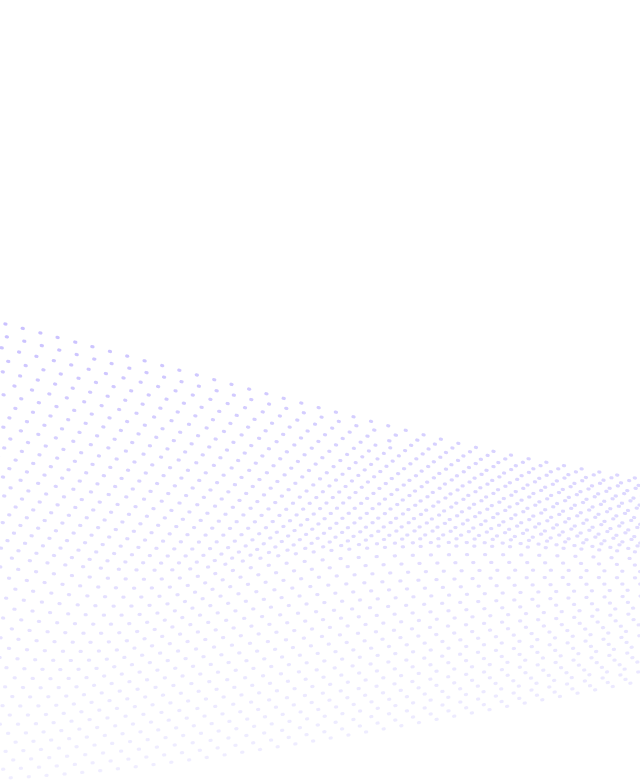
Encord Blog
Immerse yourself in vision
Trends, Tech, and beyond
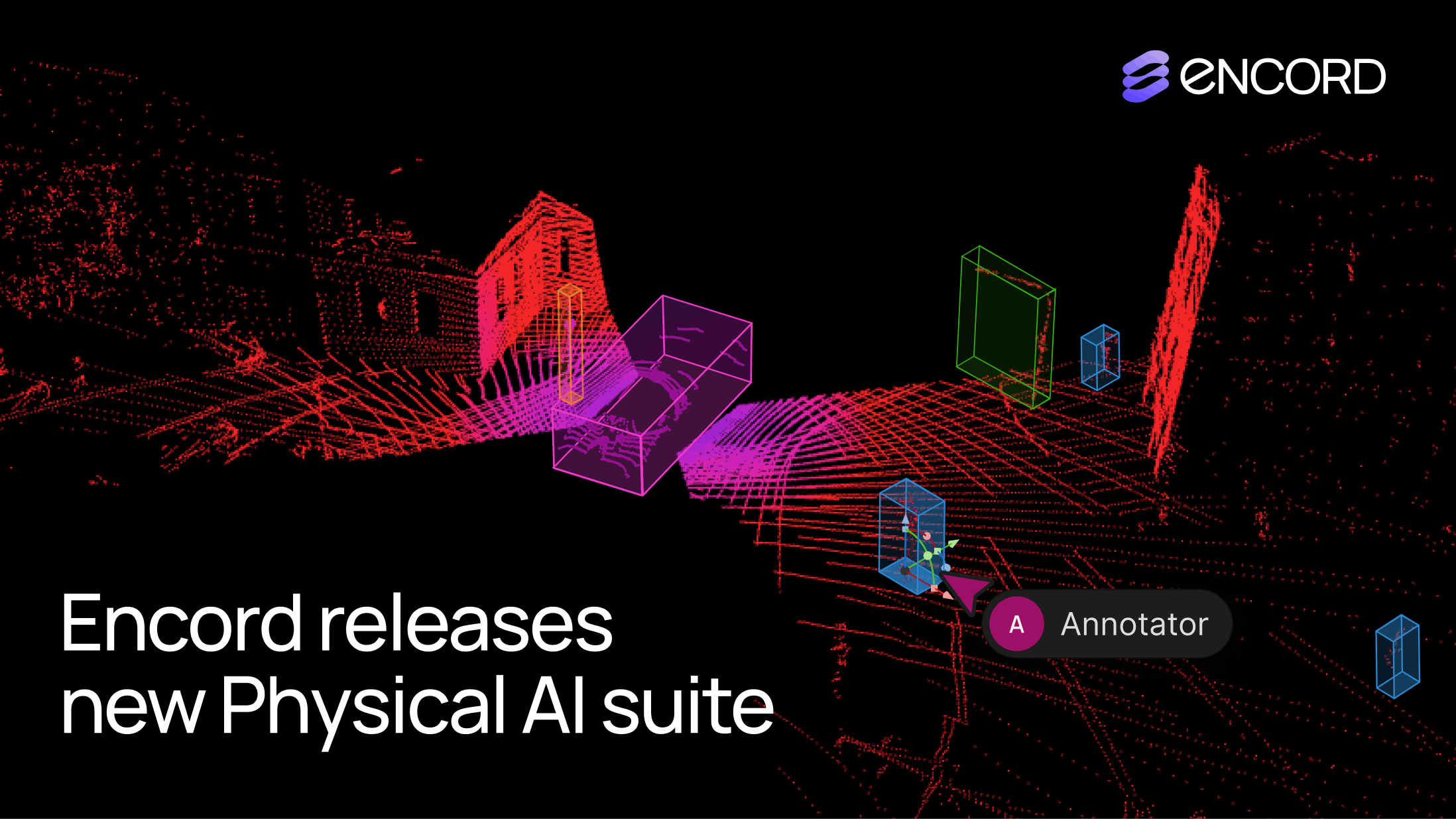
Encord Releases New Physical AI Suite with LiDAR Support
We’re excited to introduce support for 3D, LiDAR and point cloud data. With this latest release, we’ve created the first unified and scalable Physical AI suite, purpose-built for AI teams developing robotic perception, VLA, AV or ADAS systems. With Encord, you can now ingest and visualize raw sensor data (LiDAR, radar, camera, and more), annotate complex 3D and multi-sensor scenes, and identify edge-cases to improve perception systems in real-world conditions at scale. 3D data annotation with multi-sensor view in Encord Why We Built It Anyone building Physical AI systems knows it comes with its difficulties. Ingesting, organizing, searching, and visualizing massive volumes of raw data from various modalities and sensors brings challenges right from the start. Annotating data and evaluating models only compounds the problem. Encord's platform tackles these challenges by integrating critical capabilities into a single, cohesive environment. This enables development teams to accelerate the delivery of advanced autonomous capabilities with higher quality data and better insights, while also improving efficiency and reducing costs. Core Capabilities Scalable & Secure Data Ingestion: Teams can automatically and securely synchronize data from their cloud buckets straight into Encord. The platform seamlessly ingests and intelligently manages high-volume, continuous raw sensor data streams, including LiDAR point clouds, camera imagery, and diverse telemetry, as well as commonly supported industry file formats (such as MCAP). Intelligent Data Curation & Quality Control: The platform provides automated tools for initial data quality checks, cleansing, and intelligent organization. It helps teams identify critical edge cases and structure data for optimal model training, including addressing the 'long-tail' of unique scenarios that are crucial for robust autonomy. Teams can efficiently filter, batch, and select precise data segments for specific annotation and training needs. 3D data visualization and curation in Encord AI-Accelerated & Adaptable Data Labeling: The platform offers AI-assisted labeling capabilities, including automated object tracking and single-shot labeling across scenes, significantly reducing manual effort. It supports a wide array of annotation types and ensures consistent, high-precision labels across different sensor modalities and over time, even as annotation requirements evolve. Comprehensive AI Model Evaluation & Debugging: Gain deep insight into your AI model's performance and behavior. The platform provides sophisticated tools to evaluate model predictions against ground truth, pinpointing specific failure modes and identifying the exact data that led to unexpected outcomes. This capability dramatically shortens iteration cycles, allowing teams to quickly diagnose issues, refine models, and improve AI accuracy for fail-safe applications. Streamlined Workflow Management & Collaboration: Built for large-scale operations, the platform includes robust workflow management tools. Administrators can easily distribute tasks among annotators, track performance, assign QA reviews, and ensure compliance across projects. Its flexible design enables seamless integration with existing engineering tools and cloud infrastructure, optimizing operational efficiency and accelerating time-to-value. Encord offers a powerful, collaborative annotation environment tailored for Physical AI teams that need to streamline data labeling at scale. With built-in automation, real-time collaboration tools, and active learning integration, Encord enables faster iteration on perception models and more efficient dataset refinement, accelerating model development while ensuring high-quality, safety-critical outputs. Implementation Scenarios ADAS & Autonomous Vehicles: Teams building self-driving and advanced driver-assistance systems can use Encord to manage and curate massive, multi-format datasets collected across hundreds or thousands of multi-hour trips. The platform makes it easy to surface high-signal edge cases, refine annotations across 3D, video, and sensor data within complex driving scenes, and leverage automated tools like tracking and segmentation. With Encord, developers can accurately identify objects (pedestrians, obstacles, signs), validate model performance against ground truth in diverse conditions, and efficiently debug vehicle behavior. Robot Vision: Robotics teams can use Encord to build intelligent robots with advanced visual perception, enabling autonomous navigation, object detection, and manipulation in complex environments. The platform streamlines management and curation of massive, multi-sensor datasets (including 3D LiDAR, RGB-D imagery, and sensor fusion within 3D scenes), making it easy to surface edge cases and refine annotations. This helps teams improve how robots perceive and interact with their surroundings, accurately identify objects, and operate reliably in diverse, real-world conditions. Drones: Drone teams use Encord to manage and curate vast multi-sensor datasets — including 3D LiDAR point clouds (LAS), RGB, thermal, and multispectral imagery. The platform streamlines the identification of edge cases and efficient annotation across long aerial sequences, enabling robust object detection, tracking, and autonomous navigation in diverse environments and weather conditions. With Encord, teams can build and validate advanced drone applications for infrastructure inspection, precision agriculture, construction, and environmental monitoring, all while collaborating at scale and ensuring reliable performance Vision Language Action (VLA): With Encord, teams can connect physical objects to language descriptions, enabling the development of foundation models that interpret and act on complex human commands. This capability is critical for next-generation human-robot interaction, where understanding nuanced instructions is essential. For more information on Encord's Physical AI suite, click here.
Jun 12 2025
m
Trending Articles
1
The Step-by-Step Guide to Getting Your AI Models Through FDA Approval
2
Introducing: Upgraded Analytics
3
Introducing: Upgraded Project Analytics
4
18 Best Image Annotation Tools for Computer Vision [Updated 2025]
5
Top 8 Use Cases of Computer Vision in Manufacturing
6
YOLO Object Detection Explained: Evolution, Algorithm, and Applications
7
Active Learning in Machine Learning: Guide & Strategies [2025]
Explore our...
