Contents
What is In-House Data Labeling?
What Are The Pros and Cons of In-House Annotation?
What Are The Pros and Cons of Outsourcing Data Annotation?
What to Look For in an Outsourced Annotation Provider?
What To Be Careful of When Choosing a Data Annotation Partner?
7 Best Practice Tips For Working With an Annotation Outsourcing Company
What Tools Should You Use to Improve Annotation Team Projects (In-house or Outsource)?
Summary and Key Takeaways
Data Annotation Outsourcing Services for Computer Vision - FAQs
Encord Blog
The Full Guide to Outsourcing Data Labeling for Machine Learning
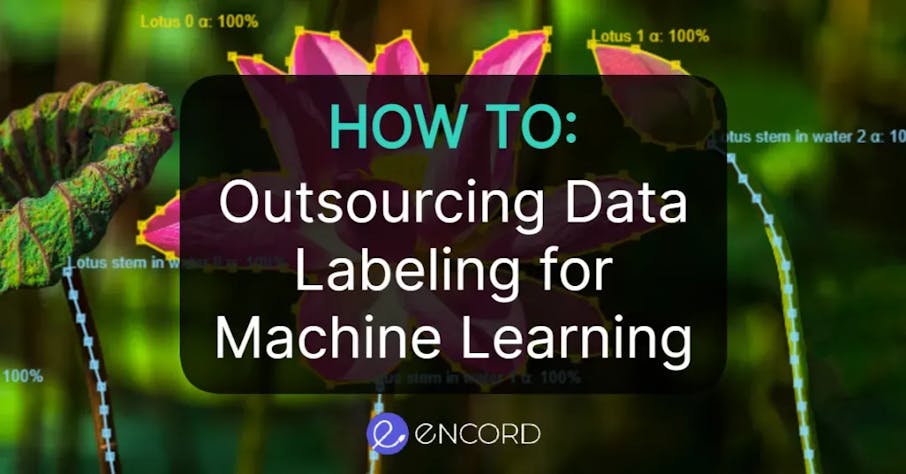
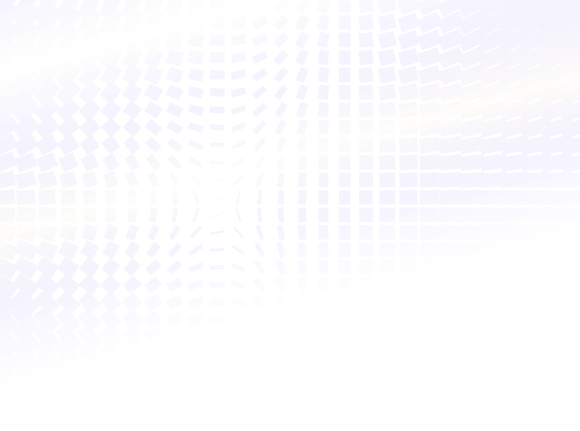
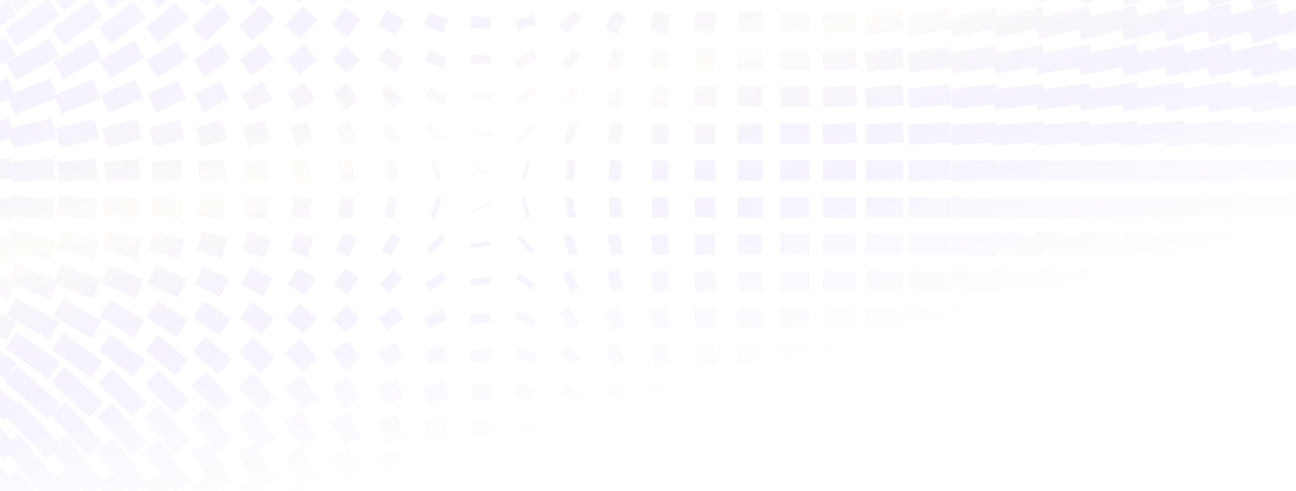
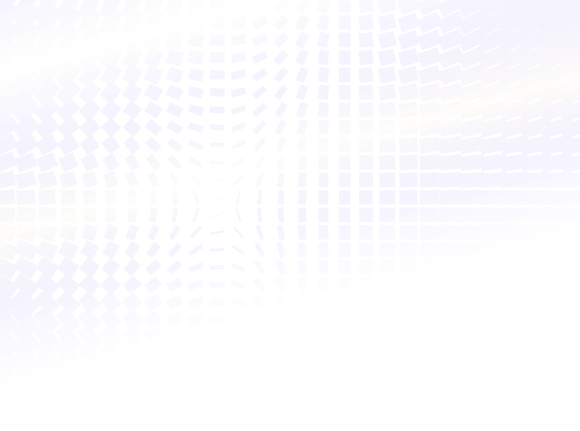
Power your AI models with the right data
Automate your data curation, annotation and label validation workflows.
Get startedContents
What is In-House Data Labeling?
What Are The Pros and Cons of In-House Annotation?
What Are The Pros and Cons of Outsourcing Data Annotation?
What to Look For in an Outsourced Annotation Provider?
What To Be Careful of When Choosing a Data Annotation Partner?
7 Best Practice Tips For Working With an Annotation Outsourcing Company
What Tools Should You Use to Improve Annotation Team Projects (In-house or Outsource)?
Summary and Key Takeaways
Data Annotation Outsourcing Services for Computer Vision - FAQs
Written by

Ulrik Stig Hansen
Annotation and labeling of raw data — images and videos — for machine learning (ML) models is the most time-consuming and laborious, albeit essential, phase of any computer vision project.
Quality outputs and the accuracy of an annotation team’s work have a direct impact on the performance of any machine learning model, regardless of whether an AI (artificial intelligence) or deep learning algorithm is applied to the imaging datasets.
Organizations across dozens of sectors — healthcare, manufacturing, sports, military, smart city planners, automation, and renewable energy — use machine learning and computer vision models to solve problems, identify patterns, and interpret trends from image and video-based datasets.
Every single computer vision project starts with annotation teams labeling and annotating the raw data; vast quantities of images and videos. Successful annotation outcomes ensure an ML model can ‘learn’ from this training data, solving the problems organizations and ML team leaders set out to solve.
Once the problem and project objectives and goals have been defined, organizations have a not-so-simple choice for the annotation phase: Do we outsource or keep imaging and video dataset annotation in-house?
In this guide, we seek to answer that question, covering the pros and cons of outsourced video and image data labeling vs. in-house labeling, 7 best practice tips, and what organizations should look for in annotation and machine learning data labeling providers.
Let’s dive in . . .
What is In-House Data Labeling?
In-house data labeling and annotation, as the name suggests, involves recruiting and managing an internal team of dataset annotators and big data specialists. Depending on your sector, this team could be image and video specialists, or professionals in other data annotation fields.
Before you decide, “Yes, this is what we need!”, it’s worth considering the pros and cons of in-house data labeling compared to outsourcing this function.
What Are The Pros and Cons of In-House Annotation?
Pros
- Providing you can source, recruit, train, and retain a team of annotators, annotation managers, and data scientists/quality control professionals, then you’ve got the human resources you need to manage ongoing annotation projects in-house.
- With an in-house team, organizations can benefit from closer monitoring, better quality control, higher levels of data security, and more control over outputs and intellectual property (IP).
- Regulatory compliance, data transfers, and storage are also easier to manage with an in-house data labeling team. Everything stays internal, there’s no need to worry about data getting lost in transit; although, there’s still the risk of data breaches to worry about.
Cons
- On the other hand, recruiting an in-house team can prove prohibitively expensive. Especially if you want the advantage of having that team close, or on-site, alongside ML, data science, and other cross-functional and inter-connected teams.
- Running an in-house data labeling service is a volume-based operation. Project leaders should ask themselves, how much data will the team need to annotate? How long should this project last? After it’s finished, do we need a team of annotators to help us solve another problem, or should we recruit on short-term contracts?
- Companies making these calculations also need to assess whether extra office space is needed. Not only that but whether you will need to build or buy in specialist software and tools for annotation and data labeling projects? All of this increases the startup costs of putting together an annotation team.
- Image and video data annotation isn’t something you can dump on data science or engineering departments. They might have the right skills and tools. But, this is a project that requires a dedicated team. Especially when you factor in quality control, compliance considerations, and ongoing requests for new data to support the active learning process.
Even for experienced project leaders, this isn’t an easy call to make. In many cases, 6 or 7-figure budgets are allocated for machine learning and computer vision projects. Outcomes and outputs depend on the quality and accurate labeling of image and video annotation training datasets, and these can have a huge impact on a company, its customers, and stakeholders.
Hence the need to consider the other option: Should we consider outsourcing data annotation projects to a dedicated, experienced, proven data labeling service provider?
What is Outsourced Data Labeling?
Instead of recruiting an in-house team, many organizations generate a more effective return on investment (ROI) by partnering with third-party, professional, data annotation service providers.
Taking this approach isn’t without risk, of course. Outsourcing never is, regardless of what services the company outsources, and no matter how successful, award-winning, or large a vendor is. There’s always a danger something will go wrong. Not everything will turn out as you hoped.
However, in many cases, organizations in need of video and image annotation and data labeling services find the upsides outweigh the risks and costs of doing this in-house. Let’s take a closer look at the pros and cons of outsourced annotation and labeling.
What Are The Pros and Cons of Outsourcing Data Annotation?
Pros
- Reduced costs. Outsourcing doesn’t involve any of the financial and legal obligations of hiring and retaining (and providing benefits for) an in-house team of annotators. Every cost is absorbed by your data labeling and annotation service provider. Including office space and annotation software, tools, and technology. Also, many outsourced providers are based in lower-cost regions and countries, generating massive savings compared to recruiting a whole team in the US or Western Europe.
- An on-demand partnership. Once a project is finished, you don’t need to worry about retaining a team when there’s nothing for them to do. An upside of this is, if there is more image and video annotation work in the pipeline, you can maintain a long-term relationship with a provider of your choosing, and return to them when you need them again.
- Upscale and downscale annotation capacity as required. If there’s a seasonal nature to your annotation project demands, then working with an outsourced provider can ensure you’ve got the resources when you need them.
- Quality control and benchmarking. Trusted and reliable outsourcing data annotation service providers know they are assessed on the quality of their work and annotation projects. External providers know they need to deliver high-quality, accurate annotations to secure long-term clients and repeat business. Professional companies should have their own quality control and benchmarking processes. Provided you’ve got in-house data science and ML experts, then you can also assess their work before training datasets are fed into machine learning models.
- Speed and efficiency. Recruiting and managing an in-house team takes time. With an outsourced partner, you can have a proof of concept (POC) project up and running quickly. An initial batch of annotated images and videos are usually delivered fairly quickly too, in comparison to the time it takes for an in-house team to get up to speed.
Cons
- Which is better, build vs. buy? There are upsides and downsides to both options. When outsourcing, you are buying annotation services, and therefore, have less control.
- Domain expertise. When you work with an external provider, they may not have the sector-specific expertise that you need. Medical and healthcare organizations need teams of annotators that have experience with medical imaging and video annotation datasets. Ideally, you need a provider who knows how to work with, annotate, and label different formats, such as DICOM or NIfTI.
- Teething problems and quality control. Working with an outsourced annotation provider involves trusting the provider to deliver, on time and within budget. Because the annotation team and process aren’t in your control, there’s always the risk of teething problems and poor-quality datasets being delivered. If that happens, project and ML leaders need to instruct the provider to re-annotate the images and videos to improve the accuracy and quality, and reduce any dataset problems, such as bias.
- Price considerations. Data annotation and labeling — for images, videos, and other datasets — is a competitive and commoditized market. Providers are often in less economically developed regions — South East Asia, Latin America, India, Africa, and Central & Eastern Europe (CEE) — ensuring that many of them offer competitive rates. However, you must remember that you get what you pay for. Cheaper doesn’t always mean better. When the quality and accuracy of dataset annotation work can have such a significant impact on the outcomes of machine learning and computer vision projects, you can’t risk valuing price over expertise, quality control, and a reliable process.
Now let’s review what you should look for in an annotation provider, and what you need to be careful of before choosing who to work with.
What to Look For in an Outsourced Annotation Provider?
Outsourcing data annotation is a reliable and cost-effective way to ensure training datasets are produced on time and within budget. Once an ML team has training data to work with, they can start testing a computer vision model. The quality, accuracy, and volume of annotated and labeled images and videos play a crucial role in computer vision project outcomes.
Consequently, you need a reliable, trustworthy, skilled, and outcome-focused data labeling service provider. Project leaders need to look for a partner who can deliver:
- High quality and levels of accuracy, especially when benchmarked against algorithmically-generated datasets;
- A provider with the right expertise and experience in your sector (especially when specialist skills are required, such as working with medical imaging datasets);
- An annotation partner that applies cutting-edge annotation best practices and automation tools as part of the process;
- An adaptable and responsive annotation partner. Project deadlines are often tight. Datasets might contain too many mistakes or too much bias, and need re-annotating, so you need to be confident an outsourced provider can handle this work.
- An annotation provider who can handle large dataset volumes without compromising timescales or quality.
What To Be Careful of When Choosing a Data Annotation Partner?
At the same time, ML and computer vision project leaders — those managing the outsourced relationship and budget — need to watch out for potential pitfalls.
Common pitfalls include:
- Annotators within the outsourcing provider teams who aren’t as skilled as others. Annotation is high-volume, mentally-taxing work, and providers often hire quickly to meet client dataset volume demands. When training is limited and the tools used aren’t cutting-edge, it could result in annotators who aren’t able to deliver in terms of accuracy or volume.
- Annotators can disagree with one another, either internally, or when labeled images and videos are sent to a client for review. It’s a red flag if there’s too much pushback and re-annotated datasets come back with limited quality or accuracy improvements.
- Watch out for the quality of annotated data providers delivers. Make sure you’ve got data scientists to run quality assurance (QA) and benchmarking processes before feeding data into machine learning models. Otherwise, poor-quality data is going to negatively impact the testing ability and outputs of computer vision models, and ultimately, whether or not an ML project solves the problems it’s tasked with solving.
Now let’s dive into the 7 best practice tips you need to know when working with an outsourced annotation provider.
7 Best Practice Tips For Working With an Annotation Outsourcing Company
Start Small: Commission a Proof of Concept Project (POC)
Data annotation outsourcing should always start with a small-scale proof of concept (POC) project, to test a new provider's abilities, skills, tools, and team. Ideally, POC accuracy should be in the 70-80 percentile range. Feedback loops from ML and data ops teams can improve the accuracy, and outcomes, and reduce dataset bias, over time.
Benchmarking is equally important, and we cover that and the importance of leveraging internal annotation teams shortly.
Carefully Monitor Progress
Annotation projects often operate on tight timescales, dealing with large volumes of data being processed every day. Monitoring progress is crucial to ensuring annotated datasets are delivered on time, at the right level of accuracy, and at the highest quality possible.
As a project leader, you need to carefully monitor progress against internal and external provider milestones. Otherwise, you risk data being delivered months after it was originally needed to feed into a computer vision model. Once you’ve got an initial batch of training data, it’s easier to assess a provider for accuracy.
Monitor and Benchmark Accuracy
When the first set of images or videos is fed into an ML/AI-based or computer vision model, the accuracy might be 70%. A model is learning from the datasets it receives. Improving accuracy is crucial. Computer vision models need larger annotated datasets with a higher level of accuracy to improve the project outcomes, and this starts with improving the quality of training data.
Some of the ways to do this are to monitor and benchmark accuracy against open source datasets, and imaging data your company has already used in machine learning models. Benchmarking datasets and algorithms are equally useful and effective, such as COCO, and numerous others.
Keep Mistakes & Errors to a Minimum
Mistakes and errors cost time and money. Outsourced data labeling providers need a responsive process to correct them quickly, re-annotating datasets as needed.
With the right tools, processes, and proactive data ops teams internally, you can construct customized label review workflows to ensure the highest label quality standards possible. Using an annotation tool such as Encord can help you visualize the breakdown of your labels in high granularity to accurately estimate label quality, annotation efficiency, and model performance.
The more time and effort you put into reducing errors, bias, and unnecessary mistakes, the higher level of annotation quality can be achieved when working closely with a dataset labeling provider.
Keep Control of Costs
Costs need to be monitored closely. Especially when re-annotation is required. As a project leader, you need to ensure costs are in-line with project estimates, with an acceptable margin for error. Every annotation project budget needs project overrun contingencies.
However, you don’t want this getting out of control, especially when any time and cost overruns are the faults of an external annotation provider. Agree on all of this before signing any contract, and ask to see key performance indicator (KPI) benchmarks and service level agreements (SLAs).
Measure performance against agreed timescales, quantity assurance (QA) controls, KPIs, and SLAs to avoid annotation project cost overruns.
Leverage In-house Annotation Skills to Assess Quality
Internally, the team receiving datasets from an external annotation provider needs to have the skills to assess images and video labels, and metadata for quality and accuracy. Before a project starts, set up the quality assurance workflows and processes to manage the pipeline of data coming in. Only once complete datasets have been assessed (and any errors corrected) can they be used as training data for machine learning models.
Use Performance Tracking Tools
Performance tracking tools are a vital part of the annotation process. We cover this in more detail next. With the right performance tracking tools and a dashboard, you can create label workflow tools to guarantee quality annotation outputs.
Clearly defined label structures reduce annotator ambiguity and uncertainty. You can more effectively guarantee higher-quality results when annotation teams use the right tools to automate image and video data labeling.
What Tools Should You Use to Improve Annotation Team Projects (In-house or Outsource)?
Performance Dashboards
Data operations team leaders need a real-time overview of annotation project progress and outputs. With the right tool, you can gain the insight and granularity you need to assess how an external annotation team is progressing.
Are they working fast enough? Are the outputs accurate enough? Questions that project managers need to ask continuously can be answered quickly with a performance dashboard, even when the annotators are working several time zones away.
Dashboards can show you a whole load of insights: a performance overview of every annotator on the project, annotation rejection and approval ratings, time spent, the volume of completed images/videos per day/team member, the types of annotations completed, and a lot more.
Example of the performance dashboard in Encord
Consensus Benchmarks
Annotation projects require consensus benchmarks to ensure accuracy. Applying annotations, labels, metadata, bounding boxes, classifications, keypoints, object tracking, and dozens of other annotation types to thousands of images and videos takes time. Mistakes are made. Errors happen.
Your aim is to reduce those errors, mistakes, and misclassifications as much as possible. To ensure the highest level of accuracy in datasets that are fed into computer vision models, benchmark datasets and other quality assurance tools can help you achieve this.
Annotation Training
When working with a new provider, annotation training and onboarding for tools they’re not familiar with is time well spent. It’s worth investing in annotation training as required, especially if you’re asking an annotation team to do something they’ve not done before.
For example, you might have picked a provider with excellent experience, but they’ve never done human pose estimation (HPE) before. Ensure training is provided at this stage to avoid mistakes and cost overruns later on.
Annotation Automation Features
Annotation projects take time. Thankfully, there are now dozens of ways to speed up this process. With powerful and user-friendly tools, such as Encord, annotation teams can benefit from an intuitive editor suite and automated features.
Automation drastically reduces the workloads of manual annotation teams, ensuring you see results more quickly. Instead of drawing thousands of new labels, annotators can spend time reviewing many more automated labels. For annotation providers, Encord’s annotate, review, and automate features can accelerate the time it takes to deliver viable training datasets.
Automatic image segmentation in Encord
Flexible tools, automated labeling, and configurable ontologies are useful assets for external annotation providers to have in their toolkits. Depending on your working relationship and terms, you could provide an annotation team with access to software such as Encord, to integrate annotation pipelines into quality assurance processes and training models.
Summary and Key Takeaways
Outsource or keep image and video dataset annotation in-house? This a question every data operations team leader struggles with at some point. There are pros and cons to both options.
In most cases, the cost and time efficiency savings outweigh the expense and headaches that come with recruiting and managing an in-house team of visual data annotators. Provided you find the right partner, you can establish a valuable long-term relationship. Finding the right provider is not easy.
It might take some trial and error and failed attempts along the way. The effort you put in at the selection stage will be worth the rewards when you do source a reliable, trusted, expert annotation vendor. Encord can help you with this process.
Our AI-powered tools can also help your data ops teams maintain efficient processes when working with an external provider, to ensure image and video dataset annotations and labels are of the highest quality and accuracy.
Data Annotation Outsourcing Services for Computer Vision - FAQs
How Do You Know You’ve Found a Good Outsourced Annotation Provider?
Finding a reliable, high-quality outsourced annotation provider isn’t easy. It’s a competitive and commoditized market. Providers compete for clients on price, using press coverage, awards, and case studies to prove their expertise.
It might take time to find the right provider. In most cases, especially if this is your organization’s first time working with an outsourced data annotation company, you might need to try and test several POC projects before picking one.
At the end of the day, the quality, accuracy, responsiveness, and benchmarking of datasets against the target outcomes is the only way to truly judge whether you’ve found the right partner.
How to Find an Outsourced Annotation Partner?
When looking for an outsourced annotation and dataset labeling provider apply the same principles used when outsourcing any mission-critical service.
Firstly, start with your network: ask people you trust — see who others recommend — and refer back to any providers your organization has worked with in the past.
Compare and contrast providers. Read reviews and case studies. Assess which providers have the right experience, and sector-specific expertise, and appear to be reliable and trustworthy. Price needs to come into your consideration, but don’t always go with the cheapest. You might be disappointed and find you’ve wasted time on a provider who can’t deliver.
It’s often an advantage to test several at the same time with a proof of concept (POC) dataset. Benchmark and assess the quality and accuracy of the datasets each provider annotates. In-house data annotation and machine learning teams can use the results of a POC to determine the most reliable provider you should work with for long-term and high-volume imaging dataset annotation projects.
What Are The Long-term Implications of Outsourced vs. In-house Annotation and Data Labeling?
In the long term, there are solid arguments for recruiting and managing an in-house team. You have more control and will have the talent and expertise internally to deliver annotation projects.
However, computer vision project leaders have to weigh that against an external provider being more cost and time-effective. As long as you find a reliable and trustworthy, quality-focused provider with the expertise and experience your company needs, then this is a partnership that can continue from one project to the next.
At Encord, our active learning platform for computer vision is used by a wide range of sectors - including healthcare, manufacturing, utilities, and smart cities - to annotate 1000s of images and video datasets and accelerate their computer vision model development.
Ready to automate and improve the quality of your data annotations?
Sign-up for an Encord Free Trial: The Active Learning Platform for Computer Vision, used by the world’s leading computer vision teams.
AI-assisted labeling, model training & diagnostics, find & fix dataset errors and biases, all in one collaborative active learning platform, to get to production AI faster. Try Encord for Free Today.
Want to stay updated?
Follow us on Twitter and LinkedIn for more content on computer vision, training data, and active learning.
