Contents
What is a Medical Imaging Dataset?
How Are Medical Imaging Datasets Used in Machine Learning?
Why is it Important to Have High-Quality Medical Imaging Datasets for Machine Learning?
Key Takeaways: 7 Ways Clinical Operations Teams Can Improve Medical Imaging Datasets
Encord Blog
7 Ways to Improve Medical Imaging Dataset
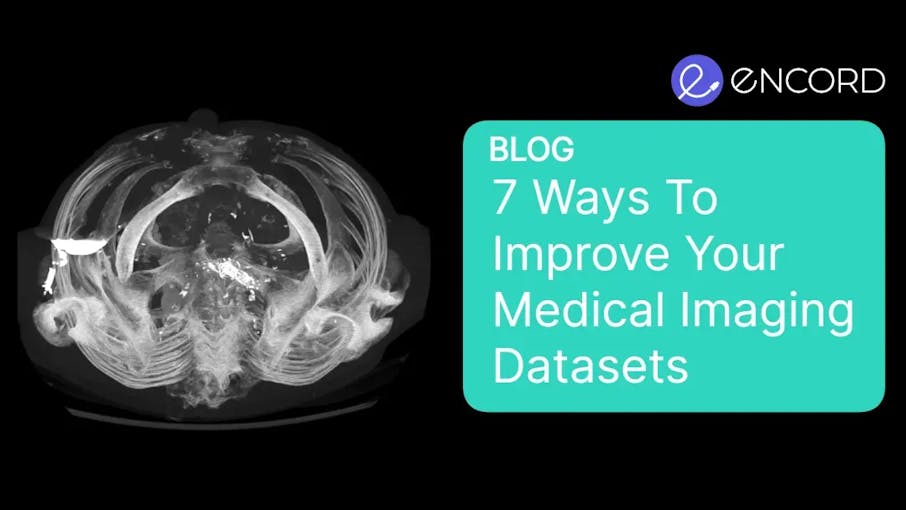
The quality of a medical imaging dataset — as is the case for imaging datasets in any sector — directly impacts the performance of a machine learning model.
In the healthcare sector, this is even more important, where the quality of large-scale medical imaging datasets for diagnostic and medical AI (artificial intelligence) or deep learning models, could be a matter of life and death for patients.
As clinical operations teams know, the complexity, formats, and layers of information are greater and more involved than non-medical images and videos. Hence the need for artificial intelligence, machine learning (ML), and deep learning algorithms to understand, interpret, and learn from annotated medical imaging datasets.
In this article, we will outline the challenges of creating training datasets from medical images and videos (especially radiology modalities), and share best practice advice for creating the highest-quality training datasets.
What is a Medical Imaging Dataset?
A medical imaging dataset can include a wide range of medical images or videos. Medical images and videos come from numerous sources, including microscopy, radiology, CT scans, MRI (magnetic resonance imaging), ultrasound images, X-rays (e.g. chest X-rays), and several others.
Medical images also come in several different formats, such as DICOM, NIfTI, and PACS. For more information on medical imaging dataset file formats:
Best Practice for Annotating DICOM and NIfTI Files
What's the difference between DICOM and NIfTI Files?
Medical image analysis is a complex field. It involves taking training data and applying ML, artificial intelligence, or deep learning algorithms to understand the content and context of images, videos, and health information to spot patterns and contribute to healthcare providers’ understanding of diseases and health conditions. Images and videos from magnetic resonance imaging (MRI) machines and radiologists are some of the most common sources of medical imaging data.
It all starts with creating accurate training data from large-scale medical imaging datasets, and for that, you need a large enough sample size. ML model performance correlates directly to the quality and statistically relevant quantity of annotated images or videos an algorithm is trained on.
How Are Medical Imaging Datasets Used in Machine Learning?
A medical imaging dataset is created, annotated, labeled, and fed into machine learning (ML) models and other AI-based algorithms to help medical professionals solve problems. The end goal is to solve medical problems, using datasets and ML models to help clinical operations teams, nurses, doctors, and other medical specialists to make more accurate diagnoses of medical illnesses.
To achieve that end goal, it’s often useful to have more than one dataset to train an ML model and a large enough sample size. For example, a dataset of patients who potentially have health problems and illnesses, such as cancer, and a healthy set, without any illnesses. ML and AI-based models are more effective when they can be trained to identify diseases, illnesses, and tumors.