Why Your AI Data Infrastructure Is the Real Competitive Advantage
Introduction: The Hidden Edge in the AI Race This guide to AI data infrastructure breaks down why it’s the real competitive advantage for your AI initiatives, showing how strong pipelines, automation, and feedback loops drive speed, accuracy, and cost efficiency. Artificial intelligence (AI) and machine learning innovation now rely more on data infrastructure than just building smarter models. Leading tech companies like Microsoft, Amazon, Alphabet, and Meta are investing in AI infrastructure. Total capital expansion is projected to grow from $253 billion in 2024 to $1.7 trillion by 2035. That investment signals the actual value is in scalable, performant, and secure data foundations. A MIT Sloan Management Review study found that organizations with high-quality data are three times more likely to gain significant benefits from AI. Managing your AI data infrastructure well and deploying AI with ready AI datasets takes you far beyond your peers in terms of speed, cost, and AI-driven decision-making accuracy. In this article, we will discuss why your AI data infrastructure matters more than model tweaks and how it drives scalability and operational efficiency. We will also go over how feeding enriched data back-to-back into training through feedback loops accelerates model improvements, as well as how infrastructure investments reduce costs and time-to-market. Why Data Ops Is Not Just a Cost—It’s a Strategic Asset An effective way to improve data infrastructure is by adopting DataOps. DataOps is a set of agile, DevOps-inspired practices that streamline end-to-end data workflows, from ingestion to insights. Many leaders view data operations as a sunk cost or a side project to support AI models. This perspective limits their AI strategy. In reality, Data Ops is a strategic asset that drives competitive advantage across your AI initiatives. Treating data like code transforms how teams develop AI systems. When you adopt data-as-code principles, your workflows become: Modular: Data pipelines and workflows are broken down into clear, manageable components. This way, you can swap pipelines or models without having to rebuild your system. Versioned: Just as software engineers version their code, dataset versioning allows you to track changes and maintain full audit trails. Additionally, it helps compare model performance across iterations. Tools like DVC (Data Version Control) enforce version tracking for datasets, just like Git for code. Reusable: Once you codify a pipeline, you can reuse it in AI projects. This reduces duplication of effort and speeds up deployment. The Winning Loop Explained Successful AI initiatives rely on a winning loop that few competitors can replicate. This loop turns data operations from a support function into a strategic growth engine. Here are the loop steps: Capture Live Signals from Real-World Usage Real-world usage generates immediate insights like clicks, transactions, chat logs, and sensor readings. Capture these live signals in real time to align models with actual user behavior. Triage and Enrich Data with Human- and Model-in-the-Loop Workflows Raw captured data is rarely perfect. The data pipeline can use automation features present in tools like Encord. Its active filtering functionality can help filter out, categorize, and pre-process extensive datasets. In addition, human-in-the-loop (HITL) and model-in-the-loop (MITL) workflows further refine this data for quality. Humans resolve edge cases, ambiguities, and context-specific labels, while AI tools streamline scalable enrichment and annotation tasks. This combined approach optimizes data quality while controlling costs. Feed Data Back into Training, Fine-Tuning, and Evaluation Once data is cleaned and enriched, it flows into versioned pipelines for training, fine-tuning, and automated evaluation. DataOps enforces Continuous Integration and Continuous Delivery (CI/CD) for data, automated tests, schema validation, and real-time Quality Assurance (QA) before the data enters production. Fig 1. Continuous Feedback Loop Drives Competitive Advantage Pro Insight: Teams running this loop continuously build compounding value. They don’t just rely on new algorithms to drive results. Instead, their AI systems evolve daily with fresh, relevant data. Meanwhile, competitors who focus only on tweaking models face diminishing returns without a strong data infrastructure to support ongoing learning. Human-in-the-Loop Machine Learning (HITL) Explained Why Competitors Can’t Copy Your Data Flywheel Having a strong data infrastructure built on an efficient DataOps workflow gives your team a lasting edge. It enables faster iteration, better quality control, and domain-specific learning that compounds over time. While competitors may replicate your models, algorithms, AI tools, or frameworks, they cannot recreate your data flywheel. This system captures real-time signals, efficiently enriches the data, and feeds it back into the model pipeline. Fig 2. Data Flywheel and How it Works Datasets as Intellectual Property Treating datasets like intellectual property assets helps you secure a competitive advantage. Companies with high data valuation often have market-to-book ratios 2–3 times higher, reflecting a premium on data quality, ownership, and governance. Internal tools and processes for data versioning, annotation, and lineage are proprietary and provide your organization with a hidden edge. Edge Cases Are Unique to Your Domain Every AI system encounters edge cases, those rare, unexpected scenarios that models struggle with. Only your team understands the full context behind edge-case exceptions, the frequency, and the business impact. Over time, your feedback loops and triage processes effectively capture and resolve these edge cases, empowering you to leverage AI capabilities for deeper domain adaptation. This leads to fine-tuned models deeply aligned with your operations, workflows, and customer interactions. For competitors, if they want to replicate your data, they may require your organizational knowledge and historical context. Real-World Example: A lead data scientist we spoke to expressed the workflow complexity surrounding edge cases and feedback. This extends beyond basic data operations and instead requires knowledge reflected in the company’s datasets, tools, and processes. How Strong Data Infrastructure Reduces Cost and Time-to-Market A well-built AI data infrastructure delivers measurable savings and accelerates deployment. It replaces fragmented, manual efforts with repeatable systems that enable scalable efficiency across teams and projects. Faster Project Onboarding According to a study, organizations using data pipeline automation saw 80% reductions in time to create new pipelines, moving fast from concept to live data. When data pipelines, workflows, and versioning are standardized, new AI initiatives get up and running quickly. Teams don’t waste weeks rebuilding ingestion scripts or annotation processes. Instead, they use existing modular frameworks to start experimentation and model training sooner, aligning with business needs efficiently. Smarter Annotation at Scale Manual data labeling becomes a bottleneck as datasets grow. Having a tool like Encord AI data management in your data stack automates data triage and integrates human-in-the-loop (HTIL) annotation seamlessly. Additionally, it deploys model-in-the-loop pre-labeling to reduce manual effort. This optimized annotation process improves data quality and speeds up training cycles. It ensures your AI applications remain accurate and reliable as workloads increase. Fig 3. Human-in-the-Loop Automation Reuse Models and Datasets Across Products An AI data infrastructure that is versioned and reusable enables teams to use existing datasets and pretrained models for new use cases. This reduces duplication of work, lowers operational costs, and shortens time-to-market for AI-powered features across products. High Marginal Costs Without Infrastructure Without a scalable data infrastructure, every new AI project faces high additional costs. Labeling costs increase linearly with customer growth unless automation is used. QA and feedback pipelines struggle to scale, which causes deployment delays and increased operational risk. This is not sustainable for companies looking to grow AI capabilities throughout their ecosystem. CEO Insight: These needs were validated by a CEO of a firm in the computer vision space, who expressed that scaling past the competition has a high marginal cost. However, there is a competitive advantage in building a data labeling infrastructure. Without it, the cost to support each customer remains high, hurting cost efficiency. The Full Guide to Automated Data Annotation Moving from Prototype to Production-Ready AI Experimentation is easy, but scaling AI to production brings a lot of challenges. Many teams build impressive proof-of-concept models. These models perform well in controlled environments but fail when exposed to real-world requirements such as auditability, consistency, and compliance. Without a strong, end-to-end data infrastructure, moving from prototype to production becomes a bottleneck. To overcome these challenges, teams must adopt repeatable, automated pipelines as the foundation of their infrastructure. Here’s why repeatable pipelines matter: Consistent Quality: Repeatable data pipelines ensure that the same standards are applied across training, validation, and deployment. This consistency is crucial for maintaining model performance when working with diverse and evolving datasets. Regulated Industry Compliance: Industries such as healthcare and finance require traceable and auditable AI systems. Without automated and versioned data workflows, proving compliance becomes manual, slow, and prone to errors. Faster Iteration Cycles: Automated pipelines reduce time spent on repetitive tasks. Engineers can quickly update models, test them with fresh data, and deploy improvements. This agility gives companies a strong competitive edge. Researcher Testimony: This is exactly what was described by a researcher at a firm developing agentic AI. While his team had working models, they did not have repeatable data infrastructure. This put them at a competitive disadvantage. Winning with Data—Not Just Models AI innovation is often framed as a race to build smarter generative AI models. Yet, the true differentiator is how your team uses AI data infrastructure to keep models learning, accurate, and ready for production. A data-focused approach, supported by automated pipelines and strong data management, turns raw datasets into a strategic asset. This boosts AI adoption across industries such as healthcare, finance, and supply chain. Teams that invest in strong AI data infrastructure build a data flywheel. This flywheel becomes an engine of continuous improvement, embedding domain expertise into every cycle. Key advantages of data-centric infrastructure include: Faster Iteration: Automated data pipelines reduce turnaround time for model updates. Teams move from weeks to days in deploying improvements, staying ahead in competitive markets. Higher Model Accuracy: Fresh, high-quality, on-distribution data improves predictive performance. Models stay aligned with evolving user behavior and edge cases unique to your ecosystem. Lower Deployment Costs: Reusable pipelines, versioned datasets, and streamlined labeling reduce infrastructure costs. Teams avoid rebuilding from scratch for each use case, maximizing operational efficiency. Fewer Failures in Production: Repeatable, audited workflows ensure that models work as expected when exposed to real-world demands, regulatory requirements, and customer interactions. Build Your Data-Centric Infrastructure with Encord Encord offers a unified platform to manage, curate, and annotate multimodal datasets. It serves as a direct enabler of robust AI data infrastructure in production settings. Unified Data Management & Curation (Index) Encord handles images, video, audio, and documents within a single environment for in-depth data management, visualization, search, and granular curation. It connects directly to cloud storage, including AWS S3, GCP, Azure, and indexes nested data structures. Fig 4. Manage & Curate Your Data AI-Assisted and Human-in-the-Loop Annotation Automated labeling offloads repetitive tasks, then humans review and correct edge-case outputs. This HTIL workflow boosts annotation accuracy by up to 30% and speeds delivery by 60%, ensuring data quality at scale. Encord Active monitors model performance, surfaces data gaps, finds failure modes, and helps improve your data quality for model retraining loops. Data‑Centric Curation & Dataset Optimization Encord applies intelligent filters to detect corrupt, redundant, or low-value datapoints. Encord index led to a 20% increase in model accuracy and a 35% reduction in dataset size for Automotus. Business Impact & Results Encord users report tangible outcomes, including $600,000 saved annually by Standard AI and a 30% improvement in annotation accuracy at Pickle Robot. Its use of the Index alone cut dataset sizes by 35%, saving on compute and annotation costs. Conclusion AI success at scale comes from data infrastructure. Tech companies are investing to scale their infrastructure because data pipelines, governance, and real-time systems deliver growth, productivity, and long-term profitability. When you treat data as a strategic asset, applying versioning, modular pipelines, and automated workflows, you gain: Faster iteration and deployment, outpacing competitors Higher accuracy and robustness, driven by quality datasets Lower costs and failure rates, thanks to reusable, scalable systems Defensible differentiation, through proprietary feedback loops and domain edge cases If you want a lasting competitive edge, focus on your AI data infrastructure. Scalable pipelines, automated workflows, and strong governance are the foundation your AI strategy needs.
