Contents
Google’s Gemini: What We Know So Far
GPT-Vision: What We Know So Far
Recommended Pre-release Reading
Future Advancements in Multimodal Learning
Encord Blog
What to Expect From OpenAI’s GPT-Vision vs. Google’s Gemini
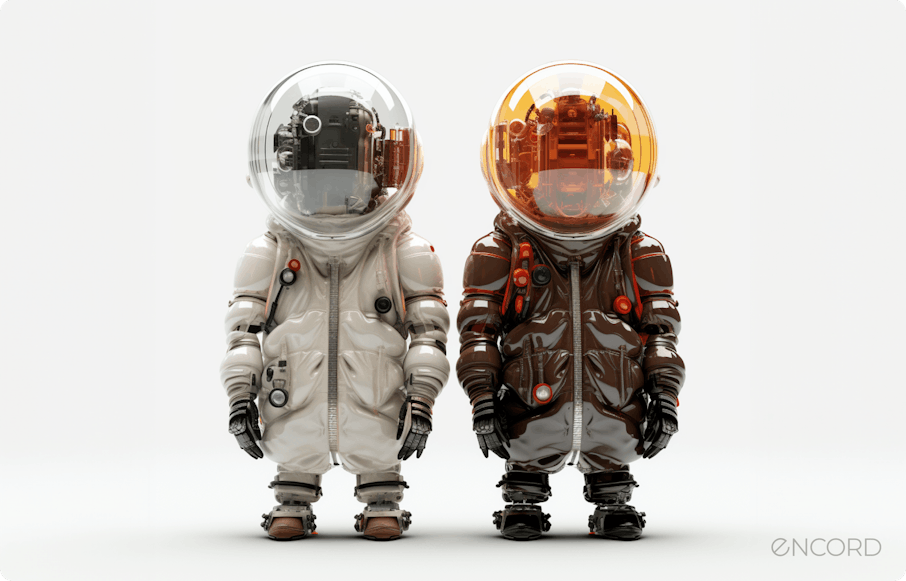
With Google gearing up to release Gemini this fall set to rival OpenAI’s GPT-Vision, it is going to be the Oppenheimer vs. Barbie of generative AI.
OpenAI and Google have been teasing their ground-breaking advancements in multimodal learning. Let's discuss what we know so far.
Google’s Gemini: What We Know So Far
At the May 2023 Google I/O developer conference, CEO Sundar Pichai unveiled Google's upcoming artificial intelligence (AI) system, codenamed Gemini. Developed by the esteemed DeepMind division, a collaboration between the Brain Team and DeepMind itself, Gemini represents a groundbreaking advancement in AI.
While detailed information remains confidential, recent interviews and reports have provided intriguing insights into the power and potential of Google's Gemini.
Gemini’s Multimodal Integration
Google CEO Sundar Pichai emphasized that Gemini combines DeepMind's AlphaGo strengths with extensive language modeling capabilities. With a multimodal design, Gemini seamlessly integrates text, images, and other data types, enabling more natural conversational abilities. Pichai also hinted at the potential for memory and planning features, which opens doors for tasks requiring advanced reasoning.
Diverse Sizes and Capabilities
Demis Hassabis, the CEO of DeepMind, provides insight into the versatility of Gemini. Drawing inspiration from AlphaGo's techniques such as reinforcement learning and tree search, Gemini is poised to acquire reasoning and problem-solving abilities. This "series of models" will be available in various sizes and capabilities, making it adaptable to a wide range of applications.
Enhancing Accuracy and Content Quality
Hassabis suggested that Gemini may employ techniques like fact-checking against sources such as Google Search and improved reinforcement learning. These measures are aimed at ensuring higher accuracy and reducing the generation of problematic or inaccurate content.