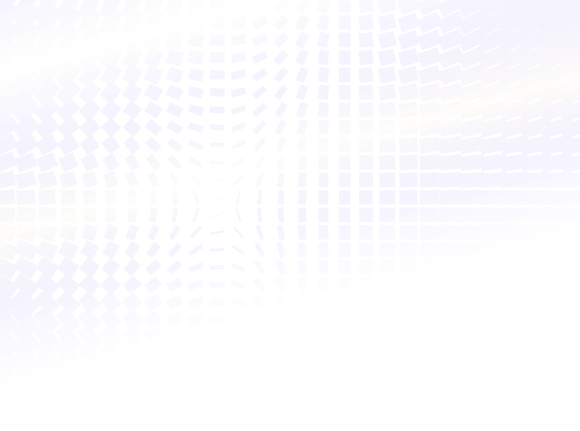
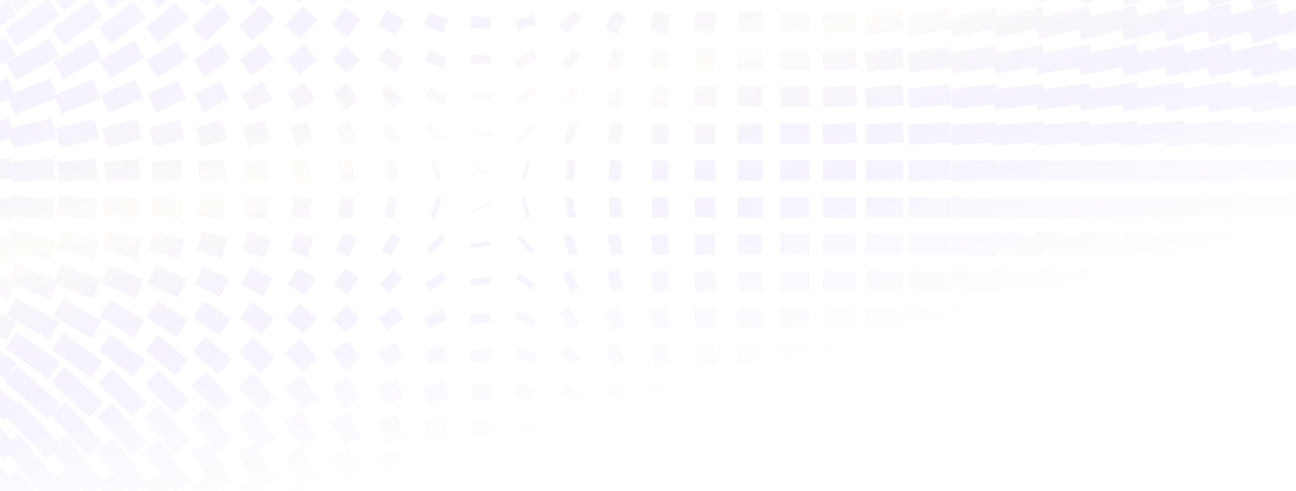
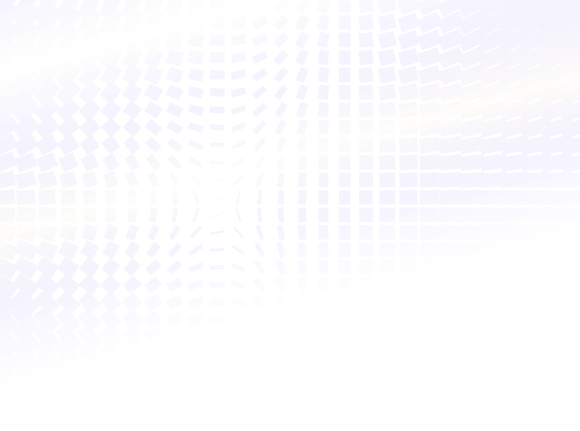
Power your AI models with the right data
Automate your data curation, annotation and label validation workflows.
Get startedWritten by

Ulrik Stig Hansen
Related blogs
The Python Developer's Toolkit for PDF Processing
PDFs (Portable Document Format) are a ubiquitous part of our digital lives, from eBooks and research papers to invoices and contracts. For developers, automating PDF processing can save time and boost productivity. 🔥Fun Fact: While PDFs may appear to contain well-structured text, they do not inherently include paragraphs, sentences, or even words. Instead, a PDF file is only aware of individual characters and their placement on the page.🔥 This characteristic makes extracting meaningful text from PDFs challenging. The characters forming a paragraph are indistinguishable from those in tables, footers, or figure descriptions. Unlike formats such as .txt files or Word documents, PDFs do not contain a continuous stream of text. A PDF document is composed of a collection of objects that collectively describe the appearance of one or more pages. These may include interactive elements and higher-level application data. The file itself contains these objects along with associated structural information, all encapsulated in a single self-contained sequence of bytes. In this comprehensive guide, we’ll explore how to process PDFs in Python using various libraries. We’ll cover tasks such as reading, extracting text and metadata, creating, merging, and splitting PDFs. Prerequisites Before diving into the code, ensure you have the following: Python installed on your system Basic understanding of Python programming Required libraries: PyPDF2, pdfminer.six, ReportLab, and PyMuPDF (fitz) You can install these libraries using pip: pip install PyPDF2 pdfminer.six reportlab PyMuPDF Reading PDFs with PyPDF2 PyPDF2 is a pure-python library used for splitting, merging, cropping, and transforming pages of PDF files. It can also add custom data, viewing options, and passwords to PDF files. Code Example Here we are reading a PDF and extracting text from it: import PyPDF2 def extract_text_from_pdf(file_path): with open(file_path, 'rb') as file: reader = PyPDF2.PdfReader(file) text = '' for page_num in range(len(reader.pages)): text += reader.pages[page_num].extract_text() return text # Usage file_path = 'sample.pdf' print(extract_text_from_pdf(file_path)) Extracting Text and Metadata with pdfminer.six pdfminer.six is a tool for extracting information from PDF documents, focusing on getting and analyzing the text data. Code Example Here’s how to extract text and metadata from a PDF: from pdfminer.high_level import extract_text from pdfminer.pdfparser import PDFParser from pdfminer.pdfdocument import PDFDocument def extract_text_with_pdfminer(file_path): return extract_text(file_path) def extract_metadata(file_path): with open(file_path, 'rb') as file: parser = PDFParser(file) doc = PDFDocument(parser) metadata = doc.info[0] return metadata # Usage file_path = 'sample.pdf' print(extract_text_with_pdfminer(file_path)) print(extract_metadata(file_path)) Creating and Modifying PDFs with ReportLab ReportLab is a robust library for creating PDFs from scratch, allowing for the addition of various elements like text, images, and graphics. Code Example To create a simple PDF: from reportlab.lib.pagesizes import letter from reportlab.pdfgen import canvas def create_pdf(file_path): c = canvas.Canvas(file_path, pagesize=letter) c.drawString(100, 750, "Hello from Encord!") c.save() # Usage create_pdf("test.pdf") To modify an existing PDF, you can use PyPDF2 in conjunction with ReportLab. Manipulating PDFs with PyPDF2 Code Example for Merging PDFs from PyPDF2 import PdfMerger def merge_pdfs(pdf_list, output_path): merger = PdfMerger() for pdf in pdf_list: merger.append(pdf) merger.write(output_path) merger.close() # Usage pdf_list = ['file1.pdf', 'file2.pdf'] merge_pdfs(pdf_list, 'merged.pdf') Code Example for Splitting PDFs from PyPDF2 import PdfReader, PdfWriter def split_pdf(input_path, start_page, end_page, output_path): reader = PdfReader(input_path) writer = PdfWriter() for page_num in range(start_page, end_page): writer.add_page(reader.pages[page_num]) with open(output_path, 'wb') as output_pdf: writer.write(output_pdf) # Usage split_pdf('merged.pdf', 0, 2, 'split_output.pdf') Code Example for Rotating Pages from PyPDF2 import PdfReader, PdfWriter def rotate_pdf(input_path, output_path, rotation_degrees=90): reader = PdfReader(input_path) writer = PdfWriter() for page_num in range(len(reader.pages)): page = reader.pages[page_num] page.rotate(rotation_degrees) writer.add_page(page) with open(output_path, 'wb') as output_pdf: writer.write(output_pdf) # Usage input_path = 'input.pdf' output_path = 'rotated_output.pdf' rotate_pdf(input_path, output_path, 90) Extracting Images from PDFs using PyMuPDF (fitz) PyMuPDF (also known as fitz) allows for advanced operations like extracting images from PDFs. Code Example Here is how to extract images from PDFs: import fitz def extract_images(file_path): pdf_document = fitz.open(file_path) for page_num in range(len(pdf_document)): page = pdf_document.load_page(page_num) images = page.get_images(full=True) for image_index, img in enumerate(images): xref = img[0] base_image = pdf_document.extract_image(xref) image_bytes = base_image["image"] image_ext = base_image["ext"] with open(f"image{page_num+1}_{image_index}.{image_ext}", "wb") as image_file: image_file.write(image_bytes) # Usage extract_images('sample.pdf') If you're extracting images from PDFs to build a dataset for your computer vision model, be sure to explore Encord—a comprehensive data development platform designed for computer vision and multimodal AI teams. Conclusion Python provides a powerful toolkit for PDF processing, enabling developers to perform a wide range of tasks from basic text extraction to complex document manipulation. Libraries like PyPDF2, pdfminer.six, and PyMuPDF offer complementary features that cover most PDF processing needs. When choosing a library, consider the specific requirements of your project. PyPDF2 is great for basic operations, pdfminer.six excels at text extraction, and PyMuPDF offers a comprehensive set of features including image extraction and table detection. As you get deeper into PDF processing with Python, explore the official documentation of these libraries for more advanced features and optimizations (I have linked them in this blog!). Remember to handle exceptions and edge cases, especially when dealing with large or complex PDF files.
Jul 17 2024
5 M
Video Data Curation Guide for Computer Vision Teams
Video data curation in computer vision shares similarities with the meticulous editing process of a film director, where each frame is carefully chosen to create a compelling narrative. Much like a director crafts a story, video data curation involves collecting, organizing, and preparing raw video data to optimize the training and performance of machine learning models. For example, well-curated dashcam footage is essential for training self-driving car models to accurately detect pedestrians, vehicles, road signs, and other objects. Conversely, models trained on poorly curated data can exhibit biases and blind spots that compromise their real-world performance. This process goes beyond ensuring data quality; it directly impacts the accuracy and efficiency of models designed for facial recognition, object detection, and automated video tagging. This article is a comprehensive guide to curating video data—selecting representative frames, accurately annotating objects, and ensuring balanced datasets—to set the stage for building quality training data for high-performance computer vision models. Importance of Video Data Curation in Computer Vision The significance of video data curation in computer vision (CV) cannot be overstated. With the exponential growth in video data fueled by advancements in digital technology and the proliferation of video content platforms, effectively managing this data becomes crucial. Data curation helps improve model performance by ensuring that the data used for training ML algorithms is high-quality, well-annotated, and representative of diverse scenarios and environments. For instance, consider a self-driving car that fails to detect pedestrians in low-light conditions because its training data lacks sufficient nighttime footage. This example highlights the critical role of data curation in ensuring the robustness and reliability of computer vision applications. Curation involves various techniques, such as selecting the most relevant and informative video frames, annotating these frames with accurate labels, and organizing the data to facilitate efficient processing and analysis. It helps reduce noise in the data, such as irrelevant frames or poorly labeled information, leading to better model accuracy and robustness. Recommendation: How to Improve the Accuracy of Computer Vision Models. Furthermore, data curation optimizes data for specific computational models and applications. For instance, embeddings—numerical representations of videos that capture their semantic content—can be generated and used with clustering or nearest neighbor search to group similar videos by content. See Also: The Full Guide to Embeddings in Machine Learning. This approach not only aids in efficient data retrieval and handling but also improves the training process by grouping similar instances, thereby improving the learning phase of models. Systematic data curation solves data diversity, volume, and annotation issues, which makes it essential to CV projects. Advantages of Video Data Curation Video data curation is essential in CV, offering numerous advantages that improve the development and deployment of robust models. Here are some of the key benefits: Improved Model Performance: Carefully curated data, free from errors and inconsistencies, leads to more accurate and reliable models. Reduced Training Time: By selecting only relevant and high-quality data, the training process becomes more efficient, saving valuable time and resources. Enhanced Generalization: Curation ensures that the data represents a wide range of scenarios, environments, and edge cases, improving the model's ability to generalize to new, unseen data. Increased Reproducibility: Well-documented curation processes make it easier to reproduce and validate results, promoting transparency and trust in the research. Cost Savings: By identifying and eliminating low-quality or irrelevant data early in the process, curation helps to avoid costly mistakes and rework later on. Watch: From Data to Diamonds: Unearthing the True Value of Quality Data. Components of Video Curation Video data curation encompasses various techniques to enhance the quality, organization, and accessibility of video data. Here are some of the key components: Different techniques for video curation (Source) Analyzing Motion: Scene Cut Detection: Identifying transitions between scenes or shots in a video is crucial for summarization and indexing tasks. Methods like frame differencing (which calculates the pixel-by-pixel differences between consecutive frames), histogram analysis (assessing changes in visual content through color comparison), or ML models (analyzing patterns) can achieve this. Optical Flow: This technique analyzes the apparent motion of objects, surfaces, or edges between consecutive frames. It helps identify and track moving objects, distinguish between static and dynamic scenes, and segment content for further analysis or editing. RAFT Model, optical flow using deep learning (Source) Detecting scene cuts remains challenging due to motion blur, compression artifacts, and intricate editing techniques that seamlessly blend scenes. However, advances in algorithmic strategies and computational capabilities are gradually mitigating these issues, improving the reliability of scene-cut detection. Enriching Content Synthetic Captioning: Generating textual descriptions of video content is essential for accessibility and content retrieval. Modern models like CoCa and VideoBLIP can automatically generate captions summarizing a video's visual content. Text Overlay Detection (OCR): Optical Character Recognition (OCR) technology is used to identify and extract text that appears over videos, such as subtitles, credits, or annotations. This information can be used for indexing, searching, and content management. Recommended: The Full Guide to Video Annotation for Computer Vision. Assessing Relevance CLIP-based Scoring: The CLIP model, developed by OpenAI, can assess the relevance of video content to textual descriptions. This technique is valuable for content retrieval and recommendation systems, ensuring videos align with user queries or textual prompts. It can also greatly improve user experience on platforms relying heavily on content discovery. 🔥 NEW RELEASE: We released TTI-Eval (text-to-image evaluation), an open-source library for evaluating zero-shot classification models like CLIP and domain-specific ones like BioCLIP against your (or HF) datasets to estimate how well the model will perform. Get started with it on GitHub, and do ⭐️ the repo if it's awesome. 🔥. While powerful, CLIP-based scoring faces challenges, such as the need for substantial computational resources due to the complexity of the model, especially when processing large volumes of video data. There’s also an ongoing need to refine these models to effectively handle diverse and nuanced video content. CLIP can also explore more complex video tasks, like action classification and recognition, across different environments. This involves recognizing a broad range of video activities that are not part of the training data. This adaptability makes CLIP-based scoring a robust tool for video analytics across varied applications. Workshop: How to Build Semantic Visual Search with ChatGPT & CLIP. Video Data Curation Process Video data curation for computer vision involves several critical steps, each contributing to effectively managing, annotating, and storing video data. This process ensures that the data is not only accessible but also primed for use in developing and training machine learning models. Here's a detailed look at each step in the video data curation process. Video Data Curation Process | Encord Video Selection and Acquisition The first step in video data curation is selecting and acquiring relevant content. This involves identifying and collecting video data from various sources that align with the specific objectives of a computer vision project. For instance, Encord allows you to ingest data by integrating different Cloud platforms, using the SDK to upload data programmatically, or importing data from local storage through the UI. Here is how you can import video datasets from your local storage to Index, the data management component of Encord: Encord Index walkthrough: uploading local data Data Management Effective data management is crucial for handling large volumes of video data and facilitating team collaboration. Encord's platform provides comprehensive tools to optimize these processes, including: Dataset Versioning: Seamlessly manage changes and iterations of video datasets. Advanced Filtering: Enhance searchability and retrievability of specific data points. Tagging: Categorize and organize video data for better structure and navigation. These features ensure that large video datasets remain manageable, accessible, and conducive to data-driven decision-making and CV workflows. Encord Inde walkthrough: Add files to the dataset Data Annotation and Labeling Data annotation involves labeling and categorizing content within video frames, and preparing the data for computer vision applications. Tools like Encord Annotate support various annotation types, such as: Bounding Boxes: Defining the location and extent of objects in a frame. Polygons: Outlining the precise shape of objects. Key Points: Marking specific points of interest, such as facial landmarks. By adding this metadata to video frames, annotation makes the data interpretable for computer vision models, enhancing the accuracy of tasks like object detection and tracking. For example, annotated video data can enable an autonomous vehicle to accurately identify and locate pedestrians, vehicles, and road signs in real-time. Here’s a walkthrough of how Index natively integrates with Annotate to create a Project to annotate the dataset: Encord Index integrates natively with Encord Annotate. Encord's automated labeling features (e.g., using SAM, object tracking, and auto-segmentation tracking) speed up your annotation. And with Active (soon coming to Index), you can pre-label data with ML-assisted algorithms. This is especially valuable for tasks like image segmentation and object detection, where it can automatically infer complex shapes from simple user interactions. Data Storage Managing the large file sizes associated with high-quality video content requires robust, scalable storage solutions. Encord Index is the data lake designed to meet the extensive data preservation needs for videos. Encord generally has large-capacity storage options that accommodate current volumes and scale to meet future demands, as well as efficient retrieval when needed. See our best practices documentation for guidelines on preserving and using your data on Encord. Data Permissions and Access Control Ensuring the security of sensitive video data is paramount, necessitating strict control over who can access it. Data management platforms often include tools for setting granular user roles and permissions and encrypting data to maintain privacy. Encord provides robust user management capabilities that allow for detailed access control, helping to safeguard data against unauthorized breaches. Encord Index walkthrough: Data permissions and access control By following these key steps in video data curation, organizations can ensure that their video datasets are well-organized, securely stored, and optimally prepared for developing cutting-edge computer vision applications. A well-designed curation workflow enables the creation of accurate, robust models that can drive significant value in various industries and use cases. Factors to Consider for Effective Video Curation Effective video curation is a multifaceted process that requires careful consideration of several key factors. These factors, including descriptive metadata, long-term accessible formats, copyright and permissions, data volume, video format, and software compatibility, collectively contribute to the success and sustainability of video curation efforts. Curators can ensure that video content is well-managed, easily discoverable, and preserved for future use by addressing these factors holistically. Descriptive Metadata Descriptive metadata plays a crucial role in video curation by enhancing the searchability and discoverability of video content. It includes information that describes the video assets for identification and discovery, such as: Unique Identifiers: Alphanumeric codes that uniquely identify each video asset. Physical/Technical Attributes: Format, duration, resolution, codec, etc. Bibliographic Attributes: Title, creator, subject, keywords, description, etc. Effective metadata management, including controlled vocabularies and metadata standards, ensures consistency and interoperability across systems, which makes the video content easily retrievable and usable. Encord Index walkthrough: Descriptive metadata. Long-term Video Accessible Formats It is vital to select the right video formats for long-term accessibility: Choose video formats known for stability and longevity (e.g., MOV, WebM, MPEG-4 with H.264 codec). Consider uncompressed or losslessly compressed formats for archival purposes. Avoid proprietary formats that may become obsolete. Copyright and Permissions Navigating copyright and permissions is a significant aspect of video curation. It involves understanding video content's legal framework, including copyright laws, fair use provisions, and licensing agreements. Curators must ensure that video content is used and distributed within legal boundaries, often requiring permissions or licenses from copyright holders. For example, a curator might need a commercial use license from the copyright owner before including a video clip in a monetized online course. Data Volume The sheer volume of video data presents storage, management, and retrieval challenges. Curators must implement strategies to handle large datasets efficiently, such as using data curation tools for categorization, tagging, and indexing. Cloud storage solutions can also provide scalable and cost-effective options for managing growing video collections. Effective data volume management ensures that video content remains organized and accessible. Video Format The choice of video format affects video content's quality, compatibility, and preservation. Curators must consider factors like compression, bit rates, and codecs when selecting formats. Using formats that balance quality with file size and compatibility is crucial for effective video curation. Compatibility with the Existing Software Ecosystem Ensuring compatibility with the existing software ecosystem is essential for seamless video curation workflows. This includes compatibility with video editing tools, digital asset management (DAM) systems, and archival software. Curators must select video formats and curation tools that integrate well with the organization's existing software infrastructure to facilitate efficient curation processes. They can develop robust video curation strategies that optimize the value and longevity of their video assets when they carefully evaluate and address these key factors. Effective video curation not only ensures the preservation and accessibility of video content but also unlocks its potential for reuse and repurposing in various contexts, from research and education to creative production and cultural heritage. Conclusion Video data curation is indispensable in computer vision, ensuring that video data is well-prepared for training accurate and efficient models. Key takeaways include: 1. Significance: Curation enhances model performance by improving data quality, reducing noise, and optimizing data for specific tasks. 2. Process: Curation involves video selection, data management, annotation, labeling, storage, and access control. 3. Techniques: Various techniques, such as scene cut detection, optical flow, synthetic captioning, text overlay detection with OCR, and CLIP-based scoring for assessing relevance, play crucial roles in enriching and organizing video data. 4. Considerations: Factors like descriptive metadata, long-term accessible formats, copyright, data volume, video format, and software compatibility are essential for successful curation. Understanding and applying these principles can unlock the full potential of video data for computer vision applications. Effective curation streamlines the development of robust models and ensures the long-term preservation and accessibility of valuable video assets.