Jul 03 2025
5 M
Best Video Annotation Tools for Healthcare 2025
This guide to video annotation tools for healthcare breaks down how AI teams can create quality annotated video datasets for building accurate healthcare computer vision systems. Every year, hospitals add tens of millions of gastrointestinal endoscopy videos to their archives. A single 15-minute procedure produces around 27,000 high-definition frames, creating a large amount of visual data. However, most of this valuable footage remains unused because converting it into reliable AI requires detailed, frame-by-frame data labeling. However, manual labeling is slow and costly, and mistakes can put patient safety at risk. Annotating just 100,000 medical images can cost over $1.6 million and take the effort of 20 people working for a whole year. Therefore, healthcare teams need video-annotation platforms like Encord that do more than draw boxes. These platforms must adhere to medical imaging standards, such as DICOM, and ensure the protection of patient health information. This article will define healthcare AI and the unique demands of video annotation in clinical settings. We will review the best video annotation tools for healthcare, focusing on platforms that create high-quality annotated videos for clinical AI systems. What Is Healthcare AI? Artificial intelligence (AI) in healthcare refers to the use of machine learning models to assist medical decision-making, diagnose diseases, and plan treatments. These models process medical data and give medical professionals important insights. This helps improve health outcomes and deliver better patient care. One of the biggest advances in healthcare AI stems from computer vision. This technology can detect objects in endoscopy videos. It can also identify organs in CT scans, which makes diagnosis faster and more accurate. Fig 1. Computer Vision in Healthcare The Importance of Video Annotation for the Success of Healthcare Model Video annotation is the process of applying descriptive tags, labels, or masks to specific objects in each frame of a video. This process converts raw video into high-quality annotated datasets that serve as the ground truth for training and validating vision models. This approach helps in the following ways: Drives Model Accuracy: Precise annotations ensure computer vision models learn from reliable data. Poor labels lead to unreliable predictions that healthcare cannot afford, and AI models struggle to meet reliability and safety standards. For example, a mislabeled polyp in a colonoscopy video can cause the model to miss a real polyp in future cases. Enables Auditability and Bias Checks: Quality-annotated data provides the foundation for auditability. This helps regulators understand and trace how an AI model arrived at a particular decision. It also helps detect bias, ensuring that AI algorithms do not repeat the existing biases found in the original data. Challenges Unique to Healthcare AI Building AI for healthcare is complex. Unlike general computer vision tasks, medical vision models face strict regulatory, technical, and ethical constraints. Common challenges include: Protected Data: Medical data contains PHI, which is highly sensitive. It is subject to strict global regulations, such as HIPAA and GDPR. Any data annotation platform must handle this information carefully and follow these regulations. High-Stakes Accuracy: A single misprediction can affect treatment, patient safety, and outcomes. This requires high-precision annotations, often needing pixel-level accuracy. Techniques like panoptic or instance segmentation are used to achieve this. Limited Expert Time: Annotating medical videos requires domain experts, such as radiologists, pathologists, or ophthalmologists. Their time is limited, making large-scale annotation slow and expensive. Regulatory Scrutiny: Healthcare AI is subject to regulatory oversight, such as the FDA, when used in diagnostics. Medical AI systems must ensure traceability, bias control, and incorporate human override mechanisms. Annotation platforms must provide transparent workflows, quality control, and complete documentation. These challenges slow AI development and increase risk. Purpose-built video annotation tools, like Encord, help address them by enabling secure labeling and supporting regulatory compliance. Fig 2. Encord's DICOM Annotation Tool Key Features to Look for in Video Annotation Tools for Healthcare When selecting video annotation tools for healthcare, consider key features tailored to the unique needs of medical applications. This ensures high-quality training data and efficient workflows. The list below highlights some crucial features a video annotation tool should have. Support for Medical Image Formats: Look for a video annotation platform that supports standard medical imaging formats, like DICOM and NIfTI. It should also handle 3D and 4D volumetric data to enable annotation across spatial and temporal dimensions. Ability to Handle Large Video Files: Medical videos are often large and high-resolution. Tools should efficiently manage large file sizes and high frame counts without performance degradation to ensure a smooth annotation process. Collaboration Features: Effective annotation requires input from multi-disciplinary healthcare experts. Look for a tool that supports collaborative workflows, allowing teams to coordinate annotation tasks and reviews efficiently. Clinician-Centric User Interface: Look for a tool with a user-friendly interface for medical professionals. Features like intuitive timelines, measurement overlays, and voice annotations for quick feedback can enhance usability by simplifying complex annotation features. Automated and Active Learning Features: AI-assisted and pre-labeling can speed up the annotation process. The human-in-the-loop approach combines the speed of automation with the precision of human quality control, ensuring high-quality datasets for machine learning models. Secure Deployment Options: Medical data demands robust security. Choose tools that provide on-premises, virtual private cloud (VPC), or fully managed cloud solutions to protect sensitive information. Compliance with Healthcare Regulations: Annotation platforms must meet HIPAA and FDA standards to protect patient data and ensure legal compliance throughout the annotation process. Evaluating tools against these features can help in selecting a video annotation platform that aligns with the specific needs of your healthcare AI projects. Top Video Annotation Tools for Healthcare: Overview Top Video Annotation Tools for Healthcare The complexity of medical video data and the need for robust annotation workflows require teams to have platforms that combine automation, collaboration features, and compliance-ready infrastructure. Below is a list of video annotation tools commonly used in medical applications. Encord Encord is a collaborative AI data annotation platform that provides enterprise-grade solutions for complex, regulated AI projects, especially in the healthcare domain. The platform's objective is to accelerate the deployment of medical AI products by allowing the development of high-quality training datasets. Encord Supported Medical Imaging Formats and Video Formats Encord supports standard medical imaging formats, including DICOM and NIfTI. It offers 3D viewing options across sagittal, axial, and coronal planes, with window levels configurable via Hounsfield units, a standard in radiology. Encord also supports various video formats and resolutions. This ensures versatility across a range of medical video sources. Fig 3. Medical Data 3D View in Encord Encord Supported Annotation Types Encord supports various annotation types for detailed medical labeling. These include polygons for irregular shapes, key points for specific anatomical landmarks, and bounding boxes for object detection. It also supports rotatable boxes for objects in different orientations, polylines for linear structures, and classifications for video segments. A valuable feature for medical imaging is its support for Primitives (skeleton templates). These are essential for specialized annotations of template shapes, such as 3D cuboids and rotated bounding boxes, used to capture an object's three-dimensional structure from video. Encord also offers panoptic segmentation, enabling pixel-level labeling of both countable objects and amorphous regions. Fig 4. Encord Pixel-Perfect Labeling Encord AI-Assisted Labeling Capabilities Encord helps automate the annotation workflow through AI-assisted labeling. This includes automated object tracking and interpolation. These techniques intelligently fill in annotations between video frames, reducing the manual effort required across long sequences. Encord also integrates Meta AI’s Segment Anything Model (SAM), allowing instant, pixel-perfect segmentation masks. Moreover, it uses a micro-model approach and Models-in-the-Loop. This allows users to seamlessly integrate their custom models for pre-labeling datasets. Fig 5. Instantly Segment Anything in Encord Learn how to annotations medical data Encord Security and Compliance Encord strictly follows compliance standards, such as HIPAA and GDPR. It uses military-grade encryption, and all your information is encrypted at rest with AES-256. The annotation platform offers flexible deployment options, including VPC and on-premises solutions, to meet the needs of organizations with strict data protection requirements. Continuous security monitoring and multi-layered, role-based access controls are also in place. Supervisely Supervisely is a computer vision platform that focuses on surgical video annotation and DICOM file management. It supports multi-planar labeling across coronal, sagittal, and axial planes, providing a comprehensive view for annotators. Supervisely's video labeling tools enable automatic object tracking, detection, and segmentation on videos by using state-of-the-art (SOTA) neural networks. It integrates advanced models, including Meta AI's SAM, MixFormer, RITM, ClickSeg, and EiSeg. These models allow annotators to provide real-time feedback for correcting model predictions, streamlining the process. Supervisely’s privacy features, such as data encryption and data anonymization, align with healthcare regulations. It supports deployment on all major cloud providers (AWS, GCP, Azure) with flexible configurations. Its user-friendly interface makes it ideal for annotating surgical videos for AI-assisted surgery, surgical robotics, and clinical research. OHIF OHIF is an open-source platform for viewing and annotating medical images, including video data. It supports various medical imaging formats, including DICOM. It uses Cornerstone3D for its strong annotation features, enabling tasks such as surgical video analysis and diagnostic imaging. OHIF features an advanced video viewport for imaging workflows, supporting HTML5 video streams. This enables precise frame-by-frame analysis and longitudinal video annotations. Its web-based interface provides easy access and real-time collaboration among medical professionals, enhancing workflow efficiency. OHIF also introduced AI-powered Labelmap Assist for quickly extending an existing segmentation to the next or previous slice using the Segment Anything (SAM) model. Fig 8. Automatic Labelmap Slice Interpolation Labellerr Labellerr is a video annotation platform known for precision, scalability, and AI integration. It provides tools and solutions for the healthcare and biotechnology sectors. It has a strong focus on reliable data annotation for medical AI applications while adhering to regulations like HIPAA and GDPR. Labellerr offers DICOM annotation tools and supports both 2D and 3D medical image formats, including DICOM and NIfTI. It also works with 2D formats like X-rays and CT scans. The platform handles various types of video data, ensuring broad applicability across different medical video sources. Fig 9. Labeller Bitmask Annotation The platform speeds up the annotation process through its AI assistance and smart feedback loop, leading to 10 times faster labeling. Automated labeling is applied to tasks such as identifying tumors, fractures, and organ structures in medical scans, reducing manual effort and improving accuracy. CVAT CVAT is an open-source image and video annotation tool. Its open-source architecture allows customization. This allows users to adapt it for specific medical imaging files like DICOM or NIfTI, which are not natively supported but can be integrated. CVAT supports a variety of annotation types, including bounding boxes, polygons, and skeletons, with interpolation for efficient labeling of video frames. As an open-source solution, CVAT provides users with a high degree of control over their data and environment. However, self-hosting CVAT in a highly secure, airtight server environment (e.g., without internet access) can present significant challenges. Fig 10. CVAT Video Annotation Kili Technology Kili's video annotation tool supports all common video formats and annotation types. This includes bounding boxes, polygons, and semantic segmentation, suitable for medical videos. Its data management supports help teams manage long videos with over 100,000 frames and multiple objects per frame. The platform’s AI-driven workflows use its Model-in-the-loop feature, which enables users to connect their models and generate pre-annotations. It also enhances segmentation tasks with foundation models such as SAM. Fig 11. Kili's Video Annotation Tool Kili Technology is SOC2 and HIPAA certified, ensuring privacy and protection against bias. It provides secure data storage on its platform or connects to popular cloud providers like Azure, Google, or AWS. The platform also supports Single Sign-On (SSO) for easy and secure access. RedBrick AI RedBrick AI is a SaaS application for medical image viewing and video annotation. It helps healthcare AI teams create quality outcomes datasets. The tool supports all radiology modalities, including X-ray, CT, MRI, and Ultrasound, as well as 2D and 3D formats. Additionally, it supports video formats and medical data formats like DICOM, NRRD, NIfTI, and MP4. RedBrick AI includes an Auto Annotator, an automatic segmentation tool powered by Meta AI's SAM. It helps generate 2D and 3D segmentation masks for hundreds of structures on CT and MR images. Fig 12. RedBrick AI Labeling Its Mask Propagation Tool allows users to annotate a single slice and then propagate that mask across a defined range of slices, making volumetric annotation much faster. RedBrick AI follows HIPAA standards, supporting radiology AI teams in building training datasets for diagnostics. 3D Slicer 3D Slicer is an open-source platform for 3D medical image and video annotation, widely used in research. It supports DICOM, NIfTI, and medical videos, with modules for time-series annotation like surgical procedures. The tool supports DICOM standard interoperability, including 2D, 3D, and 4D images, segmentation objects, and structured reports. It also provides volume rendering, surface rendering, and slice display, enabling medical data to be presented in various ways. Additionally, 3D Slicer integrates AI-assisted annotation tools that can automatically segment anatomical structures using pre-trained or custom models. It supports NVIDIA Clara AI-based automatic segmentation, along with a MONAI plugin for 3D volumes segmentation annotation. Fig 13. 3D Slicer AI-assisted Segmentation Why Encord Stands Out While all the listed tools above offer valuable capabilities for healthcare AI, Encord is particularly noteworthy. Its tailored features directly address the strict demands of medical video. Unlike many general-purpose annotation platforms, Encord is optimized explicitly for medical imaging. It offers native DICOM and NIfTI support, which is essential for managing complex multidimensional data in medical imaging. Encord is an enterprise-grade platform built to manage petabytes of medical data and multiple users without performance degradation. Its project management features make managing complex annotation project pipelines easier. The platform’s powerful tools enable high-throughput video labeling at scale. Encord offers collaboration tools that allow annotators, reviewers, and domain experts to work while maintaining strict access controls. It is SOC 2 Type II, HIPAA, and GDPR compliant, and uses military-grade encryption. This commitment to security ensures confidence in developing AI solutions in regulated healthcare environments. Learn how to automate video annotation Key Takeaways Video labeling tools are important for creating trustworthy healthcare AI systems. They help convert raw video data into quality annotated datasets that power machine learning algorithms for diagnostics, treatment planning, and clinical automation. These tools must handle complex medical imaging formats, support collaboration, and comply with healthcare regulations to ensure safe AI deployment. Below are key points to remember when selecting and using video annotation tools for healthcare AI projects. Best Use Cases for Video Annotation Tools: The most effective video annotation tools in healthcare are used for surgical video analysis, segmentation in radiology, and training models for medical robotics. These use cases demand pixel-level accuracy, expert-driven labeling, and audit-ready documentation. Challenges in Healthcare Annotation: Healthcare video annotation presents challenges. These include managing large-scale datasets, ensuring PHI security, and meeting HIPAA and FDA compliance. Tools must also support 3D and 4D data and streamline annotation workflows. Encord for Healthcare AI: Encord supports various medical imaging formats. Its AI-assisted approach to labeling videos helps teams optimize speed and consistency in producing annotated datasets. Other tools, such as Supervisely, V7, Kili, Labellerr, and RedBrick AI, also offer strong healthcare-focused capabilities. The best choice depends on your data types, project scale, regulatory needs, and workflow priorities.
