Contents
How Does Sora Work?
Technical overview of OpenAI’s Sora
Capabilities of Sora
Limitations of Sora
Safety Considerations of Sora
Noteworthy Text to Video Generation Models
Sora: Key Highlights
Encord Blog
OpenAI Releases New Text-to-Video Model, Sora
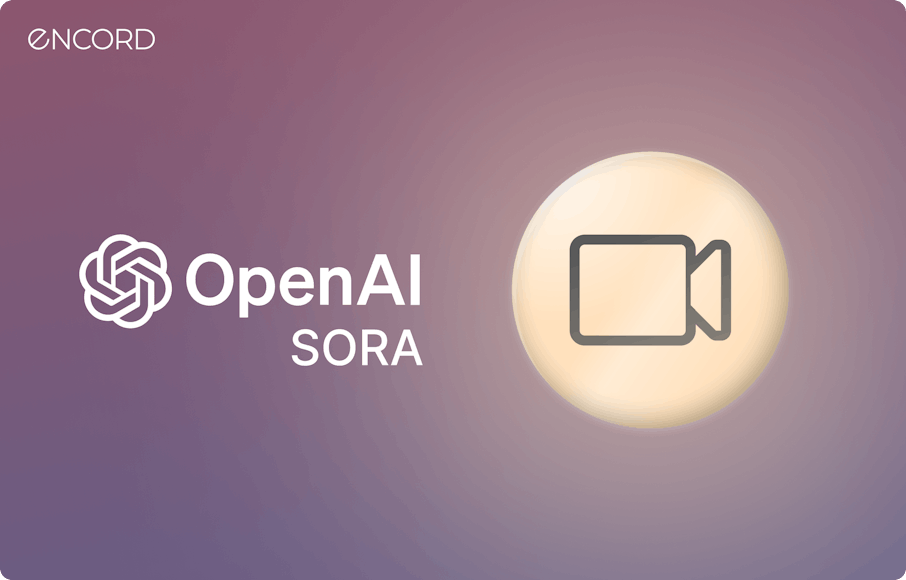
OpenAI has responded to the recent debut of Google's Lumiere, a space-time diffusion model for video generation, by unveiling its own creation: Sora. The diffusion model can transform short text descriptions into high-definition video clips up to one minute long.
How Does Sora Work?
Sora is a diffusion model that starts with a video that resembles static noise. Over many steps, the output gradually transforms by removing the noise. By providing the model with the foresight of multiple frames concurrently, OpenAI has resolved the complex issue of maintaining subject consistency, even when it momentarily disappears from view.
Similar to GPT models, Sora uses a transformer architecture. Images and videos are represented as patches, collections of smaller units of data. By representing the data in the same manner, OpenAI was able to train diffusion transformers on a wide range of data of different durations, resolutions, and aspect ratios.
Sora leverages the recaptioning techniques from DALL-E3 and as such, the model follows the user’s text instructions closely.
Technical overview of OpenAI’s Sora
OpenAI has released a few technical details on how the state-of-the-art diffusion model for video generation. Here are the key methodologies and features employed in Sora’s architecture.
Video Generated by OpenAI's Sora
Unified Representation for Large-Scale Training
Sora focuses on transforming visual data into a unified representation conducive to large-scale training of generative models. Unlike previous approaches that often concentrate on specific types of visual data or fixed-size videos, Sora embraces the variability inherent in real-world visual content. By training on videos and images of diverse durations, resolutions, and aspect ratios, Sora becomes a generalist model capable of generating high-quality videos and images spanning a wide range of characteristics.
Patch-Based Representations
Inspired by the use of tokens in large language models (LLMs), Sora adopts a patch-based representation of visual data. This approach effectively unifies diverse modalities of visual data, facilitating scalable and efficient training of generative models. Patches have demonstrated their effectiveness in modeling visual data, enabling Sora to handle diverse types of videos and images with ease.

Turning Visual Data into Patches
Video Compression Network
To convert videos into patches, Sora first compresses the input videos into a lower-dimensional latent space, preserving both temporal and spatial information. This compression is facilitated by a specialized video compression network, which reduces the dimensionality of visual data while maintaining its essential features. The compressed representation is subsequently decomposed into spacetime patches, which serve as transformer tokens for Sora's diffusion transformer architecture.
