Contents
What is Computer Vision?
10 Exciting Computer Vision Based Applications in 2024
What Does 2024 Have in Store for Computer Vision Applications?
Encord Blog
10 Most Exciting Applications of Computer Vision in 2024
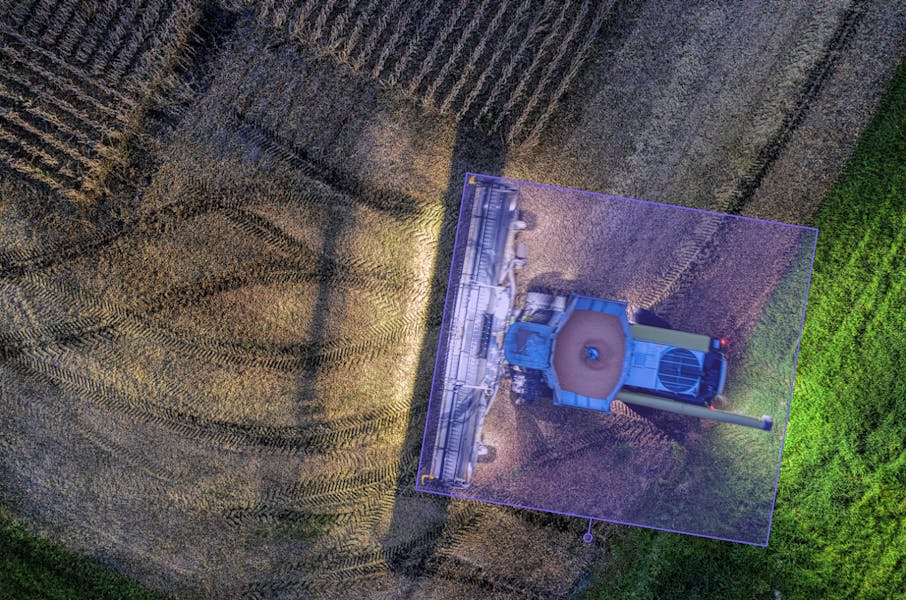
Computer Vision (CV) models are already playing a role in numerous sectors, with hundreds of use cases and commercial applications. 2023 saw an increase in the number of CV-based applications, and we expect the same in 2024 and beyond.
A lot of work, time, skills, and resources have gone into training CV models and other algorithms. Computers can’t “see” in the same way humans can, so anything a CV model identifies is a result of a painstaking process to train it. This work includes but is not limited to applying annotations and labels across thousands of image and video-based datasets.
In this article, we take a closer look at how computer vision models are used across numerous industries and what we can expect from 2024 and beyond, with new innovations and applications emerging.
We dive into computer vision applications in the following 10 sectors:
- Insurance
- Manufacturing
- Sports analytics
- Identity verification
- Agriculture
- Energy and Infrastructure
- Medical and healthcare
- Mobility and automotive
- Retail
- Geospatial
What is Computer Vision?
Going back to basics for a moment: “Computer vision is a field of artificial intelligence (AI) that enables computers and systems to derive meaningful information from digital images, videos and other visual data — and take actions or make recommendations based on that information. If AI enables computers to think, computer vision enables them to see, observe and understand”, according to an IBM definition.
At the start of any computer vision project, you need imaging or video-based datasets applicable to the intended use case and application. Cleaning the data is also integral to the process, otherwise, you risk giving a CV model unclean data, tainting the results, and wasting time and resources.
You need a team of annotators and ideally, annotation tools with automation to annotate and label the images or videos.
After the data cleaning, annotation, and labeling, your ML or data science teams need to train one or more CV, AI, or deep learningmodels to achieve a high accuracy score, ensures any bias is reduced, and that it generates the results you need. Only then can the model go from proof of concept (POC) to production, with iterative feedback loops designed to further improve the results.
10 Exciting Computer Vision Based Applications in 2024
According to Forbes, the computer vision market is currently worth around $48.6 billion and is expected to keep growing.
Here are 10 examples, real-world use cases and applications for computer vision technology:
Insurance technology
Several startups in the insurance technology sector are using computer vision systems and AI to improve the speed and accuracy of insurance claims.
Having been deployed across dozens of market-leading multi-billion dollar insurance companies, it has already been responsible for helping 1 million households, resulting in $2 billion in claims processed quicker.