Jul 02 2025
5 M
Webinar Recap: Build Smarter VLMs, Faster - How to Bootstrap With Existing ML Solutions
In a joint session hosted by our ML Lead Frederik and Neuron’s Head of AI, Doogesh, we pulled back the curtain on what it really takes to turn machine learning (ML), large language models (LLMs), and neuroscience-powered insights into systems that drive measurable ROI. Below are the key takeaways from this insight-packed webinar. How to go from ML models to measurable impact Most companies already use ML in production, but not all of them are extracting business value. This webinar provided a blueprint for bridging that gap: Align model behavior with business goals: Models are only as good as their outcomes. Ensuring alignment with ROI and implementing human-in-the-loop are critical. Unify models into VLMs: Combine Claude, GPT, and proprietary neuroscience models into vertically integrated vision-language systems (VLMs) for performance that scales. Move from suggestion to action: Battle-tested recommendation systems become the foundation for guiding GenAI tools development. Neurons AI: Science-powered ad recommendations Doogesh, Head of AI at Neurons, introduced the audience to how they deliver unlimited AI-driven ad recommendations backed by neuroscience. Here’s how Neurons has used AI to transform advertising performance: Pre-test ads instantly: No more waiting on A/B testing; get results in seconds. Visual Attention Prediction: Eye-tracking and heatmaps predict what grabs users' focus. Optimize messaging and recall: Get early signals on engagement, recall, and cognitive load. Benchmarking across industries: Track performance against industry-specific standards for more accurate insights. How neuroscience powers better ML Through tests like the Visual Memory Game (VMG) and Fast Response Test (FRT), Neurons collects high-quality data on: Memory: Identifying what users remember from fast image streams. Engagement: Reaction speed shows how compelling a visual is. Focus: Area-of-interest (AOI) heatmaps reveal content clarity. Cognitive Load & Demand: EEG and entropy-based metrics quantify complexity. This rich dataset feeds into their CIS score—a single, weighted measure that correlates strongly with ROI. ✅ Key Insight: CIS scores can serve as evaluation metrics in RL setups for autonomous recommendation systems. Industry & segment specificity matters Doogesh emphasized that not all ads, or audiences, are created equal: Different industries and demographics respond to different visual cues Models trained on generalized data underperform unless tuned to specific audience behaviors Benchmarking across segments and geographies helps ensure accurate and trusted recommendations Industry segmentation for benchmarking Annotation of advertisement using image annotation tool, Encord Models trained on generalized data underperform unless tuned to specific audience behaviors. Blueprint for VLM-powered recommendation systems Frederik and Doogesh then provided some a generalized framework for building actionable recommendation engines: Step 1: Build production-grade ML models Let them serve as the foundation for understanding what drives value today. Step 2: Feed insights into a recommender system LLMs act as judges, vibing off human edits, customer feedback, and content scoring systems like CIS. Step 3: Create agentic systems Use data and evaluation pipelines to enable iterative, autonomous systems that learn, act, and improve with minimal human input. “What activities that add value today also generate data for tomorrow’s AI?” From Evaluation to Autonomy As performance benchmarks stabilize, we move closer to autonomous agents that: Generate, evaluate, and adapt creatives Collect real-time feedback like click-through rates Train on their own outputs to self-improve. But this shift hinges on: Strong content scoring systems (like CIS), Reliable evaluation pipelines, and Rich historical data for training. Final Framework: A 3-Step Rocket to ROI 1. Build ML that Works Use neuroscience data and LLMs to create foundational models. 2. Use Models to Guide Recommendations Extract insights, benchmark, and personalize at scale. 3. Turn Recommendations into Agentic Tools Close the loop with systems that act autonomously and improve over time. Closing Thoughts The Encord x Neurons collaboration shows a future where ML systems are no longer siloed black boxes, but value-generating engines deeply embedded into business strategy, backed by science, powered by LLMs, and guided by ROI.
Jul 01 2025
5 M
Scale AI Alternatives: Why AI Teams Choose Encord
On June 12 2025 Meta confirmed a $15 billion investment for a 49 % non-voting stake in Scale AI—and hired CEO Alexandr Wang to run its new “super-intelligence” group. Customers such as Google have already paused work with the platform and Encord is experiencing and influx of customers looking for alternative to Scale. If you need vendor neutrality or faster throughput, now is the ideal moment to benchmark alternatives offering: lower or more predictable unit economics, modern quality-assurance workflows and audit trails, seamless SDK and API integrations, and true multimodal support, including emerging 3D sensors. The best AI teams are moving to Encord because these features matter to them. Encord is the scalable multimodal data engine alternative to Scale Encord’s platform indexes, curates, and annotates petabyte-scale datasets spanning images, video, DICOM, audio, documents, and—as most recently—LiDAR and point-cloud data. The new Physical AI suite unifies 3D, camera, and radar streams inside one labeling workflow, giving robotics and autonomy teams a single pane of glass for perception data. The influx of customers migrating from Scale to Encord are mentioning that these aspects matter the most to them: Encord's LiDAR launch: ingest MCAP or LAS files, visualize multi-sensor scenes, and auto-propagate 3D boxes across sequences. Encord scales from single-GPU prototypes to petabyte deployments without re-architecting. The platform works on-prem, in VPC, or SaaS; SDK covers import → train → export. Encord offers a suite of tools designed to accelerate the creation of training data. Encord's annotation platform is powered by AI-assisted labeling, enabling users to develop high-quality training data and deploy models up to 10 times faster. Encord’s active learning toolkit allows you to evaluate your models, and curate the most valuable data for labeling. See also the detailed Scale vs Encord comparison for pricing and workflow nuances. 📌 Try Encord Free – The Active Learning Platform Trusted by Industry Leaders Encord ML Pipeline & Features State-of-the-art AI-assisted labeling and workflow tooling platform powered by micro-models Perfect for image, video, DICOM, and SAR annotation, labeling, QA workflows, and training computer vision models Native support for a wide range of annotation types, including bounding box, polygon, polyline, instance segmentation, keypoints, classification, and more Easy collaboration, annotator management, and QA workflows to track annotator performance and ensure high-quality labels Utilizes quality metrics to evaluate and improve ML pipeline performance across data collection, labeling, and model training stages Effortlessly search and curate data using natural language search across images, videos, DICOM files, labels, and metadata Auto-detect and resolve dataset biases, errors, and anomalies like outliers, duplication, and labeling mistakes Export, re-label, augment, review, or delete outliers from your dataset Robust security functionality with label audit trails, encryption, and compliance with FDA, CE, and HIPAA regulations Expert data labeling services on-demand for all industries Advanced Python SDK and API access for seamless integration and easy export into JSON and COCO formats Integration and Compatibility Encord offers robust integration capabilities, allowing users to import data from their preferred storage buckets and build pipelines for annotation, validation, model training, and auditing. The platform also supports programmatic automation, ensuring seamless workflows and efficient data operations. Benefits and Customer Feedback Its users have received Encord positively, with many highlighting the platform's efficiency in reducing false acceptance rates and its ability to train models on high-quality datasets. The platform's emphasis on AI-assisted labeling and active learning has been particularly appreciated, ensuring accurate and rapid training data creation. Learn more about how computer vision teams use Encord instead of Scale Vida reduce their model false positive from 6% to 1% Floy reduce CT & MRI annotation times by ~50% Stanford Medicine reduce experiment time by 80% King's College London increase labeling efficiency by 6.4x Tractable go through hyper-growth supported by faster annotation operations As we delve into the best Scale AI alternatives, we'll explore platforms that offer an enhanced user experience, specializing in data labeling, cater to large-scale operations, and can handle many users. From platforms that leverage neural networks to those that focus on transcription, the future of AI is diverse and promising. Find a summary of these tools in the table below: An Overview of Scale AI Scale AI was a provider of data annotation and ML operations solutions, designed to accelerate the development and deployment of machine learning models. It supported a wide range of industries, including autonomous vehicles, e-commerce, and robotics. It is unclear how the company will change post the acquisition. ML Pipeline & Features Supports annotations for images, videos, LiDAR, text, and more, enabling diverse use cases like object detection, sentiment analysis, and 3D mapping. Combines machine learning algorithms with human oversight to reduce annotation time and improve accuracy. Customizable workflows for quality assurance, collaboration, and project tracking. Handles projects of varying complexities, from small datasets to enterprise-level needs, ensuring scalability. Multi-layer quality checks and advanced metrics to ensure dataset reliability and consistency. Real-time insights into labeling performance, dataset health, and model readiness. Integration and Compatibility Scale AI offers robust integrations with major cloud platforms like AWS, Google Cloud, and Azure. Its APIs allow seamless connection to data pipelines and machine learning frameworks, enabling an efficient end-to-end ML workflow. The platform supports JSON, COCO, and other formats for easy data export. Benefits and Customer Feedback Scale AI is praised for its ability to streamline the ML data pipeline, reduce project turnaround times, and maintain high-quality output across large-scale projects. Its customers highlight the platform’s flexibility, scalability, and robust automation features as critical enablers of faster machine learning development. However, its cost and steep learning curve can be challenging for smaller teams. It also may lack flexibility for niche use cases and requires careful integration into existing workflows. iMerit iMerit specializes in providing data annotation solutions, including those for LiDAR, which is crucial for applications like autonomous vehicles and robotics. With a focus on complex data types, iMerit ensures high precision and quality in its annotations, making it a preferred choice for industries that require intricate data labeling. ML Pipeline and Features Expertise in LiDAR data annotation, ensuring accurate and high-quality annotations While Scale AI is known for its broad range of data labeling services, iMerit's strength lies in its specialization in complex data types, most notably LiDAR Robust integration options, allowing seamless connection with various platforms and tools Various tools and platforms for efficient data annotation and management Emphasis on compliance and data protection, ensuring that businesses can trust them with their sensitive data Benefits and Customer Feedback iMerit has garnered positive feedback from its clientele, particularly for its expertise in LiDAR data annotation. Many users have highlighted the platform's precision, efficiency, and quality of annotations. The platform's ability to handle complex data types and provide tailored solutions has been particularly appreciated, making it a go-to solution for industries like autonomous driving and robotics. Refer to the G2 Link for customer feedback on the iMerit platform. Dataloop Dataloop, an AI-driven data management platform, is tailored to streamline the process of generating data for AI. While Scale AI is recognized for its human-centric approach to data labeling, Dataloop differentiates itself with its cloud-based platform, providing flexibility and scalability for organizations of all sizes. ML Pipeline & Features Streamlines administrative tasks efficiently, organizing management and numerical data. Dataloop's object tracking and detection feature stands out, providing users with exceptional data quality Requires a stable and fast internet connection, which might pose challenges in areas with connectivity issues. Integration and Compatibility Dataloop, being a cloud-based platform, offers the advantage of flexibility. However, it also requires a stable and fast internet connection, which might pose challenges in areas with connectivity issues. Despite this, its integration capabilities ensure users can seamlessly connect their data sources and ML models to the platform. Benefits and Customer Feedback Dataloop has received positive feedback from its users. Users have noted the platform's scalability and flexibility, making it suitable for both small projects and larger needs. However, some users have pointed out that the user interface can be challenging to navigate, suggesting the need for tutorials or a more intuitive design. Here is the G2 link for customer reviews on the Dataloop platform. SuperAnnotate SuperAnnotate offers tools to streamline annotation. Their platform is equipped with tools and automation features that enable the creation of accurate training data across multiple data types. SuperAnnotate's offerings include the LLM Editor, Image Editor, Video Editor, Text Editor, LiDAR Editor, and Audio Editor. ML Pipeline & Features Features like data insights, versioning, and a query system to filter and find relevant data Marketplace of over 400 annotation teams that speak 18 languages. This ensures high-quality annotations tailored to specific regional and linguistic requirements Dedicated annotation project managers, ensuring stellar project delivery Annotation tools for different data types, from images and videos to LiDAR and audio Certifications like SOC 2 Type 2, ISO 27001, and HIPAA Data integrations with major cloud platforms like AWS, Azure, and GCP Benefits and Customer Feedback SuperAnnotate has received positive user feedback, with companies like Hinge Health praising the platform's high and consistent quality. Refer to the G2 link for customers' thoughts about the SuperAnnotate platform. Labelbox Labelbox, a leading data labeling platform, is designed to focus on collaboration and automation. It offers a centralized hub where teams can create, manage, and maintain high-quality training data. Labelbox provides tools for image, video, and text annotations. ML Pipeline & Features Labelbox supports data collection to model training Features include MAL (Model Assisted Labeling), which uses pre-trained models to accelerate the labeling process Easy collaboration, allowing multiple team members to work on the same dataset and ensuring annotation consistencyReviewer Workflow feature enables quality assurance by allowing senior team members to review and approve annotations Ontology Manager provides a centralized location to manage labeling instructions, ensuring clarity and consistency API integrations, allowing users to connect their data sources and ML models to the platform Supports integrations with popular cloud storage solutions Integration and Compatibility Labelbox offers API integrations, allowing users to connect their data sources and ML models seamlessly to the platform. This ensures a workflow from data ingestion to model training. The platform also supports integrations with popular cloud storage solutions, ensuring flexibility in data management. Here is the G2 link for customer reviews about the LabelBox platform. Prolific Prolific, a human data platform, accelerates AI development by providing direct access to verified