Encord Blog
Encord is the world’s first fully multimodal AI data platform
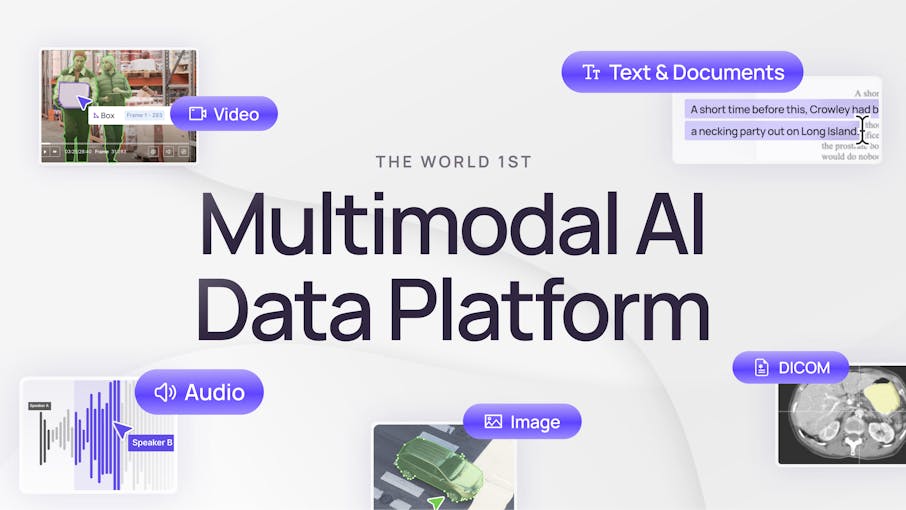
Encord is the world’s first fully multimodal AI data platform
Today we are expanding our established computer vision and medical data development platform to support document, text, and audio data management and curation, whilst continuing to push the boundaries of multimodal annotation with the release of the world's first multimodal data annotation editor.
Encord’s core mission is to be the last AI data platform teams will need to efficiently prepare high-quality datasets for training and fine-tuning AI models at scale. With recently released robust platform support for document and audio data, as well as the multimodal annotation editor, we believe we are one step closer to achieving this goal for our customers.
Key highlights:
- Introducing new platform capabilities to curate and annotate document and audio files alongside vision and medical data.
- Launching multimodal annotation, a fully customizable interface to analyze and annotate multiple images, videos, audio, text and DICOM files all in one view.
- Enabling RLHF flows and seamless data annotation to prepare high-quality data for training and fine-tuning extremely complex AI models such as Generative Video and Audio AI.
- Index, Encord’s streamlined data management and curation solution, enables teams to consolidate data development pipelines to one platform and gain crucial data visibility throughout model development lifecycles.
Multimodal Data Curation & Annotation
AI teams everywhere currently use 8-10 separate tools to manage, curate, annotate and evaluate AI data for training and fine-tuning AI multimodal models. It is time-consuming and often impossible for teams to gain visibility into large scale datasets throughout model development due to a lack of integration and consistent interface to unify these siloed tools.
As AI models become more complex, with more data modalities introduced into the project scope, the challenge of preparing high-quality training data becomes unfeasible. Teams waste countless hours and days in data wrangling tasks, using disconnected open source tools which do not adhere to enterprise-level data security standards and are incapable of handling the scale of data required for building production-grade AI.
To facilitate a new realm of multimodal AI projects, Encord is expanding the existing computer vision and medical data management, curation and annotation platform to support two new data modalities: audio and documents, to become the world’s only multimodal AI data development platform.
Offering native functionality for managing and labeling large complex multimodal datasets on one platform means that Encord is the last data platform that teams need to invest in to future-proof model development and experimentation in any direction.
Launching Document And Text Data Curation & Annotation
AI teams building LLMs to unlock productivity gains and business process automation find themselves spending hours annotating just a few blocks of content and text. Although text-heavy, the vast majority of proprietary business datasets are inherently multimodal; examples include images, videos, graphs and more within insurance case files, financial reports, legal materials, customer service queries, retail and e-commerce listings and internal knowledge systems.
To effectively and efficiently prepare document datasets for any use case, teams need the ability to leverage multimodal context when orchestrating data curation and annotation workflows.
With Encord, teams can centralize multiple fragmented multinomial data sources and annotate documents and text files alongside images, videos, DICOM files and audio files all in one interface.
Uniting Data Science and Machine Learning Teams
Unparalleled visibility into very large document datasets using embeddings based natural language search and metadata filters allows AI teams to explore and curate the right data to be labeled.