It’s especially useful when annotating and labeling large-scale medical imaging datasets, to have images that come with metadata as well as clinical reports. The more information you can feed into an ML model, the more accurately it can solve problems. Of course, this means that medical imaging datasets are data-intensive, and ML models are data-hungry.
Why is it Important to Have High-Quality Medical Imaging Datasets for Machine Learning?
Annotation and labeling work takes time, and there’s pressure on clinical operations teams to source the highest quality datasets possible. Quality control is integral to this process, especially when project outcome and model accuracy is so important
High-quality data should ideally come from multiple devices and platforms, covering images or videos of as many ethnic groups as possible, to reduce the risk of bias. Datasets should include images or videos of healthy and unhealthy patients.
Quality directly impacts machine learning model outcomes. So, the more accurate and widespread a range of images, and annotations applied, the more likely a model will train to a level of effectiveness and efficiency to make the project a worthwhile investment.
Annotators can create more accurate training data when they have the right tools, such as an AI-based tool that helps leading medical institutions and companies address some of the hardest challenges in computer vision for healthcare. Clinical operations teams need a platform that streamlines collaboration between annotation teams, medical professionals, and machine learning engineers, such as Encord.
What are the Consequences if a ‘Bad’ Dataset is Fed Into a Machine Learning Model?
Feeding a poor quality, poorly cleaned (cleansing the raw data is integral to this process), and inaccurately labeled and annotated dataset into a machine learning model is a waste of time.
It will negatively impact a model’s outcomes and outputs, potentially rendering the entire project worthless. Forcing clinical operations teams to either start again or re-do large parts of the project, costs more time and money, especially when handling large datasets.
The quality of a large dataset makes a huge difference. A poor-quality dataset could cause a model to fail, not to learn anything from the data because there’s insufficient viable material it can learn from.
Or if a model does train on an insufficiently diverse medical dataset it will produce a biased outcome. A model could be biased in numerous ways. It could be biased for or against men or women. Or biased for or against certain ethnic groups. A model could also inaccurately identify sick people as healthy, and healthy people as being sick. Hence the importance of a statistically large enough sample size within a dataset.
‘Bad’ data comes in many forms. It’s the role of annotation and labeling teams and providers to ensure clinical operations and ML teams have the highest quality data possible, with accurate annotations and labels, and strict quality control.
What are the Common Problems with Medical Imaging Datasets?
Common problems include imaging datasets that aren’t readable to machine learning models.
Hospitals sell large datasets for medical imaging research and ML-based projects. When this happens, images could be delivered without the diversity a model requires or stripped of vital clinical metadata, such as biopsy reports. Or hospitals will simply sell datasets in large quantities, without having the technical capability of filtering for the right images and videos.
However, an equally common problem is that medical data still includes identifiable personal patient information, such as names, insurance details, or addresses. Due to healthcare regulatory requirements and data protection laws (e.g. the FDA and European CE regulations), every image annotation project needs to be especially careful that datasets are cleansed of anything that could identify patients and breach confidentiality.
Other problems include using data from older models of medical devices, resulting in lower-resolution images and videos.
What are the Common Challenges in Creating a Medical Imaging Dataset?
Creating and starting to annotate and label a medical image dataset involves overcoming some of these common challenges:
- Where are you getting the data from? Will it come from in-house sources (e.g. a hospital or medical provider using its own imaging datasets), from public sources, or will you buy it from hospitals?
- Who is going to annotate and label the dataset; e.g. in-house or external providers? Remember, a radiologist's or other specialist’s time is far too valuable. You need a reliable provider for this work.
- Where and how will you store the medical imaging data?
- How will the raw data be extracted for annotation and labeling?
- How will the medical imaging data be transported? Usually. datasets contain hundreds of thousands of images, videos, and medical metadata. It’s not as easy as simply storing it in cloud-based servers. This isn’t something you can attach as a Zip folder and send in an email. Medical data needs high levels of encryption, and in some cases, armed guards.
- Are you getting all of the data you need to train a model? Remember that you need a wide enough range of images to avoid inaccuracies and bias.
- How will you validate this and sift through vast quantities of images, videos, and medical imaging data?
- How long can you keep the data? Regulators may limit this to three years.
- If data is being annotated and labeled in a developing country; what are their data protection laws, and can you legally do this, and how can you ensure the data is shipped and stored securely?
- How can you effectively implement quality control throughout the annotation process to ensure the model receives the highest quality and most accurate data possible?
All of these questions need to be considered and answered before starting a medical image dataset annotation project. And only once images or videos have been annotated and labeled can you start training a machine-learning model to solve the particular problems and challenges of the project.