participants for training, alignment, and evaluation data. Prolific's API-first architecture integrates human feedback directly into existing ML tools and workflows. This enables AI researchers and developers to source authentic, high-quality human data for their specific use cases in hours, not weeks. ML Pipeline & Features Support for varied AI alignment and evaluation needs including RLHF, model evaluation, factuality testing and real-world user experience testing 200K+ active participants across 40+ countries and 80+ languages 300+ screeners for precise participant targeting Pre-qualified AI taskers and verified domain experts across fields like STEM, languages, programming Built-in quality controls with ability to screen participants, monitor performance, and build persistent expert pools that improve with your products over time API-first infrastructure along with an intuitive UI Option to use either the self-serve platform or fully managed services Batch data collections and always-on data pipelines for continuous improvement Integration and Compatibility Prolific's API-first architecture integrates with any of your existing ML workflows and tools. The platform supports both batch data collections and always-on data pipelines. Data export is available in standard formats with webhook support for automated data ingestion. As an Encord partner, Prolific provides complementary human intelligence capabilities that enhance annotation workflows. Benefits and Customer Feedback Prolific is valued by AI teams at organizations such as Google, AI2 and Hugging Face for making AI development fast and scalable - dramatically reducing time-to-data from weeks to hours while delivering production-grade data quality. Users particularly appreciate the transparency of knowing exactly who is providing feedback, the ability to precisely target participant demographics and expertise, and the flexibility to scale from small pilots to production-level data collection. The platform excels at tasks requiring genuine human judgment - from model evaluation and bias detection to user experience testing for AI products. Scale Alternatives: Key Takeaways after the acquisition: Meta’s stake introduces potential conflicts of interest; several hyperscalers have paused new Scale AI projects. Encord now leads for scalable multimodal pipelines, adding LiDAR/3D support and active learning to compress annotation cycle times by up to 10 ×. Specialized tools still win in niche domains—iMerit for LiDAR labeling services, V7 for medical imaging—while Labelbox appeals to collaboration-heavy enterprises. Before migrating, pilot two short projects: one “easy” class and one edge-case-heavy class. Measure unit economics, cycle time, and F1 uplift side-by-side. Scale’s interactive platform has been recognized for its excellent automation and streamlined workflows tailored for various use cases. While many platforms in the market are open-source, Scale AI's proposition lies in its focus on machine learning and AI-powered algorithms. The platform offers a range of plugins and tools that provide metrics and insights in real-time. With its robust API integrations, it seamlessly connects with platforms like Amazon, ensuring that artificial intelligence is leveraged to its full potential. In this rapidly evolving domain, optimizing workflows and harnessing the power of natural language processing is paramount. Here are our key takeaways: The AI domain is witnessing a transformative phase with new platforms and tools emerging. As industries seek efficient data labeling and management solutions, platforms like Encord are becoming indispensable. Encord's AI-assisted labeling accelerates the creation of high-quality training data, making it a prime choice in this evolving landscape. One of the standout features of modern AI platforms is the ability to harness AI for faster and more accurate data annotation. Encord excels in this with its AI-powered labeling, enabling users to annotate visual data swiftly and deploy models up to 10 times faster than traditional methods. 📌 Experience AI-assisted labeling, model training, and error detection all in one place. Join the world’s leading computer vision teams and bring your AI projects to production faster. Start Your Free Trial with Encord
Jun 21 2025
8 M
Encord Releases New Physical AI Suite with LiDAR Support
We’re excited to introduce support for 3D, LiDAR and point cloud data. With this latest release, we’ve created the first unified and scalable Physical AI suite, purpose-built for AI teams developing robotic perception, VLA, AV or ADAS systems. With Encord, you can now ingest and visualize raw sensor data (LiDAR, radar, camera, and more), annotate complex 3D and multi-sensor scenes, and identify edge-cases to improve perception systems in real-world conditions at scale. 3D data annotation with multi-sensor view in Encord Why We Built It Anyone building Physical AI systems knows it comes with its difficulties. Ingesting, organizing, searching, and visualizing massive volumes of raw data from various modalities and sensors brings challenges right from the start. Annotating data and evaluating models only compounds the problem. Encord's platform tackles these challenges by integrating critical capabilities into a single, cohesive environment. This enables development teams to accelerate the delivery of advanced autonomous capabilities with higher quality data and better insights, while also improving efficiency and reducing costs. Core Capabilities Scalable & Secure Data Ingestion: Teams can automatically and securely synchronize data from their cloud buckets straight into Encord. The platform seamlessly ingests and intelligently manages high-volume, continuous raw sensor data streams, including LiDAR point clouds, camera imagery, and diverse telemetry, as well as commonly supported industry file formats (such as MCAP). Intelligent Data Curation & Quality Control: The platform provides automated tools for initial data quality checks, cleansing, and intelligent organization. It helps teams identify critical edge cases and structure data for optimal model training, including addressing the 'long-tail' of unique scenarios that are crucial for robust autonomy. Teams can efficiently filter, batch, and select precise data segments for specific annotation and training needs. 3D data visualization and curation in Encord AI-Accelerated & Adaptable Data Labeling: The platform offers AI-assisted labeling capabilities, including automated object tracking and single-shot labeling across scenes, significantly reducing manual effort. It supports a wide array of annotation types and ensures consistent, high-precision labels across different sensor modalities and over time, even as annotation requirements evolve. Comprehensive AI Model Evaluation & Debugging: Gain deep insight into your AI model's performance and behavior. The platform provides sophisticated tools to evaluate model predictions against ground truth, pinpointing specific failure modes and identifying the exact data that led to unexpected outcomes. This capability dramatically shortens iteration cycles, allowing teams to quickly diagnose issues, refine models, and improve AI accuracy for fail-safe applications. Streamlined Workflow Management & Collaboration: Built for large-scale operations, the platform includes robust workflow management tools. Administrators can easily distribute tasks among annotators, track performance, assign QA reviews, and ensure compliance across projects. Its flexible design enables seamless integration with existing engineering tools and cloud infrastructure, optimizing operational efficiency and accelerating time-to-value. Encord offers a powerful, collaborative annotation environment tailored for Physical AI teams that need to streamline data labeling at scale. With built-in automation, real-time collaboration tools, and active learning integration, Encord enables faster iteration on perception models and more efficient dataset refinement, accelerating model development while ensuring high-quality, safety-critical outputs. Implementation Scenarios ADAS & Autonomous Vehicles: Teams building self-driving and advanced driver-assistance systems can use Encord to manage and curate massive, multi-format datasets collected across hundreds or thousands of multi-hour trips. The platform makes it easy to surface high-signal edge cases, refine annotations across 3D, video, and sensor data within complex driving scenes, and leverage automated tools like tracking and segmentation. With Encord, developers can accurately identify objects (pedestrians, obstacles, signs), validate model performance against ground truth in diverse conditions, and efficiently debug vehicle behavior. Robot Vision: Robotics teams can use Encord to build intelligent robots with advanced visual perception, enabling autonomous navigation, object detection, and manipulation in complex environments. The platform streamlines management and curation of massive, multi-sensor datasets (including 3D LiDAR, RGB-D imagery, and sensor fusion within 3D scenes), making it easy to surface edge cases and refine annotations. This helps teams improve how robots perceive and interact with their surroundings, accurately identify objects, and operate reliably in diverse, real-world conditions. Drones: Drone teams use Encord to manage and curate vast multi-sensor datasets — including 3D LiDAR point clouds (LAS), RGB, thermal, and multispectral imagery. The platform streamlines the identification of edge cases and efficient annotation across long aerial sequences, enabling robust object detection, tracking, and autonomous navigation in diverse environments and weather conditions. With Encord, teams can build and validate advanced drone applications for infrastructure inspection, precision agriculture, construction, and environmental monitoring, all while collaborating at scale and ensuring reliable performance Vision Language Action (VLA): With Encord, teams can connect physical objects to language descriptions, enabling the development of foundation models that interpret and act on complex human commands. This capability is critical for next-generation human-robot interaction, where understanding nuanced instructions is essential. For more information on Encord's Physical AI suite, click here.
Jun 12 2025
5 M
Best Video Annotation Tools for Logistics 2025
This guide to video annotation tools for logistics breaks down the essentials of turning raw video data into actionable AI insights with real-world use cases, top annotation platforms, and key features that boost accuracy, automation, and operational efficiency. Artificial Intelligence (AI) is transforming the logistics industry by bringing in high levels of efficiency, safety, and operational insight like never seen before. As warehouses, distribution centers, and transportation networks largely depend upon visual data, i.e. from security cameras to drone footage, the need to accurately understand and make use of this data is very important. The logistics industry deals with an enormous amount of video data every single day, capturing crucial activities such as package handling, vehicle movements, inventory stocking, and workforce operations. To truly leverage the power of AI, this raw visual data needs to be transformed into structured insights that AI models can comprehend and analyze. This is where video annotation becomes important. Video annotation involves labeling of video data to identify and track objects, recognize actions, and highlight significant events. This annotated video data is essential for machine learning (ML) models. The ML model trained on this data enables various tasks in logistics such as real-time asset tracking, predictive maintenance, automated inventory management, and compliance monitoring etc. So, picking the right video annotation tool is a crucial decision. The success of AI applications in logistics heavily relies on the accuracy of annotations, the efficiency of workflows, and how well the tool integrates with existing systems. The best annotation tools not only make the labeling process easier but also ensure high-quality annotations, support collaborative workflow management, and integrate smoothly with current logistics systems. Video annotation example in Encord What Is Video Annotation in Logistics? In logistics setup, video annotation is the process of labeling, tracking, and segmenting objects or events captured in video data. It transforms raw visual data into structured datasets that are suitable for training ML models. Logistics operations have specific annotation requirements, such as accurately tracking multiple moving objects (e.g., packages, vehicles, forklifts), monitoring vehicle flow in busy distribution centers, handling packages efficiently, and precisely detecting significant operational events. For example, a video stream can be annotated to track package handovers from one employee to another, identify loading and unloading sequences at docks, monitor inventory movement on warehouse shelves, and flag anomalies or potential safety hazards in real-time. The ML model trained on such annotated video data optimizes operational processes, enhances security measures, and reduces the human error that ultimately leads to a smarter and more efficient logistics process. Why Video Annotation Matters for Logistics AI In logistics, AI systems depend fundamentally on annotated video data. To determine how effectively the AI model can perform in a real-world environment, the quality and accuracy of these annotations is very important. High-quality annotations ensure that AI models are trained well and can precisely detect, interpret, and predict critical logistics tasks. Such models lead to significant improvements in the efficiency of the logistics operations. The relationship between annotation quality and AI outcomes in logistics is direct and powerful for the following reasons: Accuracy of Object Detection: AI systems trained on accurately annotated videos can detect and track packages, vehicles, workers, and equipment etc. accurately. Even minor inaccuracies in labeling can result in misidentification or tracking errors, potentially disrupting the entire supply chain operation. Route Optimization: High-quality annotations enable AI models to accurately identify patterns in vehicle movements and identify and track delays, congestion, or inefficiencies in warehouse flows. Accurate annotation ensures the AI can recommend better routes and optimize vehicle scheduling to reduce or remove delays. Event Recognition: Clear and accurate annotation of key events (such as package drop-off, pick-up, delays, or security breaches) allows AI systems to learn and detect anomalies promptly and improve response times and operational reliability. Following are the common AI use cases in logistics that rely on accurate video annotations: Automated Parcel Tracking Annotated video data helps AI track packages throughout sorting centers, warehouses, and delivery vehicles. By accurately annotating each handover or movement, AI models become capable of real-time tracking. This improves tracking of package movements in the logistic workflow. It reduces errors due to manual tracking and ensures timely deliveries. Forklift and Vehicle Navigation Video annotations that involve annotation of navigation routes, vehicle movements, obstacles, and worker paths are important for training AI powered autonomous forklifts and vehicles. Accurately annotated data enables safe navigation, operational efficiency, and smooth interactions between humans and machines. This helps to minimize workplace accidents and streamline the processes. Inventory and Shelf Monitoring Accurately annotated video data of warehouse shelves and storage areas allows AI models to accurately assess inventory levels, spot out-of-stock items, and automatically initiate replenishment workflows. These precise annotations enhance predictions, improve inventory accuracy, and help maintain optimal stock levels. Security and Safety Incident Detection Annotating incidents like unauthorized access, falls, collisions, or protocol breaches helps train AI models that can accurately identify and respond to these events. High-quality annotations ensure that AI systems can quickly alert personnel, helping to prevent accidents, theft, and reduce liabilities. Predictive Maintenance via Video Streams AI models can predict potential failures or maintenance needs before they arise. It requires annotated