Jun 04 2024
5 M
Dataset Distillation: Algorithm, Methods and Applications
As the world becomes more connected through digital platforms and smart devices, a flood of data is straining organizational systems’ ability to comprehend and extract relevant information for sound decision-making. In 2023 alone, users generated 120 zettabytes of data, with reports projecting the volume to approach 181 by 2025. While artificial intelligence (AI) is helping organizations leverage the power of data to gain valuable insights, the ever-increasing volume and variety of data require more sophisticated AI systems that can process real-time data. However, real-time systems are now more challenging to deploy due to the constant streaming of extensive data points from multiple sources. While several solutions are emerging to deal with large data volumes, dataset distillation is a promising technique that trains a model on a few synthetic data samples for optimal performance by transferring knowledge of large datasets into a few data points. This article discusses dataset distillation, its methods, algorithms, and applications in detail to help you understand this new and exciting paradigm for model development. What is Dataset Distillation? Dataset distillation is a technique that compresses the knowledge of large-scale datasets into smaller, synthetic datasets, allowing models to be trained with less data while achieving similar performance to models trained on full datasets. This approach was proposed by Wang et al. (2020), who successfully distilled the 60,000 training images in the MNIST dataset into a smaller set of synthetic images, achieving 94% accuracy on the LeNet architecture. The idea is based on Geoffrey Hinton's knowledge distillation method, in which a sophisticated teacher model transfers knowledge to a less sophisticated student model. However, unlike knowledge distillation, which focuses on model complexity, dataset distillation involves reducing the training dataset's size while preserving key features for model training. A notable example by Wang et al. involved compressing the MNIST dataset into a distilled dataset of ten images, demonstrating that models trained on this reduced dataset achieved similar performance to those trained on the full set. This makes dataset distillation a good option for limited storage or computational resources. Dataset distillation differs from core-set or instance selection, where a subset of data samples is chosen using heuristics or active learning. While core-set selection also aims to reduce dataset size, it may lead to suboptimal outputs due to its reliance on heuristics, potentially overlooking key patterns. Dataset distillation, by contrast, creates a smaller dataset that retains critical information, offering a more efficient and reliable approach for model training. Benefits of Dataset Distillation The primary advantage of dataset distillation is its ability to encapsulate the knowledge and patterns of a large dataset into a smaller, synthetic one, which dramatically reduces the number of samples required for effective model training. This provides several key benefits: Efficient Training: Dataset distillation streamlines the training process, allowing data scientists and model developers to optimize models with fewer training samples. This reduces the computational load and accelerates the training process compared to using the full dataset. Cost-effectiveness: The reduced size of distilled data leads to lower storage costs and fewer computational resources during training. This can be especially valuable for organizations with limited resources or those needing scalable solutions. Better Security and Privacy: Since distilled datasets are synthetic, they do not contain sensitive or personally identifiable information from the original data. This significantly reduces the risk of data breaches or privacy concerns, providing a safer environment for model training. Faster experimentation: The smaller size of distilled datasets allows for rapid experimentation and model testing. Researchers can quickly iterate over different model configurations and test scenarios, speeding up the model development cycle and reducing the time to market. Want to learn more about synthetic data generation? Read our article on what synthetic data generation is and why it is useful. Dataset Distillation Methods Multiple algorithms exist to generate synthetic examples from large datasets. Below, we will discuss the four main methods used for distilling data: performance matching, parameter matching, distribution matching, and generative techniques. Performance Matching Performance matching involves optimizing a synthetic dataset so that training a model on this data will give the same performance as training it on a larger dataset. The method by Wang et al. (2020) is an example of performance matching. Parameter Matching Zhao et al. (2021) first introduced the idea of parameter matching for dataset distillation. The method involves training a single network on the original and distilled dataset. The network optimizes the distilled data by ensuring the training parameters are consistent during the training process. Distribution Matching Distribution matching creates synthetic data with statistical properties similar to those of the original dataset. This method uses metrics like Maximum Mean Discrepancy or Kullback-Leibler (KL) divergence to measure the distance between data distributions and optimize the synthetic data accordingly. By aligning distributions, this method ensures that the synthetic dataset maintains the key statistical patterns of the original data. Generative Methods Generative methods train generative adversarial networks (GANs) to generate synthetic datasets that resemble original data. The technique involves training a generator to get latent factors or embeddings that resemble those of the original dataset. Additionally, this approach benefits storage and resource efficiency, as users can generate synthetic data on demand from latent factors or embeddings. Dataset Distillation Algorithm While the above methods broadly categorize the approaches used for dataset condensation, multiple learning algorithms exist within each approach to obtain distilled data. Below, we discuss eight algorithms for distilling data and mention the categories to which they belong. 1. Meta-learning-based Method The meta-learning-based method belongs to the performance-matching category of algorithms. It involves minimizing a loss function, such as cross-entropy, over the pixels between the original and synthetic data samples. The algorithm uses a bi-level optimization technique. An inner loop uses single-step gradient descent to get a distilled dataset, and the outer loop compares the distilled samples with the original data to compute loss. It starts by initializing a random set of distilled samples and a learning ratehyperparameter. It also samples a random parameter set from a probability distribution. The parameters represent pixels compared against those of the distilled dataset to minimize loss. Algorithm After updating the parameter set using a single gradient-descent step, the algorithm compares the new parameter set with the pixels of the original dataset to compute the validation loss. The process repeats for multiple training steps and involves backpropagation to update the distilled dataset. For a linear loss function, Wang et al. (2020) show that the number of distilled data samples should at least equal the number of features for a single sample in the original dataset to obtain the most optimal results. In computer vision (CV), where features represent each image’s pixels, the research implies that the number of distilled images should equal the number of pixels for a single image. Zhou et al. (2021) also demonstrate how to improve generalization performance using a Differentiable Siamese Augmentation (DSA) technique. The method applies crop, cutout, flip, scale, rotate, and color jitter transformations to raw data before using it for synthesizing new samples. 2. Kernel Ridge Regression-Based Methods The meta-learning-based method can be inefficient as it backpropagates errors over the entire training set. It makes the technique difficult to scale since performing the outer loop optimization step requires significant GPU memory. The alternative is kernel ridge regression (KRR), which performs convex optimization using a non-linear network architecture to avoid the inner loop optimization step. The method uses the neural tangent kernel (NTK) to optimize the distilled dataset. NTK is an artificial neural network kernel that determines how the network converts input to output vectors. For a wide neural net, the NTK represents a function after convergence, representing how a neural net behaves during training. Since NTK is a limiting function for wide neural nets, the dataset distilled using NTK is more robust and approximates the original dataset more accurately. 3. Single-step Parameter Matching In single-step parameter matching—also called gradient matching—a network trains on the distilled and original datasets in a single step. The method matches the resulting gradients after the update step, allowing the distilled data to match the original samples closely. Single-step parameter matching After updating the distilled dataset after a single training step, the network re-trains on the updated distilled data to re-generate gradients. Using a suitable similarity metric, a loss function computes the distance between the distilled and original dataset gradients. Lee et al. (2022) improve the method by developing a loss function that learns class-discriminative features. They average the gradients over all classes to measure distance. A problem that often occurs with gradient matching is that a particular network’s parameters tend to overfit synthetic data due to its small size. Kim et al. (2022) propose a solution that optimizes using a network trained on the original dataset. The method trains a network on the larger original dataset and then performs gradient matching using synthetic data. Zhang et al. (2022) also use model augmentations to create a pool of models with weight perturbations. They distill data using multiple models from the pool to obtain a highly generalized synthetic dataset using only a few optimization steps. 4. Multi-step Parameter Matching Multi-step parameter matching—also called matching training trajectories (MTT)—trains a network on synthetic and original datasets for multiple steps and matches the final parameter sets. The method is better than single-step parameter matching, which ignores the errors that may accumulate further in the process where the network trains on synthetic data. By minimizing the loss between the end results, MTT ensures consistency throughout the entire training process. MTT It also includes a normalization step, which improves performance by ensuring the magnitude of the parameters across different neurons during the later training epochs does not affect the similarity computation. An improvement involves removing parameters that are difficult to match from the loss function if the similarity between the parameters of the original and distilled dataset is below a certain threshold. 5. Single-layer Distribution Matching Single-layer distribution matching optimizes a distilled dataset by ensuring the embeddings of synthetic and original datasets are close. The method uses the embeddings generated by the last linear layer before the output layer. It involves minimizing a metric measuring the distance between the embedding distributions. Single-layer Distribution Matching Using the mean vector of embeddings for each class is a straightforward method for ensuring that synthetic data retains the distributional features of the original dataset. 6. Multi-layer Distribution Matching Multi-layer distribution matching enhances the single-layer approach by extracting features from real and synthetic data from each layer in a neural network except the last. The objective is to match features in each layer for a more robust representation. In addition, the technique uses another classifier function to learn discriminative features between different classes. The objective is to maximize the probability of correctly detecting a specific class based on the actual data sample, synthetic sample, and mean class embedding. The technique combines the discriminative loss and the loss from the distance function to compute an overall loss to update the synthetic dataset. 7. GAN Inversion Zhao et al. (2022) use GAN inversion to get latent factors from the real dataset and use the latent feature to generate synthetic data samples. GANs The generator used for GAN inversion is a pre-trained network that the researchers initialize using the latent set representing real images. Next, a feature extractor network computes the relevant features using real images and synthetic samples created using the generator network. Optimization involves minimizing the distance between the features of real and synthetic images to train the generator network. 8. Synthetic Data Parameterization Parameterizing synthetic data helps users store data more efficiently without losing information in the original data. However, a problem arises when users consider storing synthetic data in its raw format. If storage capacity is limited and the synthetic data size is relatively large, preserving it in its raw format could be less efficient. Also, storing only a few synthetic data samples may result in information loss.. Synthetic Data Parameterization The solution is to convert a sufficient number of synthetic data samples into latent features using a learnable differentiable function. Once learned, the function can help users re-generate synthetic samples without storing a large synthetic dataset. Deng et al. (2022) propose Addressing Matrices that learn representative features of all classes in a dataset. A row in the matrix corresponds to the features of a particular class. Users can extract a class-specific feature from the matrix and learn a mapping function that converts the features into a synthetic sample. They can also store the matrix and the mapping function instead of the actual samples. Do you want to learn more about embeddings? Learn more about embeddings in our full guide to embeddings in machine learning. Performance Comparison of Data Distillation Methods Liu et al. (2023) report a comprehensive performance analysis of different data distillation methods against multiple benchmark datasets. The table below reports their results. Performance results DD refers to the meta-learning-based algorithm, DC is data condensation through gradient matching, DSA is differentiable Siamese augmentation, DM is distribution matching, MTT is matching training trajectory, and FRePO is Feature Regression with Pooling and falls under KRR. FRePO performs highly on MNIST and Fashion-MNIST and has state-of-the-art performance on CIFAR-10, CIFAR-100, and Tiny-ImageNET. Dataset Distillation Applications Since dataset distillation reduces data size for optimal training, the method helps with multiple computationally intensive tasks. Below, we discuss seven use cases for data distillation, including continual and federated learning, neural architecture search, privacy and robustness, recommender systems, medicine, and fashion. Continual Learning Continual learning (CL) trains machine learning models (ML models) incrementally using small batches from a data stream. Unlike traditional supervised learning, the models cannot access previous data while learning patterns from the new dataset. This leads to catastrophic forgetting, where the model forgets previously learned knowledge. Dataset distillation helps by synthesizing representative samples from previous data. These distilled samples act as a form of "memory" for the model, often used in techniques like knowledge replay or pseudo-rehearsal. They ensure that past knowledge is retained while training on new information. Federated Learning Federated learning trains models on decentralized data sources, like mobile devices. This preserves privacy, but frequent communication of model updates between devices and the central server incurs high bandwidth costs. Dataset distillation offers a solution by generating smaller synthetic datasets on each device, which represent the essence of the local data. Transmitting these distilled datasets for central model aggregation reduces communication costs while maintaining performance. Neural Architecture Search (NAS) NAS is a method to find the most optimal network from a large pool of networks. This process is computationally expensive, especially with large datasets, as it involves training many candidate architectures. Dataset distillation provides a faster solution. By training and evaluating models on distilled data, NAS can quickly identify promising architectures before a more comprehensive evaluation of the full dataset. Privacy and Robustness Training a network on distilled can help prevent data privacy breaches and make the model robust to adversarial attacks. Dong et al. (2022) show how data distillation relates to differential privacy and how synthetic data samples are irreversible, making it difficult for attackers to extract real information. Similarly, Chen et al. (2022) demonstrate that dataset distillation can help generate high-dimensional synthetic data to ensure differential privacy and low computation costs. Recommender Systems Recommender systems use massive datasets generated from user activity to offer personalized suggestions in multiple domains, such as retail, entertainment, healthcare, etc. However, the ever-increasing size of real datasets makes these systems suffer from high latency and security risks. Dataset distillation provides a cost-effective solution as the system can use a small synthetic dataset to generate accurate recommendations. Also, distillation can help quickly fine-tune large language models (LLMs) used in modern recommendation frameworks using synthetic data samples instead of the entire dataset. Medicine Anonymization is a critical requirement when processing medical datasets. Dataset distillation offers an easy solution by allowing experts to use synthetic medical images that retain the knowledge from the original dataset while ensuring data privacy. Li et al. (2022) uses performance and parameter matching to create synthetic datasets. They also apply label distillation, which involves using soft labels instead of one-hot vectors for each class. Fashion Distilled image samples often have unique, aesthetically pleasing patterns that designers can use on clothing items. Cazenavette et al. (2022) use data distillation on an image dataset to generate synthetic samples with exotic textures for use in clothing designs. Distilled image patterns Similarly, Chen et al. (2022) use dataset distillation to develop a fashion compatibility model that extracts embeddings from designer and user-generated clothing items through convolutional networks. Fashion Compatibility Model The model learns embeddings from clothing images using uses dataset distillation to obtain relevant features. They also use and employs an attention-based mechanism to measure the compatibility of designer items with user-generated fashion trends. Dataset Distillation: Key Takeaways Dataset distillation is an evolving research field with great promise for using AI in multiple industrial domains such as healthcare, retail, and entertainment. Below are a few key points to remember regarding dataset distillation. Data vs. Knowledge Distillation: Dataset distillation maps knowledge in large datasets to small synthetic datasets, while knowledge distillation trains a small student model using a more extensive teacher network. Data Distillation Methods: The primary distillation methods involve parameter matching, performance matching, distribution matching, and generative processes. Dataset Distillation Algorithms: Current algorithms include meta-based learning, kernel ridge regression, gradient matching, matching training trajectories, single and multi-layer distribution matching, and GAN inversion. Dataset Distillation Use Cases: Dataset distillation significantly improves continual and federated learning frameworks, neural architecture search, recommender systems, medical diagnosis, and fashion-related tasks.
Apr 26 2024
8 M
Data Lake Explained: A Comprehensive Guide for ML Teams
What is a Data Lake? A data lake is a centralized repository where you can store all your structured, semi-structured, and unstructured data types at any scale for processing, curation, and analytics. It supports batch and real-time streams to combine raw data from diverse sources (databases, IoT devices, mobile apps, etc.) into the repository without a predefined schema. It has been 12 years since the New York Times published an interesting article on ‘The Age of Big Data,’ in which most of the talk and tooling were centered around analytics. Fast-forward to today, and we are continuously grappling with the influx of data at the petabyte (PB) and zettabyte (ZB) scales, which is getting increasingly complex in dimensions (images, videos, point cloud data, etc.). It is clear that solutions that can help manage the size and complexity of data are needed for organizational success. This has urged data, AI, and technology teams to look towards three pivotal data management solutions: data lakes, data warehouses, and cloud services. This article focuses on understanding data lakes as a data management solution for machine learning (ML) teams. You will learn: What a data lake is and how it differs from a data warehouse. Benefits and limitations of a data lake for ML teams. The data lake architecture. Best practices for setting up a data lake. On-premise vs. cloud-based data lakes. Computer vision use cases of data lakes. TL; DR A data lake is a centralized repository for diverse, structured, and unstructured data. Key architecture components include Data Sources, Data Ingestion, Data Persistence and Storage, Data Processing Layer, Analytical Sandboxes, Data Lake Zones, and Data Consumption. Best practices for data lakes involve defining clear objectives, robust data governance, scalability, prioritizing security, encouraging a data-driven culture, and quality control. On-premises data lakes offer control and security; cloud-based data lakes provide scalability and cost efficiency. Data lakes are evolving with advanced analytics and computer vision use cases, emphasizing the need for adaptable systems and adopting forward-thinking strategies. Overview: Data Warehousing, Data Lake, and Cloud Storage Data Warehouses A data warehouse is a single location where an organization's structured data is consolidated, transformed, and stored for query and analysis. The structured data is ideal for generating reports and conducting analytics that inform business decisions. Limitations Limited agility in handling unstructured or semi-structured data. Can create data silos, hindering cross-departmental data sharing. Data Lakes A data lake stores vast amounts of raw datasets in their native format until needed, which includes structured, semi-structured, and unstructured data. This flexibility supports diverse applications, from computer vision use cases to real-time analytics. Challenges Risk of becoming a "data swamp" if not properly managed, with unclear, unclean, or redundant data. Requires robust metadata and governance practices to ensure data is findable and usable. Cloud Storage and Computing Cloud computing encompasses a broad spectrum of services beyond storage, such as processing power and advanced analytics. Cloud storage refers explicitly to storing data on the internet through a cloud computing provider that manages and operates data storage as a service. Risks Security concerns, requiring stringent data access controls and encryption. Potential for unexpected costs if usage is not monitored. Dependence on the service provider's reliability and continuity. Data lake overview with the data being ingested from different sources. Most ML teams misinterpret the role of data lakes and data warehouses, choosing an inappropriate management solution. Before delving into the rest of the article, let’s clarify how they differ. Data Lake vs. Data Warehouse Understanding the strengths and use cases of data lakes and warehouses can help your organization maximize its data assets. This can help create an efficient data infrastructure that supports various analytics, reporting, and ML needs. Let’s compare a data lake to a data warehouse based on specific features. Choosing Between Data Lake and Data Warehouse The choice between a data lake and a warehouse depends on the specific needs of the analysis. For an e-commerce organization analyzing structured sales data, a data warehouse offers the speed and efficiency required for such tasks. However, a data lake (or a combination of both solutions) might be more appropriate for applications that require advanced computer vision (CV) techniques and large visual datasets (images, videos). Benefits of a Data Lake Data lakes offer myriad benefits to organizations using complex datasets for analytical insights, ML workloads, and operational efficiency. Here's an overview of the key benefits: Single Source of Truth: When you centralize data in data lakes, you get rid of data silos, which makes data more accessible across the whole organization. So, data lakes ensure that all the data in an organization is consistent and reliable by providing a single source of truth. Schema on Read: Unlike traditional databases that define data structure at write time (schema on write), data lakes allow the structure to be imposed at read time to offer flexibility in data analysis and utilization. Scalability and Cost-Effectiveness: Data lakes' cloud-based nature facilitates scalable storage solutions and computing resources, optimizing costs by reducing data duplication. Decoupling of Storage and Compute: Data lakes let different programs access the same data without being dependent on each other. This makes the system more flexible and helps it use its resources more efficiently. Architectural Principles for Data Lake Design When designing a data lake, consider these foundational principles: Decoupled Architecture: Data ingestion, processing, curation, and consumption should be independent to improve system resilience and adaptability. Tool Selection: Choose the appropriate tools and platforms based on data characteristics, ingestion, and processing requirements, avoiding a one-size-fits-all approach. Data Temperature Awareness: Classify data as hot (frequently accessed), warm (less frequently accessed), or cold (rarely accessed but retained for compliance) to optimize storage strategies and access patterns based on usage frequency. Leverage Managed Services: Use managed or serverless services to reduce operational overhead and focus on value-added activities. Immutability and Event Journaling: Design data lakes to be immutable, preserving historical data integrity and supporting comprehensive data analysis. They should also store and version the data labels. Cost-Conscious Design: Implement strategies (balancing performance, access needs, budget constraints) to manage and optimize costs without compromising data accessibility or functionality. Data Lake Architecture A robust data lake architecture is pivotal for harnessing the power of large datasets so organizations can store, process, and analyze them efficiently. This architecture typically comprises several layers dedicated to a specific function within the data management ecosystem. Below is an overview of these key components: Data Sources Diverse Producers: Data lakes can ingest data from a myriad of sources, including, but not limited to, IoT devices, cameras, weblogs, social media, mobile apps, transactional databases (SQL, NoSQL), and external APIs. This inclusivity enables a holistic view of business operations and customer interactions. Multiple Formats: They accommodate a wide range of data formats, from structured data in CSVs and databases to unstructured data like videos, images, DICOM files, documents, and multimedia files, providing a unified repository for all organizational data. This, of course, does not exclude semi-structured data like XML and JSON files. Data Ingestion Batch and Streaming: Data ingestion mechanisms in a data lake architecture support batch and real-time data flows. Use tools and services to auto-ingest the data so the system can effectively capture it. Validation and Metadata: Data is tagged with metadata during ingestion for easy retrieval, and initial validation checks are performed to ensure data quality and integrity. Data Governance Zone Access Control and Auditing: Implementing robust access controls, encryption, and auditing capabilities ensures data security and privacy, crucial for maintaining trust and compliance. Metadata Management: Documenting data origins, formats, lineage, ownership, and usage history is central to governance. This component incorporates tools for managing metadata, which facilitates data discovery, lineage tracking, and cataloging, enhancing the usability and governance of the data lake. Data Persistence and Staging Raw Data Storage: Data is initially stored in a staging area in raw, unprocessed form. This approach ensures that the original data is preserved for future processing needs and compliance requirements. Staging Area: Data may be staged or temporarily held in a dedicated area within the lake before processing. To efficiently handle the volume and variety of data, this area is built on scalable storage technologies, such as HDFS (Hadoop Distributed File System) or cloud-based storage services like Amazon S3. Data Processing Layer Transformation and Enrichment: This layer transforms data into a more usable format, often involving data cleaning, enrichment, deduplication, anonymization, normalization, and aggregation processes. It also improves data quality and ensures reliability for downstream analysis. Processing Engines: To cater to various processing needs, the architecture should support multiple processing engines, such as Hadoop for batch processing, Spark for in-memory processing, and others for specific tasks like stream processing. Data Indexing: This component indexes processed data to facilitate faster search and retrieval. It is crucial for supporting efficient data exploration and curation. Related: Interested in learning the techniques and best data cleaning and preprocessing practices? Check out one of our most-read guides, “Mastering Data Cleaning & Data Preprocessing.” Data Quality Monitoring Continuous Quality Checks: Implements automated processes for continuous monitoring of data quality, identifying issues like inconsistencies, duplications, or anomalies to maintain the accuracy, integrity, and reliability of the data lake. Quality Metrics and Alerts: Define and track data quality metrics, set up alert mechanisms for when data quality thresholds are breached, and enable proactive issue resolution. Related: Read how you can automate the assessment of training data quality in this article. Analytical Sandboxes Exploration and Experimentation: Computer vision engineers and data scientists can use analytical sandboxes to experiment with data sets, build models, and visually explore data (e.g., images, videos) and embeddings without impacting the integrity of the primary data (versioned data and labels). Tool Integration: These sandboxes support a wide range of analytics, data, and ML tools, giving users the flexibility and choice to work with their preferred technologies. Worth Noting: Building computer vision applications? Encord Active integrates with Annotate (with cloud platform integrations) and provides explorers with a way to explore image embeddings for any scale of data visually. See how to use it in the docs. Data Consumption Access and Integration: Data stored in the data lake is accessible to various downstream applications and users, including BI tools, reporting systems, computer vision platforms, or custom applications. This accessibility ensures that insights from the data lake can drive decision-making across the organization. APIs and Data Services: For programmatic access, APIs and data services enable developers and applications to query and retrieve data from the data lake, integrating data-driven insights into business processes and applications. Best Practices for Setting Up a Data Lake Implementing a data lake requires careful consideration and adherence to best practices to be successful and sustainable. Here are some suggested best practices to help you set up a data lake that can grow with your organization’s changing and growing data needs: #1. Define Clear Objectives and Scope Understand Your Data Needs: Before setting up a data lake, identify the types of data you plan to store, the insights you aim to derive, and the stakeholders who will consume this data. This understanding will guide your data lake's design, architecture, and governance model. Set Clear Objectives: Establish specific, measurable objectives for your data lake, such as improving data accessibility for analytics, supporting computer vision projects, or consolidating disparate data sources. These objectives will help prioritize features and guide decision-making throughout the setup process. #2. Ensure Robust Data Governance Implement a Data Governance Framework: A strong governance framework is essential for maintaining data quality, managing access controls, and ensuring compliance with regulatory standards. This framework should include data ingestion, storage, management, and archival policies. Metadata Management: Cataloging data with metadata is crucial for making it discoverable (indexing, filtering, sorting) and understandable. Implement tools and processes to automatically capture metadata, including data source, tags, format, and access permissions, during ingestion or at rest. Metadata can be technical (data design; schema, tables, formats, source documentation), business (docs on usage), and operational (events, access history, trace logs). #3. Focus on Scalability and Flexibility Choose Scalable Infrastructure: Whether on-premises or cloud-based, ensure your data lake infrastructure can scale to accommodate future data growth without significant rework or additional investment. Plan for Varied Data Types: Design your data lake to handle structured, semi-structured, and unstructured data. Flexibility in storing and processing different data types (images, videos, DICOM, blob files, etc.) ensures the data lake can support a wide range of use cases. #4. Prioritize Security and Compliance Implement Strong Security Measures: Security is paramount for protecting sensitive data and maintaining user trust. Apply encryption in transit and at rest, manage access with role-based controls, and regularly audit data access and usage. Compliance and Data Privacy: Consider the legal and regulatory requirements relevant to your data. Incorporate compliance controls into your data lake's architecture and operations, including data retention policies and the right to be forgotten. #5. Foster a Data-Driven Culture Encourage Collaboration: Promote collaboration between software engineers, CV engineers, data scientists, and analysts to ensure the data lake meets the diverse needs of its users. Regular feedback loops can help refine and enhance the data lake's utility. Education and Training: Invest in stakeholder training to maximize the data lake's value. Understanding how to use the data lake effectively can spur innovation and lead to new insights across the organization. #6. Continuous Monitoring and Optimization Monitor Data Lake Health: Regularly monitor the data lake for performance, usage patterns, and data quality issues. This proactive approach can help identify and resolve problems before they impact users. Iterate and Optimize: Your organization's needs will evolve, and so will your data lake. Continuously assess its performance and utility, adjusting based on user feedback and changing business requirements. Cloud-based Data Lake Platforms Cloud-based data lake platforms offer scalable, flexible, and cost-effective solutions for storing and analyzing large amounts of data. These platforms provide Data Lake as a Service (DLaaS), which simplifies the setup and management of data lakes. This allows organizations to focus on deriving insights rather than infrastructure management. Let's explore the architecture of data lake platforms provided by AWS, Azure, Snowflake, GCP, and their applications in multi-cloud environments. AWS Data Lake Architecture Amazon Web Services (AWS) provides a comprehensive and mature set of services to build a data lake. The core components include: Ingestion: AWS Glue for ETL processes and AWS Kinesis for real-time data streaming. Storage: Amazon S3 for scalable and secure data storage. Processing and Analysis: Amazon EMR is used for big data processing, AWS Glue for data preparation and loading, and Amazon Redshift for data warehousing. Consumption: Send your curated data to AWS SageMaker to run ML workloads or Amazon QuickSight to build visualizations, perform ad-hoc analysis, and quickly get business insights from data. Security and Governance: AWS Lake Formation automates the setup of a secure data lake, manages data access and permissions, and provides a centralized catalog for discovering and searching for data. Azure Data Lake Architecture Azure's data lake architecture is centered around Azure Data Lake Storage (ADLS) Gen2, which combines the capabilities of Azure Blob Storage and ADLS Gen1. It offers large-scale data storage with a hierarchical namespace and a secure HDFS-compatible data lake. Ingestion: Azure Data Factory for ETL operations and Azure Event Hubs for real-time event processing. Storage: ADLS Gen2 for a highly scalable data lake foundation. Processing and Consumption: Azure Databricks for big data analytics running on Apache Spark, Azure Synapse Analytics for querying (SQL serverless) and analysis (Notebooks), and Azure HDInsight for Hadoop-based services. Power BI can connect to ADLS Gen2 directly to create interactive reports and dashboards. Security and Governance: Azure provides fine-grained access control with Azure Role-Based Access Control (RBAC) and secures data with Microsoft Entra ID. Snowflake Data Lake Architecture Snowflake's unique architecture separates compute and storage, allowing users to scale them independently. It offers a cloud-agnostic solution operating across AWS, Azure, and GCP. Ingestion: Within Snowflake, Snowpipe Streaming runs on top of Apache Kafka for real-time ingestion. Apache Kafka acts as the messaging broker between the source and Snowlake. You can run batch ingestion with Python scripts and the PUT command. Storage: Uses cloud provider's storage (S3, ADLS, or Google Cloud Storage) or internal (i.e., Snowflake) stages to store structured, unstructured, and semi-structured data in their native format. Processing and Curation: Snowflake's Virtual Warehouses provide dedicated compute resources for data processing for high performance and concurrency. Snowpark can implement business logic within existing programming languages. Data Sharing and Governance: Snowflake enables secure data sharing between Snowflake accounts with governance features for managing data access and security. Consumption: Snowflake provides native connectors for popular BI and data visualization tools, including Google Analytics and Looker. Snowflake Marketplace provides users access to a data marketplace to discover and access third-party data sets and services. Snowpark helps with features for end-to-end ML. High-level architecture for running data lake workloads using Snowpark in Snowflake Google Cloud Data Lake Architecture In addition to various processing and analysis services, Google Cloud Platform (GCP) bases its data lake solutions on Google Cloud Storage (GCS), the primary data storage service. Ingestion: Cloud Pub/Sub for real-time messaging Storage: GCS offers durable and highly available object storage. Processing: Cloud Data Fusion offers pre-built transformations for batch and real-time processing, and Dataflow is for serverless stream and batch data processing. Consumption and Analysis: BigQuery provides serverless, highly scalable data analysis with an SQL-like interface. Dataproc runs Apache Hadoop and Spark jobs. Vertex AI provides machine learning capabilities to analyze and derive insights from lake data. Security and Governance: Cloud Identity and Access Management (IAM) controls resource access, and Cloud Data Loss Prevention (DLP) helps discover and protect sensitive data. Data Lake Architecture on Multi-Cloud Multi-cloud data lake architectures leverage services from multiple cloud providers, optimizing for performance, cost, and regulatory compliance. This approach often involves: Cloud-Agnostic Storage Solutions: Storing data in a manner accessible across cloud environments, either through multi-cloud storage services or by replicating data across cloud providers. Cross-Cloud Services Integration: This involves using best-of-breed services from different cloud providers for ingestion, processing, analysis, and governance, facilitated by data integration and orchestration tools. Unified Management and Governance: Implement multi-cloud management platforms to ensure consistent monitoring, security, and governance across cloud environments. Implementing a multi-cloud data lake architecture requires careful planning and robust data management strategies to ensure seamless operation, data consistency, and compliance across cloud boundaries. On-Premises Data Lakes and Cloud-based Data Lakes Organizations looking to implement data lakes have two primary deployment models to consider: on-premises and cloud-based (although more recent approaches involve a hybrid of both solutions). Cost, scalability, security, and accessibility affect each model's advantages and disadvantages. On-Premises Data Lakes: Advantages Control and Security: On-premises data lakes offer organizations complete control over their infrastructure, which can be crucial for industries with stringent regulatory and compliance requirements. This control also includes data security, so security measures can be tailored to each organization's needs. Performance: With data stored locally, on-premises solutions can provide faster data access and processing speeds, which is beneficial for time-sensitive applications that require rapid data retrieval and analysis. On-Premises Data Lakes: Challenges Cost and Scalability: Establishing an on-premises data lake requires a significant upfront investment in hardware and infrastructure. Scaling up can also require additional hardware purchases and be time-consuming. Maintenance: On-premises data lakes necessitate ongoing maintenance, including hardware upgrades, software updates, and security patches, which require dedicated IT staff and resources. Cloud-based Data Lakes: Advantages Scalability and Flexibility: Cloud-based data lakes can change their storage and computing power based on changing data volumes and processing needs without changing hardware. Cost Efficiency: A pay-as-you-go pricing model allows organizations to avoid substantial upfront investments and only pay for their storage and computing resources, potentially reducing overall costs. Innovative Features: Cloud service providers always add new technologies and features to their services, giving businesses access to the most advanced data management and analytics tools. Cloud-based Data Lakes: Challenges Data Security and Privacy: While cloud providers implement robust security measures, organizations may have concerns about storing sensitive data off-premises, particularly in industries with strict data sovereignty regulations. Dependence on Internet Connectivity: Access to cloud-based data lakes relies on stable internet connectivity. Any disruptions in connectivity can affect data access and processing, impacting operations. Understanding these differences enables organizations to select the most appropriate data lake solution to support their data management strategy and business objectives. Computer Vision Use Cases of Data Lakes Data lakes are pivotal in powering computer vision applications across various industries by providing a scalable repository for storing and analyzing vast large image and video datasets in real-time. Here are some compelling use cases where data lakes improve computer vision applications: Healthcare: Medical Imaging and Diagnosis In healthcare, data lakes store vast collections of medical images (e.g., X-rays, MRIs, CT scans, PET) that, combined with data curation tools, can improve image quality, detect anomalies, and provide quantitative assessments. CV algorithms analyze these images in real time to diagnose diseases, monitor treatment progress, and plan surgeries. Case Study: Viz.ai uses artificial intelligence to speed care and improve patient outcomes. In this case study, learn how they ingest, annotate, curate, and consume medical data. Autonomous Vehicles: Navigation and Safety Autonomous vehicle developers use data lakes to ingest and curate diverse datasets from vehicle sensors, including cameras, LiDAR, and radar. This data is crucial for training computer vision algorithms that enable autonomous driving capabilities, such as object detection, automated curb management, traffic sign recognition, and pedestrian tracking. Case Study: Automotus builds real-time curbside management automation solutions. Learn how they ingested raw, unlabeled data into Encord via Annotate and curated a balanced, diverse dataset with Active in this case study. How Automotus increased mAP 20% by reducing their dataset size by 35% with visual data curation Agriculture: Precision Farming In the agricultural sector, data lakes store and curate visual data (images and videos) captured by drones or satellites over farmland. Computer vision techniques analyze this data to assess crop health, identify pest infestations, and evaluate water usage, so farmers can make informed decisions and apply treatments selectively. Case Study: Automated harvesting and analytics company Four Growers uses Encord’s platform and annotators to help build its training datasets from scratch, labeling millions of instances of greenhouses and plants. Learn how the platform has halved the time it takes for them to build training data in this case study. Security and Surveillance: Threat Detection Government and private security agencies use data lakes to compile video feeds from CCTV cameras in public spaces, airports, and critical infrastructure. Real-time analysis with computer vision helps detect suspicious activities, unattended objects, and unauthorized entries, triggering immediate responses to potential security threats. ML Team's Data Lake Guide: Key Takeaways Data lakes have become essential for scalable storage and processing of diverse data types in modern data management. They facilitate advanced analytics, including real-time applications like computer vision. Their ability to transform sectors ranging from finance to agriculture by enhancing operational efficiencies and providing actionable insights makes them invaluable. As we look ahead: The continuous evolution of data lake architectures, especially within cloud-native and multi-cloud contexts, promises to bring forth advanced tools and services for improved data handling. This progression presents an opportunity for enterprises to transition from viewing data lakes merely as data repositories to leveraging them as strategic assets capable of building advanced CV applications. To maximize data lakes, address the problems associated with data governance, security, and quality. This will ensure that data remains a valuable organizational asset and a catalyst for data-driven decision-making and strategy formulation.