Universal Personal Assistant
In a recent interview, Sundar Pichai discussed Gemini's place in Google's product roadmap. He made it clear that conversational AI systems like Bard represent mere waypoints, not the ultimate goal. Pichai envisions Gemini and its future iterations as "incredible universal personal assistants," seamlessly integrated into people's daily lives, spanning various domains such as travel, work, and entertainment. He even suggests that today's chatbots will appear "trivial" compared to Gemini's capabilities within a few years.
GPT-Vision: What We Know So Far
OpenAI recently introduced GPT-4, a multimodal model that has the ability to process both textual and visual inputs, and in turn, generate text-based outputs. GPT-4, which was unveiled in March, was initially made available to the public through a subscription-based API with limited usage. It is speculated that the full potential of GPT-4 will be revealed in the autumn as GPT-Vision, coinciding with the launch of Google’s Gemini.
According to the paper published by OpenAI, the following is the current information available on GPT-Vision:
Transformer-Based Architecture
At its core, GPT-Vision utilizes a Transformer-based architecture that is pre-trained to predict the next token in a document, similar to its predecessors. Post-training alignment processes have further improved the model's performance, particularly in terms of factuality and adherence to desired behavior.
Human-Level Performance
GPT-4's capabilities are exemplified by its human-level performance on a range of professional and academic assessments. For instance, it achieves remarkable success in a simulated bar exam, with scores that rank among the top 10% of test takers. This accomplishment marks a significant improvement over its predecessor, GPT-3.5, which scored in the bottom 10% on the same test. GPT-Vision is expected to show similar performance if not better.
Reliable Scaling and Infrastructure
A crucial aspect of GPT-4's development involved establishing robust infrastructure and optimization methods that behave predictably across a wide range of scales. This predictability allowed us to accurately anticipate certain aspects of GPT-Vision's performance, even based on models trained with a mere fraction of the computational resources.
Test-Time Techniques
GPT-4 effectively leverages well-established test-time techniques developed for language models, such as few-shot prompting and chain-of-thought. These techniques enhance its adaptability and performance when handling both images and text.
Recommended Pre-release Reading
Multimodal Learning
Multimodal learning is a fascinating field within artificial intelligence that focuses on training models to understand and generate content across multiple modalities. These modalities encompass text, images, audio, and more. The main goal of multimodal learning is to empower AI systems to comprehend and generate information from various sensory inputs simultaneously. Multimodal learning demonstrates tremendous potential across numerous domains, including natural language processing, computer vision, speech recognition, and other areas where information is presented in diverse formats.
Generative AI
Generative AI refers to the development of algorithms and models that have the capacity to generate new content, such as text, images, music, or even video, based on patterns and data they've learned during training. These models are not only fascinating but also incredibly powerful, as they have the ability to create content that closely resembles human-produced work. Generative AI encompasses a range of techniques, including generative adversarial networks (GANs), autoencoders, and transformer-based models. It has wide-ranging applications, from creative content generation to data augmentation and synthesis.
Transformers
Transformers are a class of neural network architectures that have significantly reshaped the field of deep learning. Introduced in the landmark paper "Attention Is All You Need" by Vaswani et al. in 2017, Transformers excel at processing sequential data. They employ self-attention mechanisms to capture relationships and dependencies between elements in a sequence, making them highly adaptable for various tasks. Transformers have revolutionized natural language processing, enabling state-of-the-art performance in tasks like machine translation and text generation. Their versatility extends to other domains, including computer vision, audio processing, and reinforcement learning, making them a cornerstone in modern AI research.
Future Advancements in Multimodal Learning
Recent Advances and Trends in Multimodal Deep Learning: A Review
Multimodal Image Description
- Enhanced language generation models for accurate and grammatically correct captions.
- Advanced attention-based image captioning mechanisms.
- Incorporation of external knowledge for context-aware image descriptions.
- Multimodal models for auto video subtitling.
Multimodal Video Description
- Advancements in video dialogue systems for human-like interactions with AI.
- Exploration of audio feature extraction to improve video description in the absence of visual cues.
- Leveraging real-world event data for more accurate video descriptions.
- Research on combining video description with machine translation for efficient subtitling.