video data to monitor machinery conditions, vehicle performance, or environmental factors. Accurate annotations of such data is important for differentiating between normal operating conditions and early-stage anomalies. This helps to minimize downtime and lower maintenance costs. Key Features to Look for in a Logistics Video Annotation Tool When choosing a video annotation tool specifically for training AI models for logistics applications, it is important to understand the unique demands of logistics workflows. The following are the essential features of a video annotation tool that has to be used to prepare dataset for training AI models for logistic applications. Multi-object and Multi-class Tracking In logistics, operational scenes often include multiple objects and classes simultaneously such as parcels, workers, forklifts, delivery vehicles, and various equipment moving dynamically in complex environments. A video annotation tool must: Enable simultaneous annotation and tracking of numerous objects across frames. Maintain consistent tracking IDs to accurately follow each object's trajectory. Provide easy labeling for different classes, clearly distinguishing between object types (e.g., “worker,” “forklift,” “parcel,” etc.). Allow quick review and adjustments to ensure consistent labeling, especially in crowded, fast-paced logistics hubs. Multi-Object Tracking in Encord Frame-accurate Event Tagging In logistics processes various events must be precisely recognized, such as package pickups, deliveries, or anomaly detection like accidental drops or equipment malfunctions. Therefore a video annotation tool should: Allow annotators to pinpoint exact frames where an event occurs. Facilitate precise temporal annotations to clearly define the start and end times of events. Offer visual timelines or video playback control to ease frame-accurate tagging. Event tagging in Encord using Dynamic Attribute Flexible Workflow Management Logistics operations generate large amounts of continuous video data from multiple cameras located at different points within facilities and across transportation routes. The video annotation tool must: Easily manage batch processing of thousands of video files or continuous video streams. Allow customizable workflows to suit different annotation tasks (e.g., simple object labeling versus detailed event annotations). Provide intelligent assignment of tasks, handling simultaneous inputs from multiple annotators seamlessly. Workflow in Encord Collaboration and Quality Assurance (QA) Features Annotation projects often involve distributed teams working remotely or from different locations. Thus, the tool should have strong collaborative capabilities: It should enable real-time collaborative annotation, allowing multiple annotators and reviewers to work concurrently without conflicts. Incorporate role-based access control so administrators can assign tasks, permissions, and project visibility. Include robust QA workflows, offering multi-stage annotation review, feedback loops, and clear audit trails. Provide version control and annotation histories to review edits, corrections, and improve accountability across teams. Scalability A typical logistics annotation project involves annotating potentially thousands of hours of video footage that demand substantial scalability. Therefore the video annotation tool must: Support handling of extremely large-scale datasets without performance degradation. Offer cloud-based scalability to expand annotation resources quickly when data volumes increase. Provide features to automate repetitive workflows. AI-assisted & Automated Annotation AI assistance can accelerate annotation tasks and improve accuracy. It also reduces manual workload for repetitive scenes. COnsidering this, the good video annotation tool should: Incorporate pre-trained models to automate annotation of common objects and scenarios. Enable active learning loops, where annotation feedback continually refines AI suggestions for improved accuracy. Include auto-tracking for objects moving predictably to reduce manual annotation time. AI-Assisted Annotation for All Your Visual Data in Encord Annotation Types Training AI models for Logistics scenarios often require flexible annotation formats depending on the complexity and precision. Therefore a video annotation too must provide following annotation types: Bounding Boxes: Ideal for simple, consistent tracking of objects like parcels, vehicles, or equipment. Polygonal Annotations: Essential for detailed shape annotation of irregularly-shaped packages, forklifts, equipment, or loading areas. Polyline Annotations: Useful for marking paths, conveyor belts, forklift trajectories, or route paths within warehouses or sorting centers. Key-point Annotation: Helpful for marking specific parts of objects or equipment (e.g., door handles, package labels, equipment parts for predictive maintenance). Keypoint annotation can also be used to annotate human workers to analyze their pose. Keypoint annotation in Encord Selecting a video annotation tool equipped with these comprehensive features ensures that logistics AI systems receive the precise, reliable, and actionable data needed for training a robust AI model to improve optimal operational performance and strategic advantage. Tools Overview The Best Video Annotation Tools for Logistics Encord Encord is a complete, enterprise-grade data annotation platform specifically built for managing large-scale, complex annotation tasks efficiently. It combines advanced automation, collaboration features, and a highly customizable workflow environment ideal for demanding logistics applications. Multi-object & Multi-class Tracking: Encord excels with robust multi-object tracking, maintaining precise IDs across frames, ideal for complex logistics scenarios involving numerous parcels, vehicles, and workers. Frame-accurate Event Tagging: Encord supports Dynamic Attributes annotations that enable accurate event identification (e.g., parcel handoffs, forklift movements, delivery drop-offs). Flexible Workflow Management: Encord offers workflow customization, task management, and easy integration into logistics systems, handling massive streams efficiently. Collaboration & QA Features: Encord provides strong team management, real-time collaboration, comprehensive review cycles, annotation history/versioning, and detailed QA workflows. Scalability: Encord is highly scalable cloud architecture capable of annotating thousands of hours of logistics footage efficiently. AI-assisted & Automated Annotation: Encord offers AI-assisted labeling, active learning, and auto-tracking capabilities to improve annotation efficiency. Annotation Types: Encord supports bounding boxes, polygons, polylines, keypoints, segmentation masks which are essential for logistics annotation needs. AI-Assisted Video annotation in Encord CVAT (Computer Vision Annotation Tool) CVAT is an open-source data annotation platform with support for video annotation. It is popular among researchers and businesses due to its flexibility, user-friendly interface, and robust annotation capabilities. It is particularly suitable for teams requiring customizable solutions. Multi-object & Multi-class Tracking: CVAT supports multi-object tracking with interpolation between frames which is suitable for moderately complex logistics use cases. Frame-accurate Event Tagging: CVAT offers detailed timeline controls for precise event labeling. Flexible Workflow Management: CVAT requires some setup for handling extensive datasets or complex logistics workflows. Collaboration & QA Features: CVAT supports basic collaboration with task assignment and review cycles. However, enterprise-level QA features may require custom setup. Scalability: CVAT has good scalability for medium-sized projects. The large-scale logistics operations might require custom infrastructure tuning. AI-assisted & Automated Annotation: CVAT offers basic auto-labeling via external integrations and support for automatic interpolation between keyframes. Annotation Types: CVAT provides bounding boxes, polygons, polylines, and segmentation masks which are flexible for diverse logistics scenarios. Video annotation in CVAT Supervisely Supervisely is a versatile AI data labeling platform emphasizing collaboration, automation, and ease-of-use. Known for its intuitive interface and robust tracking features, Supervisely is a good choice for teams looking to manage smooth annotation workflows. Multi-object & Multi-class Tracking: Supervisely supports advanced multi-object tracking and labeling objects across video frames. Frame-accurate Event Tagging: Supervisely provides video timeline annotation tools for precise event marking like parcel deliveries or inventory checks. Flexible Workflow Management: Supervisely has rich customization options and workflow automation features for complex logistics operations as well as multiple concurrent projects. Collaboration & QA Features: Supervisely provides strong collaborative environment, task management, real-time annotation review, feedback loops, and QA. Scalability:Supervisely offers scalable cloud-based infrastructure to support large video annotation projects. AI-assisted & Automated Annotation: Supervisely has strong AI-assistance capabilities, including object detection models, tracking automation, and active learning integration. Annotation Types: Supervisely supports bounding boxes, polygons, keypoints, polylines, segmentation masks. Video Annotation in Supervisely Kili Technology Kili Technology is an advanced annotation platform emphasizing scalability, automation, and integration capabilities. Built for enterprise deployments, Kili excels in handling complex annotation workflows and integrates seamlessly with AI model training pipelines in logistics environments. Multi-object & Multi-class Tracking: Kili supports multi-class labeling effectively and its multi-object tracking capabilities are good for logistics tasks with moderate complexity. Frame-accurate Event Tagging:Kili has precise temporal annotation capabilities for accurately labeling logistics events. Flexible Workflow Management: Kili supports highly customizable workflows and APIs for integration with model training pipelines. Collaboration & QA Features: Kili has extensive collaborative features, including detailed project and task management, annotation reviews, and annotation-quality analytics. Scalability: Kili is highly scalable and is designed specifically for enterprise-grade annotation tasks for large-scale annotation projects. AI-assisted & Automated Annotation: Kili offers robust auto-labeling, automated annotation via built-in or custom AI models which improves annotation efficiency. Annotation Types: Kili has rich support for bounding boxes, polygons, segmentation masks, and other logistics-relevant annotation formats. Video annotation in Kili Label Studio Label Studio is an open-source, highly customizable annotation tool supporting multiple data formats. It appeals especially to teams seeking flexibility and custom integration options, making it ideal for logistics scenarios with specific workflow needs. Video annotation in Label Studio Multi-object & Multi-class Tracking: Label Studio supports multi-class labeling. However, tracking across video frames requires plugins or additional customization. Frame-accurate Event Tagging: Label Studio has good temporal tagging capabilities. It is especially suitable for simpler event annotations. The highly precise frame-level annotation requires custom setups. Flexible Workflow Management: Label Studio facilitates data labeling workflows by enabling teams to efficiently manage projects, configure interfaces, and leverage machine learning to automate labeling tasks. Collaboration & QA Features: Label Studio has basic collaboration built-in, with task assignment and reviews; advanced QA features need additional configurations. Scalability: Label Studio's scalability support is enhanced by its compatibility with technologies like Kubernetes and Helm and integration with storage solutions like Azure Blob Storage (ABS). These integrations enable easy scaling of the Label Studio platform to handle large datasets and annotation tasks. AI-assisted & Automated Annotation: Label Studio utilizes AI-assisted labeling through its ML Backend integration, enabling interactive pre-annotations. Annotation Types: Label Studio supports different annotation types, including bounding boxes, polygons, polylines, and keypoints, offering good logistics annotation coverage. Labelbox Labelbox is an industry-leading annotation solution known for its powerful AI-assisted labeling, enterprise-level scalability, and collaboration. Designed to accelerate the annotation lifecycle, Labelbox suits logistics enterprises aiming for efficiency, scalability, and accuracy. Video annotation in Labelbox Multi-object & Multi-class Tracking: Labelbox provides robust multi-object and multi-class annotation, supporting effective tracking of logistics-specific scenarios like warehouse movements. Frame-accurate Event Tagging: Labelbox has precise event annotations with detailed frame-level tagging tools, ideal for logistics event recognition tasks. Flexible Workflow Management: Labelbox is highly flexible with customizable workflows, suitable for integration with complex operational data pipelines. Collaboration & QA Features: Labelbox provides excellent team collaboration, version control, annotation reviews, and detailed QA processes, aligning with logistics operation needs. Scalability: Labelbox has a strong scalability feature capable of handling extensive logistics annotation projects without performance issues. AI-assisted & Automated Annotation: Comprehensive AI-powered annotation and tracking tools significantly improving annotation speed and accuracy. Annotation Types: Offers a wide range of annotation types (bounding boxes, polygons, polylines, keypoints, segmentation masks) fully compatible with logistics use cases. Labellerr Labellerr is a user-friendly, accessible annotation platform designed to simplify the labeling process for medium-scale data projects. Its straightforward interface and moderate AI-assistance make it suitable for smaller to mid-size logistics operations. Video annotation in Labellerr Multi-object & Multi-class Tracking: Labellerr provides solid multi-class labeling support for complex scenes as well as multi-object tracking effective for simpler scenarios. Frame-accurate Event Tagging: Labellerr has good support for temporal tagging, suitable for clearly defined logistics events. It may lack advanced timeline controls for very complex scenarios. Flexible Workflow Management: Labellerr supports workflow management for mid-scale logistics applications; might need additional configuration for very complex pipelines. Collaboration & QA Features: Labellerr provides basic to moderate collaboration and QA features, suitable for small to medium-sized annotation projects. Scalability: Labellerr is scalable for medium-sized logistics operations. It may require additional infrastructure for large-scale datasets. AI-assisted & Automated Annotation: Labellerr supports AI assisted annotation to speedup data annotation tasks.. Annotation Types: Labellerr Supports essential annotation types (bounding boxes, polygons, keypoints) suitable for a range of logistics annotation needs. Video Annotation for Logistics: Key Takeaways This blog highlights how video annotation plays a crucial role in building effective AI systems for logistics operations. Here are the core insights from this blog: Video Annotation Transforms Visual Data into Insights: In logistics, large volumes of video data from cameras are collected daily. Annotating this data is essential for training machine learning (ML) models that enable automation, tracking, and operational intelligence. Logistics-Specific Use Cases Require Precision: Applications such as real-time parcel tracking, forklift navigation, inventory monitoring, security incident detection, and predictive maintenance all rely on high-quality, frame-accurate video annotations. Annotation Quality Impacts AI Performance: Accurate annotations directly influence object detection, event recognition, and route optimization, enabling AI to make precise predictions and reduce errors in logistics workflows. Choosing the right annotation tool is essential for building accurate and scalable logistics AI systems, and the tools listed offer various strengths tailored to different logistics needs.
