Contents
Customer: Meet Four Growers
Problem: Insufficient Access to Annotated Datasets Hinders Development of Automated Harvesting Models
Solution: Encord Annotate for automated labeling and a team of annotators
Results: Significant Time Reduction and Streamlined Annotation Process Achieved with Encord's Platform
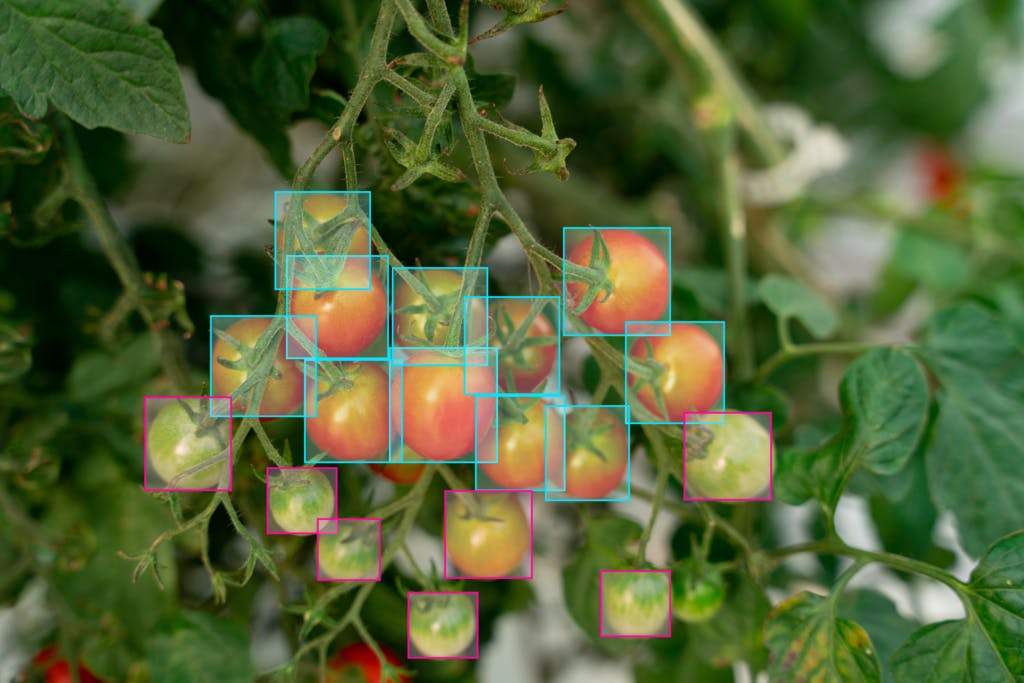
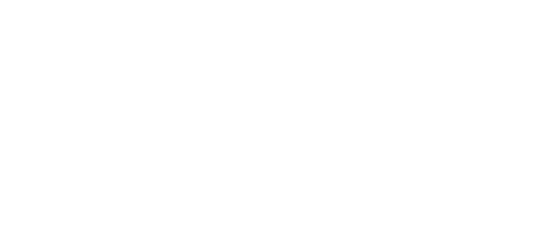
Transforming Fruit and Vegetable Harvesting & Analytics with Computer Vision
Automated harvesting and analytics company Four Growers uses Encord’s platform and annotators to help build its training datasets from scratch labeling millions of instances of greenhouses and plants. So far, Encord has halved the time it takes Four Growers to get its datasets in working order.
Customer: Meet Four Growers
Four Growers was founded to provide healthy, affordable, local produce by reducing the production costs of greenhouse growers through autonomous harvesting robots.
The GR-100 robot has a robotic arm, calibrated by four stereo cameras that allow it to precisely detect and harvest produce using computer vision, focusing on tomatoes initially. Now in its second iteration, the GR-100 also offers heat mapping and yield forecasting abilities, as well as patented packing technology.
By automating the harvesting process, Four Growers hopes to minimize food waste, increase quality, and contribute to making produce more affordable for everyone.
Problem: Insufficient Access to Annotated Datasets Hinders Development of Automated Harvesting Models
Currently, commercial greenhouses are expansive and it is impossible for manual pickers to get all of the ripe fruits on time. Over time, this leads to a large quantity of fruit just rotting in the greenhouse.
