Contents
Introducing Customer: Floy
Problem: Unreliable and Inflexible Tools for Radiologists
Solution: DICOM Image Specialization and Quality Control Features
Results: Higher-quality Data in Less Labeling Time
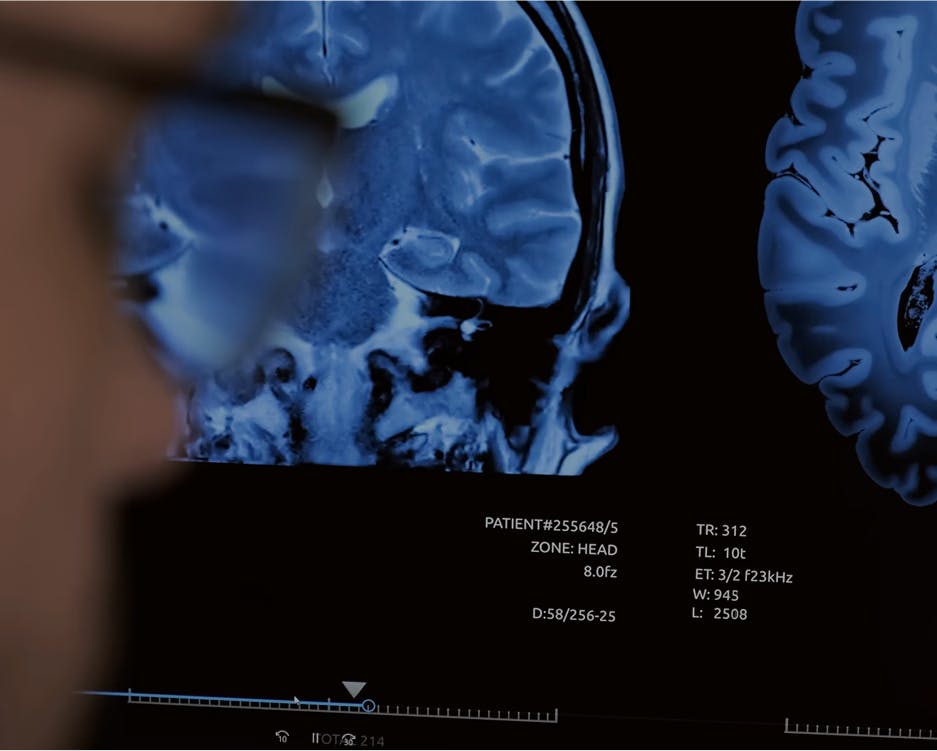
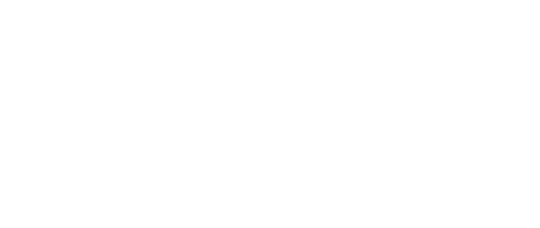
Floy Reduced CT & MRI Annotation Time with AI-Assisted Labeling
Floy develops AI that helps radiologists detect critical incidental findings in medical images. Floy partners with radiologists who use their medical expertise to annotate data used for training diagnostic models. When other annotation tools didn’t provide the flexibility or speed that radiologists need, Floy turned to Encord to improve its medical-imaging annotation process.
Introducing Customer: Floy
Floy’s technology scans radiological images to detect abnormalities, providing a second pair of eyes for radiologists. Radiologists have to interpret and make diagnoses from complex images, such as X-rays, CT scans, and MRIs. They are highly skilled medical professionals, but they’re also human, and their diagnoses are vulnerable to human error.
Radiologists can make perceptual errors, in which they overlook abnormality, and interpretive errors in which they identify an abnormality but draw the wrong conclusions. Because radiologists have a tremendous workload – sometimes hundreds of images a day – perceptual errors account for 80 percent of diagnostic radiology errors.
Floy’s AI helps solve the perceptual error problem for inpatient and outpatient radiologists. After radiologists complete reading of scans, they use Floy’s product to screen for abnormalities – such as tumors or metastasis – in spinal scans. The AI will alert the doctors to abnormal patterns that may have been overlooked by the human eye, requesting that they double check the scan before making a declarative diagnosis.
Problem: Unreliable and Inflexible Tools for Radiologists
Developing high-quality medical AI products depends on having high-quality training data. Having processes in place for obtaining consistent, high-quality annotations is of great importance.
Floy tried working with open source labeling tools for medical images, but they didn’t have an option for building efficient workflows or sharing data across a team of radiologists. Floy’s radiologists had to download images locally, annotate them using the open source tool, and re-uploaded the image segmentations back to Floy. This unscalable, cumbersome process wasted radiologists’ precious time, decreasing productivity and increasing the likelihood of errors.