Mar 28 2024
11 M
Top 12 Dimensionality Reduction Techniques for Machine Learning
Dimensionality reduction is a fundamental technique in machine learning (ML) that simplifies datasets by reducing the number of input variables or features. This simplification is crucial for enhancing computational efficiency and model performance, especially as datasets grow in size and complexity. High-dimensional datasets, often comprising hundreds or thousands of features, introduce the "curse of dimensionality." This effect slows down algorithms by making data scarceness (sparsity) and computing needs grow exponentially. Dimensionality reduction changes the data into a simpler, lower-dimensional space that is easier to work with while keeping its main features. This makes computation easier and lowers the risk of overfitting. This strategy is increasingly indispensable in the era of big data, where managing vast volumes of information is a common challenge. This article provides insight into various approaches, from classical methods like principal component analysis (PCA) and linear discriminant analysis (LDA) to advanced techniques such as manifold learning and autoencoders. Each technique has benefits and works best with certain data types and ML problems. This shows how flexible and different dimensionality reduction methods are for getting accurate and efficient model performance when dealing with high-dimensional data. Here are the Twelve (12) techniques you will learn in this article: Manifold Learning (t-SNE, UMAP) Principal Component Analysis (PCA) Independent Component Analysis (ICA) Sequential Non-negative Matrix Factorization (NMF) Linear Discriminant Analysis (LDA) Generalized Discriminant Analysis (GDA) Missing Values Ratio (MVR): Threshold Setting Low Variance Filter High Correlation Filter Forward Feature Construction Backward Feature Elimination Autoencoders Classification of Dimensionality Reduction Techniques Dimensionality reduction techniques preserve important data, make it easier to use in other situations, and speed up learning. They do this using two steps: feature selection, which preserves the most important variables, and feature projection, which creates new variables by combining the original ones in a big way. Feature Selection Techniques Techniques classified under this category can identify and retain the most relevant features for model training. This approach helps reduce complexity and improve interpretability without significantly compromising accuracy. They are divided into: Embedded Methods: These integrate feature selection within model training, such as LASSO (L1) regularization, which reduces feature count by applying penalties to model parameters and feature importance scores from Random Forests. Filters: These use statistical measures to select features independently of machine learning models, including low-variance filters and correlation-based selection methods. More sophisticated filters involve Pearson’s correlation and Chi-Squared tests to assess the relationship between each feature and the target variable. Wrappers: These assess different feature subsets to find the most effective combination, though they are computationally more demanding. Feature Projection Techniques Feature projection transforms the data into a lower-dimensional space, maintaining its essential structures while reducing complexity. Key methods include: Manifold Learning (t-SNE, UMAP). Principal Component Analysis (PCA). Kernel PCA (K-PCA). Linear Discriminant Analysis (LDA). Quadratic Discriminant Analysis (QDA). Generalized Discriminant Analysis (GDA). 1. Manifold Learning Manifold learning, a subset of non-linear dimensionality reduction techniques, is designed to uncover the intricate structure of high-dimensional data by projecting it into a lower-dimensional space. Understanding Manifold Learning At the heart of Manifold Learning is that while data may exist in a high-dimensional space, the intrinsic dimensionality—representing the true degrees of freedom within the data—is often much lower. For example, images of faces, despite being composed of thousands of pixels (high-dimensional data points), might be effectively described with far fewer dimensions, such as the angles and distances between key facial features. Core Techniques and Algorithms t-Distributed Stochastic Neighbor Embedding (t-SNE): t-SNE is powerful for visualizing high-dimensional data in two or three dimensions. It converts similarities between data points to joint probabilities and minimizes the divergence between them in different spaces, excelling in revealing clusters within data. Uniform Manifold Approximation and Projection (UMAP): UMAP is a relatively recent technique that balances the preservation of local and global data structures for superior speed and scalability. It's computationally efficient and has gained popularity for its ability to handle large datasets and complex topologies. Isomap (Isometric Mapping): Isomap extends classical Multidimensional Scaling (MDS) by incorporating geodesic distances among points. It's particularly effective for datasets where the manifold (geometric surface) is roughly isometric to a Euclidean space, allowing global properties to be preserved. Locally Linear Embedding (LLE): LLE reconstructs high-dimensional data points from their nearest neighbors, assuming the manifold is locally linear. By preserving local relationships, LLE can unfold twisted or folded manifolds. t-SNE and UMAP are two of the most commonly applied dimensionality reduction techniques. At Encord, we use UMAP to generate the 2D embedding plots in Encord Active. 2. Principal Component Analysis (PCA) The Principal Component Analysis (PCA) algorithm is a method used to reduce the dimensionality of a dataset while preserving as much information (variance) as possible. As a linear reduction method, PCA transforms a complex dataset with many variables into a simpler one that retains critical trends and patterns. What is variance? Variance measures the data spread around the mean, and features with low variance indicate little variation in their values. These features often need to be more formal for subsequent analysis and can hinder model performance. What is Principal Component Analysis (PCA)? PCA identifies and uses the principal components (directions that maximize variance and are orthogonal to each other) to effectively project data into a lower-dimensional space. This process begins with standardizing the original variables, ensuring their equal contribution to the analysis by normalizing them to have a zero mean and unit variance. Step-by-Step Explanation of Principal Component Analysis Standardization: Normalize the data so each variable contributes equally, addressing PCA's sensitivity to variable scales. Covariance Matrix Computation: Compute the covariance matrix to understand how the variables of the input dataset deviate from the mean and to see if they are related (i.e., correlated). Finding Eigenvectors and Eigenvalues: Find the new axes (eigenvectors) that maximize variance (measured by eigenvalues), making sure they are orthogonal to show that variance can go in different directions. Sorting and Ranking: Prioritize eigenvectors (and thus principal components) by their ability to capture data variance, using eigenvalues as the metric of importance. Feature Vector Formation: Select a subset of eigenvectors based on their ranking to form a feature vector. This subset of eigenvectors forms the principal components. Transformation: Map the original data into this principal component space, enabling analysis or further machine learning in a more tractable, less noisy space. Dimensionality reduction using PCA Applications PCA is widely used in exploratory data analysis and predictive modeling. It is also applied in areas like image compression, genomics for pattern recognition, and financial data for uncovering latent patterns and correlations. PCA can help visualize complex datasets by reducing data dimensionality. It can also make machine learning algorithms more efficient by reducing computational costs and avoiding overfitting with high-dimensional data. 3. Independent Component Analysis (ICA) Independent Component Analysis (ICA) is a computational method in signal processing that separates a multivariate signal into additive, statistically independent subcomponents. Statistical independence is critical because Gaussian variables maximize entropy given a fixed variance, making non-Gaussianity a key indicator of independence. Originating from the work of Hérault and Jutten in 1985, ICA excels in applications like the "cocktail party problem," where it isolates distinct audio streams amid noise without prior source information. Example of the cocktail party problem The cocktail party problem involves separating original sounds, such as music and voice, from mixed signals recorded by two microphones. Each microphone captures a different combination of these sounds due to its varying proximity to the sound sources. ICA is distinct from methods like PCA because it focuses on maximizing statistical independence between components rather than merely de-correlating them. Principles Behind Independent Component Analysis The essence of ICA is its focus on identifying and separating independent non-Gaussian signals embedded within a dataset. It uses the fact that these signals are statistically independent and non-Gaussian to divide the mixed signals into separate parts from different sources. This demixing process is pivotal, transforming seemingly inextricable data (impossible to separate) into interpretable components. Two main strategies for defining component independence in ICA are the minimization of mutual information and non-Gaussianity maximization. Various algorithms, such as infomax, FastICA, and kernel ICA, implement these strategies through measures like kurtosis and negentropy. Algorithmic Process To achieve its goals, ICA incorporates several preprocessing steps: Centering adjusts the data to have a zero mean, ensuring that analyses focus on variance rather than mean differences. Whitening transforms the data into uncorrelated variables, simplifying the subsequent separation process. After these steps, ICA applies iterative methods to separate independent components, and it often uses auxiliary methods like PCA or singular value decomposition (SVD) to lower the number of dimensions at the start. This sets the stage for efficient and robust component extraction. By breaking signals down into basic, understandable parts, ICA provides valuable information and makes advanced data analysis easier, which shows its importance in modern signal processing and beyond. Let’s see some of its applications. Applications of ICA The versatility of ICA is evident across various domains: In telecommunications, it enhances signal clarity amidst interference. Finance benefits from its ability to identify underlying factors in complex market data, assess risk, and detect anomalies. In biomedical signal analysis, it dissects EEG or fMRI data to isolate neurological activity from artifacts (such as eye blinks). 4. Sequential Non-negative Matrix Factorization (NMF) Nonnegative matrix Factorization (NMF) is a technique in multivariate analysis and linear algebra in which a matrix V is factorized into two lower-dimensional matrices, W (basis matrix) and H (coefficient matrix), with the constraint that all matrices involved have no negative elements. This factorization works especially well for fields where the data is naturally non-negative, like genetic expression data or audio spectrograms, because it makes it easy to understand the parts. The primary aim of NMF is to reduce dimensionality and uncover hidden/latent structures in the data. Principle of Sequential Non-negative Matrix Factorization The distinctive aspect of Sequential NMF is its iterative approach to decomposing matrix V into W and H, making it adept at handling time-series data or datasets where the temporal evolution of components is crucial. This is particularly relevant in dynamic datasets or applications where data evolves. Sequential NMF responds to changes by repeatedly updating W and H, capturing changing patterns or features important in online learning, streaming data, or time-series analysis. In text mining, for example, V denotes a term-document matrix over time, where W represents evolving topics and H indicates their significance across different documents or time points. This dynamic representation allows the monitoring of trends and changes in the dataset's underlying structure. Procedure of feature extraction using NMF Applications The adaptability of Sequential NMF has led to its application in a broad range of fields, including: Medical Research: In oncology, Sequential NMF plays a pivotal role in analyzing genetic data over time, aiding in the classification of cancer types, and identifying temporal patterns in biomarker expression. Audio Signal Processing: It is used to analyze sequences of audio signals and capture the temporal evolution of musical notes or speech. Astronomy and Computer Vision: Sequential NMF tracks and analyzes the temporal changes in celestial bodies or dynamic scenes. 5. Linear Discriminant Analysis (LDA) Linear Discriminant Analysis (LDA) is a supervised machine learning technique used primarily for pattern classification, dimensionality reduction, and feature extraction. It focuses on maximizing class separability. Unlike PCA, which optimizes for variance regardless of class labels, LDA aims to find a linear combination of features that separates different classes. It projects data onto a lower-dimensional space using class labels to accomplish this. Imagine, for example, a dataset of two distinct groups of points spread in space; LDA aims to find a projection where these groups are as distinct as possible, unlike PCA, which would look for the direction of highest variance regardless of class distinction. This method is highly efficient in scenarios where the division between categories of data is to be accentuated. PCA Vs. LDA: What's the Difference? Assumptions of LDA Linear Discriminant Analysis (LDA) operates under assumptions essential for effectively classifying observations into predefined groups based on predictor variables. These assumptions, elaborated below, play a critical role in the accuracy and reliability of LDA's predictions. Multivariate Normality: Each class must follow a multivariate normal distribution (multi-dimensional bell curve). You can asses this through visual plots or statistical tests before applying LDA. Homogeneity of Variances (Homoscedasticity): Ensuring uniform variance across groups helps maintain the reliability of LDA's projections. Techniques like Levene's test can assess this assumption. Absence of Multicollinearity: LDA requires predictors to be relatively independent. Techniques like variance inflation factors (VIFs) can diagnose multicollinearity issues. Working Methodology of Linear Discriminant Analysis LDA transforms the feature space into a lower-dimensional one that maximizes class separability by: Calculating mean vectors for each class. Computing within-class and between-class scatter matrices to understand the distribution and separation of classes. Solving for the eigenvalues and eigenvectors that maximize the between-class variance relative to the within-class variance. This defines the optimal projection space to distinguish the classes. Tools like Python's Scikit-learn library simplify applying LDA with functions specifically designed to carry out these steps efficiently. Applications LDA's ability to reduce dimensionality while preserving as much of the class discriminatory information as possible makes it a powerful feature extraction and classification tool applicable across various domains. Examples: In facial recognition, LDA enhances the distinction between individual faces to improve recognition accuracy. Medical diagnostics benefit from LDA's ability to classify patient data into distinct disease categories, aiding in early and accurate diagnosis. In marketing, LDA helps segment customers for targeted marketing campaigns based on demographic and behavioral data. 6. Generalized Discriminant Analysis (GDA) Generalized Discriminant Analysis (GDA) extends linear discriminant analysis (LDA) into a nonlinear domain. It uses kernel functions to project input data vectors into a higher-dimensional feature space to capture complex patterns that LDA, limited to linear boundaries, might miss. These functions project data into a higher-dimensional space where inseparable classes in the original space can be distinctly separated. Step-by-step Explanation of Generalized Discriminant Analysis The core objective of GDA is to find a low-dimensional projection that maximizes the between-class scatter while minimizing the within-class scatter in the high-dimensional feature space. Let’s examine the GDA algorithm step by step: 1. Kernel Function Selection: First, choose an appropriate kernel function (e.g., polynomial, radial basis function (RBF)) that transforms the input data into a higher-dimensional space. 2. Kernel Matrix Computation: Compute the kernel matrix K, representing the high-dimensional dot products between all pairs of data points. This matrix is central to transforming the data into a feature space without explicitly performing the computationally expensive mapping. 3. Scatter Matrix Calculation in Feature Space: In the feature space, compute the within-class scatter matrix SW and the between-class scatter matrix SB, using the kernel matrix K to account for the data's nonlinear transformation. 4. Eigenvalue Problem: Solving this problem in the feature space identifies the projection vectors that best separate the classes by maximizing the SB/SW ratio. This step is crucial for identifying the most informative projections for class separation. 5. Projection: Use the obtained eigenvectors to project the input data onto a lower-dimensional space that maximizes class separability to achieve GDA's goal of improved class recognition. Applications GDA has been applied in various domains, benefiting from its ability to handle nonlinear patterns: Image and Video Recognition: GDA is used for facial recognition, object detection, and activity recognition in videos, where the data often exhibit complex, nonlinear relationships. Biomedical Signal Processing: In analyzing EEG, ECG signals, and other biomedical data, GDA helps distinguish between different physiological states or diagnose diseases. Text Classification and Sentiment Analysis: GDA transforms text data into a higher-dimensional space, effectively separating documents or sentiments that are not linearly separable in the original feature space. 7. Missing Values Ratio (MVR): Threshold Setting Datasets often contain missing values, which can significantly impact the effectiveness of dimensionality reduction techniques. One approach to addressing this challenge is to utilize a missing values ratio (MVR) thresholding technique for feature selection. Process of Setting Threshold for Missing Values The MVR for a feature is calculated as the percentage of missing values for data points. The optimal threshold is dependent on several factors, including the dataset’s nature and the intended analysis: Determining the Threshold: Use statistical analyses, domain expertise, and exploratory data analysis (e.g., histograms of missing value ratios) to identify a suitable threshold. This decision balances retaining valuable data against excluding features that could introduce bias or noise. Implications of Threshold Settings: A high threshold may retain too many features with missing data, complicating the analysis. Conversely, a low threshold could lead to excessive data loss. Regularly, thresholds between 20% to 60% are considered, but this range varies widely based on the data context and analysis goals. Contextual Considerations: The dataset's specific characteristics and the chosen dimensionality reduction technique influence the threshold setting. Methods sensitive to data sparsity or noise may require a lower MVR threshold. Example: In a dataset with 100 observations, a feature with 75 missing values has an MVR of 75%. If the threshold is set at 70%, this feature would be considered for removal. Applications High-throughput Biological Data Analysis: Technical limitations often render Gene expression data incomplete. Setting a conservative MVR threshold may preserve crucial biological insights by retaining genes with marginally incomplete data. Customer Data Analysis: Customer surveys may have varying completion rates across questions. MVR thresholding identifies which survey items provide the most complete and reliable data, sharpening customer insights. Social Media Analysis: Social media data can be sparse, with certain users' entries missing. MVR thresholding can help select informative features for user profiling or sentiment analysis. 8. Low Variance Filter A low variance filter is a straightforward preprocessing technique aimed at reducing dimensionality by eliminating features with minimal variance, focusing analysis on more informative aspects of the dataset. Steps for Implementing a Low Variance Filter Calculate Variance: For each feature in the dataset, compute the variance. Prioritize scaling or normalizing data to ensure variance is measured on a comparable basis across all features. Set Threshold: Define a threshold for the minimum acceptable variance. This threshold often depends on the specific dataset and analysis objectives but typically ranges from a small percentage of the total variance observed across features. Feature Selection: Exclude features with variances below the threshold. Tools like Python's `pandas` library or R's `caret` package can efficiently automate this process. Applications of Low Variance Filter Across Domains Sensor Data Analysis: Sensor readings might exhibit minimal fluctuation over time, leading to features with low variance. Removing these features can help focus on the sensor data's more dynamic aspects. Image Processing: Images can contain features representing background noise. These features often have low variance and can be eliminated using the low variance filter before image analysis. Text Classification: Text data might contain stop words or punctuation marks that offer minimal information for classification. The low variance filter can help remove such features, improving classification accuracy. 9. High Correlation Filter The high correlation filter is a crucial technique for addressing feature redundancy. Eliminating highly correlated features optimizes datasets for improved model accuracy and efficiency. Steps for Implementing a High Correlation Filter Compute Correlation Matrix: Assess the relationship between all feature pairs using an appropriate correlation coefficient, such as Pearson for continuous features (linear relationships) and Spearman for ordinal (monotonic relationships). Define Threshold: Establish a correlation coefficient threshold above highly correlated features. A common threshold of 0.8 or 0.9 may vary based on specific model requirements and data sensitivity. Feature Selection: Identify sets of features whose correlation exceeds the threshold. From each set, retain only one feature based on criteria like predictive power, data completeness, or domain relevance and remove the others. Applications Financial Data Analysis: Stock prices or other financial metrics might exhibit a high correlation, often reflecting market trends. The high correlation filter can help select a representative subset of features for financial modeling. Bioinformatics: Gene expression data can involve genes with similar functions, leading to high correlation. Selecting a subset of uncorrelated genes can be beneficial for identifying distinct biological processes. Recommendation Systems: User profiles often contain correlated features like similar purchase history or browsing behavior. The high correlation filter can help select representative features to build more efficient recommendation models. While the Low Variance Filter method removes features with minimal variance, discarding data points that likely don't contribute much information, the High Correlation Filter approach identifies and eliminates highly correlated features. This process is crucial because two highly correlated features carry similar information, increasing redundancy within the model. 10. Forward Feature Construction Forward Feature Construction (FFC) is a methodical approach to feature selection, designed to incrementally build a model by adding features that offer the most significant improvement. This technique is particularly effective when the relationship between features and the target variable is complex and needs to be fully understood. Algorithm for Forward Feature Construction Initiate with a Null Model: Start with a baseline model without any predictors to establish a performance benchmark. Evaluation Potential Additions: For each candidate feature outside the model, assess potential performance improvements by adding that feature. Select the Best Feature: Incorporate the feature that significantly improves performance. Ensure the model remains interpretable and manageable. Iteration: Continue adding features until further additions fail to offer significant gains, considering computational efficiency and the risk of diminishing returns. Practical Considerations and Implementation Performance Metrics: To gauge improvements, use appropriate metrics, such as the Akaike Information Criterion (AIC) for regression or accuracy and the F1 score for classification, adapting the choice of metric to the model's context. Challenges: Be mindful of computational demands and the potential for multicollinearity. Implementing strategies to mitigate these risks, such as pre-screening features or setting a cap on the number of features, can be crucial. Tools: Leverage software tools and libraries (e.g., R's `stepAIC` or Python's `mlxtend.SequentialFeatureSelector`) that support efficient FFC application and streamline feature selection. Applications of FFC Across Domains Clinical Trials Prediction: In clinical research, FFC facilitates the identification of the most predictive biomarkers or clinical variables from a vast dataset, optimizing models for outcome prediction. Financial Modeling: In financial market analysis, this method distills a complex set of economic indicators down to a core subset that most accurately forecasts market movements or financial risk. 11. Backward Feature Elimination Backward Feature Elimination (BFE) systematically simplifies machine learning models by iteratively removing the least critical features, starting with a model that includes the entire set of features. This technique is particularly suited for refining linear and logistic regression models, where dimensionality reduction can significantly improve performance and interpretability. Algorithm for Backward Feature Elimination Initialize with Full Model: Construct a model incorporating all available features to establish a comprehensive baseline. Identify and Remove Least Impactful Feature: Determine the feature whose removal least affects or improves the model's predictive performance. Use metrics like p-values or importance scores to eliminate it from the model. Performance Evaluation: After each removal, assess the model to ensure performance remains robust. Utilize cross-validation or similar methods to validate performance objectively. Iterative Optimization: Continue this evaluation and elimination process until further removals degrade model performance, indicating that an optimal feature subset has been reached. Learn how to validate the performance of your ML model in this guide to validation model performance with Encord Active. Practical Considerations for Implementation Computational Efficiency: Given the potentially high computational load, especially with large feature sets, employ strategies like parallel processing or stepwise evaluation to simplify the Backward Feature Elimination (BFE) process. Complex Feature Interactions: Special attention is needed when features interact or are categorical. Consider their relationships to avoid inadvertently removing significant predictors. Applications Backward Feature Elimination is particularly useful in contexts like: Genomics: In genomics research, BFE helps distill large datasets into a manageable number of significant genes to improve understanding of genetic influences on diseases. High-dimensional Data Analysis: BFE simplifies complex models in various fields, from finance to the social sciences, by identifying and eliminating redundant features. This could reduce overfitting and improve the model's generalizability. While Forward Feature Construction is beneficial for gradually building a model by adding one feature at a time, Backward Feature Elimination is advantageous for models starting with a comprehensive set of features and needing to identify redundancies. 