When images or videos of damage are processed through a CV model, insurance companies can better determine the cost of damage and whether the claim is valid, allowing them to process these claims quicker.
{{opensource_on_github_bar}}
Manufacturing
Computer vision algorithms are also being deployed across the manufacturing sector, interconnected with Internet of Things (IoT) devices embedded in production machines. In the last 10 years, there’s been a digital revolution in manufacturing; some are calling this “Factory 3.0”, or even “Factory 4.0”. CV models are playing a role in this ongoing digital transformation.
In factories, CV models and real-world applications of annotated image and video datasets are being used to:
- Implement fully automated production line assembly;
- Detecting defects before products leave the factory;
- Generating real-time 3D models, using computer vision algorithms, to improve manufacturing processes that humans struggle with (e.g. hyper-detailed manufacturing, and putting together small parts in machines in everything from electronics to oil and gas);
- Rotary and laser die cutting: incredibly precise cutting during the manufacturing process;
- Predictive maintenance to increase efficiency and reduce downtime;
- Improve health and safety and security;
- Inventory management, packaging, shipping, and a whole load more!
Sports Analytics
Analytics and various data-centric monitoring solutions have been in use across the sporting sector for over 20 years. More recently, computer vision technology has been used to better understand player movements. Combining computer vision models with hyper-accurate recording cameras and other applications is taking sports analytics to the next level and giving coaches a wider range of insights.
Identity Verification
In most cases, identity verification software has been developed in the US and Western Europe. As a result, the datasets this software and the relevant face recognition models have been trained on are representative of the majority demographics in those countries and regions.
Vida, a digital identity, facial recognition, and verification platform that serves customers throughout Southeast Asia, is overcoming this with its own CV-based solution.
Vida solved this problem by collecting and training models on South East Asian image-based datasets. In particular, over 60,000 images of people across Indonesia, an ethnically diverse and large series of islands with a population of 276 million.
Using Encord’s tools, Vida has been able to effectively train CV models with a higher quality of data at a much faster rate and with superior accuracy than previously.
For more information on how computer vision and Encord are being used for identity verification, here’s an article for a more in-depth look.
(Source)
Agriculture
In the agricultural sector, computer vision systems are being used to reduce the production costs of growing plants in greenhouses.
For fruit and vegetable growers, this is a game-changing innovation that’s helping them to increase yields and profits while reducing waste and selling more affordable, healthier, local produce. AgriTech companies deploy CV models in the growing and monitoring process, ensuring that plants are reaching their ideal yield size so they can be picked at the right time in turn reducing food waste, growers' and farmers' carbon footprints, and increasing profits.
Energy and Infrastructure
The energy sector incurs massive losses across vast infrastructure networks. The amount of power a nuclear plant or wind farm generates isn’t the same volume of energy that reaches consumers and businesses. Inefficiencies and a crumbling infrastructure cost the sector billions every year.
Using a data-driven and AI-based approach to infrastructure inspections and asset management decisions has changed this. Images and videos of the component parts of infrastructure networks are processed, labeled, annotated, and run through computer vision models to identify the repairs and replacements needed.
Inspection costs are reduced, as are asset maintenance and management costs; actively increasing energy outputs from generation plants, national transmission, and distribution networks.
Medical Use Cases for Computer Vision
Medical use cases and applications for computer vision techniques are enormous, with new, innovative approaches emerging everyday.
Encord has worked with dozens of healthcare providers and technology companies in this sector to support CV-based innovations. The platform is equipped to handle DICOM and NIfTI in their native format.
Here are a few examples:
- Computer vision models are being used to generate secondary insights from medical imaging scans by an abnormality detection company. For example, scans taken of patients in an MRI machine are to detect a specific illness. However, the scans come back clear, and yet the patient is still showing symptoms of something that can’t be identified.
- In gastrointestinal care (GI), a company is using computer vision machine learning algorithms in pre-trial screenings of patients for clinical trials to improve diagnosis accuracy. These models are trained to analyze large datasets faster and in greater detail than a team of medical specialists would be able to
- CV models are trained to analyze thousands of images faster, more accurately, and in much greater detail than a team of medical specialists ever could. The aim of dozens of medical and healthcare use cases is to improve diagnosis accuracy.
