Contents
ImageBind: One Embedding Space to Bind Them All
Mask DINO: Towards A Unified Transformer-based Framework for Object Detection and Segmentation
Improvising Visual Representation Learning through Perceptual Understanding
Learning Neural Parametric Head Models
Honorable mentions
Encord Blog
CVPR 2023: Most Anticipated Papers [Encord Community Edition]
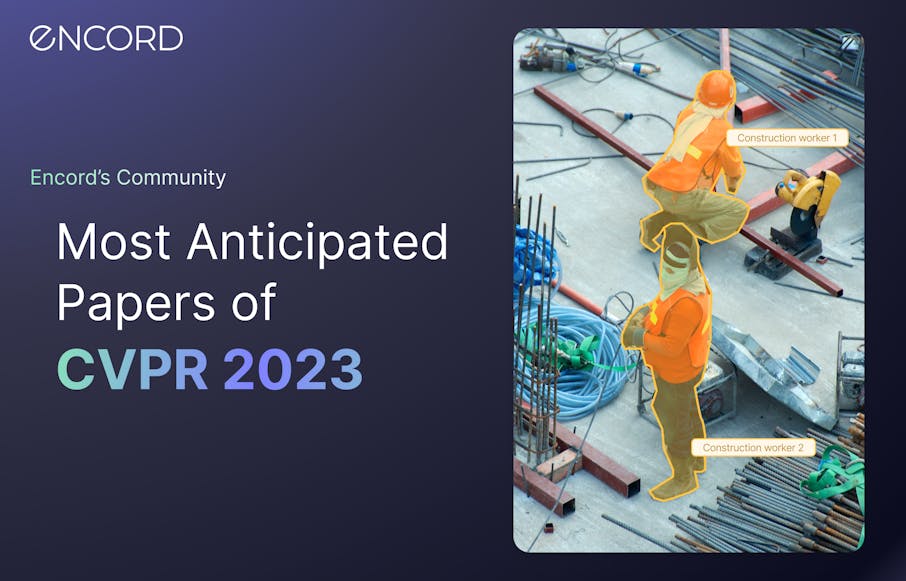
Each year, the Computer Vision and Pattern Recognition (CVPR) conference brings together some of the brightest minds in the computer vision space. And with the whirlwind the last year has been, CVPR 2023 looks on track to be one of the biggest ones yet!
So we asked our team and our readers which papers they we are most excited about going into next week's CVPR. And the votes are in!
And now onto the best part...
7 most anticipated papers from CVPR 2023 (Encord community edition):
- ImageBind: One Embedding Space to Bind Them All
- Mask DINO: Towards A Unified Transformer-based Framework for Object Detection and Segmentation
- Improving Visual Representation Learning through Perceptual Understanding
- Learning Neural Parametric Head Models
- Data-driven Feature Tracking for Event Cameras
- Humans As Light Bulbs: 3D Human Reconstruction From Thermal Reflection
- Trainable Projected Gradient Method for Robust Fine-Tuning
ImageBind: One Embedding Space to Bind Them All
Authors: Rohit Girdhar, Alaaeldin El-Nouby, Zhuang Liu, Mannat Singh, Kalyan Vasudev Alwala, Armand Joulin, Ishan Misra
One of Meta’s many open source releases of the year, ImageBind has the potential to revolutionise generative AI. Made up of a modality-specific encoder, cross-modal attention module and a joint embedding space, the model has the ability to integrate six different types of data into a single embedding space. The future will be multimodal, and ImageBind was an exciting first advancement in this direction.
ImageBind’s release opens up a host of new avenues in areas from immersive experiences, to healthcare and imaging and much more. As multimodal learning continues to advance, models like ImageBind will be crucial to the future of AI.
You can read the paper in full in Arxiv.
Further reading
ImageBind MultiJoint Embedding Model from Meta Explained
Mask DINO: Towards A Unified Transformer-based Framework for Object Detection and Segmentation
Authors: Feng Li, Hao Zhang, Huaizhe Xu, Shilong Liu, Lei Zhang, Lionel M. Ni, Heung-Yeung Shum
Through adding a mask prediction branch to DINO, Mask DINO has provided state of the art results (at time of publishing) for tasks such as instance segmentation, panoptic segmentation (both on COCO dataset) and semantic segmentation (on ADE20K).