12. Autoencoders Autoencoders are a unique type of neural network used in deep learning, primarily for dimensionality reduction and feature learning. They are designed to encode inputs into a compressed, lower-dimensional form and reconstruct the output as closely as possible to the original input. This process emphasizes the encoder-decoder structure. The encoder reduces the dimensionality, and the decoder attempts to reconstruct the input from this reduced encoding. How Does Autoencoders Work? They achieve dimensionality reduction and feature learning by mimicking the input data through encoding and decoding. 1. Encoding: Imagine a bottle with a narrow neck in the middle. The data (e.g., an image) is the input that goes into the wide top part of the bottle. The encoder acts like this narrow neck, compressing the data into a smaller representation. This compressed version, often called the latent space representation, captures the essential features of the original data. The encoder is typically made up of multiple neural network layers that gradually reduce the dimensionality of the data. The autoencoder learns to discard irrelevant information and focus on the most important characteristics by forcing the data through this bottleneck. 2. Decoding: Now, imagine flipping the bottle upside down. The decoder acts like the wide bottom part, trying to recreate the original data from the compressed representation that came through the neck. The decoder also uses multiple neural network layers, but this time, it gradually increases the data's dimensionality, aiming to reconstruct the original input as accurately as possible. Variants and Advanced Applications Sparse Autoencoders: Introduce regularization terms to enforce sparsity in the latent representation, enhancing feature selection. Denoising Autoencoders: Specifically designed to remove noise from data, these autoencoders learn to recover clean data from noisy inputs, offering superior performance in image and signal processing tasks. Variational Autoencoders (VAEs): VAEs make new data samples possible by treating the latent space as a probabilistic distribution. This opens up new ways to use generative modeling. Training Nuances Autoencoders use optimizers like Adam or stochastic gradient descent (SGD) to improve reconstruction accuracy by improving their weights through backpropagation. Overfitting prevention is integral and can be addressed through methods like dropout, L1/L2 regularization, or a validation set for early stopping. Applications Autoencoders have a wide range of applications, including but not limited to: Dimensionality Reduction: Similar to PCA but more powerful (as non-linear alternatives), autoencoders can perform non-linear dimensionality reductions, making them particularly useful for preprocessing steps in machine learning pipelines. Image Denoising: By learning to map noisy inputs to clean outputs, denoising autoencoders can effectively remove noise from images, surpassing traditional denoising methods in efficiency and accuracy. Generative modeling: Variational autoencoders (VAEs) can make new data samples similar to the original input data by modeling the latent space as a continuous probability distribution. (e.g., Generative Adversarial Networks (GANs)). Impact of Dimensionality Reduction in Smart City Solutions Automotus is a company at the forefront of using AI to revolutionize smart city infrastructure, particularly traffic management. They achieve this by deploying intelligent traffic monitoring systems that capture vast amounts of video data from urban environments. However, efficiently processing and analyzing this high-dimensional data presents a significant challenge. This is where dimensionality reduction techniques come into play. The sheer volume of video data generated by Automotus' traffic monitoring systems necessitates dimensionality reduction techniques to make data processing and analysis manageable. PCA identifies the most significant features in the data (video frames in this case) and transforms them into a lower-dimensional space while retaining the maximum amount of variance. This allows Automotus to extract the essential information from the video data, such as traffic flow patterns, vehicle types, and potential congestion points, without analyzing every pixel. Partnering with Encord, Automotus led to a 20% increase in model accuracy and a 35% reduction in dataset size. This collaboration focused on dimensionality reduction, leveraging Encord Annotate’s flexible ontology, quality control capabilities, and automated labeling features. That approach helped Automotus reduce infrastructure constraints, improve model performance to provide better data to clients, and reduce labeling costs. Efficiency directly contributes to Automotus's business growth and operational scalability. The team used Encord Active to visually inspect, query, and sort their datasets to remove unwanted and poor-quality data with just a few clicks, leading to a 35% reduction in the size of the datasets for annotation. This enabled the team to cut their labeling costs by over a third. Interested in learning more? Read the full story on Encord's website for more details. Dimensionality Reduction Technique: Key Takeaways Dimensionality reduction techniques simplify models and enhance computational efficiency. They help manage the "curse of dimensionality," improving model generalizability and reducing overfitting risk. These techniques are used for feature selection and extraction, contributing to better model performance. They are applied in various fields, such as image and speech recognition, financial analysis, and bioinformatics, showcasing their versatility. By reducing the number of input variables, these methods ensure models are computationally efficient and capture essential data patterns for more accurate predictions.
Mar 22 2024
10 M
Improving Data Quality Using End-to-End Data Pre-Processing Techniques in Encord Active
In computer vision, you cannot overstate the importance of data quality. It directly affects how accurate and reliable your models are. This guide is about understanding why high-quality data matters in computer vision and how to improve your data quality. We will explore the essential aspects of data quality and its role in model accuracy and reliability. We will discuss the key steps for improving quality, from selecting the right data to detecting outliers. We will also see how Encord Active helps us do all this to improve our computer vision models. This is an in-depth guide; feel free to use the table of contents on the left to navigate each section and find one that interests you. By the end, you’ll have a solid understanding of the essence of data quality for computer vision projects and how to improve it to produce high-quality models. Let’s dive right into it! Introduction to Data Quality in Computer Vision Defining the Attributes of High-Quality Data High-quality data includes several attributes that collectively strengthen the robustness of computer vision models: Accuracy: Precision in reflecting real-world objects is vital; inaccuracies can lead to biases and diminished performance. Consistency: Uniformity in data, achieved through standardization, prevents conflicts and aids effective generalization. Data Diversity: By incorporating diverse data, such as different perspectives, lighting conditions, and backgrounds, you enhance the model's adaptability, making it resilient to potential biases and more adept at handling unforeseen challenges. Relevance: Data curation should filter irrelevant data, ensuring the model focuses on features relevant to its goals. Ethical Considerations: Data collected and labeled ethically, without biases, contributes to responsible and fair computer vision models. By prioritizing these data attributes, you can establish a strong foundation for collecting and preparing quality data for your computer vision projects. Next, let's discuss the impact of these attributes on model performance. Impact of Data Quality on Model Performance Here are a few aspects of high-quality data that impact the model's performance: Accuracy Improvement: Curated and relevant datasets could significantly improve model accuracy. Generalization Capabilities: High-quality data enables models to apply learned knowledge to new, unseen scenarios. Increased Model Robustness: Robust models are resilient to variations in input conditions, which is perfect for production applications. As we explore enhancing data quality for training computer vision models, it's essential to underscore that investing in data quality goes beyond mere accuracy. It's about constructing a robust and dependable system. By prioritizing clean, complete, diverse, and representative data, you establish the foundation for effective models. Considerations for Training Computer Vision Models Training a robust computer vision model hinges significantly on the training data's quality, quantity, and labeling. Here, we explore the key considerations for training CV models: Data Quality The foundation of a robust computer vision model rests on the quality of its training data. Data quality encompasses the accuracy, completeness, reliability, and relevance of the information within the dataset. Addressing missing values, outliers, and noise is crucial to ensuring the data accurately reflects real-world scenarios. Ethical considerations, like unbiased representation, are also paramount in curating a high-quality dataset. Data Diversity Data diversity ensures that the model encounters many scenarios. Without diversity, models risk being overly specialized and may struggle to perform effectively in new or varied environments. By ensuring a diverse dataset, models can better generalize and accurately interpret real-world situations, improving their robustness and reliability. Data Quantity While quality takes precedence, an adequate volume of data is equally vital for comprehensive model training. Sufficient data quantity contributes to the model's ability to learn patterns, generalize effectively, and adapt to diverse situations. The balance of quality and quantity ensures a holistic learning experience for the model, enabling it to navigate various scenarios. It's also important to balance the volume of data with the model's capacity and computational efficiency to avoid issues like overfitting and unnecessary computational load. Label Quality The quality of its labels greatly influences the precision of a computer vision model. Consistent and accurate labeling with sophisticated annotation tools is essential for effective training. Poorly labeled data can lead to biases and inaccuracies, undermining the model's predictive capabilities. Read How to Choose the Right Data for Your Computer Vision Project to learn more about it. Data Curation and Management Tool A robust data curation and management tool is crucial for maintaining high-quality datasets. These tools support the organization, cleaning, and integration of data, enhancing its overall reliability. By offering intuitive interfaces, efficient data workflows, and comprehensive management options, these tools simplify the process of refining and maintaining valuable datasets. Well-curated data ensures that the model is trained on clean, relevant, and well-organized datasets, greatly enhancing its learning process and overall performance. Data Annotation Tool A reliable data annotation tool is equally essential to ensuring high-quality data. These tools facilitate the labeling of images, improving the quality of the data. By providing a user-friendly interface, efficient workflows, and diverse annotation options, these tools streamline the process of adding valuable insights to the data. Properly annotated data ensures the model receives accurate ground truth labels, significantly contributing to its learning process and overall performance. Selecting the Right Data for Your Computer Vision Projects The first step in improving data quality is data curation. This process involves defining criteria for data quality and establishing mechanisms for sourcing reliable datasets. Here are a few key steps to follow when selecting the data for your computer vision project: Criteria for Selecting Quality Data The key criteria for selecting high-quality data include: Accuracy: Data should precisely reflect real-world scenarios to avoid biases and inaccuracies. Completeness: Comprehensive datasets covering diverse situations are crucial for generalization. Consistency: Uniformity in data format and preprocessing ensures reliable model performance. Timeliness: Regular updates maintain relevance, especially in dynamic or evolving environments. Evaluating and Sourcing Reliable Data The process of evaluating and selecting reliable data involves: Quality Metrics: Validating data integrity through comprehensive quality metrics, ensuring accuracy, completeness, and consistency in the dataset. Ethical Considerations: Ensuring data is collected and labeled ethically without introducing biases. Source Reliability: Assessing and selecting trustworthy data sources to mitigate potential biases. Case Studies: Improving Data Quality Improved Model Performance by 20% When faced with challenges managing and converting vast amounts of images into labeled training data, Autonomous turned to Encord. The flexible ontology structure, quality control capabilities, and automated labeling features of Encord were instrumental in overcoming labeling obstacles. The result was twofold: improved model performance and economic efficiency. With Encord, Autonomous efficiently curated and reduced the dataset by getting rid of data that was not useful. This led to a 20% improvement in mAP (mean Average Precision), a key metric for measuring the accuracy of object detection models. This was not only effective in addressing the accuracy of the model but also in reducing labeling costs. Efficient data curation helped prioritize which data to label, resulting in a 33% reduction in labeling costs. Thus, improving the accuracy of the models enhanced the quality of the data that Autonomous delivered to its customers. Read the case study on how Automotus increased mAP by 20% by reducing their dataset size by 35% with visual data curation to learn more about it. Exploring Data Curation and Management using Encord Index Encord Index is a tool designed to streamline data curation and management for machine learning and AI applications. It offers a range of features that help organizations effectively manage their datasets, ensuring data quality and integrity throughout the machine learning lifecycle. Key Features Data Versioning: Encord Index tracks different versions of your datasets, allowing you to monitor changes and compare data distributions over time. This feature helps in identifying data drift and ensures that your models are trained on the most current and relevant data. Quality Assurance: Encord Index includes robust quality assurance tools that detect and rectify data quality issues. By identifying labeling errors, missing values, and inconsistencies, it ensures that your datasets are of the highest quality, which is critical for training accurate models. Metadata Management: Effective metadata management is crucial for organizing and retrieving datasets. Encord Index allows you to create and manage metadata, making it easier to search, filter, and understand your data. Visualization and Analysis: The platform provides powerful visualization tools that help you analyze your data, identify patterns, and make informed decisions. Visualizing data distributions and anomalies can significantly enhance the data curation process. Following data curation, the next step involves inspecting the quality of the data. Let's learn how to explore data quality with Encord Active. Exploring Data Quality using Encord Active Encord provides a comprehensive set of tools to curate, maintain, evaluate, and improve the quality of your data. It uses quality metrics to assess the quality of your data, labels, and model predictions. Data Quality Metrics analyzes your images, sequences, or videos. These metrics are label-agnostic and depend only on the image content. Examples include image uniqueness, diversity, area, brightness, sharpness, etc. Label Quality Metrics operates on image labels like bounding boxes, polygons, and polylines. These metrics can help you sort data, filter it, find duplicate labels, and understand the quality of your annotations. Examples include border proximity, broken object tracks, classification quality, label duplicates, object classification quality, etc. Read How to Detect Data Quality Issues in a Torchvision Dataset Using Encord Active for a more comprehensive insight. In addition to the metrics that ship with Encord Active, you can define custom quality metrics for indexing your data. This allows you to customize the evaluation of your data according to your specific needs. Here's a step-by-step guide to exploring data quality through Encord Active: Create an Encord Active Project Initiating your journey with Encord Active begins with creating a project in Index and then Annotate, setting the foundation for an efficient and streamlined data annotation process. Follow these steps for a curation workflow from Index to Annotate to Active: Create a Project in Index. Add an existing dataset or create your own dataset. To label the curated dataset, create a project in Annotate. Set up the ontology of the annotation project. Customize the workflow design to assign tasks to annotators and for expert review. Start the annotation process! Read the documentation to learn how to create your annotation project on Encord Annotate. Import Encord Active Project Once you label a project in Annotate, transition to Active by clicking Import Annotate Project. Read the documentation to learn how to import your Encord Annotate project to Encord Active Cloud. Using Quality Metrics After choosing your project, navigate to Filter on the Explorer page >> Choose a Metric from the selection of data quality metrics to visually analyze the quality of your dataset. Great! That helps you identify potential issues such as inconsistencies, outliers, etc., which helps make informed decisions regarding data cleaning. Guide to Data Cleaning Data cleaning involves identifying and rectifying errors, inconsistencies, and inaccuracies in datasets. This critical phase ensures that the data used for computer vision projects is reliable, accurate, and conducive to optimal model performance. Understanding Data Cleaning and Its Benefits Data cleaning involves identifying and rectifying data errors, inconsistencies, and inaccuracies. The benefits include: Improved Data Accuracy: By eliminating errors and inconsistencies, data cleaning ensures that the dataset accurately represents real-world phenomena, leading to more reliable model outcomes. Increased Confidence in Model Results: A cleaned dataset instills confidence in the reliability of model predictions and outputs. Better Decision-Making Based on Reliable Data: Organizations can make better-informed decisions to build more reliable AI. Read How to Clean Data for Computer Vision to learn more about it. Selecting the right tool is essential for data cleaning tasks. In the next section, you will see criteria for selecting data cleaning tools to automate repetitive tasks and ensure thorough and efficient data cleansing. Selecting a Data Cleaning Tool Some criteria for selecting the right tools for data cleaning involve considering the following: Diversity in Functionality: Assess whether the tool specializes in handling specific data issues such as missing values or outlier detections. Understanding the strengths and weaknesses of each tool enables you to align them with the specific requirements of their datasets. Scalability and Performance: Analyzing the performance of tools in terms of processing speed and resource utilization helps in selecting tools that can handle the scale of the data at hand efficiently. User-Interface and Accessibility: Tools with intuitive interfaces and clear documentation streamline the process, reducing the learning curve. Compatibility and Integration: Compatibility with existing data processing pipelines and integration capabilities with popular programming languages and platforms are crucial. Seamless integration ensures a smooth workflow, minimizing disruptions during the data cleaning process. Once a suitable data cleaning tool is selected, understanding and implementing best practices for effective data cleaning becomes imperative. These practices ensure you can optimally leverage the tool you choose to achieve desired outcomes. Best Practices for Effective Data Cleaning Adhering to best practices is essential for ensuring the success of the data cleaning process. Some key practices include: Data Profiling: Understand the characteristics and structure of the data before initiating the cleaning process. Remove Duplicate and Irrelevant Data: Identify and eliminate duplicate or irrelevant images/videos to ensure data consistency and improve model training efficiency. Anomaly Detection: Utilize anomaly detection techniques to identify outliers or anomalies in image/video data, which may indicate data collection or processing errors. Documentation: Maintain detailed documentation of the cleaning process, including the steps taken and the rationale behind each decision. Iterative Process: Treat data cleaning as an iterative process, revisiting and refining as needed to achieve the desired data quality. For more information, read Mastering Data Cleaning & Data Preprocessing. Overcoming Challenges in Image and Video Data Cleaning Cleaning image and video data presents unique challenges compared to tabular data. Issues such as noise, artifacts, and varying resolutions require specialized techniques. These challenges need to be addressed using specialized tools and methodologies to ensure the accuracy and reliability of the analyses. Visual Inspection Tools: Visual data often contains artifacts, noise, and anomalies that may not be immediately apparent in raw datasets. Utilizing tools that enable visual inspection is essential. Platforms allowing users to view images or video frames alongside metadata provide a holistic understanding of the data. Metric-Based Cleaning: Implementing quantitative metrics is equally vital for effective data cleaning. You can use metrics such as image sharpness, color distribution, blur, changing your image backdrop, and object recognition accuracy to identify and address issues. Tools that integrate these metrics into the cleaning process automate the identification of outliers and abnormalities, facilitating a more objective approach to data cleaning. Using tools and libraries streamlines the cleaning process and contributes to improved insights and decision-making based on high-quality visual data. Watch the webinar From Data to Diamonds: Unearth the True Value of Quality Data to learn how tools help. Using Encord Active to Clean the Data Let’s take an example of the COCO 2017 dataset imported to Encord Active. Upon analyzing the dataset, Encord Active highlights both severe and moderate outliers. While outliers bear significance, maintaining a balance is crucial. Using Filter, Encord Active empowers users to visually inspect outliers and make informed decisions regarding their inclusion in the dataset. Taking the Area metric as an example, it reveals numerous severe outliers. We identify 46 low-resolution images with filtering, potentially hindering effective training for object detection. Consequently, we can select the dataset, click Add to Collection, remove these images from the dataset, or export them for cleaning with a data preprocessing tool. Encord Active facilitates visual and analytical inspection, allowing users to detect datasets for optimal preprocessing. This iterative process ensures the data is of good quality for the model training stage and improves performance on computer vision tasks. Watch the webinar Big Data to Smart Data Webinar: How to Clean and Curate Your Visual Datasets for AI Development to learn how to use tools to efficiently curate your data.. Case Studies: Optimizing Data Cleaning for Self-Driving Cars with Encord Active Encord Active (EA) streamlines the data cleaning process for computer vision projects by providing quality metrics and visual inspection capabilities. In a practical use case involving managing and curating data for self-driving cars, Alex, a DataOps manager at self-dr-AI-ving, uses Encord Active's features, such as bulk classification, to identify and curate low-quality annotations. These functionalities significantly improve the data curation process. The initial setup involves importing images into Active, where the magic begins. Alex organizes data into collections, an example being the "RoadSigns" Collection, designed explicitly for annotating road signs. Alex then bulk-finds traffic sign images using the embeddings and similarity search. Alex then clicks Add to a Collection, then Existing Collection, and adds the images to the RoadSigns Collection. Alex categorizes the annotations for road signs into good and bad quality, anticipating future actions like labeling or augmentation. Alex sends the Collection of low-quality images to a new project in Encord Annotate to re-label the images. After completing the annotation, Alex syncs the Project data with Active. He heads back to the dashboard and uses the model prediction analytics to gain insights into the quality of annotations. Encord Active's integration and efficient workflows empower Alex to focus on strategic tasks, providing the self-driving team with a streamlined and improved data cleaning process that ensures the highest data quality standards. Data Preprocessing What is Data Preprocessing? Data preprocessing transforms raw data into a format suitable for analysis. In computer vision, this process involves cleaning, organizing, and using feature engineering to extract meaningful information or features. Feature engineering helps algorithms better understand and represent the underlying patterns in visual data. Data preprocessing addresses missing values, outliers, and inconsistencies, ensuring that the image or video data is conducive to accurate analyses and optimal model training. Data Cleaning Vs. Data Preprocessing: The Difference Data cleaning involves identifying and addressing issues in the raw visual data, such as removing noise, handling