Back to Blogs
Contents
Leveraging LLM-based Marketing Analytics Copilots and Agents
Unleashing Potential: POCs to a Promising Future in Agentic AI
Multimodal RAG for Video
AI-Powered Fundraising for Conservation: Transforming Grant Discovery
Personalized Video Ads at Scale with GenAI
Encord Blog
Key Insights from the Inaugural AI After Hours
September 27, 2024
|5 mins
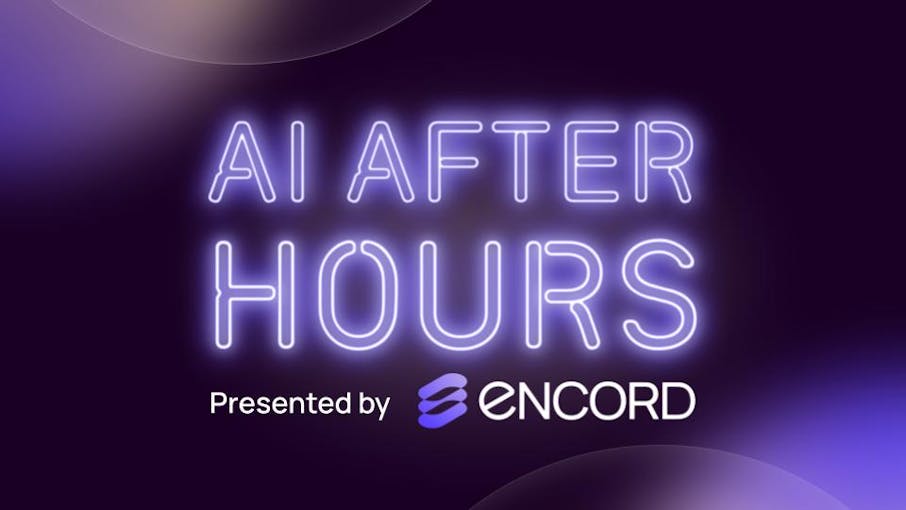
On September 24, Encord hosted its first AI After Hours in San Francisco, featuring five talks from AI practitioners working on applications across industries.
Here are the key takeaways from each presentation:
Leveraging LLM-based Marketing Analytics Copilots and Agents
Presenter: Sai Kumar Arava, Machine Learning Manager at Adobe.
- LLMs and their use in marketing analytics: LLMs, like GPT-4 and Llama, are transforming marketing analytics by allowing natural language queries and automating tasks such as SQL generation, tabular analysis, and document summarization. This shift reduces reliance on technical teams and speeds up workflows.
- LLM challenges: Key issues include hallucination (inaccurate data generation), context length limitations, poor mathematical accuracy (especially for numerical tasks), and interpretability issues, all of which need to be addressed for enterprise-grade applications like marketing analytics.
- Fine-tuning and specialized agents: Fine-tuning LLMs for specific domains (e.g., marketing, legal, healthcare) is critical for improving performance and accuracy. Techniques like LoRA (Low-Rank Adaptation) are popular for efficient fine-tuning.
- AI agents for end-to-end automation: AI agents are evolving to automate entire marketing processes, from dynamic dashboard generation to customer service. They leverage planning and tool orchestration techniques to complete tasks with minimal human intervention.
- Sophisticated agent architectures: AI agent architectures are increasingly sophisticated, incorporating long-term memory, personalization APIs, and orchestration layers for tool and agent management. These advanced architectures help agents handle complex workflows across various sectors.
- Performance and scalability advancements: LLMs have made significant strides in performance and scalability, particularly in multi-agent and tool orchestration environments.
- Ethical and safety considerations: As AI agents become more prevalent, ensuring transparency, safety, and alignment with ethical guidelines is crucial to prevent unintended consequences. Human-AI collaboration remains necessary for critical decision-making.
Unleashing Potential: POCs to a Promising Future in Agentic AI
Presenter: Meghana Puvvadi, Director of Engineering for AI/ML Enterprise Assistants at NVIDIA.
- Four pillars of agentic systems: Memory (retaining user preferences), tools (API access for actions like code generation and search), planning (LLMs handling complex tasks), and reasoning (breaking down tasks into logical steps).
- Key use cases: Simple, deterministic scenarios such as new hire onboarding and code assistance serve as ideal starting points for agentic AI implementation. More complex tasks, like supply chain management and customer interaction, benefit from agents employing multi-path reasoning.
- Decoupled architecture: Building AI applications with decoupled components—memory, tool invocation, planning, and reasoning—allows flexibility in swapping LLMs and adapting to new models as they emerge.