Now here are 7 ways clinical operations teams can improve the quality and accuracy of medical imaging datasets.
Key Takeaways: 7 Ways Clinical Operations Teams Can Improve Medical Imaging Datasets
#1: Getting the right data and getting enough data
Before embarking on any computer vision project, you need to get the right data and it needs to be of a high enough quality and quantity for statistical weighting purposes. As we’ve mentioned, quality is so important, it can have a direct positive or negative impact on the outcomes of ML-based models.
Project leaders need to coordinate with machine learning, data science, and clinical teams before ordering medical imaging datasets. Doing this should help you overcome some of the challenges of getting ‘bad’ data or annotation teams having to sift through thousands of irrelevant or poor quality images and videos when annotating training data, and the associated cost/time impacts.
#2: Addressing regulatory concerns and compliance when annotating medical imaging datasets
Regulatory and compliance questions need to be addressed before buying or extracting medical image datasets, either from in-house sources, or external suppliers, such as hospitals.
Project leaders and ML teams need to ensure the medical imaging datasets comply with the relevant FDA, European CE regulations, HIPAA, or any other data protection laws.
Regulatory compliance concerns need to cover how data is stored, accessed, and transported, the time a project will take, and ensuring the images or videos are sufficiently anonymous (without any specific patient identifiers). Otherwise, you risk breaking laws that come with hefty fines, and even the risk of data breaches, especially when working with third-party annotation providers.
#3: Give annotation teams powerful AI-based tools that specialize in medical image datasets
Medical image annotation for machine learning models requires accuracy, efficiency, a high level of quality, and security.
With powerful AI-based image annotation tools, medical annotators and professionals can save hours of work and generate more accurately labeled medical images. Ensure your annotation teams have the tools they need to turn medical imaging datasets into training data that AI, ML, or deep learning models can use and learn from.
#4: Ensure medical imaging datasets are easy to transfer and use in machine-learning models
Clinical data needs to be delivered and transferred in an easily parsable format, easy to annotate, portable, and once annotated, fast and efficient to feed into an ML model. Having the right tools helps too, as annotators and ML teams can annotate images and videos in a native format, such as DICOM and NIfTI.
When searching for the ground truth of medical datasets, imaging modalities, and medical image segmentation all play a role. Giving deep learning algorithms a statistical range and quality of images alongside anonymized health information, dimensionality (in the case of DICOM images), and biomedical imaging data can produce the outcomes that ML teams and project leaders are looking for.
#5: Ensuring clinical operations and ML teams have the viewing capacity for large volumes of imaging data
Viewing capacity is a concern that project leaders need to factor in when there are large volumes of images or videos within a medical imaging dataset. Do your annotation and ML teams have enough devices to view this data on? Can you increase resources to ensure viewing capacity doesn’t cause a blockage in the project?
#6: Overcoming storage and transfer challenges
As we’ve mentioned before, storage and transfer challenges also have to be overcome. Medical imaging datasets are often many hundreds or thousands of terabytes. You can’t simply email a Zip folder to an annotation provider. Project leaders need to ensure the buying or medical raw data extraction, cleansing, storage, and transfer process is secure and efficient from end to end.
#7: Apply automation and other tools during the annotation process
When annotating thousands of medical images or videos, you need automation and other tools to support annotation teams. Make sure they’ve got the right tools, equipped to handle medical imaging datasets, so that whatever the quality and quantity they need to handle, you can be confident it will be managed efficiently and cost-effectively.
Encord has developed our medical imaging dataset annotation software in close collaboration with medical professionals and healthcare data scientists, giving you a powerful automated image annotation suite, fully auditable data, and powerful labeling protocols.
Ready to automate and improve the quality of your medical data annotations?
Sign-up for an Encord Free Trial: The Active Learning Platform for Computer Vision, used by the world’s leading computer vision teams.
AI-assisted labeling, model training & diagnostics, find & fix dataset errors and biases, all in one collaborative active learning platform, to get to production AI faster. Try Encord for Free Today.
Want to stay updated?
Follow us on Twitter and LinkedIn for more content on computer vision, training data, and active learning.
Join our Discord channel to chat and connect.
Data infrastructure for multimodal AI
Click around the platform to see the product in action.