corrupt images, or correcting image errors. This step ensures the data is accurate and suitable for further processing. Data preprocessing includes a broader set of tasks beyond cleaning, encompassing operations like resizing images, normalizing pixel values, and augmenting data (e.g., rotating or flipping images). The goal is to prepare the data for the specific requirements of a computer vision model. Techniques for Robust Data Preprocessing Image Standardization: Adjusting images to a standardized size facilitates uniform processing. Cropping focuses on relevant regions of interest, eliminating unnecessary background noise. Normalization: Scaling pixel values to a consistent range (normalization) and ensuring a standardized distribution enhances model convergence during training. Data Augmentation: Introduces variations in training data, such as rotations, flips, and zooms, and enhances model robustness. Data augmentation helps prevent overfitting and improves the model's generalization to unseen data. Dealing with Missing Data: Addressing missing values in image datasets involves strategies like interpolating or generating synthetic data to maintain data integrity. Noise Reduction: Applying filters or algorithms to reduce image noise, such as blurring or denoising techniques, enhances the clarity of relevant information. Color Space Conversion: Converting images to different color spaces (e.g., RGB to grayscale) can simplify data representation and reduce computational complexity. Now that we've laid the groundwork with data preprocessing, let's explore how to further elevate model performance through data refinement. Enhancing Models with Data Refinement Unlike traditional model-centric approaches, data refinement represents a paradigm shift, emphasizing nuanced and effective data-centric strategies. This approach empowers practitioners to leverage the full potential of their models through informed data selection and precise labeling, fostering a continuous cycle of improvement. By emphasizing input data refinement, you can develop a dataset that optimally aligns with the model's capabilities and enhances its overall performance. Model-centric vs Data-centric Approaches Model-Centric Approach: Emphasizes refining algorithms and optimizing model architectures. This approach is advantageous in scenarios where computational enhancements can significantly boost performance. Data-Centric Approach: Prioritizes the quality and relevance of training data. It’s often more effective when data quality is the primary bottleneck in achieving higher model accuracy. The choice between these approaches often hinges on the specific challenges of a given task and the available resources for model development. Download the free whitepaper How to Adopt a Data-Centric AI to learn how to make your AI strategy data-centric and improve performance. Data Refinement Techniques: Active Learning and Semi-Supervised Learning Active Learning: It is a dynamic approach that involves iteratively selecting the most informative data points for labeling. For example, image recognition might prioritize images where the model's predictions are most uncertain. This method optimizes labeling efforts and enhances the model's learning efficiency. Semi-Supervised Learning: It tackles scenarios where acquiring labeled data is challenging. This technique combines labeled and unlabeled data for training, effectively harnessing the potential of a broader dataset. For instance, in a facial recognition task, a model can learn general features from a large pool of unlabeled faces and fine-tune its understanding with a smaller set of labeled data. With our focus on refining data for optimal model performance, let's now turn our attention to the task of identifying and addressing outliers to improve the quality of our training data. Improving Training Data with Outlier Detection Outlier detection is an important step in refining machine learning models. Outliers, or abnormal data points, have the potential to distort model performance, making their identification and management essential for accurate training. Understanding Outlier Detection Outliers, or anomalous data points, can significantly impact the performance and reliability of machine learning models. Identifying and handling outliers is crucial to ensuring the training data is representative and conducive to accurate model training. Outlier detection involves identifying data points that deviate significantly from the expected patterns within a dataset. These anomalies can arise due to errors in data collection, measurement inaccuracies, or genuine rare occurrences. For example, consider a scenario where an image dataset for facial recognition contains rare instances with extreme lighting conditions or highly distorted faces. Detecting and appropriately addressing these outliers becomes essential to maintaining the model's robustness and generalization capabilities. Implementing Outlier Detection with Encord Active The outlier detection feature in Encord Active is robust. It can find and label outliers using predefined metrics, custom metrics, label classes, and pre-calculated interquartile ranges. It’s a systematic approach to debugging your data. This feature identifies data points that deviate significantly from established norms. In a few easy steps, you can efficiently detect outliers: Accessing Data Quality Metrics: Navigate to the Analytics > Data tab within Encord Active. Quality metrics offer a comprehensive overview of your dataset. In a practical scenario, a data scientist working on traffic image analysis might use Encord Active to identify and examine atypical images, such as those with unusual lighting conditions or unexpected objects, ensuring these don’t skew the model’s understanding of standard traffic scenes. Read the blog Improving Training Data with Outlier Detection to learn how to use Encord Active for efficient outlier detection. Understanding and Identifying Imbalanced Data Addressing imbalanced data is crucial for developing accurate and unbiased machine learning models. An imbalance in class distribution can lead to models that are skewed towards the majority class, resulting in poor performance in minority classes. Strategies for Achieving Balanced Datasets Resampling Techniques: Techniques like SMOTE for oversampling minority classes or Tomek Links for undersampling majority classes can help achieve balance. Synthetic Data Generation: Using data augmentation or synthetic data generation (e.g., GANs, generative models) to create additional examples for minority classes. Ensemble Methods: Implement ensemble methods that assign different class weights, enabling the model to focus on minority classes during training. Cost-Sensitive Learning: Adjust the misclassification cost associated with minority and majority classes to emphasize the significance of correct predictions for the minority class. When thoughtfully applied, these strategies create balanced datasets, mitigate bias, and ensure models generalize well across all classes. Balancing Datasets Using Encord Active Encord Active can address imbalanced datasets for a fair representation of classes. Its features facilitate an intuitive exploration of class distributions to identify and rectify imbalances. Its functionalities enable class distribution analysis. Automated analysis of class distributions helps you quickly identify imbalance issues based on pre-defined or custom data quality metrics. For instance, in a facial recognition project, you could use Encord Active to analyze the distribution of different demographic groups within the dataset (custom metric). Based on this analysis, apply appropriate resampling or synthetic data generation techniques to ensure a fair representation of all groups. Understanding Data Drift in Machine Learning Models What is Data Drift? Data drift is the change in statistical properties of the data over time, which can degrade a machine learning model's performance. Data drift includes changes in user behavior, environmental changes, or alterations in data collection processes. Detecting and addressing data drift is essential to maintaining a model's accuracy and reliability. Strategies for Detecting and Addressing Data Drift Monitoring Key Metrics: Regularly monitor key performance metrics of your machine learning model. Sudden changes or degradation in metrics such as accuracy, precision, or recall may indicate potential data drift. Using Drift Detection Tools: Tools that utilize statistical methods or ML algorithms to compare current data with training data effectively identify drifts. Retraining Models: Implement a proactive retraining strategy. Periodically update your model using recent and relevant data to ensure it adapts to evolving patterns and maintains accuracy. Continuous Monitoring and Data Feedback: Establish a continuous monitoring and adaptation system. Regularly validate the model against new data and adjust its parameters or retrain it as needed to counteract the effects of data drift. Practical Implementation and Challenges Imagine an e-commerce platform that utilizes a computer vision-based recommendation system to suggest products based on visual attributes. This system relies on constantly evolving image data for products and user interaction patterns. Identifying and addressing data drift Monitoring User Interaction with Image Data: Regularly analyzing how users interact with product images can indicate shifts in preferences, such as changes in popular colors, styles, or features. Using Computer Vision Drift Detection Tools: Tools that analyze changes in image data distributions are employed. For example, a noticeable shift in the popularity of particular styles or colors in product images could signal a drift. Retraining the recommendation model Once a drift is detected, you must update the model to reflect current trends. This might involve retraining the model with recent images of products that have gained popularity or adjusting the weighting of visual features the model considers important. For instance, if users start showing a preference for brighter colors, the recommendation system is retrained to prioritize such products in its suggestions. The key is to establish a balance between responsiveness to drift and the practicalities of model maintenance. Read the blog How To Detect Data Drift on Datasets for more information. Next, let's delve into a practical approach to inspecting problematic images to identify and address potential data quality issues. Inspect the Problematic Images Encord Active provides a visual dataset overview, indicating duplicate, blurry, dark, and bright images. This accelerates identifying and inspecting problematic images for efficient data quality enhancement decisions. Use visual representations for quick identification and targeted resolution of issues within the dataset. Severe and Moderate Outliers In the Analytics section, you can distinguish between severe and moderate outliers in your image set, understand the degree of deviation from expected patterns, and address potential data quality concerns. For example, below is the dataset analysis of the COCO 2017 dataset. It shows the data outliers in each metric and their severity. Blurry Images in the Image Set The blurry images in the image set represent instances where the visual content lacks sharpness or clarity. These images may exhibit visual distortions or unfocused elements, potentially impacting the overall quality of the dataset. You can also use the filter to exclude blurry images and control the quantity of retained high-quality images in the dataset. Darkest Images in the Image Set The darkest images in the image set are those with the lowest overall brightness levels. Identifying and managing these images is essential to ensure optimal visibility and clarity within the dataset, particularly in scenarios where image brightness impacts the effectiveness of model training and performance analysis. Duplicate or Nearly Similar Images in the Set Duplicate or nearly similar images in the set are instances where multiple images exhibit substantial visual resemblance or share identical content. Identifying and managing these duplicates is important for maintaining dataset integrity, eliminating redundancy, and ensuring that the model is trained on diverse and representative data. Next Steps: Fixing Data Quality Issues Once you identify problematic images, the next steps involve strategic methods to enhance data quality. Encord Active provides versatile tools for targeted improvements: Re-Labeling Addressing labeling discrepancies is imperative for dataset accuracy. Use re-labeling to rectify errors and inconsistencies in low-quality annotation. Encord Active simplifies this process with its Collection feature, selecting images for easy organization and transfer back for re-labeling. This streamlined workflow enhances efficiency and accuracy in the data refinement process. Active Learning Leveraging active learning workflows to address data quality issues is a strategic move toward improving machine learning models. Active learning involves iteratively training a model on a subset of data it finds challenging or uncertain. This approach improves the model's understanding of complex patterns and improves predictions over time. In data quality, active learning allows the model to focus on areas where it exhibits uncertainty or potential errors, facilitating targeted adjustments and continuous improvement. Quality Assurance Integrate quality assurance into the data annotation workflow, whether manual or automated. Finding and fixing mistakes and inconsistencies in annotations is possible by using systematic validation procedures and automated checks. This ensures that the labeled datasets are high quality, which is important for training robust machine learning models.
Feb 03 2024
10 M
Top 6 Computer Vision Data Management Tools
Google recently released its latest virtual try-on computer vision (CV) model that lets you see how a clothing item will look on a particular model in different poses. While this is a single example of how CV is changing the retail industry, multiple applications exist where CV models are revolutionizing how humans interact with artificial intelligence (AI) systems. However, creating advanced CV applications requires training CV models on high-quality data, and maintaining such quality is challenging due to the ever-increasing data volume and variety. You need robust CV tools for scalable data management that let you quickly identify and fix issues before using the data for model development. This article explores: The significance and challenges of data management. The factors to consider when choosing an ideal CV data management tool. Top CV data management tools. What is Data Management? Data management involves ingesting, storing, and curating data to ensure users can access high-quality datasets for model training and validation. Data curation is a significant aspect of data management, which involves organizing and preprocessing raw data from different sources and maintaining transformed data to improve the quality of the data. With the rise of big data, data curation has become a vital element for boosting data quality. Properly curated datasets increase shareability because different team members can readily use them to develop and test models. It also helps improve data annotation quality by letting you develop robust labeling workflows that involve automated data pipelines and stringent review processes to identify and fix labeling errors. Data management ensures compliance with global data regulations such as the General Data Protection Regulation (GDPR) by implementing data security protocols and maintaining privacy guidelines to prevent users from exploiting Personally Identifiable Information (PII). Data Management Challenges While management is crucial for maintaining data integrity, it can be challenging to implement throughout the data lifecycle. Below are a few challenges you can face when dealing with large datasets. Data Security Maintaining data security is a significant challenge as data regulations increase and cyberattack risks become more prevalent. Implementing data room software can provide an additional layer of security and regulatory compliance, ensuring that sensitive data is managed and stored with the highest standards. The problem is more evident in CV models, which require training datasets containing images with sensitive information, such as facial features, vehicle registration numbers, personal video footage, etc. Even the slightest breach can cause a business to lose customers and pay hefty penalties. Mitigation strategies can involve vigorous data encryption, regular security audits with effective access management procedures, and ethical data handling practices. Data Annotation Labeling images and ensuring accuracy is tedious, as it can involve several human annotators manually tagging samples for model development. The process gets more difficult if you have different data types requiring expert supervision. A more cost-effective method for labeling images is to use novel learning algorithms, such as self-supervised learning frameworks, zero-shot models, and active learning techniques, with efficient review systems to automate and speed up the annotation workflow. Managing complex data ecosystems Most modern projects have data scattered across several platforms and have an on-premises, cloud-based, or hybrid infrastructure to collect, store, and manage information from multiple data sources. Ensuring integration between these platforms and compatibility with existing infrastructure is essential to minimizing disruptions to work routines and downtime. However, managing multiple systems is challenging since you must consider several factors, such as establishing common data standards, maintaining metadata, creating shared access repositories, hiring skilled staff, etc. Comprehensive data governance frameworks can be a significant help here. They involve data teams establishing automated data pipelines, access protocols, shared glossaries, guidelines for metadata management, and a collaborative culture to prevent data silos. Large Data Volume and Variety Data volume rapidly increases with new data types, such as point-cloud data from Light Detection and Ranging (LiDAR) and Digital Imaging and Communications in Medicine (DICOM) within computer vision. This raises management-related issues, as engineers require effective strategies to analyze these datasets for model optimization. Efficient tools to handle various data types and storage platforms for real-time data collection can help deal with this issue. Learn how you can use data curation in CV to address data management challenges by reading Data Curation in Computer Vision Factors for Selecting the Right Computer Vision Data Management Tool A recurring mitigation strategy highlighted above is using the right data management and visualization tools. Below are a few factors you should consider before choosing a suitable tool. User experience: Seek intuitive but also customizable tools, with collaborative features and comprehensive support services to ensure your team can use them effectively. Integration: Ensure the tool can integrate smoothly with your existing tech stack, supported by reliable data integration systems offering APIs and compatibility with various data formats to minimize disruptions and maintain workflow efficiency. Searchability: A tool with robust search capabilities, including AI-enhanced features, indexing, and diverse filter options, will significantly streamline selecting and using data. Metadata management: Metadata helps provide important information about the dataset such as the source, location, and timestamp. Choose a tool that provides robust metadata management, offering features like version control, data lineage tracking, and automated metadata generation. Security: Opt for tools with robust encryption protocols (e.g., AES, SSL/TLS) and compliance with industry standards like ISO 27001 or SOC2 to safeguard your data. Pricing: Evaluate the tool's cost against its features, scalability, and potential long-term expenses, ensuring it fits your budget and provides a high return on investment (ROI). Top 6 Data Visualization and Management Tools Below is a list of the six best data curation tools for efficient data management and visualization, selected based on functionality, versatility, scalability, and price. Encord Encord is an end-to-end data platform that enables you to annotate, curate, and manage computer vision datasets through AI-assisted annotation features. It also provides intuitive dashboards to view insights on key metrics, such as label quality and annotator performance, to optimize workforce efficiency and ensure model excellence. Key Features User experience: It has a user-friendly interface (UI) that is easy to navigate. Scale: Explore and curate 10s of millions of images and videos in the same folder. Integration: Features an SDK, API, and pre-built integrations that let you customize data pipelines. Multimodal Search: Encord supports natural language search, external image, and similarity search to find desired visual assets quickly. Metadata management: Manage all your metadata in one place with simple metadata import and schemas. Custom & Pre-computed Embeddings: Explorer your data with Encords or your own embeddings using various embeddings-based features. Security: Encord is SOC 2 and GDPR compliant. Additional Features Data Management and Curation: Encord Index offers tools for compiling raw data into curated datasets, organizing datasets into folders, and sending datasets for labeling. It includes capabilities to visualize, search, sort, and control data efficiently. Annotation types: Encord Annotate supports label editors and multiple data labeling methods for CV, such as polygons, keypoint selection, frame classifications, polylines, and hanging protocols. Active learning workflows: Encord provides features to create active learning workflows with Encord Active and Annotate. Model evaluation: It provides data-driven insights for model performance and label quality. Automated labeling: Encord gives you multiple distinct automated labeling techniques to help you create labels quickly and with little effort. Best For Teams that wish for a scalable solution with features to streamline computer vision data management through automated labeling and easy-to-use UI. Price Simple volume-based pricing for teams and enterprises. Scenebox Scenebox is a platform that provides data management features to discover, curate, debug, visualize, secure, and synchronize multimodal data for CV models. Scenebox Key Features User experience: Scenebox has an easy-to-use UI for managing datasets. Integration: It integrates easily with open-source labeling tools for streamlining data annotation. Searchability: It lets you search data with any format and metadata schema using the Python client and the web app. Metadata management: The tool allows you to add metadata to image annotations. Additional Features Visualize embeddings: It lets you visualize image embeddings for data exploration. Model failure modes: The platform lets you identify labeling gaps by comparing predictions from other models. Best For Teams that deal with massive unstructured data in different formats. Pricing Pricing is not publicly available. Picsellia Picsellia is an AI-powered data management and data visualization platform with automated labeling functionality. Picsellia Key Features User interface: Picsellia has a user-friendly UI to upload, create, and visualize data. Searchability: It has an easy-to-use query-based search bar. Integration: Picsellia integrates with Azure, AWS, and Google Cloud. Metadata management: The tool offers pre-defined tags for creating metadata. Additional Features Custom query language: The platform has a visual search feature to find similar images. Versioning system: Its built-in versioning system keeps track of all historical datasets. Best For Teams that want a lightweight labeling and management tool for small-scale CV projects. Pricing Picsellia offers standard, business, and enterprise plans. DataLoop DataLoop is a data management tool with cloud storage integrations and a Python SDK for building end-to-end custom data preparation pipelines for data labeling and model training. DataLoop Key Features Data security: DataLoop is GDPR, SOC, and ISO 27001 certified. User interface: The tool has an intuitive user interface. Searchability: The UI features a data browser for searching datasets. Integration: It integrates with cloud platforms like AWS and Azure. Metadata management: DataLoop lets you add metadata using the DataLoop Query Language. Additional Features Support for multiple data formats: DataLoop supports several data types, including point-cloud data from LiDAR, audio, video, and text. Analytics dashboard: Features an analytics dashboard that shows real-time progress on annotation processes. Best For Teams that are looking for a high-speed and data-type-agnostic platform. Pricing Pricing is not publicly available. Tenyks Tenyks is an MLOps platform that helps you identify, visualize, and fix data quality issues by highlighting data gaps, such as outliers, noise, and class imbalances. Tenyks Key Features Data security: Tenyks is SOC 2-certified User interface: Tenyks has a user-friendly interface to set up your datasets. Searchability: The tool features a robust multi-modal search function. Additional Features Mine edge cases: It offers engaging visualizations to identify data failures and mine edge cases. Model comparison: It lets you compare multiple models across different data slices. Best For Teams that are looking for a quick solution to streamline data preprocessing. Pricing Tenyks offers a Free, Starter, Pro, and Enterprise plan. Scale Nucleus Nucleus by Scale is a management tool that lets you curate and visualize data while allowing you to collaborate with different team members through an intuitive interface. Nucleus Key Features Data security: Nucleus is SOC 2 and ISO 27001 certified. User interface: Nucleus has an easy-to-use interface that lets you visualize, curate, and annotate datasets. Natural language search: It features natural language search for easy image data discovery. Metadata management: It allows you to upload metadata as a dictionary for each dataset. Unique Features Find edge cases: The platform has tools to help you find edge cases. Model debugging: Nucleus also consists of model debugging features to reduce false positives. Best For Teams that want a solution for managing computer vision data for generative AI use cases. Pricing Nucleus offers a self-serve and enterprise version. Data Visualization and Management: Key Takeaways Data management is a critical strategic component for your company’s success. The following are a few crucial points you should remember. Importance of data management: Streamlined data management is key to efficient annotation, avoiding data silos, ensuring compliance, and ultimately leading to faster and more reliable decisions. Data curation: A vital element of data management, data curation directly impacts the quality and accuracy of the insights drawn from it. Management challenges: Continuous monitoring and updating are required to ensure data security and integrity in an increasingly complex and evolving data ecosystem. Data curation tools: Choose robust, adaptable tools to meet these challenges, focusing on those that offer ongoing updates and support to keep pace with technological advancements and changing data needs.
Jan 31 2024
8 M
Structured Vs. Unstructured Data: What is the Difference?
