowel disease (IBD). In 2015 around 3 million people in the US had been diagnosed with IBD, a condition associated with higher likelihood of respiratory, liver, and cardiovascular disease among others.¹ Add this to the litany of other conditions and symptoms encapsulated under the GI umbrella (acid reflux, indigestion, haemorrhoids, etc.) and we find that the scope (no pun intended) of GI knows few bounds with its effect on the population. But gastroenterology is also very important for the AI community. It is one of the early vanguards of commercial adoption of medical AI. Companies such as Pentax, FujiFilm, and Medtronic have all been part of the crop of medical device companies that are running into the field to build their own AI enabled scoping technology. These models can run live detection of polyps and act as a gastroenterologist’s assistant during a scoping procedure, sometimes even catching the doctor’s blind spots. Polyp detection in action Progress in this field will be a beacon to the rest of a skeptical medical community that AI is not just a playground for mathematicians and computer scientists, but a practical tool that directly matters in people’s lives. But, there is a problem. The Problem Unlike a machine learning model that is serving up a binge-y Netflix show to an unsuspecting attention victim (where the stakes of a mistake are that you end up watching an episode of Emily in Paris), getting a polyp detection wrong or mis-diagnosing ulcerative colitis has drastic implications for people’s health. The models that are developed thus need to be as foolproof as you can get in the machine learning world. This requires prodigious quantities of data. Empirically, models tend to require ever increasing amounts of data to combat stagnation in performance. Getting a model from 0% to 75% accuracy might require the same amount of data as 75% to 85% which requires the same as from 85% to 90% etc. To get over 99% accuracy, with the current methods and models we have, you need to throw a lot of data at the problem. The issue is that for a model to train from this data, it needs to be annotated. These annotations can only effectively be completed by doctors themselves, who have the expertise to correctly identify and classify the patient video and images. This is a massive drain on doctor time. A high accuracy endoscopy model might require one million annotated frames. Assuming a conservative estimate of 20 seconds per frame, including review from one or two other doctors, that’s 230 days of doctor time, about the number of working days in a year. That’s a doctor’s working year which is certainly better spent treating and caring for patients(and practicing their handwriting). This opportunity cost was the original motivation for starting Encord. We wanted to save valuable time for anyone that has to undergo the necessary evil of data annotation, with doctors being the most egregious case. And after building our platform, we wanted to see if it actually worked. So, we ran an experiment. The Experiment We decided to run a simple A/B test of our platform against the most widely used open sourced video annotation tool (CVAT). Openly available video annotation tools are difficult to come by, but CVAT stands out as a platform with one of the most active users and stars on GitHub. We set up a sample of data from an open source gastrointestinal dataset (the Hyper-Kvasir dataset) to perform the experiment. From the paper: Using a subsample of polyp videos from the Hyper-Kvasir dataset[7], five independent annotators were asked to draw bounding boxes around polyps identified in videos from the dataset. A test set of 25,744 frames was used. The experimental setup was: Each annotator would have two hours on Encord and two hours on CVAT The annotators would run through the data in the same order for both platforms and use any available feature from each platform Annotators could only submit frames that at the end of the process they had reviewed and were happy with At the end of the two hours, we would simply count the number of approved frames from each annotator on each platform The power of the Encord platform (termed CdV in the paper) lies in its ability to quickly train and use annotation specific models, but for the experiment no labels or models were seeded for the annotators. They could only use models that they trained themselves with the data they had annotated within the time limit of the experiment. Normally this would not be the case of course. If you are tagging hundreds of thousands of frames, you will already have models and intelligence to pull from, but we wanted to stack the deck as much against as us we could and have the annotators start cold. The Results The results were not close. From the paper: In the 120-minute project, a mean±SD of 2241±810 frames (less than 10% of the total) were labelled with CVAT compared to 10674±5388 with CdV (p=0.01). Average labelling speeds were 18.7/min and 121/min, respectively (a 6.4-fold increase; p=0.04) while labelling dynamics were also faster in CdV (p<0.0005; figure 2). The project dataset was exhausted by 3 of 5 annotators using CdV (in a mean time of 99.1±15.2 minutes), but left incomplete by all in CVAT. With CVAT, most annotators did not make it past the third video. Every single annotator was able to produce more labels with Encord than CVAT. What was the most encouraging was that the most senior doctor of the annotators, the one who had the least experience with any annotation software, got a 16x increase in efficiency from Encord. This was the exact target user we designed the platform for, so it was very encouraging to see these results pan out. It was a major win for the realisation of our hypothesis. Briefly, the reason Encord was more efficient was simply the automation of most of the labelling: Labellers were allowed to adopt their own labelling strategies with any functionality offered in each platform. With CVAT, this consisted of tools to draw bounding boxes and propagate them across frames using linear interpolation of box coordinates. With CdV, labellers had access to both hand labelling annotation tools and CdV’s embedded intelligence features. This embedded intelligence was composed of object tracking algorithms and functionality to train and run convolutional neural networks (CNNs) to annotate the data. Even with a completely cold start, Encord’s “embedded intelligence” automated over 96% of the labels produced during the experiment: With CdV, only 3.44%±2.71% of labels produced were hand drawn by annotators. The remainder were generated through either models or tracking algorithms. Thus with CdV far more labels were produced with far less initial manual input (Figure 3). Automated labels still required manual time for review and/or adjustment. For model generated labels, a mean of 36.8±12.8 minutes of the allocated annotator time was spent looking over them frame by frame and making corrections. The most interesting observation in my opinion was the acceleration of labelling rate under the Encord platform. For CVAT, label rate remained approximately constant for the duration of the experiment. With Encord, however, for every twenty minute interval on the platform, annotation speed increased by a median of 55%(!). Every label marginally informed the next. The hope is that with more labels and even larger projects, this effect will lead to a precipitous drop in the temporal (and financial) cost of creating training data sets. Conclusion While the results were favourable, we recognise there is a lot more to do. Polyp detection is a relatively simple annotation task, so while it is a costly tax on doctors, we realise there are even costlier ones that we need to address. Our software is designed to deal with arbitrarily complex labelling structures, but designing automation around this complexity is a tricky but interesting problem that we are working on. That being said, we showed that we could save doctors a bunch of time annotating their data. Give them intelligent but accessible tools, and they will save their own time. With that, the bottleneck to the next iteration of medical AI does not need to be lack of training data. If you want to chat about annotation or AI feel free to reach out to me at eric.landau@cord.tech. ¹https://www.cdc.gov/ibd/data-statistics.htm
Nov 11 2022
7 M
Software To Help You Turn Your Data Into AI
Forget fragmented workflows, annotation tools, and Notebooks for building AI applications. Encord Data Engine accelerates every step of taking your model into production.