The off-the-shelf annotation platforms Floy tried contained cloud-based features and allowed users to structure workflows, but they didn’t have specialized annotation features when labeling DICOM images. Radiologists couldn’t annotate seamlessly in these platforms without encountering issues, and some of these tools had bugs which caused image labels to shift location, meaning the radiologists had to redo hours of work.
Floy needed a training data tool that enabled high-quality labeling as well as efficient workflow structuring and labeling. The company also needed a tool that made key employees happy.
Image annotation is also the most time consuming and expensive part of product development for medical AI, so labeling efficiency is key for keeping costs down.
Solution: DICOM Image Specialization and Quality Control Features
Looking for a tool that enabled DICOM image annotation and improved workflows for radiologists, Floy turned to Encord’s collaborative data labeling platform.
Encord’s DICOM annotation tool can render 20,000+ pixel intensities as opposed to existing tools that manage just 256. Created specifically for medical image labeling, the technology perfectly fits Floy’s annotation needs.
Annotating DICOM images in Encord with windowing pre-set set to 'bone'
For data privacy reasons, Floy needed to work with a German cloud provider. Encord built out integrations with Open Telekom so that Floy could store patient data on local servers while still streaming it quickly into Encord’s platform for annotation.
Encord’s user-friendly platform enables radiologists to move quickly from image slice to image slice, and it creates a workflow that prioritizes images by order of importance. It also allows reviewers and annotators to collaborate so that annotators can leave notes for reviewers, and reviewers can provide annotators with modified labels and feedback, helping them to improve.
Using Encord’s quality assessment tool, Floy’s team has visibility into the entire annotation and review process. Not only does this tool help ensure that labels are of the highest possible quality, it also provides Floy’s team with insights about the annotators themselves. For instance, if one annotator produces consistently low-quality labels, Floy’s team can have them retrained. They can also see whether an annotator performs better on certain scan types and then adjust their annotation tasks according to their strengths.
Annotator management dashboard in Encord
Results: Higher-quality Data in Less Labeling Time
With Encord, Floy has built a scalable workflow process that results in high-quality annotated data that feeds back into its models.
Using Encord’s platform, Floy’s annotation team has labeled 5,100 scans, each containing between about 16 to 200 images, or a total of approximately 60,000 images. Equally important, Floy’s annotators are very happy when using the product. The time it takes for them to label images has reduced by approximately 50 percent for CT scans and 20 percent for MRI scans.
Encord’s platform also facilitates new types of collaboration beyond those between annotators and reviewers.
Floy and Encord’s teams have benefited from close collaboration. The two companies solve problems together in real time. When Floy has provided feedback about the product from radiologists or data engineers, Encord’s team implemented features and improvements based on that feedback.
Working with Encord, Floy can be certain that it’s training its models on high-quality data so that it can build great products that serve patients. As the company expands and builds technology that works with new modalities and different body parts, Encord’s platform can help them ramp up their training data processes to develop new products quickly.
Explore our products
Yes. In addition to being able to train models & run inference using our platform, you can either import model predictions via our APIs & Python SDK, integrate your model in the Encord annotation interface if it is deployed via API, or upload your own model weights.
At Encord, we take our security commitments very seriously. When working with us and using our services, you can ensure your and your customer's data is safe and secure. You always own labels, data & models, and Encord never shares any of your data with any third party. Encord is hosted securely on the Google Cloud Platform (GCP). Encord native integrations with private cloud buckets, ensuring that data never has to leave your own storage facility.
Any data passing through the Encord platform is encrypted both in-transit using TLS and at rest.
Encord is HIPAA&GDPR compliant, and maintains SOC2 Type II certification. Learn more about data security at Encord here.Yes. If you believe you’ve discovered a bug in Encord’s security, please get in touch at security@encord.com. Our security team promptly investigates all reported issues. Learn more about data security at Encord here.
Yes - we offer managed on-demand premium labeling-as-a-service designed to meet your specific business objectives and offer our expert support to help you meet your goals. Our active learning platform and suite of tools are designed to automate the annotation process and maximise the ROI of each human input. The purpose of our software is to help you label less data.
The best way to spend less on labeling is using purpose-built annotation software, automation features, and active learning techniques. Encord's platform provides several automation techniques, including model-assisted labeling & auto-segmentation. High-complexity use cases have seen 60-80% reduction in labeling costs.
Encord offers three different support plans: standard, premium, and enterprise support. Note that custom service agreements and uptime SLAs require an enterprise support plan. Learn more about our support plans here.