For more information about computer vision use cases in the medical sector, and future applications of computer vision for healthcare, check out this article.
Encord has developed our medical imaging dataset annotation software in close collaboration with medical professionals and healthcare data scientists, giving you a powerful automated image annotation suite, fully auditable data, and powerful labeling protocols.
Mobility and automotive
Self-driving and autonomous vehicles are still in the development phase. Despite numerous successful tests and trials, car manufacturers are still slowly attempting to safely reach Level 3, “Highly Automated Driving.”
There are five stages to truly autonomous self-driving capabilities. The top level, 5, would be when you could get in a car, you could even fall asleep, and wake up at your destination. Self-driving vehicles aren’t there yet. Even Tesla isn’t ready to sanction self-driving capabilities.
Every Tesla can come equipped with an Autopilot feature; however, this is only a Level 2 advanced driver-assistance system (ADAS), you still need a fully-attentive driver at the wheel to adhere to the law and make sure you don’t crash.
With that in mind, computer vision models are already playing an important role in self-driving automation. Numerous CV and ML models have been deployed within self-driving algorithms to ensure a vehicle can see what’s in front and around it.
Computer vision models are also being used to monitor parking lots and improve parking management. Urban planners and local governments use computer vision models to monitor pedestrian traffic and improve safety in busy urban areas.
(Source)
Retail
Computer vision models have numerous use cases in the retail sector, such as:
- Retail heatmaps: Retailers are now using them to analyze the movement of customers in a store, including how long they spend looking at products and displays.
- Stores without checkouts. Amazon has trialed this concept, whereby customers pay via facial recognition connected to their accounts, using real-time, one-shot computer vision models.
- AI-powered traffic analysis. Retailers need to know how many people are going in and out, or passing by, to assess the ongoing viability of specific locations.
- Inventory management and CV/AI-based loss prevention. Retailers lose money when stock levels are low. If customers can’t find what they’re looking for they’ll go elsewhere or online. At the same time, computer vision models are useful for detecting theft, thereby preventing losses that way too.
- … And many more
{{opensource_on_github_bar}}
(Source)
Geospatial
Computer vision geospatial use cases cover many industries and sectors. Geospatial analytics leverages a combination of satellite imagery (including Synthetic-Aperture Radar), geographic and spatial data, and location information. Naturally, computer vision models play an important role in these processes.
The role of computer vision models in geospatial use cases includes academic research, conservation projects, disaster response, humanitarian action, and at the other end of the spectrum, military applications and battlefield deployment.
(Source)
What Does 2024 Have in Store for Computer Vision Applications?
At Encord, we fully expect computer vision innovation to accelerate in 2024, driving commercial applications and the adoption of this technology forward. We expect this to continue across every field CV technology touches, including medical science and treatments, manufacturing, augmented and virtual reality (AR/VR), self-driving cars, robotics, satellite imagery, manufacturing, retail, and analytics.
Specific innovations we expect to see more of include:
- An increase in the use of active learning pipelines. Active learning (AL) is a process whereby computer vision models — through ML and data ops leaders — actively ask for more information (images, videos, etc.), to improve the performance of the model. Using active learning accelerates model development and improves performance, as we explain in more detail in this article.
- Applying computer vision in the application and development of foundational models. Generative AI is currently accelerating at an unprecedented rate. CV applications and use cases are going to play a more important role in this.
- Deploying computer vision closer to the source of the data, using Edge AI (or Edge Intelligence). Edge AI is when data is captured, stored, and processed close to the source of the data (on edge devices). Applying this using computer vision models would involve pulling the image or video datasets into an annotation platform and then feeding them directly into a CV micro-model or active learning pipeline. Edge computing using AI is a viable and practical use case in several sectors and could generate enormous real-world value in healthcare, security, manufacturing, and other industries.
- Moving to a data-centric computer vision model. CV data scientists are increasingly focusing on improving the outputs of computer vision models from a different perspective: focusing on the data rather than the models. Instead of constantly trying to modify the models and algorithms, with more effort being put into improving the quality of the datasets, such as the labels and annotations, and the process to create and manage those, the outputs from models can improve dramatically, in some cases.
In reality, whether these innovations and new applications are big or small, we anticipate new use cases, applications, products, and services to emerge from the innovative world of computer vision research and technology startups.