Jun 08 2025
5 M
Best Data Annotation Tools for Generative AI 2025
This guide to data annotation tools for Generative AI breaks down how teams can improve model accuracy and align LLMs with human values. It also explains how to scale AI projects with the right platforms and workflows. Today, around 72% of companies use Gen-AI in at least one business function. This number is almost triple the share just three years ago. However, over half of artificial intelligence (AI) initiatives never reach production. Many Gen-AI pilots fail due to incomplete, biased, or poorly labeled data. AI teams need structured feedback loops like Reinforcement Learning from Human Feedback (RLHF) to train safe, high-performing models. They also require specialized data-annotation platforms like Encord, which can manage multimodal data, annotation at scale, and automated quality checks. This article explains what data annotation means and outlines the six must-have features of a modern annotation platform. We will also compare the best data annotation tools for Generative AI. What Is Data Annotation in the Context of Generative AI? Data annotation means adding human-readable labels to raw text, images, audio, video, or documents so a model can learn from them. In generative AI, the model's quality, safety, and ethics depend on how people label “what’s in the data” and “which output is better.” Unlike traditional supervised learning, which uses labels to classify one correct category. Gen-AI annotation reflects more complex human judgment. It is about encoding human preferences, safety rules, and multimodal context to teach models how to think, not just what to see. Why High-Quality Annotation Determines Gen AI Success Quality data annotation drives the success of generative AI projects. Accurate, diverse datasets ensure AI models deliver reliable, safe outputs. Models can generate hallucinations, biases, or irrelevant results without precise data labeling, undermining their effectiveness. Accurate annotation offers the following benefits: Alignment & RLHF: Human preference labels guide LLMs and multimodal AI systems toward helpfulness and safety. These labels help AI experts to fine-tune model performance, ensuring their outputs match human values in diverse use cases. They also let teams develop and ship reliable AI models faster. Bias control: High-quality labeled datasets prevent harmful or skewed outputs. Unbiased annotation processes categorize data types to reduce risks of bias and keep the labeling process fair and traceable for teams. Model generalization: Without quality-labeled training datasets, hallucination rates increase, and models may struggle with generalization. This occurs when LLMs face rare prompts and multimodal models need fine-grained object detection and pixel-level semantic segmentation. Annotation Challenges in Generative AI Generative AI projects require robust data annotation, but several challenges complicate the process. Addressing them can help build high-quality datasets for AI models. Scale & Velocity: LLMs and multimodal AI models consume terabyte-class datasets. Manual data labeling cannot keep pace, causing pipelines to stall and model updates to lag. Teams need automation and batch workflows that stream high-volume, real-time input through a single data annotation platform. Multimodal Complexity: Modern use cases mix text, images, video, audio, LiDAR, and PDFs. Each data type requires different annotation types. Managing different editors or file formats encourages version drift and slow project management. Quality Assurance: Ensuring quality data is tough when annotation errors occur. Labeled datasets can degrade without rigorous quality control, causing poor model performance. Human-in-the-loop workflows and active learning help maintain accuracy by flagging issues in real-time. Security & Compliance: Annotated medical scans, chat logs, and financial docs often contain Personally Identifiable Information (PII) and Protected Health Information (PHI). GDPR, HIPAA, and SOC 2 rules demand encrypted storage, audit trails, and on-premise deployment options. Cost Pressure: RLHF, red-teaming, and human-in-the-loop review can incur significant costs. Without AI-assisted labeling and usage-based pricing, annotation costs can quickly escalate, draining resources before AI applications reach production. Key Features to Look For in an AI Annotation Tool Given the challenges in data annotation, we must be cautious when selecting a platform. The best annotation tools streamline workflows, improve scalability, and ensure model performance in diverse AI applications. Below are some features to prioritize when choosing an annotation tool. RLHF Support: Look for platforms that support RLHF. It enables annotators to rank outputs, score safety, and generate reward signals for fine-tuning LLMs more efficiently. Multimodal Editors: Modern AI systems combine different data formats. A strong platform handles all data types, from bounding boxes and polygons in image annotation to pixel-level semantic segmentation. It also supports text annotation for natural language processing (NLP) and 3-D point-cloud labels for autonomous driving. AI-assisted Labeling & Active Learning: Look for the tool that supports AI-powered annotation to predict labels, auto-draw boxes, or suggest classes, so human annotators focus on edge cases. This automation cuts costs on large datasets while boosting scalability. Collaboration & Quality Control: High-quality data requires reviewer consensus and real-time metrics dashboards. Look for task routing, comment threads, and role-based permissions that help data scientists, domain experts, and QA stay aligned. Secure Infrastructure: Data security is non-negotiable. Platforms must meet SOC-2 and GDPR standards, providing on-premise or cloud-based options to protect sensitive AI data, especially in regulated fields like healthcare. SDK / API & Cloud Integrations: Scalable tools provide APIs and SDKs for seamless integration with model pipelines. This helps in automation, supports Python-based workflows, and streamlines data management for end-to-end model training. Tools Overview Best Data Annotation Tools for Generative AI Many annotation platforms now bundle multimodal editors, RLHF workflows, and active-learning automation so you can push large datasets through a single, secure pipeline. Below, we cover the best annotation tools that address the unique demands of data for generative AI. Encord – Multimodal Data Platform Built for RLHF Encord is a multimodal labeling tool that unifies text, image, video, audio, and native DICOM within one data annotation platform. This lets AI teams label their data all in a shared, user-friendly interface. Analyze and annotate multimodal data in one view Encord Image Annotation Encord’s image toolkit lets you draw bounding boxes, polygons, keypoints, or pixel-level semantic-segmentation masks in the same editor. It uses model-in-the-loop suggestions from Meta-AI’s SAM-2 to automate the labeling. Auto-labeling reduces the annotation time by roughly 70% on large datasets while maintaining 99% accuracy. Every label is saved, so active-learning loops in Encord Active can flag drift or low-quality labels before they get used in training data. Image annotation using Encord Encord Video Annotation Encord streams footage at native frame rates for video pipelines. It then applies smart interpolation to propagate labels forward and backward. This process means you do not need to label each frame by hand, yielding 6 times faster labeling throughput. Built-in advanced features include multi-object tracking, scene-level metadata, and automated pre-labeling to maintain high quality for gen AI training data. Meanwhile, background pre-computations allow annotators to scrub long clips without latency spikes. Video annotation using Encord Encord Text Annotation On the NLP side, Encord supports annotations such as entity, intent, sentiment, and free-form span tagging. More importantly, it adds preference-ranking templates for RLHF so teams can vote on which LLM response is safer or more helpful. Encord text annotation integrates SOTA models such as GPT4o and Gemini Pro 1.5 into annotation workflows. This integration speeds up document annotation processes, improving the accuracy of text training data for LLMs. Text annotation using Encord Encord Audio Annotation Encord’s audio module lets you slice, label, and classify waveforms for speech recognition, speaker diarization, and sound-event detection. Its AI-assisted labeling uses models like OpenAI Whisper to pre-label audio data, pauses, and speaker identities, reducing manual effort. Paired with foundation models such as Google’s AudioLM, it accelerates audio curation. This allows a faster feed of high-quality clips into generative pipelines. Audio annotation using Encord Learn how to automate data labeling Scale AI – Generative-AI Data Engine Scale AI offers a comprehensive Generative-AI Data Engine that supports end-to-end workflows for building and refining large language models (LLMs) and other generative AI systems. The platform includes tools for RLHF, synthetic data generation, and red teaming, essential for aligning models with human values and ensuring safety. Its synthetic-data module generates millions of language or vision examples on demand. This helps improve the detection of the rare class for object detection or multilingual NLP. Scale AI’s expertise in combining AI-based techniques with human-in-the-loop annotation allows for high-quality, scalable data labeling. This approach meets the demands of complex generative AI projects. Scale AI synthetic data Kili Technology – Hybrid Human-Plus-AI Labeling Kili Technology combines human expertise with AI pre-labeling to achieve a balance of speed and accuracy that suits Gen AI’s demanding annotation tasks. It supports various data types, including text, images, video, and PDFs, and provides customizable annotation tasks optimized for quality. A key feature is the use of foundation models like ChatGPT and SAM for AI-assisted pre-labeling, which accelerates the annotation process. Kili Technology also emphasizes collaboration with machine learning experts. It provides tools for quality control, ensuring that the annotated data meets the high standards required for generative AI. Its flexible on-premise deployment options cater to industries like finance and defense, where data security is critical. Model-based labeling in Kili Appen Appen is a leading provider of data annotation services, offering high-quality datasets for training generative AI models. It supports a vast, vetted workforce that delivers richly annotated data across text, image, audio, and video modalities. Appen's workforce ensures multilingual support, reducing cultural bias in NLP outputs. It also offers differential privacy options to protect personal data. Additionally, Appen provides pre-labeled datasets and custom data collection services, tailored to specific use cases in generative AI, such as sentiment analysis and content moderation. Multimodal data annotation in Appen Dataloop – RLHF Studio & Feedback Loops Dataloop provides an enterprise-grade AI development platform with robust data annotation tools for generative AI. Dataloop’s RLHF studio enables prompt engineering. This allows annotators to offer their feedback on model-generated responses to prompts. It supports various data types, including images, video, audio, text, and LiDAR, and offers drag-and-drop data pipelines for efficient data management. Dataloop integrates with multiple cloud services and offers a marketplace for models and datasets. This makes it a comprehensive solution for generative AI projects. Its Python SDK allows for programmatic control of annotation workflows, enhancing automation and scalability. Dataloop AI data annotation Amazon SageMaker Ground Truth Plus Amazon SageMaker Ground Truth Plus data labeling service supports the creation of high-quality training datasets for generative AI applications. It supports customizable templates for LLM safety reviews, dialogue ranking, and multimodal scoring. Tight identity and access management (IAM) and VPC peering ensure your data remains secure within your cloud environment. When labeled, assets automatically fill up in S3. This starts SageMaker processes for retraining models or checking for bias. The system uses active learning to reassess low-confidence labels, and metrics dashboards display accuracy and recall rates. Amazon SageMaker ground truth image annotation Which is the Best Data Annotation Tool for Generative AI? Among the platforms we covered above, Encord stands out for turning complex, multi-step Gen-AI annotation workflows into a single, secure workspace. Its support for multimodal data annotation within a single platform makes it a better choice for teams working on generative AI projects. It also eliminates the need for multiple tools and reduces workflow complexity. Encord's integration of RLHF workflows enables teams to compare and rank outputs from generative AI models and align them with ethical and practical standards. Whether it’s improving model behavior or meeting compliance needs, RLHF makes Encord a standout choice. Encord supports seamless cloud integration with major cloud storage providers such as AWS S3, Azure Blob Storage, and Google Cloud Storage. This allows teams to efficiently manage and annotate large datasets directly from their preferred cloud environments. Encord's developer-friendly API and SDK enable programmatic access to projects, datasets, and labels. This facilitates seamless integration into machine learning model pipelines and enhances automation. Encord SDK Moreover, Security is another area where Encord is a better choice. It is SOC2, HIPAA, and GDPR compliant, offering robust security and encryption standards to protect sensitive and confidential data. Learn how to improving data quality using end-to-end data pre-processing techniques in Encord Active Final Thoughts Data annotation tools are vital for building a generative AI application. They help create high-quality datasets that power models capable of producing human-like text, images, and more. These tools must manage large datasets and diverse data types to ensure AI outputs are reliable and aligned with human expectations. Below are key points to remember when selecting and using data annotation tools for generative AI projects. Best Use Cases for Data Annotation Tools: The best data annotation tools excel at preference ranking, training models with human feedback, red-teaming models with challenge inputs, and enhancing model transparency. These functions are essential for developing safe, effective, and interpretable generative AI systems. Challenges in Data Annotation: Generative AI annotation comes with difficulties such as rapidly managing large-scale datasets, processing multimodal data, maintaining consistent data quality over time, ensuring security and regulatory compliance, and controlling costs. Addressing these challenges is essential for successful AI model deployment. Encord for Generative AI: Encord features a multimodal editor, RLHF support, and secure AI-assisted workflows. Other tools such as Scale AI, Labelbox, Kili, Appen, Dataloop, and SageMaker also provide strong capabilities. The best choice depends on your data types, project scale, and workflow needs.