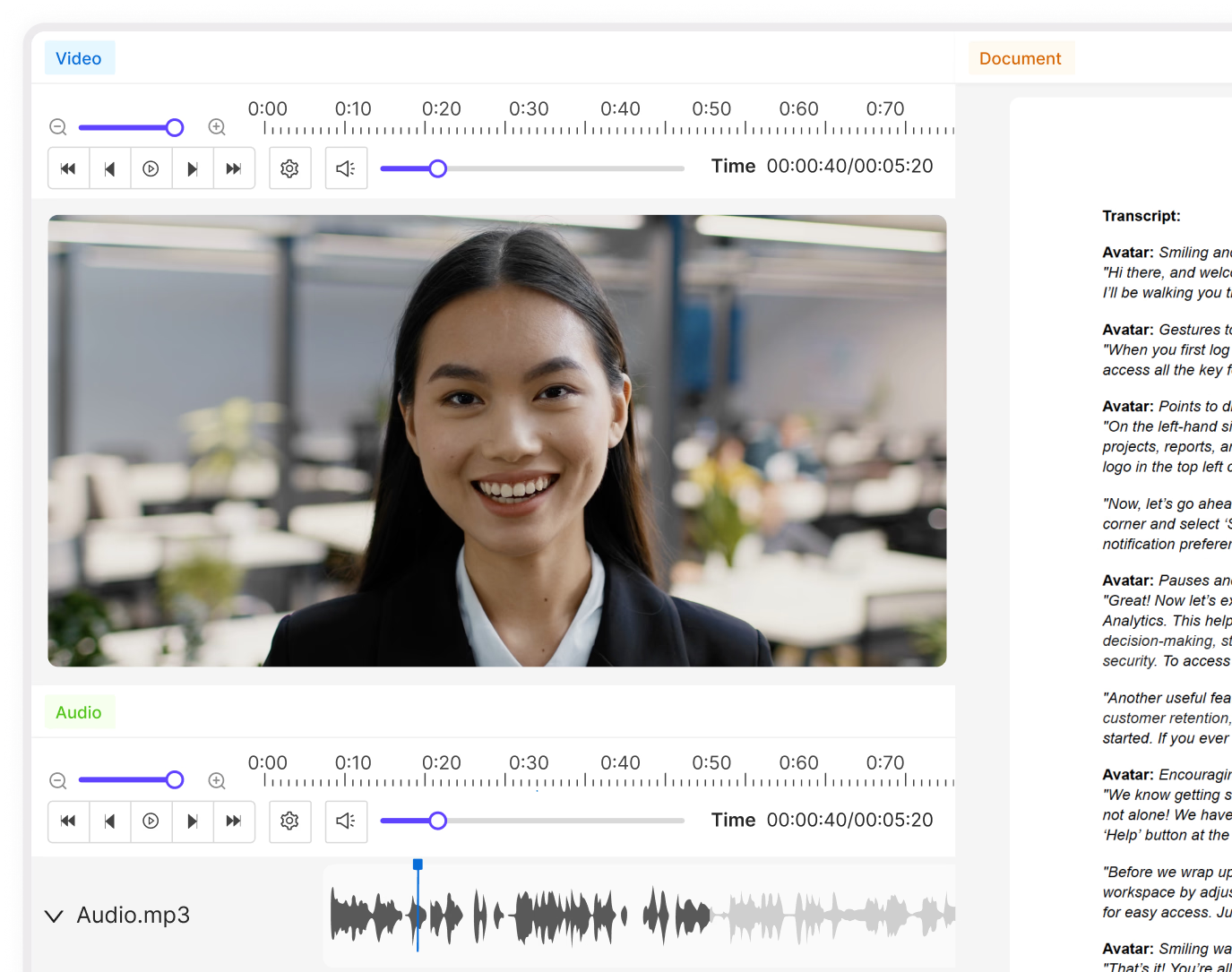
Written by
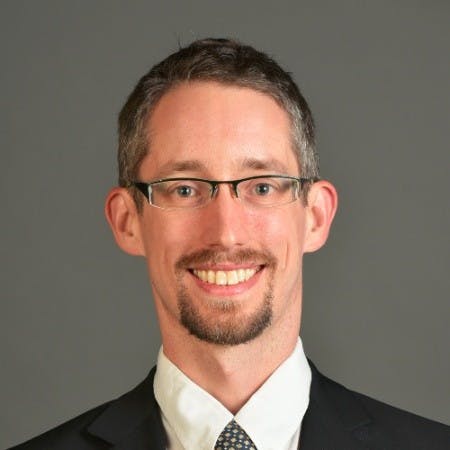
Dr. Andreas Heindl
Explore our products