Teams can then set up highly customized data annotation workflows to perform labeling on the curated datasets all on the same platform. This significantly speeds up data development workflows by reducing the time wasted in migrating data between multiple separate AI data management, curation and annotation tools to complete different siloed actions.
Encord’s annotation tooling is built to effectively support any document and text annotation use case, including Named Entity Recognition, Sentiment Analysis, Text Classification, Translation, Summarization and more. Intuitive text highlighting, pagination navigation, customizable hotkeys and bounding boxes as well as free text labels are core annotation features designed to facilitate the most efficient and flexible labeling experience possible.
Teams can also achieve multimodal annotation of more than one document, text file or any other data modality at the same time. PDF reports and text files can be viewed side by side for OCR based text extraction quality verification.
Launching Audio Data Curation & Annotation
Accurately annotated data forms the backbone of high-quality audio and multimodal AI models such as speech recognition systems, sound event classification and emotion detection as well as video and audio based GenAI models.
We are excited to introduce Encord’s new audio data curation and annotation capability, specifically designed to enable effective annotation workflows for AI teams working with any type and size of audio dataset. Within the Encord annotation interface, teams can accurately classify multiple attributes within the same audio file with extreme precision down to the millisecond using customizable hotkeys or the intuitive user interface.
Whether teams are building models for speech recognition, sound classification, or sentiment analysis, Encord provides a flexible, user-friendly platform to accommodate any audio and multimodal AI project regardless of complexity or size.
Launching Multimodal Data Annotation
Encord is the first AI data platform to support native multimodal data annotation. Using the customizable multimodal annotation interface, teams can now view, analyze and annotate multimodal files in one interface. This unlocks a variety of use cases which previously were only possible through cumbersome workarounds, including:
- Analyzing PDF reports alongside images, videos or DICOM files to improve the accuracy and efficiency of annotation workflows by empowering labelers with extreme context.
- Orchestrating RLHF workflows to compare and rank GenAI model outputs such as video, audio and text content.
- Annotate multiple videos or images showing different views of the same event. Customers would otherwise spend hours manually
Customers with early access have already saved hours by eliminating the process of manually stitching video and image data together for same-scenario analysis. Instead, they now use Encord’s multimodal annotation interface to automatically achieve the correct layout required for multi-video or image annotation in one view.
AI Data Platform: Consolidating Data Management, Curation and Annotation Workflows
Over the past few years, we have been working with some of the world’s leading AI teams such as Synthesia, Philips, and Tractable to provide world-class infrastructure for data-centric AI development. In conversations with many of our customers, we discovered a common pattern: teams have petabytes of data scattered across multiple cloud and on-premise data storages, leading to poor data management and curation.
Introducing Index: Our purpose-built data management and curation solution
Index enables AI teams to unify large scale datasets across countless fragmented sources to securely manage and visualize billions of data files on one single platform. By simply connecting cloud or on prem data storages via our API or using our SDK, teams can instantly manage and visualize all of your data on Index. This view is dynamic, and includes any new data which organizations continue to accumulate following initial setup.
Teams can leverage granular data exploration functionality within to discover, visualize and organize the full spectrum of real world data and range of edge cases:
- Embeddings plots to visualize and understand large scale datasets in seconds and curate the right data for downstream data workflows.
- Automatic error detection helps surface duplicates or corrupt files to automate data cleansing.
- Powerful natural language search capabilities empower data teams to automatically find the right data in seconds, eliminating the need to manually sort through folders of irrelevant data.
- Metadata filtering allows teams to find the data that they already know is going to be the most valuable addition to your datasets.
As a result, our customers have achieved on average, a 35% reduction in dataset size by curating the best data, seeing upwards of 20% improvement in model performance, and saving hundreds of thousands of dollars in compute and human annotation costs.
Encord: The Final Frontier of Data Development
Encord is designed to enable teams to future-proof their data pipelines for growth in any direction - whether teams are advancing laterally from unimodal to multimodal model development, or looking for a secure platform to handle immense scale rapidly evolving and increasing datasets.
Encord unites AI, data science and machine learning teams with a consolidated platform everywhere to search, curate and label unstructured data including images, videos, audio files, documents and DICOM files, into the high quality data needed to drive improved model performance and productionize AI models faster.
Power your AI models with the right data
Automate your data curation, annotation and label validation workflows.
Get startedWritten by

Eric Landau
Explore our products