Diffusion Transformer
Sora leverages a diffusion transformer architecture, demonstrating remarkable scalability as video models. Diffusion transformers have proven effective across various domains, including language modeling, computer vision, and image generation. Sora's diffusion transformer architecture enables it to effectively handle video generation tasks, with sample quality improving significantly as training compute increases.

Scaling Transformers for Video Generation
Native Size Training for High-Quality Video Generation
Sora benefits from training on data at its native size, rather than resizing, cropping, or trimming videos to standardized dimensions. This approach offers several advantages, including sampling flexibility, improved framing and composition, and enhanced language understanding. By training on videos at their native aspect ratios, Sora achieves superior composition and framing, resulting in high-quality video generation.
Language Understanding and Text-to-Video Generation
Training Sora for text-to-video generation involves leveraging advanced language understanding techniques, including re-captioning and prompt generation using models like DALL·E and GPT. Highly descriptive video captions improve text fidelity and overall video quality, enabling Sora to generate high-quality videos accurately aligned with user prompts.
Capabilities of Sora
OpenAI’s Sora can generate intricate scenes encompassing numerous characters, distinct forms of motion, and precise delineations of subject and background. As OpenAI states “The model understands not only what the user has asked for in the prompt, but also how those things exist in the physical world.”
Here is an extensive list of capabilities of Sora that OpenAI demonstrated. This definitely says a lot about how powerful it is as a text-to-video tool for creating content generation and simulation tasks.
Prompting with Images and Videos
Sora's flexibility extends to accepting inputs beyond text prompts, including pre-existing images or videos.