Hoping to expedite this process, Four Growers set about developing their harvesting and analytics robot. But when it came to training its deep learning models for automated harvesting, Four Growers found that while there is a lot of awareness around growing in greenhouses, there was a distinct lack of publicly available data sets to draw from.
This meant that the company had to build its own datasets from scratch, initially employing a number of freelance contractors to manually annotate all of the data, which consisted mostly of images of greenhouses and tomato plants.
This process was incredibly time-consuming and manual. By having multiple external annotators using various tools, the Four Growers team had to often deal with multiple different annotation platforms at once, consolidating the data into one succinct database at a later stage.
For Mira Murali, Computer Vision Engineer at Four Growers, this manual approach was eating into her schedule every week taking as long as 10 hours to manually review and condense data from multiple annotators that came through in various formats.
However, despite the huge amount of time, it was taking to have the data manually labeled and then verified by the team at Four Growers, it felt like a necessary task given the importance of having clean accurate data to begin with.
“You can tweak your models all you want but if your data at the beginning is wrong, then no matter how good your model is, you won’t get the end resource that you want,” Mira says.
Solution: Encord Annotate for automated labeling and a team of annotators
The team at Four Growers needed a data annotation tool that could handle the labeling of millions of object instances at the same time and this is where Encord came into the mix.
Initially, the team was attracted to Encord because not only did the platform offer automatic annotating, but it also allowed Four Growers to work with an in-house team of human annotators. So rather than having different freelance contractors using different systems that had to be later consolidated, this streamlined approach instantly gave the Four Growers team time back in their days to focus on innovating their product and growing the business.
“The fact that Encord was able to offer both an automatic labeling tool as well as a team of annotators that we could use was very attractive. We need that flexibility at this stage in our journey,” says Murali.
Encord Active is another tool beginning to be utilized by the Four Growers team. It is an open-source toolkit that can be used for testing, validating, and evaluating your models. This allows Four Growers to further segment their datasets and build on their predictive model, making the harvesting even more precise.
Results: Significant Time Reduction and Streamlined Annotation Process Achieved with Encord's Platform
For Four Growers, a fast-growing and lean business, the biggest win with using Encord has been the time saved. Now, instead of spending hours upon hours dealing with contractors and re-verifying all of that work, the team can simply upload their images to Encord’s platform, wait for a ping to say the annotation work is complete, and focus on their core jobs.
Speaking about the experience with Encord’s platform, Murali says:
“Using Encord has more than halved the time it takes us to provide feedback on imagery. What could have previously taken us 2 hours now takes approximately 30 minutes.”
Four Growers currently works with a number of large commercial greenhouses located in the US, Canada, and the Netherlands. As the company continues to grow its business and expand into more regions and crops, the company plans to continue its journey with Encord, leaning into the data analysis aspect of the platform to further understand how the team can improve its model, further streamline harvesting and help reduce food waste.
Explore our products
Yes. In addition to being able to train models & run inference using our platform, you can either import model predictions via our APIs & Python SDK, integrate your model in the Encord annotation interface if it is deployed via API, or upload your own model weights.
At Encord, we take our security commitments very seriously. When working with us and using our services, you can ensure your and your customer's data is safe and secure. You always own labels, data & models, and Encord never shares any of your data with any third party. Encord is hosted securely on the Google Cloud Platform (GCP). Encord native integrations with private cloud buckets, ensuring that data never has to leave your own storage facility.
Any data passing through the Encord platform is encrypted both in-transit using TLS and at rest.
Encord is HIPAA&GDPR compliant, and maintains SOC2 Type II certification. Learn more about data security at Encord here.Yes. If you believe you’ve discovered a bug in Encord’s security, please get in touch at security@encord.com. Our security team promptly investigates all reported issues. Learn more about data security at Encord here.
Yes - we offer managed on-demand premium labeling-as-a-service designed to meet your specific business objectives and offer our expert support to help you meet your goals. Our active learning platform and suite of tools are designed to automate the annotation process and maximise the ROI of each human input. The purpose of our software is to help you label less data.
The best way to spend less on labeling is using purpose-built annotation software, automation features, and active learning techniques. Encord's platform provides several automation techniques, including model-assisted labeling & auto-segmentation. High-complexity use cases have seen 60-80% reduction in labeling costs.
Encord offers three different support plans: standard, premium, and enterprise support. Note that custom service agreements and uptime SLAs require an enterprise support plan. Learn more about our support plans here.