Data, often called oil for its resource value, is crucial in machine learning (ML). Machine learning has evolved significantly since its inception in the 1940s thanks to contributions from pioneers like Turing and McCarthy and developments in neural networks and algorithms. This evolution underscores the transition of data from mere information to a driver of growth and innovation. Data can be categorized into structured and unstructured types. Structured data is organized in databases, making it easily searchable. It is also ideal for quantitative analysis due to its organization. This type includes data in rows and columns, such as financial records in spreadsheets or customer information in CRM systems. In contrast, unstructured data forms the bulk of today's data generation and is not confined to a specific format. This includes different forms like images, videos, text, and audio files. They provide valuable insights but also pose analytical challenges. Unstructured data is complex with diverse data structures. It requires advanced AI and ML technologies for effective processing. Understanding data types is crucial because it directly impacts the accuracy and effectiveness of machine learning models. Proper selection and processing of data types enable more precise algorithms and inform innovation and decision-making in AI applications. By the end of this article, readers will gain a comprehensive understanding of the differences between structured and unstructured data and how each type impacts the field of machine learning and data-driven decision-making. Structured Data What is Structured Data? Structured data is organized in a specific format, typically rows and columns, to facilitate processing and analysis by computer systems. This data type adheres to a clear structure defined by a schema or data model. Examples include numerical data, dates, and strings in relational databases like SQL. Structured data can be efficiently indexed and queried, making it ideal for various applications, from business intelligence to data analytics. Sources of Structured Data Structured data sources are diverse and include various systems and platforms where data is methodically organized. Key sources include: Relational Databases (RDBMS): Stores data in a structured format using tables. Examples include MySQL, PostgreSQL, and Oracle. They are widely used for managing large volumes of structured data in enterprises. Customer Relationship Management (CRM) Systems: These platforms manage customer data, interactions, and business information in a structured format, enabling businesses to track and analyze customer activities and trends like gym owners managing their customer data through gym CRM software Online Transaction Processing (OLTP) Systems: They manage transaction-oriented applications. OLTP systems are designed to process high volumes of transactions efficiently and typically structure the data to support quick, reliable transaction processing. Enterprise Resource Planning (ERP) Systems: ERP systems integrate various business processes and manage related datasets within an organization. They store and process the data in a structured format for functions like finance, HR, and supply chain management. Spreadsheets and CSV Files: Common in business and data analysis contexts, spreadsheets and CSV files structure data in rows and columns, making it easy to organize, store, and analyze information. Data Warehouses: These systems are used for reporting and analysis, acting as central repositories of integrated data from one or more sources. Data warehouses store structured data extracted from various operational systems and are used for creating analytical reports. APIs and Web Services: Many modern APIs and web services return data in a structured format, like JSON or XML, which can be easily parsed and integrated into various applications. Internet of Things (IoT) Devices: Many IoT devices generate and transmit data in a structured format, which can be used for monitoring, analysis, and decision-making in various applications, including smart homes, healthcare, and industrial automation. Types of Structured Data Structured data sources are vast, ranging from traditional databases to modern IoT devices, each playing a pivotal role in the data ecosystem. Use Cases of Structured Data SEO Tools: Web developers use structured data to enhance SEO. By embedding microdata tags into the HTML of a webpage, they provide search engines with more context, improving the page's visibility in search results. Machine Learning: Structured data is pivotal in training supervised machine learning algorithms. Its well-defined nature facilitates the creation of labeled datasets that guide machines to learn specific tasks. Data Management: In business intelligence, structured data is essential for managing core data like customer information, financial transactions, and login credentials. Tools like SQL databases, OLAP, and PostgreSQL are commonly employed. ETL Processes: In ETL (Extract, Transform, Load) processes, structured data is extracted from various sources, transformed for consistency, and loaded into a data warehouse for analysis. Advantages of Structured Data Accessibility and Manageability: The well-defined organization of structured data makes it easily accessible and manageable. It simplifies data storage, retrieval, and analysis, particularly for users with varying technical expertise. Data Analysis: Structured data allows for stable and reliable analytics workflows due to its standardized nature. This enables businesses to derive insights and make informed decisions more effectively. Support with Mature Tools: A wide array of mature tools and models are available to process structured data, making it easier for organizations to integrate it into their decision-making processes. Facilitates Data Democratization: The simplicity and accessibility of structured data empower an organization's broader range of professionals to leverage data for decision-making, promoting a data-informed culture. Limitations of Structured Data Limited Scope: Structured data accounts for about 20% of enterprise data, providing a narrow view of business functions. Relying solely on structured data means missing out on insights you could derive from unstructured data. Rigidity: Structured data is often rigid in its format, making it less flexible for various data manipulation and analysis techniques. This can be restrictive when diverse data needs arise. Cost Implications: Structured data is typically stored in relational databases or data warehouses, which can be more expensive than data lakes used for unstructured data storage. Disruption in Workflow: Changes in reporting or analytics requirements can disrupt existing ETL and data warehousing workflows due to the structured nature of the data. While structured data remains essential in many business applications due to its organized format and ease of use, it is necessary to consider its limitations and the potential benefits of integrating unstructured data into the data strategy. The balance between structured and unstructured data handling can provide more comprehensive insights for business growth and decision-making. Unstructured Data What is Unstructured Data? Unstructured data refers to information that does not have a predefined data model or schema. This data type is typically qualitative and includes various formats such as text, video, audio, images, and social media posts. Unlike structured data, which is easy to search and analyze in databases or spreadsheets, unstructured data is more challenging to process and research due to its lack of organization. For example, while the structure of web pages is defined in HTML code, the actual content, which can be text, images, or video, remains unstructured. Sources of Unstructured Data Web Pages: The internet is a vast source of unstructured data. Web pages contain diverse content like text, images, and unstructured videos. Open-Ended Survey Responses: Surveys with open-ended questions generate unstructured data through textual responses. This data provides more nuanced insights compared to structured, multiple-choice survey data. Images, Audio, and Video: Multimedia files are considered unstructured data. Technologies like speech-to-text and facial recognition software analyze these data types. Emails: Emails are a form of semi-structured data where the metadata (like sender, recipient, and date) is structured but the email content remains unstructured. An SPF record checker help companies ensure the authenticity of incoming emails, protecting against phishing attacks. Social Media and Customer Feedback: Social media posts, blogs, product reviews, and customer feedback generate a significant amount of unstructured data. This data includes customer preferences, market trends, and brand perception insights. Types of Unstructured Data Use Cases of Unstructured Data Social Media Monitoring: Social media platforms generate vast unstructured data through posts, comments, and interactions. Businesses utilize machine learning tools to analyze this data, gaining insights into brand perception, customer satisfaction, and market trends. Customer Feedback Analysis: Companies collect feedback from online reviews, surveys, and emails. Analyzing this unstructured data helps understand customer needs, preferences, and areas for improvement. Content Analysis of Webpages: The internet, with its myriad of webpages containing text, images, and videos, is a significant source of unstructured data. Businesses use this data for competitive analysis, market research, and understanding public sentiment. Analysis of Open-Ended Survey Responses: Surveys often include open-ended questions where respondents answer in their own words. Analyzing these responses uncovers nuanced insights that can guide business strategies and product development. Multimedia Analysis: The analysis of images, audio, and video files, though challenging, can reveal crucial information. Advancements in speech-to-text and image recognition make extracting and analyzing data from these sources easier. Advantages of Unstructured Data Unstructured data presents a vast and largely untapped resource for engineers seeking to extract valuable insights and drive innovation. Unlike structured data, which adheres to a predefined schema, unstructured data possesses inherent advantages that can unlock new possibilities across various disciplines. Richer Insights: Unstructured data captures the real-world nuance and complexity often missing in structured datasets. This includes text, audio, video, and images, allowing engineers to analyze human sentiment, behavior, and interactions in their natural forms. Increased Flexibility: Unstructured data's lack of rigid schema allows for greater flexibility and adaptability. ML and Data Engineers can explore diverse data sources without being constrained by predefined formats. Enhanced Innovation: Unstructured data fuels the engine of innovation by providing ML models with a broader and deeper understanding of the world around them. Scalability and Cost-Effectiveness: With the increasing affordability of data storage and processing technologies, handling vast amounts of unstructured data becomes more feasible. Competitive Advantage: In today's data-driven world, embracing the power of unstructured data is critical for gaining a competitive advantage. However, it's essential to acknowledge that unstructured data also presents inherent challenges despite its advantages. Limitations of Unstructured Data The inherent lack of structure in unstructured data presents several limitations that you must consider. Difficulty in Processing: Due to their diverse formats and need for standardized schema, analyzing unstructured data requires specialized tools and techniques such as Natural Language Processing (NLP) algorithms, text analytics software, and machine learning models. Data Bias: Unstructured data can be susceptible to biases inherent in its source or collection process. This can lead to accurate or misleading insights if addressed appropriately. Data Privacy and Security: Unstructured data often contains sensitive information that requires robust security measures to protect individual privacy. Data Quality Concerns: Unstructured data can be incomplete, noisy, and inconsistent, demanding significant effort to clean and prepare before you can analyze it effectively. Lack of Standardization: Unstandardized formats and structures in unstructured data present data integration and interoperability challenges. Despite these limitations, the potential benefits of unstructured data outweigh the challenges. By developing the necessary skills and expertise, you can effectively address the limitations and unlock the vast potential of this valuable resource, driving innovation and gaining a competitive edge in the data-driven world. Structured vs Unstructured Data Semi-Structured Data What is Semi-Structured Data? Semi-structured data is rapidly becoming ubiquitous across various industries, posing unique challenges and opportunities for data engineers. This section delves into the technical aspects of semi-structured data, exploring its characteristics, sources, and critical considerations for effective management and utilization. Traditional data storage methods, such as relational databases, rely on rigid schema structures. However, the increasing proliferation of diverse data sources, including sensor readings, social media posts, and weblogs, necessitates flexible approaches. Enter semi-structured data, characterized by its reliance on self-describing formats like JSON, XML, and YAML and lack of a predefined schema. Sources of Semi-Structured Data The requirement for semi-structured data stems from its inherent flexibility, making it ideal for capturing complex and evolving information. Key sources include: Web Applications: User interactions, log files, and API responses often utilize semi-structured formats for easy data exchange and representation. Internet of Things (IoT) Devices: Sensor data, device logs, and operational information are frequently represented in semi-structured formats for efficient transmission and analysis. Social Media Platforms: User posts, comments, and interactions generate vast amounts of semi-structured data valuable for social listening and sentiment analysis. Scientific Research: Experiment results, gene sequencing data, and scientific observations often utilize semi-structured formats for flexible data representation and analysis. Use Cases of Semi-Structured Data Real-time Analytics: Analyze real-time sensor data, social media feeds, and website traffic to make informed decisions and identify problems quickly. Fraud Detection: Spot fraudulent activity in financial transactions and online interactions by looking for patterns in semi-structured data. Customer Personalization: Make product recommendations and content more relevant for each user based on their preferences and behavior data. Log Analysis: Find the root causes of system errors and performance bottlenecks by analyzing log files in their native semi-structured formats. Scientific Research: Manage and analyze complex scientific data, like gene sequences, experimental results, and scientific observations, effectively using the flexibility of semi-structured formats. Advantages of Semi-Structured Data Flexible: Adapt your data model as needed without changing the schema. This lets you add new information and handle changes easily. Scalable: Efficiently store and process large datasets by eliminating unnecessary structure and overhead. Enables Deep Analysis: Capture the relationships and context within your data to gain deeper insights. Cost-Effective: Often cheaper to store and process than structured data. Limitations of Semi-Structured Data Complexity: You'll need specialized tools and techniques to handle and process semi-structured data. It doesn't have a standard format, so finding the right tools can be tricky. Data Quality: Semi-structured data can be inconsistent, missing, or noisy. You'll need to clean and process it before you can use it. Security and Privacy: Ensure you have robust security measures to protect sensitive information in your semi-structured data. Interoperability: Sharing data between different systems can be complex because of the need for standardized formats. Limited Tools and Techniques: There are fewer established tools and techniques for analyzing semi-structured data than structured data. You can unlock its vast potential by learning how to handle semi-structured data effectively and using the right tools. Structured Vs. Unstructured Data vs Semi-Structured Data I have outlined some key differentiating characteristics of the different data sources in the table below. Best Practices in Data Management Effective data management is the cornerstone of data-driven decision-making and AI success. By implementing the following best practices, you can establish a robust and efficient data management system that empowers them to leverage the full potential of their data: Process Mapping and Stakeholder Identification: Clearly define data workflows and identify all stakeholders involved in data creation, storage, and utilization. This transparency facilitates collaboration, ensures accountability, and prevents confusion. Data Ownership and Responsibility: Establish clear ownership for data quality and ensure accountability at every data lifecycle stage. This promotes consistent data management practices, reduces errors, and facilitates data reliability. Efficient Data Capture: Implement reliable mechanisms for capturing relevant data accurately and comprehensively. This might involve utilizing scraping techniques, web scraping APIs, or sensor data collection tools tailored to the specific data source. Standardize Data Naming Conventions: Establish consistent naming conventions for data elements to increase data discoverability, accessibility, and analysis. Standardized names facilitate easier identification, retrieval, and manipulation of specific data points. Centralized Data Storage: Utilize a centralized data storage solution, such as a data lake or data warehouse, to enable efficient access, retrieval, and analysis of data from various sources. This centralized approach promotes data accessibility and allows for data aggregation and integration. Data Quality Management: Prioritize data quality by implementing data quality checks and cleansing processes. This ensures data accuracy, completeness, and consistency, reducing the risk of errors and misinterpretations in data analysis and decision-making. Robust Data Security: Implement robust data security measures to protect sensitive information and comply with regulatory requirements. This might involve data encryption, access controls, intrusion detection systems, and data security protocols tailored to the specific data types and organizational needs. Data-Driven Culture: Foster a data-driven culture within the organization. This involves providing engineers and other stakeholders access to relevant data and encouraging its use in problem-solving, strategic planning, and data-driven decision-making across all levels. Collaboration and Communication: Foster effective collaboration and communication between data engineers and stakeholders, such as business analysts and domain experts. This ensures data is collected, managed, and utilized in a way that aligns with business objectives and drives organizational success. Continuous Monitoring and Improvement: Regularly monitor data management processes and performance metrics. Analyze the collected data to identify areas for improvement and implement changes to optimize data management practices and ensure data accessibility, reliability, and security. By adopting these best practices, organizations can establish a data management system that empowers them to unlock the full potential of data for informed decision-making and innovative solutions, driving success and competitive advantage. Structured Vs. Unstructured Data: Key Takeaways In the ever-evolving data landscape, harnessing the potential of diverse data types necessitates a comprehensive approach to data management. By understanding the unique characteristics of structured, semi-structured, and unstructured data (quantitative, qualitative), organizations can leverage the strengths of each type and overcome inherent challenges. Utilizing APIs and choosing appropriate file formats (XML, CSV, JSON) ensures data accessibility and interoperability across different systems and applications, further enhancing data utilization. Adopting best practices, including utilizing cloud-based storage solutions and implementing efficient data pipelines (ETL), ensures scalability and the ability to handle increasing data volumes. Additionally, addressing data quality concerns through cleansing processes is crucial for reliable data-driven decisions that impact every aspect of an organization's operations (decision-making, scalability). Embracing a data-driven culture fosters collaboration and communication (APIs) across various teams, including data scientists and programmers using diverse programming languages. This collaborative approach unlocks the full potential of data, driving innovation and long-term success. Furthermore, adhering to ethical considerations in data collection and usage protects individual privacy rights, builds trust, and ensures responsible data management practices. Ultimately, organizations can unlock valuable insights, gain a competitive edge, and navigate the ever-changing, data-driven world by effectively managing and utilizing data in all its forms. By embracing the challenges and opportunities presented by different data types, organizations can position themselves for continued growth and success.
Dec 20 2023
8 M
Improving Training Data with Outlier Detection
In machine learning, training data plays a vital role in the accuracy and effectiveness of models. However, not all data is created equal, and the presence of outliers can significantly impact the performance of these models. In this blog post, we will explore the concept of outlier detection and how it can be leveraged to improve training data with Encord Active. What is Outlier Detection? Outlier detection refers to identifying data points that deviate significantly from the normal distribution of a dataset. Outliers can arise due to various factors such as measurement errors, data corruption, or anomalies in the data. Detecting and handling outliers is crucial as they can distort statistical analysis and affect the performance of your ML models. In data analysis and machine learning, you can encounter two types of outliers: Data outliers Label outliers Data Outliers Data outliers refer to observations or instances in a dataset that significantly deviate from the expected or typical values. These outliers arise due to measurement errors, data corruption, or anomalies. Data outliers can distort statistical analysis, affect the performance of machine learning models, and lead to inaccurate predictions. Detecting and addressing data outliers is crucial to ensure a high-quality dataset. Label Outliers Label outliers pertain to mislabeled or incorrectly assigned labels in a dataset. These outliers can occur due to human error during the labeling process or ambiguous instances that are challenging to classify accurately. Label outliers can substantially impact the performance of supervised learning algorithms by introducing noise and misguiding the training process. Identifying and rectifying label outliers is essential for training models with accurate ground truth and improving their predictive capabilities. Both data outliers and label outliers require careful analysis and handling to ensure the quality and reliability of data for your machine learning tasks. You must employ robust outlier detection techniques and quality assurance procedures to identify and address these outliers for more accurate and dependable models. Outlier Detection in Encord Active Encord Active offers a robust solution to identify and label outliers for pre-defined metrics, custom metrics, and label classes using precomputed interquartile ranges With this feature, you can easily spot data points that deviate significantly from the norm, enabling you to take appropriate actions and ensure data quality. By leveraging Interquartile ranges, Encord Active streamlines your outlier detection workflow, helping to debug your data. Setup To install Encord Active, follow these simple commands in your favorite Python environment: python3.9 -m venv ea-venv source ea-venv/bin/activate pip install encord-active 💡 Check out the documentation for installation for more information. Let's explore the steps in improving training data with outlier detection in Encord Active. Here we will be using the BDD dataset. Data Outliers Finding the Data Outliers Encord Active provides an intuitive interface to locate outliers in your dataset. Navigate to the Data Quality > Summary tab and access the Quality Metrics, which are presented as expandable panes. Click on a specific metric to reveal moderate to severe outliers, with the most severe ones displayed first, and use the slider to navigate through Tagging the Data Outliers Once you identify outliers of interest, Encord Active allows you to tag them individually or in bulk, to easily manage and work with them for further analysis. Acting on the Data Outliers After creating the tagged image group, access it conveniently in the Actions tab at the bottom of the left sidebar, with a range of actions at your disposal. Select "Filter data frame on" and choose the "tags" option to focus on the tagged outliers. You can then export the outliers, relabel them, augment the data, review them in detail, or even delete them from your dataset. Label Outliers Find Label Outliers To begin, navigate to the Label Quality > Summary tab in Encord Active. Here, you will find each Quality Metric presented as expandable panes, providing an overview of label quality. Click on a specific metric to gain deeper insights into moderate and severe label outliers. Like data outliers, the pane will prioritize presenting the severe outliers first, allowing you to focus on the most critical issues. Tag Label Outliers Once you have identified label outliers of interest, you can utilize individual and bulk tagging features to select and group the corresponding images. You can conveniently organize and manipulate these outliers for subsequent analysis and actions by tagging them. Access the tagged image group at the bottom of the left sidebar in the Actions tab. Act on Label Outliers Within the Actions tab, click "Filter data frame on" and select the "tags" option, allowing you to narrow the data frame to focus solely on the tagged label outliers. You can choose the desired actions from here, such as exporting the outliers, relabeling them, augmenting the dataset, reviewing them in detail, or even deleting them when necessary. How to Improve Training Data in Encord After reviewing the outlier detection procedure using Encord, let's examine its advantages for enhancing training data. Data Cleaning With its comprehensive set of tools for outlier detection, data visualization, and data quality assessment, Encord Active empowers users to identify problematic data points easily. You can efficiently detect and address problematic data points early in your machine learning pipeline by leveraging these features. This proactive approach ensures that you can identify and mitigate potential issues early on, leading to improved data quality and more reliable machine learning models. 💡Encord allows you to filter data in 3 ways: Standard filter feature, embeddings plot, and natural language search. Balancing Data Distribution The Actions tab in Encord Active offers two valuable options for refining your dataset: Filtering and creating a new dataset Balancing your existing dataset These options are accompanied by visualization capabilities, enabling you to make informed decisions about dataset quality without resorting to data augmentation or solely relying on the original dataset. By creating a small, balanced dataset through filtering, you can conduct thorough tests on your machine learning model. This approach helps you finalize your model in the pipeline, assess its performance, and determine if they require any further adjustments or augmentations. It also helps you evaluate different machine learning models. That enables you to make informed decisions about the most effective approach for your specific task. By leveraging the flexibility of the Actions tab in Encord Active and utilizing visualizations effectively, you can make data-driven choices regarding dataset balancing, augmentation, and model selection. This iterative process ensures that your training data is refined and optimized for the best possible model performance. 💡To know more about data balancing, read the blog Introduction to Balanced and Imbalanced Datasets in Machine Learning. Data Management By utilizing Encord Index, a product of Encord, for data curation and management, teams can ensure datasets are clean, well-organized, and relevant to the training task. This includes maintaining data versioning to track changes over time, implementing quality assurance measures to validate data integrity, and optimizing metadata management for efficient dataset retrieval and understanding. Such practices not only streamline the preparation of high-quality training datasets but also contribute to improved model performance by reducing noise, ensuring data consistency, and enhancing the overall reliability of the training process. Continuous Iteration and Feedback Continuous iteration and refinement of training data based on model performance and feedback are crucial for achieving optimal results. Regularly evaluating the model's accuracy, identifying areas for improvement, and updating the training data accordingly are essential steps in this process. Encord Active offers a range of tools to monitor model performance and assess the impact of data modifications, enabling informed decision-making. Model quality metrics provide a valuable means to evaluate data and labels using a trained model and imported predictions. By leveraging these metrics, you can gain insights into the strengths and weaknesses of your dataset, enabling targeted improvements. Encord Active also helps with data versioning, allowing you to compare and assess the model's performance on each version. This iterative approach helps identify the best version of the dataset that yields optimal model performance. By leveraging the feedback loop between model evaluation, dataset refinement, and performance assessment in Encord Active, you can continuously optimize your training data to improve model quality. Conclusion Improving training data quality is crucial for boosting the accuracy and effectiveness of machine learning models. Outlier detection, as explored in this blog post, plays a vital role in identifying and managing data outliers and label outliers. Encord Active offers robust outlier detection features that enable users to easily spot significant deviations in data. Encord Active streamlines the workflow by tagging and organizing outliers and ensuring data quality through its intuitive interface and comprehensive toolset. However, the benefits of Encord Active extend beyond outlier detection. The platform empowers users to perform data cleaning, balance data distribution, and iterate on the dataset based on model performance and feedback. With data visualization, dataset filtering, and model quality metrics, users can make data-driven decisions and continually optimize their training data. By proactively addressing problematic data points, balancing data distribution, and iterating on the dataset, users can enhance the quality and reliability of their machine learning models. Encord Active serves as a powerful platform for these tasks, enabling users to refine and optimize their training data to achieve optimal model performance. Are you ready to improve your training data with Encord Active? Sign-up for a free trial of Encord: The Data Engine for AI Model Development, used by the world’s pioneering computer vision teams. Follow us on Twitter and LinkedIn for more content on computer vision, training data, and active learning.
Jul 19 2023
5 M
How to Scale Your Data Labeling Operations
Data labeling operations are integral to the success of machine learning and computer vision projects. Data operation teams manage the entire end-to-end lifecycle of data labeling, including data sourcing, cleaning, and collaborating with ML teams to implement model training, quality assurance, and auditing workflows. The scalability of these teams is crucial. Behind the scenes, data operations teams ensure that artificial intelligence projects run smoothly. As computer vision, machine learning, and deep learning projects scale and data volumes expand, it is critical that data ops teams grow, streamline, and adapt to meet the challenge of handling more labeling tasks. In this article, we will cover 6 steps that data operations managers need to take to scale their teams and operational practices. What is Data Labeling for Machine Learning and Computer Vision? Data labeling or data annotation ⏤ the two terms that are often used synonymously, ⏤ is the act of applying labels and annotations to unlabeled data for the purpose of machine learning algorithms. Labels can be applied to various types of data, including images, video, text, and voice. For the purpose of this article, we will focus on data labeling for computer vision use cases, in which labels are applied to images and videos to create high-quality training datasets for AI models. Data labeling tasks could be as simple as applying a bounding box or polygon annotation with “cat” label or as complicated as microcellular labels applied to segmentations of tumors for a healthcare computer vision project. Regardless of complexity, accuracy is essential in the labeling process to ensure high-quality training datasets and to optimize model performance. Data labeling can be time-consuming and expensive. As such, companies must weigh the advantages and disadvantages of outsourcing or hiring in-house. While outsourcing is often more cost-effective, it comes with quality control concerns and data security risks. And, while in-house teams are expensive, they guarantee higher labeling quality and real-time insight into team members labeling tasks. The quality of training data directly impacts the performance of machine learning algorithms.,, Ultimately, it comes down to the labeling quality, a responsibility entrusted to data labeling teams. High-quality data requires a quality-centric data operations process with systems and management that can handle large volumes of labeling tasks for images or videos. 💡Find out more with Encord’s guides on How to choose the best datasets for machine learning and How to choose the right data for computer vision projects. Challenges of Scaling Data Labeling Operations Data labeling is a time-consuming and resource-intensive function. Data ops team members have to account for and manage everything from sourcing data to data cleaning, building and maintaining a data pipeline, quality assurance, and training a model using training, validation, and test sets. Even with an automated data annotation tool, there is a lot for data ops managers to oversee. There are several challenges that data labeling teams face when scaling: Project resources: Scaling requires additional resources and funding. Determining the best allocation of both can be a challenge Hiring and training: Hiring and training new team members require time and resources to align with project requirements and data quality standards. This forces teams to consider the options of outsourcing or managing teams in-house? Quality control: As the volume of data increases, maintaining How do we maintain high-quality labels becomes challenging. Workflow and data security: As data labeling tasks increase, it can be challenging to maintain data security, compliance, and audit trails. Annotation software: As image and video volumes increase, it can be challenging to manage projects. It is imperative to use the right tools, as teams can often benefit from the automation of data labeling tasks. Let’s look at how to solve these challenges. 6 Best Practices to Implement Scalable Data Labeling Operations Data operations teams are crucial for supporting data scientists and engineers. Here are 6 best practices for managing and implementing data labeling operations at scale. 1. Design a workflow-centric process Designing workflow-centric processes is crucial for any AI project. Data ops managers need to establish the data labeling project’s processes and workflows by creating standard operating procedures. 💡For more information, read Best Practice Guide for Computer Vision Data Operations Teams The support of senior leadership is vital to obtain the resources and budget to grow the data ops team, use the right tools, and employ a workforce for data labeling that can handle the volume needed. 2. Select an effective workforce for data labeling To select the appropriate workforce for data labeling operations, there are three options available: an in-house team, outsourced labeling services, or a crowd-sourced labeling team. The choice depends on several factors: Data volume Specialist knowledge Data security Cost considerations Management In many cases, the benefits of using outsourced labeling service providers outweigh the associated risks and costs. In regulated sectors like healthcare, however, the use of in-house teams is often the only option given data security concerns and the highly specialized knowledge required. Crowdsourcing through platforms like Amazon Mechanical Turk (MTurk) and SageMaker Ground Truth is another viable option for computer vision projects. Proper systems and processes, including workforce and workflow management and annotator training, are essential to the success of crowdsourcing or outsourcing. 3. Automate the data labeling process Similar to the staffing question, there are three options for automating data labeling: in-house tools, open-source, or commercial annotation solutions such as Encord. Open-source data labeling tools are suitable for projects with limited funding, such as academia or research, or for when a small team is building an MVP (minimum viable product) version of an AI model. These tools, however, often don’t meet the requirements for large-scale commercial projects. Developing an in-house tool can be a time-consuming and costly endeavor, taking 9 to 18 months and involving significant R&D expenses. In contrast, an off-the-shelf labeling platform can be quickly implemented. While pricing is higher than open-source (usually free for basic versions), it is cheaper than building an in-house data labeling tool. With an AI-assisted labeling and annotation platform, such as Encord, data ops teams can manage and scale the annotation workflows. The right tool also provides quality control mechanisms and training data-fixing solutions. 4. Leverage software principles for DataOps Software development principles can be leveraged when scaling data labeling and training for a computer vision project. Since data engineers, scientists, and analysts often engage in code-intensive tasks, integrating practices like continuous integration and delivery (CI/CD) and version control into data ops workflows is logical and advantageous. 5. Implement quality assurance (QA) iterative workflows To ensure quality control and assurance at scale, it is crucial to establish a fast-moving and iterative process. One effective approach is to establish an active learning pipeline and dashboard. This allows data ops leaders to maintain tight control over quality at both a high-level and individual label level. 💡Here are our guides on 5 Ways to Improve The Quality of Data Labels and an Introduction to Quality Metrics in Computer Vision 6. Ensure transparency and audibility in the data and labeling pipeline Label transparency and audibility are essential throughout the data pipeline. A clear, user-logged, and timestamped audit trail is critical for projects in secure or regulated sectors like healthcare where FDA compliance is required. With new AI laws likely to come into force worldwide in the next few years, a data labeling audit trail could also become mandatory for commercial AI models in non-regulated industries. 💡 Find out more with our Best Practice Guide for Computer Vision Data Operations Teams Scaling Data Labeling Operations: Key Takeaways High-quality training datasets are essential for optimizing model performance. The function of data operations teams is to ensure the labeling quality and labeling workflow are smooth and frictionless. Follow these 6 best practices to scale your data operations properly: Design workflow-centric processes Select an effective workforce for data labeling Automate the data labeling process Leverage software principles for DataOps Implement QA iterative workflows Ensure transparency and audibility in the data and labeling pipeline With an AI-powered annotation platform, data ops managers can oversee complex workflows, make annotation more efficient, and achieve labeling quality and productivity targets. Are you ready to scale your data labeling operations and need a powerful AI-based software suite for computer vision projects? Sign-up for a free trial of Encord: The Data Engine for AI Model Development, used by the world’s pioneering computer vision teams. Follow us on Twitter and LinkedIn for more content on computer vision, training data, and active learning.