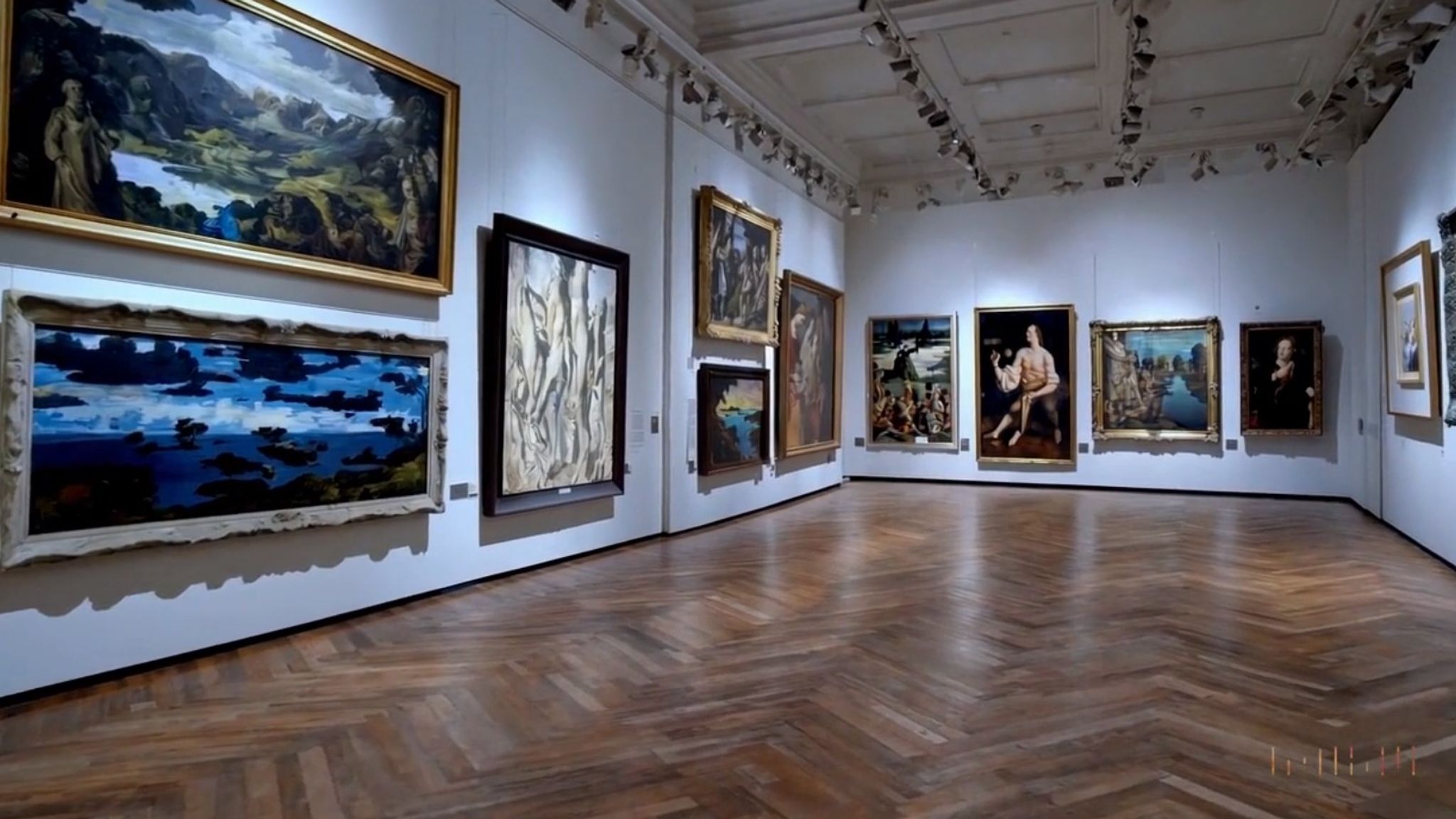
Glimpse of Prompt Generated Artwork of an Art Gallery by OpenAI's Sora
Animating DALL-E Images
Sora can generate videos from static images produced by DALL·E, showcasing its ability to seamlessly animate still images and bring them to life through dynamic video sequences.
Current techniques for animating images utilize neural-based rendering methods to produce lifelike animations. However, despite these advancements, achieving precise and controllable image animation guided by text remains a challenge, especially for open-domain images taken in diverse real-world environments. Models like AnimateDiff, AnimateAnything, etc have also demonstrated promising results for animating static images.
Extending Generated Videos
Sora is adept at extending videos, whether forward or backward in time, to create seamless transitions or produce infinite loops. This capability enables Sora to generate videos with varying starting points while converging to a consistent ending, enhancing its utility in video editing tasks.
Video-to-Video Editing
Leveraging diffusion models like SDEdit, Sora enables zero-shot style and environment transformation of input videos, showcasing its capability to manipulate video content based on text prompts and editing techniques.
Connecting Videos
Sora facilitates gradual interpolation between two input videos, facilitating seamless transitions between videos with different subjects and scene compositions. This feature enhances Sora's ability to create cohesive video sequences with diverse visual content.
Image Generation
Sora is proficient in generating images by arranging patches of Gaussian noise in spatial grids with a temporal extent of one frame, offering flexibility in generating images of variable sizes up to 2048 x 2048 resolution.
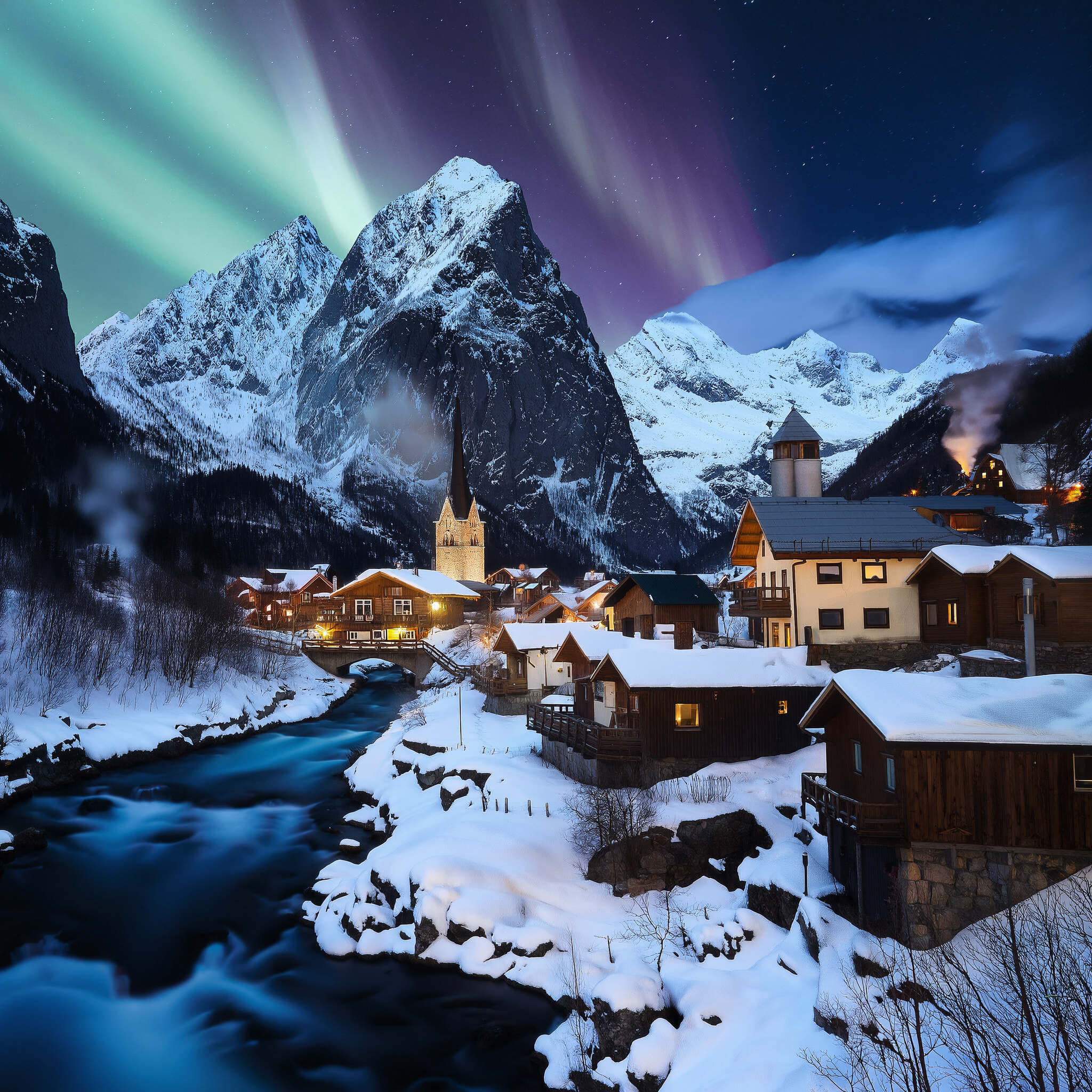
Photorealistic Image Generation Capability of OpenAI Sora
Simulation Capabilities
At scale, Sora exhibits amazing simulation capabilities, enabling it to simulate aspects of people, animals, environments, and digital worlds without explicit inductive biases. These capabilities include:
- 3D Consistency: Generating videos with dynamic camera motion, ensuring consistent movement of people and scene elements through three-dimensional space.
- Long-Range Coherence and Object Permanence: Effectively modeling short- and long-range dependencies, maintaining temporal consistency even when objects are occluded or leave the frame.
- Interacting with the World: Simulating actions that affect the state of the world, such as leaving strokes on a canvas or eating a burger with persistent bite marks.
Simulating Digital Worlds: Simulating artificial processes, including controlling players in video games like Minecraft while rendering high-fidelity worlds and dynamics.
Limitations of Sora
Limitation of OpenAI's Sora - Glass Shattering Effect
OpenAI acknowledged that the current AI model has known weaknesses, including:
- Struggling to accurately simulate complex space
- Understand some instances of cause and effect
- Confuse spatial details of a prompt
- Precise descriptions of events over time
Safety Considerations of Sora
OpenAI is currently working with a team of red teamers to test the AI model prior to making Sora available to OpenAI users. These red teamers consist of domain experts familiar with misinformation, hateful content, and bias.
In their release, OpenAI has stated that they will not only leverage existing safety methods leveraged for the release of DALL-E3 but also going one step further to build tools to detect misleading content, including a detection classifier that can identify a video generated by Sora.
Once the model is released in OpenAI’s products, they will include C2PA metadata and be monitored by their text and image classifiers: input prompts that violate their usage policy will be rejected and video outputs will be reviewed frame by frame.
In addition to all these safety precautions, OpenAI has also stated they will engage policymakers, educators, and artists to understand concerns and identify use cases for the model.