- Challenges and considerations: Key challenges include managing costs and latency due to frequent LLM calls, securing data with proper access controls, and continuously updating data to keep AI models relevant.
- Security and permissions: With easier access to information through agents, companies need to ensure strong permission management and avoid exposing sensitive information unintentionally.
- Multi-agent architectures: These architectures are evolving rapidly, with different models such as layered, decentralized, and centralized systems, each suited for varying levels of interaction and autonomy in tasks.
Multimodal RAG for Video
Presenter: Anup Gosavi, CEO and co-founder of VideoDB.
- Multimodal RAG overview: Current multimodal RAG (Retrieval-Augmented Generation) primarily supports unimodal output (text) despite inputs being multimodal (video, images, text). There's a growing need for more comprehensive video outputs beyond short clips.
- Video query challenges: Video retrieval demands complex processing, including transforming videos into images, identifying objects and actions, and managing multiple steps to compile meaningful results.
- Limitations of multimodal models: Existing multimodal models often require manual compilation and editing, leading to high costs and latency in video processing. Additionally, the large token requirements for processing video data can quickly become unmanageable and expensive.
- RAG benefits: RAG enables pre-processing of video content tailored to specific use cases, resulting in improved indexing, retrieval, and lower latency. By optimizing the retrieval process, developers can manage costs more effectively as video data scales.
- Video RAG architecture: The proposed architecture involves a systematic approach to handle video inputs, including audio processing, image extraction, and text transcription, leading to efficient data storage and retrieval. The emphasis is on the need for effective ranking of search results to ensure relevance and efficiency.
- Use cases: Potential applications include generating video answers in chatbots, real-time content modification, and personalized highlights from events. The video content should be treated as data to facilitate dynamic access to various modalities.
AI-Powered Fundraising for Conservation: Transforming Grant Discovery
Presenter: Prajakta Pardeshi, Senior Machine Learning Scientist at Walmart Global Tech.
- AI chatbot for fundraising: The chatbot employs a fine-tuned BERT model to analyze user inputs, extracting key features such as funding amounts and project details to identify relevant donors or grants.
- Data pipeline and embedding: A sophisticated data pipeline leverages web-scraped donor information to create embeddings for both user queries and potential grants, enabling efficient donor matching.
- Grant diversification: The system incorporates a diversification strategy to ensure a varied selection of grants based on factors such as funding amount and geographical relevance, enhancing the breadth of available options.
- Future enhancements: Plans include transitioning to vector indexing for improved data storage and querying, as well as exploring advanced algorithms for similarity matching to boost the overall efficiency of donor discovery.
Personalized Video Ads at Scale with GenAI
Presenter: Shradha Agrawal. Engineering Manager for GenAI and Computer Vision at Adobe.
- The need for hyper-personalization: In today's marketing landscape, the demand for hyper-personalized video ads is crucial, as traditional one-size-fits-all approaches no longer effectively target specific audiences.
- GenAI tool development: Adobe's generative AI tool empowers marketers to create personalized video ads efficiently by adapting a single marketing video to match individual customer preferences through target images and text prompts.
- Tool overview: The system utilizes stable diffusion models enhanced with a temporal attention module to create personalized video content. The model allows for fine-tuning with just one source video, streamlining the creation process.
- Inverted mask and feature flow: The system incorporates an inverted mask to specify target object placement in the video and employs feature flow for temporal consistency across frames, improving the visual coherence of generated videos.
- Performance metrics: Generated videos are evaluated using CLIP and DINO scores, measuring alignment with target text and images, respectively. Results indicate that the tool outperforms existing state-of-the-art methods, particularly in scenarios requiring shape changes.
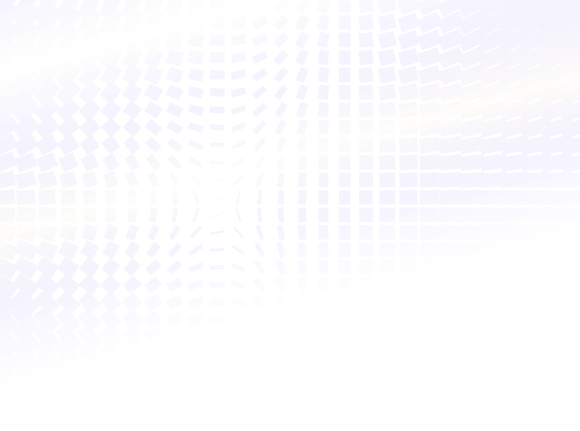
Power your AI models with the right data
Automate your data curation, annotation and label validation workflows.
Book a demoWritten by

Ulrik Stig Hansen
Explore our products