- Focus on making video subtitling processes cost-effective.
Multimodal Visual Question Answering (VQA)
- Design of goal-oriented datasets to support real-time applications and specific use cases.
- Exploration of evaluation methods for open-ended VQA frameworks.
- Integration of context or linguistic information to enhance VQA performance.
- Adoption of context-aware image feature extraction techniques.
Multimodal Speech Synthesis
- Enhancement of data efficiency for training End-to-End (E2E) DLTTS (Deep Learning Text-to-Speech) models.
- Utilization of specific context or linguistic information to bridge the gap between text and speech synthesis.
- Implementation of parallelization techniques to improve efficiency in DLTTS models.
- Integration of unpaired text and speech recordings for data-efficient training.
- Exploration of new feature learning techniques to address the "curse of dimensionality" in DLTTS.
- Research on the application of speech synthesis for voice conversion, translation, and cross-lingual speech conversion.
Multimodal Emotion Recognition
- Development of advanced modeling and recognition techniques for non-invasive emotion analysis.
- Expansion of multimodal emotion recognition datasets for better representation.
- Investigation into the preprocessing of complex physiological signals for emotion detection.
- Research on the application of automated emotion recognition in real-world scenarios.
Multimodal Event Detection
- Advancements in feature learning techniques to address the "curse of dimensionality" issue.
- Integration of textual data with audio and video media for comprehensive event detection.
- Synthesizing information from multiple social platforms using transfer learning strategies.
- Development of event detection models that consider real-time applications and user interactions.
- Designing goal-oriented datasets for event detection in specific domains and applications.
- Exploration of new evaluation methods for open-ended event detection frameworks.
From scaling to enhancing your model development with data-driven insightsLearn more
Better Data, Better AI
Enhance your AI with automated data curation, annotation, and validation.
Try it todayWritten by
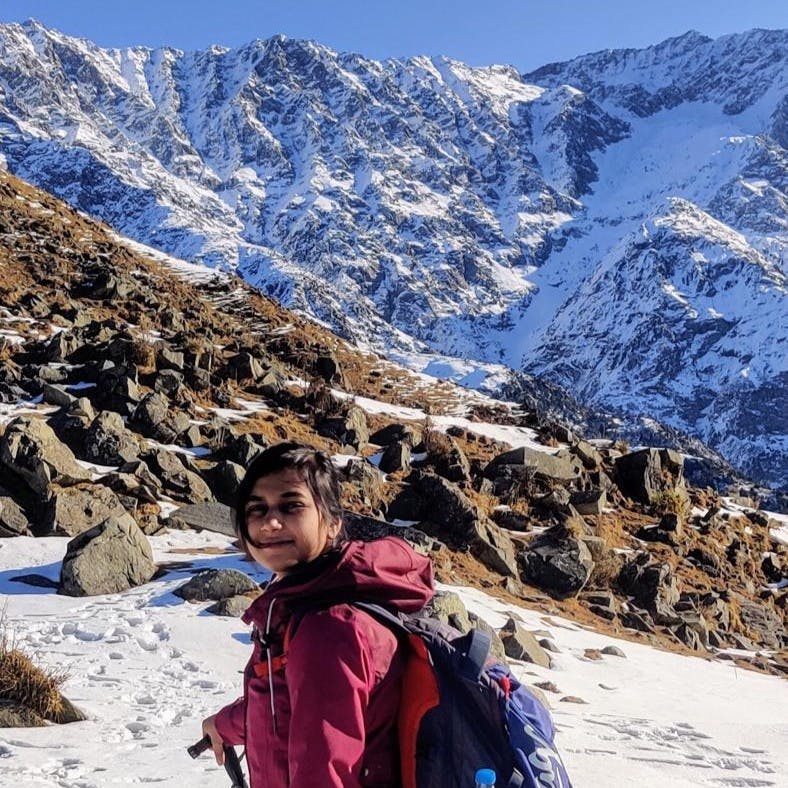
Akruti Acharya
Explore our products