Text-to-video synthesis with Sora
Noteworthy Text to Video Generation Models
Google’s Lumiere
Google’s recent introduction of its text-to-video diffusion model, Lumiere is truly remarkable as well. It is designed to generate realistic, diverse, and coherent motion in videos. Lumiere’s capabilities include:
- text-to-video generation
- image-to-video generation
- stylized generation
- text-based video editing
- animating content of an image within a user-provided region
- Video inpainting
Unlike traditional approaches that rely on cascaded designs involving distant keyframe generation and subsequent temporal super-resolution, Lumiere introduces Space-Time I-Net architecture. This architecture allows Lumiere to generate the entire temporal duration of the video at once, streamlining the synthesis process and improving global temporal consistency.
:no_upscale():format(webp)/cdn.vox-cdn.com/uploads/chorus_asset/file/24086506/imagen_multi_clip_gif.gif)
Google Lumiere's Prompt Generated AI Video
By incorporating spatial and temporal down- and up-sampling techniques and leveraging pre-trained text-to-image diffusion models, Lumiere achieves remarkable results in generating full-frame-rate, low-resolution videos. This approach not only enhances the overall visual quality of the synthesized videos but also facilitates a wide range of content creation tasks and video editing applications, including image-to-video conversion, video inpainting, and stylized generation.
Stability AI’s Stable Video Diffusion
Stability AI introduced Stable Video Diffusion, a latent video diffusion model designed for state-of-the-art text-to-video and image-to-video generation tasks. Leveraging recent advancements in latent diffusion models (LDMs) initially trained for 2D image synthesis, Stability AI extends its capabilities to generate high-resolution videos by incorporating temporal layers and fine-tuning them on specialized video datasets.
Stability AI addresses the lack of standardized training methods by proposing and evaluating three key stages for successful training of video LDMs: text-to-image pretraining, video pretraining, and high-quality video finetuning. Emphasizing the importance of a meticulously curated pretraining dataset for achieving high-quality video synthesis, Stability AI presents a systematic curation process, including strategies for captioning and data filtering, to train a robust base model.
The Stable Video Diffusion model demonstrates the effectiveness of finetuning the base model on high-quality data, resulting in a text-to-video model that competes favorably with closed-source video generation methods. The base model not only provides a powerful motion representation for downstream tasks such as image-to-video generation but also exhibits adaptability to camera motion-specific LoRA modules.
It also showcases the versatility of its model by demonstrating its strong multi-view 3D-prior capabilities, serving as a foundation for fine-tuning a multi-view diffusion model that generates multiple views of objects in a feedforward manner. This approach outperforms image-based methods while requiring a fraction of their compute budget, highlighting the efficiency and effectiveness of Stable Video Diffusion in generating high-quality videos across various applications.
Meta’s Make-A-Video
Meta two years ago introduced Make-A-Video. Make-A-Video leverages paired text-image data to learn representations of the visual world and utilize unsupervised learning on unpaired video data to capture realistic motion. This innovative approach offers several advantages:
- It expedites the training of text-to-video models by leveraging pre-existing visual and multimodal representations
- It eliminates the need for paired text-video data
- It inherits the vast diversity of aesthetic and fantastical depictions from state-of-the-art image generation models.
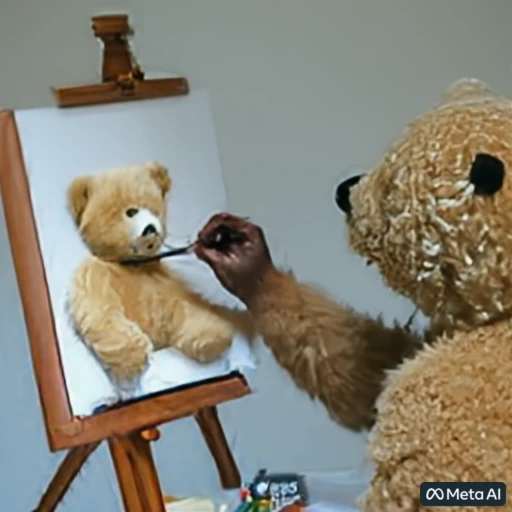
Meta's Make-A-Video Generated Graphic
Make-A-Video is a simple yet effective architecture that builds on text-to-image models with novel spatial-temporal modules. First, full temporal U-Net and attention tensors are decomposed and approximated in space and time. Then, a spatial-temporal pipeline is designed to generate high-resolution and frame-rate videos, incorporating a video decoder, interpolation model, and two super-resolution models to enable various applications beyond text-to-video synthesis.