Ready to improve the performance and scale your data operations, labeling, and automated annotation?
Sign-up for an Encord Free Trial: The Active Learning Platform for Computer Vision, used by the world’s leading computer vision teams.
AI-assisted labeling, model training & diagnostics, find & fix dataset errors and biases, all in one collaborative active learning platform, to get to production AI faster. Try Encord for Free Today.
Want to stay updated?
- Follow us on Twitter and LinkedIn for more content on computer vision, training data, and active learning.
- Join the Slack community to chat and connect.
Data infrastructure for multimodal AI
Click around the platform to see the product in action.
Explore the platformWritten by
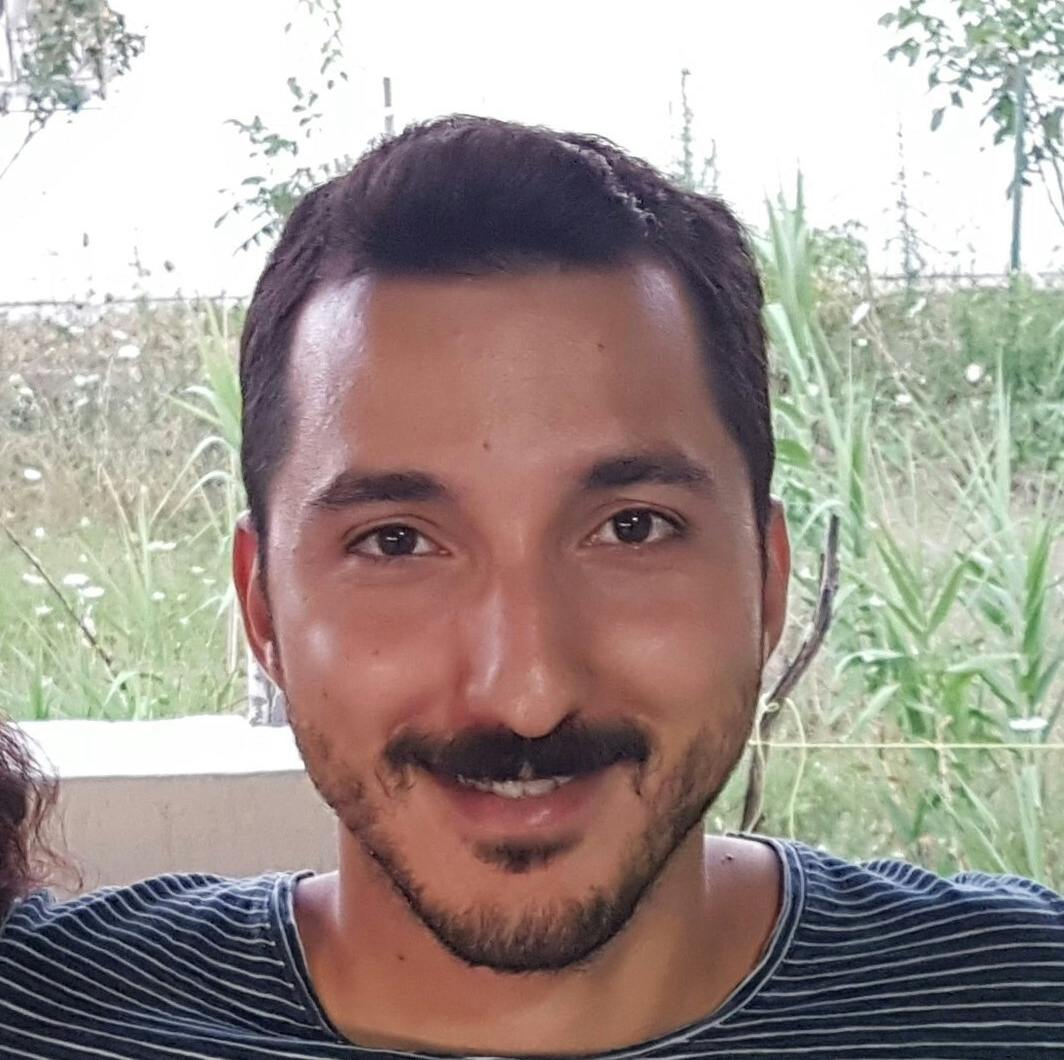
Görkem Polat
Explore our products