Jun 05 2025
5 M
Top Video Annotation Tools for Robotics in 2025
This Guide to the Best Video Annotation Tools for Robotics explains about what is video annotation, and essential features and popular video annotation tools that help turn raw video streams into meaningful ground-truth datasets fueling high-precision robotic-vision models. In the fast-growing field of robotics, processing video data and generating insights from it is crucial. A robotic system often uses live camera feeds to see and understand things around it. Therefore, a robotic system must be properly trained using the live video data so that it can understand its surroundings better. This training enables the robot to perceive and navigate the world effectively. The training that allows the robotic system to understand its environment, relies on high-quality video annotation. The video annotation involves labeling objects, people, and actions in a video that act as ground truth data for training machine learning models. The machine learning models use this data to learn about their environment. Video Annotation in Encord Therefore, video annotation is essential for training ML models that help robotic systems to understand its environment and perform actions. The video annotation provides well-labeled examples that give robots their “eyes,” enabling capabilities such as self-driving cars, surveillance drones, activity recognition systems, and more. Without properly annotated videos, even the most advanced ML models would struggle with basic perception tasks. High-quality annotations have a real impact; for instance, precise video labeling can directly improve the safety and efficiency of industrial robots by enabling more accurate object recognition. Defining Robotics in the AI World Robotics is a branch of engineering and computer science that focuses on creating, building, and using robots. Robots are machines that perform complex tasks automatically or semi-automatically. In the earlier days Robots used to perform repetitive, rule-based tasks. Robotics is now being combined with Artificial Intelligence (AI). Today, AI enabled robots are capable of perceiving, reasoning, learning, and adapting to dynamic environments. Because of the use of AI, robots are now evolving towards truly intelligent machines. Figure Robot, powered by AI, capable of performing human like task How Does AI Improve Robotics? AI plays an important role in Robotics. AI gives robots "smart" abilities with the help of Machine learning (ML) trained on annotated datasets that act as a brain to these machines. These ML models are used to enable various capabilities such as: Mobile robots in a warehouse Allow Robots to sense their surroundings: It Helps robots to detect and identify objects, environments, or people. For example, a robot arm locating a component on an assembly line. Allow Robots to understand and respond to humans: With Natural Language Processing (NLP), robots can understand humans and communicate with them. For example, voice-controlled home assistants or healthcare bots understand user queries and answer accordingly. Allow Robots to learn from experience: Reinforcement learning enables Robots to learn optimal strategies through trial and error. For example, a robotic dog learning to climb stairs. What is Video Annotation in the Context of Robotics? Video annotation is the process of labeling key elements (e.g. objects, actions, or regions of interest) in a video stream so that machine learning models can learn to interpret and understand the visual world. In the field of robotics, this process is foundational for enabling perception, decision-making, and autonomy. Why Does Video Annotation Matter in Robotics? Robots depend on visual data from cameras to "see" and "understand" their environment. However, raw video data is just a piece of pixels until it is transformed into structured data that robots can learn from. Video annotation provides that necessary structure, turning unlabelled data into rich training datasets by identifying what is in the frame, where it is, and what is happening. Without annotated data, AI models can not be trained to recognize objects or comprehend what is going on in a video stream, making video annotation a vital step in creating intelligent robotic systems. When there is no annotated data, AI models cannot be trained to recognize objects or understand things in a video stream, making video annotation an essential stage of developing intelligent robotic systems. How Video Annotation Transforms Raw Footage into Actionable Data? There are various types of annotation used in robotic applications: Object Detection (Bounding Boxes): This involves drawing rectangular boxes around significant objects (like humans, animals, or items) in each frame and assigning labels. It helps robots detect and respond appropriately. For instance, in a robotic arm on an assembly line, bounding boxes label different components (like screws, chips, or PCBs) so the robot can identify and pick the right part. Object Tracking: This technique assigns persistent IDs to objects as they move across frames, allowing robots to understand motion and behavior over time. For example, in autonomous delivery robots, object tracking helps follow the movement of pedestrians or vehicles, enabling the robot to predict motion and avoid collisions. Semantic Segmentation (Pixel-Level Labels): Here, each pixel in the frame is labeled according to its class (like road, sidewalk, pedestrian, or obstacle). This provides ultra-fine perception, allowing robots to navigate their environments with greater accuracy. Imagine training a warehouse robot to recognize and pick fragile items. Annotated video data helps it learn the visual differences between a glass bottle and a plastic bottle. The robot tracks their positions as a conveyor belt moves, and manipulates them delicately using a gripper. Without labeled video data, the robot would not understand what is there and how to handle or act. Key Features in a Video Annotation Tool for Robotics Selecting a video annotation tool with features specifically aligned with robotics applications is important to build effective AI robotic systems. Here are essential features that is must for annotation tools to meet the demanding requirements of robotic systems: Multi-Frame Object Tracking Object tracking feature enables annotators to identify an object in one frame and track it across subsequent frames. By maintaining continuity in annotations, it minimizes manual work and guarantees both consistency and accuracy when labeling moving objects. For example, an autonomous drone that monitors vehicles on a bustling highway relies on multi-frame object tracking to reliably predict vehicle paths, ensuring safe navigation. 3D Annotation Support 3D annotation is all about tagging objects with depth information and spatial coordinates (X, Y, Z). This often involves using LiDAR data or stereo camera footage, which helps create annotations that accurately reflect the real-world positions and sizes of objects. Take autonomous vehicles, for example, annotators use 3D bounding boxes around pedestrians, cars, and traffic signs to provide the vehicle with a precise understanding of distances, which is vital for safe driving in traffic. Automation & AI Assistance Automation and AI assistance in annotation tools uses machine learning algorithms to predict annotations that significantly speed up the labeling process. Human annotators then verify, correct, and refine these AI-generated annotations, enhancing workflow efficiency and ensuring high accuracy. For example, when training a robot for picking items in a warehouse, AI-assisted annotation can automatically create bounding boxes around known products. Human annotators can quickly check or modify these annotations, which greatly accelerates the training process. Collaboration Features Annotation in robotics often requires teamwork where multiple annotators are working on the same dataset. Collaboration features like shared annotation projects, commenting, version control, task assignments, and real-time updates are essential for keeping everyone on the same page and enhancing productivity. Imagine a team of annotators working together on a dataset for autonomous vehicles. They use built-in communication tools and task management features to quickly clear up any uncertainties, making sure that the labeling of complex traffic situations stays consistent. Integration Capabilities Data integration refers to how well an annotation tool can connect with existing data pipelines, machine learning workflows, cloud storage solutions, and model training frameworks. Smooth integration simplifies processes, enhances data accessibility, and accelerates the development of AI models. For example, a robotics team might link their annotation tool directly with TensorFlow or PyTorch, allowing labeled datasets to flow seamlessly into their model training pipelines. This setup enables them to iterate and deploy robotic vision models more efficiently. Scalability Scalability is all about the ability of a tool to manage larger datasets and more complex annotation needs without losing performance or usability. Scalable tools keep things running smoothly, are easy to use, and organize data effectively, even as projects expand significantly. Take, for example, an autonomous robotics startup that starts with a small pilot dataset and then quickly ramps up to millions of frames for deployment. A scalable annotation tool can handle this growth with ease, allowing the team to maintain both quality and productivity. Overview of Top Tools Top Video Annotation Tools for Robotics Selecting the right video annotation tool is important for developing a high performance robotic system that makes use of AI. Robots rely extensively on accurately annotated visual data for various tasks. The choice of annotation tool directly impacts model accuracy and real-world applicability. In this detailed comparison, we evaluate the top video annotation platforms against essential criteria including multi-frame tracking, 3D annotation, automation capabilities, collaboration, integration flexibility, and scalability. Encord Encord is an all-in-one data annotation and management platform designed for advanced annotation tasks including robotics. It handles complex, multimodal datasets and provides a comprehensive environment for teams that require high precision and sophisticated annotation methods. Video Annotation in Encord Multi-Frame Object Tracking: Encord makes it easy to track moving objects across frames in a video stream and enhances annotation efficiency when it comes to video annotation. 3D Annotation Support: With strong support for 3D annotation, Encord is essential for achieving accurate robotic perception. Automation & AI Assistance: Thanks to its advanced AI-driven automation, Encord dramatically cuts down on the manual annotation workload. Collaboration Features: Encord enables real-time collaboration, complete with user management, feedback loops, and a detailed version history. Integration Capabilities: Encord integrates smoothly into existing ML pipelines, cloud storage solutions, and third-party platforms. Scalability: Encord is built to scale effortlessly, whether you're working on small experimental projects or tackling large enterprise-level annotation tasks. CVAT (Computer Vision Annotation Tool) CVAT is a widely-used, open-source annotation tool. It is known for its flexibility and customizability. It is popular among robotics teams requiring specific workflows and direct control over their annotation processes. Tracking harvester movement across farms in CVAT Multi-Frame Object Tracking: CVAT supports an efficient multi frame object tracking feature across video sequences which is essential for consistent annotation. 3D Annotation Support: CVAT provides good support for 3D annotations (e.g., LiDAR and point clouds), though customization or plugins may be needed. Automation & AI Assistance: CVAT supports automation including auto-annotation and integration with external AI models. Collaboration Features: CVAT has team collaboration functionalities allowing team members to work on annotation projects. Integration Capabilities: CVAT is highly customizable. It can be easily integrated via APIs, supporting a broad range of workflows. Scalability: CVAT is scalable to large-scale projects, though may require additional engineering resources for extensive use. keylabs.ai Keylabs.ai is simple and easy to use and good for robotics teams that want to annotate data quickly with minimal setup. It has good performance with a balanced set of features. Video annotation in keylabs.ai Multi-Frame Object Tracking: keylabs.ai includes object tracking capabilities suitable for a variety of robotic applications. 3D Annotation Support: It also supports 3D annotation, including LiDAR and point cloud data. Automation & AI Assistance: With AI assisted annotation, keylabs.ai streamlines the process.. Collaboration Features: The platform is built for collaboration, making it ideal for small to medium-sized teams. Integration Capabilities: keylabs.ai integrates with popular ML frameworks. Scalability: It is well-suited for mid-sized annotation projects, though it may not be the best fit for very large enterprise workflows. Supervisely Supervisely is known for its full-fledged, developer-friendly environment for robotics teams that need advanced customization, many integrations and complex annotation workflows. Supervisely Video Annotation Toolbox 3.0 Multi-Frame Object Tracking: Supervisely tracks objects across frames and helps to annotate moving objects precisely. 3D Annotation Support: Supervisely is good at annotating complex 3D data types for detailed robotic tasks. Automation & AI Assistance: Supervisely has full automation and AI-driven labeling assistance. Collaboration Features: Supervisely has robust collaboration and task management. Integration Capabilities: Supervisely integrates with many ML tools, cloud storage and data platforms. Scalability: Supervisely is designed for large-scale robotic annotation projects. Kili Technology Kili Technology serves enterprise-level annotation requirements by integrating advanced annotation methods with solid project management. It is especially well-suited for teams that emphasize quality assurance and structured annotation workflows. Video Annotation in Kili Technology Multi-Frame Object Tracking: Kili provides reliable tracking features for consistent video annotation. 3D Annotation Support: Kili offers 3D annotation capabilities but with some limitations compared to top-tier platforms. Automation & AI Assistance: Kili has a strong AI-driven labeling assistance tool that speeds up annotation productivity. Collaboration Features: Kili features comprehensive collaboration and management tools that are suitable for larger teams. Integration Capabilities: Kili enables effective integration into enterprise workflows and machine learning environments. Scalability: Kili is designed to scale efficiently to extensive datasets and team sizes. basic.ai Basic.ai has simple and fast annotation tools for robotics teams that need to deploy fast and easy to use. It’s perfect for teams that need basic annotation features without setup. Video Annotation in Basic.ai Multi-Frame Object Tracking: basic.ai has good multi-frame annotation. 3D Annotation Support: basic.ai has basic 3D annotation. Automation & AI Assistance: basic.ai has AI assisted annotation automation. Collaboration Features: basic.ai has basic collaboration. Integration Capabilities: basic.ai integrates with common ML and data workflows. Scalability: basic.ai is good for small to medium annotation workloads. When choosing a video annotation tool for robotics multimodal data handling, collaboration needs, integration flexibility and scalability parameters should be considered. Encord and Supervisely are great for multimodal data, advanced tracking and enterprise features. CVAT is good for custom and open-source environments, while keylabs.ai, Kili Technology and basic.ai are good for teams of all sizes and project complexity. Encord is the best of these, it’s a full data management platform with additional features like advanced automation and integration. Key Takeaways Video annotation is crucial for training AI powered robots. It involves labeling objects, actions, and areas in video footage, which is essential for developing machine learning and computer vision models that enable machines to "see" and understand their environments. Here are the key takeaways from this blog: Video annotation helps robots learn: Labeling videos teach robots to recognize objects and understand actions, making them smarter and safer. AI enhances robot intelligence: With AI, robots can now detect, learn, and make decisions rather than just following preset rules. Robotics requires specialized annotation features: Annotation tools need to support capabilities like tracking moving objects, 3D labeling, and automation to be effective in robotics. Choosing the right tool is vital: The quality of the annotation tool directly impacts the development of a robust ML model, which in turn affects the robot’s performance in real-world environments. Encord is a top choice: Encord stands out with its strong automation, collaboration features, video annotation support, and seamless integration with AI workflows, making it an excellent option for robotics applications.
Jun 04 2025
5 M
Explore our products