Despite the limitations of text describing images, Make-A-Video demonstrates surprising effectiveness in generating short videos. By extending spatial layers to include temporal information and incorporating new attention modules, Make-A-Video accelerates the T2V training process and enhances visual quality.
Sora: Key Highlights
With a SOTA diffusion model, Sora empowers users to effortlessly transform text descriptions into captivating high-definition video clips, revolutionizing the way we bring ideas to life.
Here are the key highlights of Sora:
- Sora's Architecture: Utilizes a diffusion model and transformer architecture for efficient training.
- Sora's Methodologies: Sora uses methodologies like unified representation, patch-based representations, video compression network, and diffusion transformer.
- Capabilities: Includes image and video prompting, DALL·E image animation, video extension, editing, image generation, etc.
- Limitations: Weaknesses in simulating complex space and understanding causality.
- Sora's Safety Considerations: Emphasizes safety measures like red team testing, content detection, and engagement with stakeholders.
- Other significant text-to-video models: Lumiere, Stable Video Diffusion, and Make-A-Video.
Data infrastructure for multimodal AI
Click around the platform to see the product in action.
Explore the platformWritten by
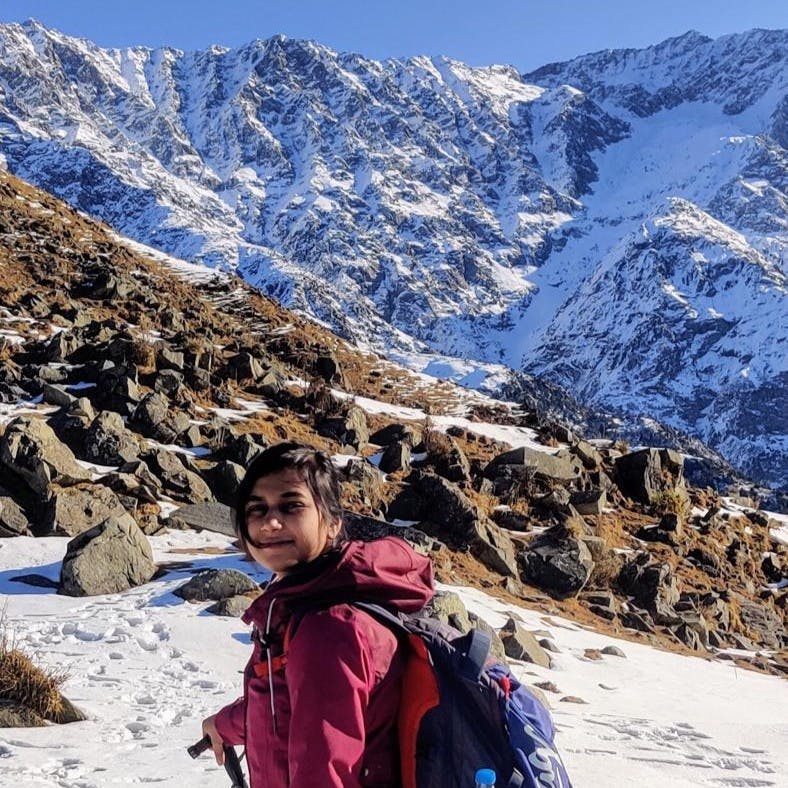
Akruti Acharya
Explore our products
- OpenAI's Sora utilizes a diffusion model and transformer architecture. It starts with noisy video frames and gradually refines them using multiple steps, maintaining subject consistency.
- Using OpenAI's Sora involves providing text prompts that describe the desired video content. Sora then generates high-definition video clips based on these prompts.
- Other text-to-video generation models include Google's Lumiere, Stability AI's Stable Video Diffusion, and Meta's Make-A-Video.
- As of now, OpenAI has not made Sora public. OpenAI is currently working with red teamers who are testing the model against misinformation, hateful content, bias, and other security concerns regarding AI-generated video.
- Sora is not available for public use yet.
- OpenAI is teaming up with red teamers consisting of policymakers, educators, filmmakers, and visual artists around the world to understand their concerns and to identify positive use cases for this new AI video generation model.
- With the recent release of GPT-4 with vision capabilities few months before, it won’t be surprising to see the chatbot with video capabilities. This is a step towards artificial general intelligence (AGI).