Although the framework has limitations regarding state of the art detection performance in a large-scale setting, the evolution of DINO through this addition of a mask prediction branch makes the papers one of the most exciting at CVPR this year!
You can read the paper in full in Arxiv.
Further reading
Grounding-DINO + Segment Anything Model (SAM) vs Mask-RCNN: A comparison
Improvising Visual Representation Learning through Perceptual Understanding
Authors: Samyakh Tukra, Frederick Hoffman, Ken Chatfield
Presenting an extension to masked autoencoders (MAE), the paper seeks to improve the learned representations by explicitly promoting the learning of higher-level scene features.
Perceptual MAE not only enhances pixel reconstruction but also captures higher-level details within images through the introduction of a perceptual similarity term; incorporating techniques from adversarial training; and utilizing multi-scale training and adaptive discriminator augmentation.
Perceptual MAE improves the learning of higher-level features in masked autoencoders, boosting performance in tasks like image classification and object detection. Through a perceptual loss term and utilizing adversarial training, it enhances the representations learned by MAE and improves data efficiency. This helps bridge the gap between image and text modeling approaches and explores learning cues of the right level of abstraction directly from image data.
You can read the paper in full in Arxiv.
Learning Neural Parametric Head Models
Authors: Simon Giebenhain, Tobias Kirschstein, Markos Georgopoulos, Martin Rünz, Lourdes Agapito, Matthias Nießner
This paper introduces a novel 3D Morphable model for complete human heads that does not require subjects to wear bathcaps, unlike other models such as FaceScape.
This approach utilizes neural parametric representation to separate identity and expressions into two distinct latent spaces. It employs Signed Distance field and Neural Deformation fields to capture a person’s identity and facial expressions, respectively.
A notable aspect of the paper is the creation of a new high-resolution dataset with 255 individuals’ heads and 3.5 million meshes per scan. During inference, the model can be fitted to sparse, partial input point clouds to generate complete heads, including hair regions. Although it has limitations in capturing loose hairs, it is reported to outperform existing 3D morphable models in terms of performance and coverage of the head.
You can read the paper in full in Arxiv.
Honorable mentions
- Data-driven Feature Tracking for Event Cameras (in Arxiv)
Poster Session TUE-PM
Authors: Nico Messikommer, Carter Fang, Mathias Gehrig, Davide Scaramuzza
Event cameras, with their high temporal resolution, resilience to motion blur, and sparse output, are well-suited for low-latency, low-bandwidth feature tracking in challenging scenarios. However, existing feature tracking methods for event cameras either require extensive parameter tuning, are sensitive to noise, or lack generalization across different scenarios. To address these limitations, the team propose the first data-driven feature tracker for event cameras, which uses low-latency events to track features detected in grayscale frames. Their tracker surpasses existing approaches in relative feature age by up to 120% through a novel frame attention module that shares information across feature tracks. Real-date tracker performance also increased by up to 130% (via self-supervision approach). - Humans As Light Bulbs: 3D Human Reconstruction From Thermal Reflection (in Arxiv)
Poster Session WED-PM
Authors: Ruoshi Liu, Carl Vondrick
By leveraging the thermal reflections of a person onto objects, the team's analysis-by-synthesis framework accurately locates a person's position and reconstructs their pose, even when they are not visible to a regular camera. This is possible due to the human body emitting long-wave infrared light, which acts as a thermal reflection on surfaces in the scene. The team combine generative models and differentiable rendering of reflections, to achieve results in challenging scenarios such as curved mirrors or complete invisibility to a regular camera. - Trainable Projected Gradient Method for Robust Fine-Tuning (in Arxiv)
Poster Session TUE-PM
Authors: Junjiao Tian, Xiaoliang Dai, Chih-Yao Ma, Zecheng He, Yen-Cheng Liu, Zsolt Kira
The team introduce Trainable Projected Gradient Method (TPGM) to address the challenges in fine-tuning pre-trained models for out-of-distribution (OOD) data. Unlike previous manual heuristics, TPGM automatically learns layer-specific constraints through a bi-level constrained optimization approach, enhancing robustness and generalization. By maintaining projection radii for each layer and enforcing them through weight projections, TPGM achieves superior performance without extensive hyper-parameter search. TPGM outperforms existing fine-tuning methods in OOD performance while matching the best in-distribution (ID) performance.
There we go!
Congratulations to everyone who submitted papers this year. We look forward to all the poster sessions next week and to meeting many of you in person!
Explore our products