Jul 04 2023
4 M
How to build Semantic Visual Search with ChatGPT & CLIP
OpenAI’s ChatGPT and CLIP releases have revolutionised the ways in which organisations and individual contributors can ship features to their users. At Encord, we’ve focused on how the neural network (CLIP) and LLM (ChatGPT) can be combined to build an effective and powerful Semantic Visual Search. Frederik Hvilshøj, Lead ML Engineer with a PhD in Generative AI, joins Eric Landau, CEO and Co-Founder of Encord, to provide actionable insights into how to build this function from scratch. Here are the key resources from the webinar: Collaboration notebook used by Frederik CLIP [paper/repo] ChatGPT [product updates, documentation] Encord blog: Lessons Learned: Employing ChatGPT as an ML Engineer for a Day Encord blog: What is vector similarity search?
Jun 15 2023
60 M
How to use SAM to Automate Data Labeling in Encord
Meta recently released their new Visual Foundation Model (VFM) called the Segment Anything Model (SAM). An open-source VFM with powerful auto-segmentation workflows. Here’s our guide for how to use SAM to automate data labeling in Encord. As data ops, machine learning (ML), and annotation teams know, labeling training data from scratch is time intensive and often requires expert labeling teams and review stages. Manual data labeling can quickly become expensive, especially for teams still developing best practices or annotating large amounts of data. Efficiently speeding up the data labeling process can be a challenge; this is where automation comes in. Incorporating automation into your workflow is one of the most effective ways to produce high-quality data fast. If you want to learn about image segmentation, check out the full guide Recently, Meta released their new Visual Foundation Model (VFM) called the Segment Anything Model (SAM), an open-source VFM with incredible abilities to support auto-segmentation workflows. If you want to learn about SAM, please read our SAM explainer. First, let's discuss a brief overview of SAM's functionality. How does SAM work? SAM’s architecture is based on three main components: An image encoder (a masked auto-encoder and pre-trained vision transformer) A prompt encoder (sparse and dense masks) And a mask decoder The image encoder processes input images to extract features, while the prompt encoder encodes user-provided prompts. These prompts specify which objects to segment in the image. The mask decoder combines information from both encoders to generate pixel-level segmentation masks. This approach enables SAM to efficiently segment objects in images based on user instructions, making it adaptable for various segmentation tasks in computer vision. Given this new release, Encord is excited to introduce the first SAM-powered auto-segmentation tool to help teams generate high-quality segmentation masks in seconds. MetaAI’s SAM x Encord Annotate The integration of MetaAI's SAM with Encord Annotate provides users with a powerful tool for automating labeling tasks. By leveraging SAM's capabilities within the Encord platform, users can streamline the annotation process and achieve precise segmentations across different file formats. This integration enhances efficiency and accuracy in labeling workflows, empowering users to generate high-quality annotated datasets effortlessly. Create quality masks with our SAM-powered auto-segmentation tool Whether you're new to labeling data for your model or have several models in production, our new SAM-powered auto-segmentation tool can help you save time and streamline your labeling process. To maximize the benefits of this tool, follow these steps: Set up Annotation Project Setup your image annotation project by attaching your dataset and ontology. Activate SAM Click the icon within the Polygon or Bounding box class in the label editor to activate SAM. Alternatively, use the Shift + A keyboard shortcut to toggle SAM mode. Create Labels for Existing Instances Navigate to the desired frame. Click "Instantiate object" next to the instance, or press the instance's hotkey. Press Shift + A on your keyboard to enable SAM. Segment the Image with SAM Click the area on the image or frame to segment. A pop-up will indicate that auto-segmentation is running. Alternatively, click and drag your cursor to select the part of the image to segment. Include/Exclude Areas from Selection After the prediction, a part of the image or frame will be highlighted in blue. Left-click to add to the selected area or right-click to exclude parts. To restart, click Reset on the SAM tool pop-up. Confirm Label Once the correct section is highlighted, click "Apply Label" on the SAM pop-up or press Enter on your keyboard to confirm. The result will be a labeled area outlined based on the selection (bounding box or polygon shape). Integrate an AI-assisted Micro-model to make labeling even faster While AI-assisted tools such as SAM-powered auto-segmentation can be great for teams just getting started with data labeling, teams who follow more advanced techniques like pre-labeling can take automation to the next level with micro-models. By using your model or a Micro-model, pre-labels can significantly increase labeling efficiency. As the model improves with each iteration, labeling costs decrease, allowing teams to focus their manual labeling efforts on edge cases or areas where the model may not perform as well. This results in faster, less expensive labeling with improved model performance. Check out our case study to learn more about our pre-labeling workflow, powered by AI-assisted labeling, and how one of our customers increased their labeling efficiency by 37x using AI-assisted Micro-models. Try our auto-segmentation tool on an image labeling project or start using model-assisted labeling today. If you are interested in using SAM for your computer vision project and would like to fine-tune SAM, it's essential to carefully consider your specific task requirements and dataset characteristics. By fine-tuning SAM, you can tailor its performance to suit your project's needs, ensuring accurate and efficient segmentation of images for your application. Fine-tuning SAM allows you to leverage its promptability and adaptability to new image distributions, maximizing its effectiveness in addressing your unique computer vision challenges. Read the blog How To Fine-Tune Segment Anything for a detailed explanation with code. Key Takeaways Meta's Segment Anything Model (SAM) is a powerful and effective open-source Visual Foundation Model (VFM) that will make a positive difference to automated segmentation workflows for computer vision and ML projects. AI-assisted labeling can reduce labeling costs and improve with each iteration. Our SAM-powered auto-segmentation tool and AI-assisted labeling workflow are available to all customers. We're excited for our users to see how automation can significantly reduce costs and increase labeling efficiency. Ready to improve the performance and scale your data operations, labeling, and automated annotation? Sign-up for an Encord Free Trial: The Active Learning Platform for Computer Vision, used by the world’s leading computer vision teams. AI-assisted labeling, model training & diagnostics, find & fix dataset errors and biases, all in one collaborative active learning platform, to get to production AI faster. Try Encord for Free Today. Want to stay updated? Follow us on Twitter and LinkedIn for more content on computer vision, training data, and active learning. Join the Slack community to chat and connect. Segment Anything Model (SAM) in Encord Frequently Asked Questions (FAQs) What is a Segmentation Mask? A segmentation mask assigns a label to each pixel in an image, identifying objects or regions of interest. This is done by creating a binary image where object pixels are marked with 1, and the rest with 0. It's used in computer vision for object detection and image segmentation and for training machine learning models for image recognition. How Does the SAM-powered Auto-segmentation Work? Combining SAM with Encord Annotate offers users an auto-segmentation tool with powerful ontologies, an interactive editor, and media support. SAM can segment objects or images without prior exposure using basic keypoint info and a bounding box. Encord annotate utilizes SAM to annotate various media types such as images, videos, satellite data, and DICOM data. If you want to better understand SAM’s inner workings and importance, please refer to the SAM explainer. Can I use SAM in Encord for Bounding Boxes? Encord’s auto-segmentation feature supports various types of annotations such as bounding boxes, polyline annotation, and keypoint annotation. Additionally, Encord utilizes SAM for annotating images, videos, and specializes data types including satellite (SAR), DICOM, NIfTI, CT, X-ray, PET, ultrasound and MRI files. For more information on auto-segmentation for medical imaging computer vision models, please refer to the documentation. Can I Fine-tune SAM? The image encoder of SAM is a highly intricate architecture containing numerous parameters. To fine-tune the model, it is more practical to concentrate on the mask encoder instead, which is lightweight and, therefore simpler, quicker, and more efficient in terms of memory usage. Please read Encord’s tutorial on how to fine-tune Segment Anything. You can find the Colab Notebook with all the code you need to fine-tune SAM here. Keep reading if you want a fully working solution out of the box! Can I try SAM in Encord for free? Encord has integrated its powerful ontologies, interactive editor, and versatile data type support with SAM to enable segmentation of any type of data. SAM's auto-annotation capability can be utilized for this purpose. Encord offers a free trial that can be accessed by logging in to our platform or please contact our team to get yourself started 😀
May 03 2023
8 M
5 Strategies To Build Successful Data Labeling Operations
Data labeling operations are an essential component of training and building a computer vision model. Data operations are a function that oversees the full lifecycle of data labeling and annotation, from sourcing and cleaning through to training and making a model production-ready. Data scientists and machine learning engineers aren’t wizards. Getting computer vision projects production-ready involves a lot of hard work, and behind the scenes are tireless professionals known as data operations teams. Data operations, also known as data labeling operations teams, play a mission-critical role in implementing computer vision artificial intelligence projects. Especially when a project is data-centric. It’s important and helpful to have an automated, AI-backed labeling and annotation tool, but for a project to succeed, you also need a team and process to ensure the work goes smoothly. In other words, to ensure data labels and annotations are high-quality, a data labeling operations function is essential. In this article, we will cover: Why data labeling operations are crucial for any algorithmic learning project (e.g., CV, ML, AI, etc.)? What are the benefits of data labeling operations? Should you buy or build data labeling and annotation software? 5 strategies for creating successful data labeling operations Let’s dive in . . . Why do Computer Vision Projects Need Data Labeling? Data labeling, also known as data annotation, is a series of tasks that take raw, unlabeled data and apply annotations and labels to image or video-based datasets (or other sources of data) for computer vision and other algorithmic models. Quality and accuracy are crucial for computer vision projects. Inputting poor-quality, badly labeled, and annotated images or videos will generate inaccurate results. Data labeling can be implemented in a number of ways. If you’ve only got a small dataset, your annotation team might be able to manage using manual annotation. Going through each image or video frame one at a time. However, in most cases, it helps to have an automated data annotation tool and to establish automated workflows to accelerate the process and improve quality and accuracy. Why are Data Labeling Operations Mission-critical? An algorithmic model's performance is only as effective as the data it’s trained on. Dozens of sectors, including medical and healthcare, manufacturing, and satellite images for environmental and defense purposes, rely on high-quality, highly-accurate data labeling operations. Annotations and labels are how you show an algorithmic model, including computer vision models and what’s in images or videos. Algorithms can’t see. We have to show them. Labels and annotations are how humans train algorithms to see, understand, and contextualize the content/objects within images and videos. Data labeling operations make all of this possible. There’s a lot of work that goes into making data training and then production-ready, including data cleaning tasks, establishing and maintaining a data pipeline, quality control, and checking models for bias and error. It all starts with sourcing the data. Either this is proprietary or can come from open-source datasets. Here’s our guides for: How to choose the best datasets for machine learning. How to choose the right data for computer vision projects. What Are The Benefits of Data Labeling Operations? There are numerous benefits to having a highly-skilled and smoothly-ran data labeling operations team, such as: Improved accuracy and performance of machine learning and computer vision models, thanks to higher-quality training data going into them. Reduced time and cost of implementing full-cycle data labeling and annotations. Improved quality of training data, especially when data ops is responsible for quality control and iterative learning and applies automated tools using a supervised or semi-supervised approach. An effective data ops team ensures a smooth and unending flow of high-quality data, helping to train a computer vision model. With the right data ops team, you can make sure the machine learning ops (MLops) team is more effective, supporting model training to produce the desired outcomes. Buy vs. Build for Data Labeling Ops Tools? Whether to buy or build data labeling tools is a question many teams and project leaders consider. It might seem an advantage to develop your own data labeling and annotation software. However, the downsides are that it’s a massively expensive and time-consuming investment. Having developed in-house software, you will need engineers to maintain and update it. And what if you need new features? Your ability to scale and adapt is more restricted. There are open-source tools and lots of them. However, for commercial data ops teams, most don’t meet the right requirements. Compared to building your own solution, buying/signing-up for a commercial platform is several orders of magnitude more cost and time-effective. Plus, you can be up and running in a matter of days, even hours, compared to 9 to 18 months if you go the in-house route. For projects that need tools for specific use cases, such as collaborative annotating tooling for clinical data ops teams and radiologists, there are commercial platforms on the market tailored for numerous industries, including healthcare. 5 Strategies to Create Successful Data Labeling Operations Now let’s look at 5 strategies for creating successful data labeling operations. Understand the use case Before launching into a project, data ops and ML leaders need to understand the problems they’re trying to solve for the particular use case. It’s a helpful exercise to map out a series of questions and work with senior leadership to understand project objectives and the routes to achieving them successfully. Begin the process of establishing data operations by asking yourself the following questions: What are the project objects? How much data and what type of data does this project need? How accurate does the model have to be when it’s production-ready to achieve the objectives? How much time does the project have to achieve the goals? What outcomes are senior leadership expecting? Is the allocated budget and resources sufficient to produce the results senior leaders want? If not, how can we argue the business case to increase the budget if needed? What’s the best way to implement data labeling operations: In-house, outsourced, or crowd-sourced? Once you’ve worked through the answers to these questions, it’s time to get build a data labeling operations team, processes, and workflows. Document labeling workflows and create instructions Taking a data-centric approach to data ops means that you can treat datasets, including the labels and annotations, as part of your organization's and project's intellectual property (IP). Making it more important to document the entire process. Documenting labeling workflows means that you can create standard operating procedures (SOPs), making data ops more scalable. It’s also essential for safeguarding datasets from data theft and cyberattacks and maintaining a clearly auditable and compliant data pipeline. Designing operational workflows before a project starts is essential. Otherwise, you’re putting the entire project at risk once data starts flowing through the pipeline. Create clear processes. Get the tools you need, budget, senior leadership buy-in, and resources, including operating procedures, before the project starts. Plan for the long haul (make your ontology expandable) Whether the project involves video annotation or image annotation or you’re using an active learning pipeline to accelerate a model’s iterative learnings, it’s important to make your ontology expandable. Regardless of the project, use case, or sector, including whether you’re annotating medical image files, such as DICOM and NIfTI, an expandable ontology means it’s easier to scale. Getting the ontology and label structure right at the start is important. No matter which approaches you take to labeling tasks or whether you automate them, everything flows from the labels and ontology you create. Start small and iterate The best way to build a successful data labeling operations workflow is to start small, learn from small setbacks, iterate, and then scale. Otherwise, you risk trying to annotate and label too much data in one go. Annotators make mistakes, meaning there will be more errors to fix. It will take you more time to attempt to annotate and label a larger dataset, to begin with, than to start on a smaller scale. Once you’ve got everything running smoothly, including integrating the right labeling tools, then you can expand the operation. Use iterative feedback loops, implement quality assurance, and continuously improve Iterative feedback loops and quality assurance/control are an integral part of creating and implementing data operations. Labels need to be validated. You need to make sure annotation teams are applying them correctly. Monitor for errors, bias, and bugs in the model. It’s impossible to avoid errors, inaccuracies, poorly-labeled images or video frames, and bugs. With the right AI-powered, automated data labeling and annotation tool, you can reduce the number and impact of errors, inaccuracies, poorly-labeled images or video frames, and bugs in training data and production-ready datasets. Pick an automation tool that integrates into your quality control workflows to ensure bugs and errors are fixed faster. This way, you’ll have more time and cost-effective feedback loops, especially if you’ve deployed an automated data pipeline, active learning pipelines, or micro-models. Build More Streamlined and Effective Data Label Operations With Encord With Encord and Encord Active, automated tools used by world-leading AI teams, you can build data labeling operations more effectively, securely, and at scale. Encord was created to improve the efficiency of automated image and video data labeling for computer vision projects. Our solution also makes managing data ops and a team of annotators easier, more time, and cost-effective while reducing errors, bugs, and bias. Encord Active is an open-source active learning platform of automated tools for computer vision: in other words, it's a test suite for your labels, data, and models. With Encord, you can achieve production AI faster with ML-assisted labeling, training, and diagnostic tools to improve quality control, fix errors, and reduce dataset bias. Make data labeling more collaborative, faster, and easier to manage with an interactive dashboard and customizable annotation toolkits. Improve the quality of your computer vision datasets, and enhance model performance. Key Takeaways: How to build successful data labeling operations Building a successful data labeling operation is essential for the success of computer vision projects. It takes time, work, and resources. But once you’ve got the people, processes, and tools in place, you can take data operations to the next level and scale computer vision projects more effectively. Successful data operations need the following scalable processes: Project goals and objectives; Documented workflows and processes; An expandable ontology; Iterative feedback loops and quality assurance workflows; And the right tools to make everything run more smoothly, including automated, AI-based annotation and labeling. Ready to improve the performance and scale your data operations, labeling, and automated annotation? Sign-up for an Encord Free Trial: The Active Learning Platform for Computer Vision, used by the world’s leading computer vision teams. AI-assisted labeling, model training & diagnostics, find & fix dataset errors and biases, all in one collaborative active learning platform, to get to production AI faster. Try Encord for Free Today. Want to stay updated? Follow us on Twitter and LinkedIn for more content on computer vision, training data, and active learning. Join the Slack community to chat and connect.
Apr 28 2023
5 M
What is Data Labeling? The Ultimate Guide [2024]
Data labeling constitutes a cornerstone within the domain of machine learning, addressing a fundamental challenge in artificial intelligence: transforming raw data into a format intelligible to machines. At its core, data annotation solves the issue that unstructured information presents: machines struggle to comprehend the complexities of the real world because they lack human-like cognition. In this interplay between data and intelligence, data labeling assumes the role of an orchestrator, imbuing raw information with context and significance. This blog explains the importance, methodologies, and challenges associated with data labeling. Understanding Data Labeling In machine learning, data is the fuel that propels algorithms to decipher patterns, make predictions, and enhance decision-making processes. However, not all data is equal; the success of a machine learning project hinges on the meticulous process of data labeling, a task akin to providing a roadmap for machines to navigate the complexities of the real world. What is Data Labeling? Data labeling, often called data annotation, involves the meticulous tagging or marking of datasets. These annotations are the signposts that guide machine learning models during their training phase. As models learn from labeled data, the accuracy of these annotations directly influences the model's ability to make precise predictions and classifications. Significance of Data Labeling in Machine Learning Data annotation or tagging provides context for the data that machine learning algorithms can comprehend. The algorithms learn to recognize patterns and make predictions based on the labeled data. The significance of data labeling lies in its ability to enhance the learning process, enabling machines to generalize from labeled examples to make informed decisions on new, unlabeled data. Accurate and well-labeled data sets contribute to creating robust and reliable machine learning models. These models, whether for image recognition, natural language processing, or other applications, heavily rely on labeled data to understand and differentiate between various input patterns. The quality of data labeling directly impacts the model's performance, influencing its precision, recall, and overall predictive capabilities. In industries such as healthcare, finance, and autonomous vehicles, where the stakes are high, the precision of machine learning models is critical. Properly labeled data ensures that models can make informed decisions, improving efficiency and reducing errors. How does Data Labeling Work? Understanding the intricacies of how data labeling operates is fundamental to grasping its impact on machine learning models. This section discusses the mechanics of data labeling, distinguishing between labeled and unlabeled data, explaining data collection techniques, and shedding light on the tagging process. Labeled vs. Unlabeled Data In the dichotomy of supervised and unsupervised machine learning, the distinction lies in the presence or absence of labeled data. Supervised learning thrives on labeled data, where each example in the training set is coupled with a corresponding output label. This labeled data becomes the blueprint for the model, guiding it to learn the relationships and patterns necessary for accurate predictions. Conversely, unsupervised learning operates in the realm of unlabeled data. The algorithm navigates the dataset without predefined labels, seeking inherent patterns and structures. Unsupervised learning is a journey into the unknown, where the algorithm must uncover the latent relationships within the data without explicit guidance. Data Collection Techniques The process of data labeling begins with the acquisition of data, and the techniques employed for this purpose play a pivotal role in shaping the quality and diversity of the labeled dataset. Manual Data Collection One of the most traditional yet effective methods is manual data collection. Human annotators meticulously label data points based on their expertise, ensuring precision in the annotation process. While this method guarantees high-quality annotations, it can be time-consuming and resource-intensive. Open-Source Datasets In the era of collaborative knowledge-sharing, leveraging open-source datasets has become a popular approach. These datasets, labeled by a community of experts, provide a cost-effective means of accessing diverse and well-annotated data for training machine learning models. Synthetic Data Generation To address the challenge of limited real-world labeled data, synthetic data generation has gained prominence. This technique involves creating artificial data points that mimic real-world scenarios, augmenting the labeled dataset, and enhancing the model's ability to generalize to new, unseen examples. Data Tagging Process The data tagging process is a critical step that demands attention to detail and precision to ensure the resulting labeled dataset accurately represents the real-world scenarios the model is expected to encounter. Ensuring Data Security and Compliance With heightened data privacy concerns, ensuring the security and compliance of labeled data is non-negotiable. Implementing robust measures to safeguard sensitive information during the tagging process is imperative. Encryption, access controls, and adherence to data protection regulations are vital components of this security framework. Data Labeling Techniques Manual Labeling Process The manual labeling process involves human annotators meticulously assigning labels to data points. This method is characterized by its precision and attention to detail, ensuring high-quality annotations that capture the intricacies of real-world scenarios. Human annotation brings domain expertise into the labeling process, enabling nuanced distinctions that automated systems might struggle to discern. However, the manual process can be time-consuming and resource-intensive, necessitating robust quality control measures. Quality control is essential to identify and rectify any discrepancies in annotations, maintaining the accuracy of the labeled dataset. Establishing a ground truth, a reference point against which annotations are compared, is a key element in quality control, enabling the assessment of annotation consistency and accuracy. Semi-Supervised Labeling Semi-supervised labeling strikes a balance between labeled and unlabeled data, leveraging the strengths of both. Active learning, a technique within semi-supervised labeling, involves the model actively selecting the most informative data points for labeling. This iterative process optimizes the learning cycle, focusing on areas where the model exhibits uncertainty or requires additional information. Combination labeling, another facet of semi-supervised labeling, integrates labeled and unlabeled data to enhance model performance. Synthetic Data Labeling Synthetic data labeling involves creating artificial data points to supplement real-world labeled datasets. This technique addresses the challenge of limited labeled data by generating diverse examples that augment the model's understanding of various scenarios. While synthetic data is a valuable resource for training models, ensuring its relevance and compatibility with real-world data is crucial. Automated Data Labeling Automatic data labeling employs algorithms to assign labels to data points, streamlining the labeling process. This approach significantly reduces the manual effort required, making it efficient for large-scale labeling tasks. However, the success of automatic labeling hinges on the accuracy of the underlying algorithms, and quality control measures must be in place to rectify any mislabeling or inconsistencies. Check out the tutorial to learn more about How to Automate Data Labeling [Examples + Tutorial] Active Learning Active learning is a dynamic technique where the model actively selects the most informative data points for labeling. This iterative approach optimizes the learning process, directing attention to areas where the model's uncertainty prevails or additional information is essential. Active learning Active learning enhances efficiency by prioritizing the labeling of data that maximizes the model's understanding. Learn more about active learning in the video The Future of ML Teams: Embracing Active Learning Outsourcing Labeling Outsourcing data labeling to specialized service providers or crowdsourced platforms offers scalability and cost-effectiveness. This approach allows organizations to tap into a distributed workforce for annotating large volumes of data. While outsourcing enhances efficiency, maintaining quality control and ensuring consistency across annotators are critical challenges. Crowdsourced Labeling Crowdsourced labeling leverages the collective efforts of a distributed online workforce to annotate data. This decentralized approach provides scalability and diversity but demands meticulous management to address potential issues of label consistency and quality control. It takes careful planning to navigate the wide range of data labeling strategies while considering the project's needs, resources, and desired level of control. Achieving the ideal balance between automated efficiency and manual accuracy is essential to the success of the data labeling project. Types of Data Labeling Data labeling is flexible enough to accommodate the various requirements of machine learning applications. This section explores the various data labeling techniques tailored to specific domains and applications. Computer Vision Labeling Supervised learning Supervised learning forms the backbone of computer vision labeling. In this paradigm, models are trained on labeled datasets, where each image or video frame is paired with a corresponding label. This pairing enables the model to learn and generalize patterns, making accurate predictions on new, unseen data. Applications of supervised learning in computer vision include image classification, object detection, and facial recognition. Unsupervised learning In unsupervised learning for computer vision, models operate on unlabeled data, extracting patterns and structures without predefined labels. This exploratory approach is particularly useful for tasks that discover hidden relationships within the data. Unsupervised learning applications include clustering similar images, image segmentation, and anomaly detection. Semi-supervised learning Semi-supervised learning balances labeled and unlabeled data, offering the advantages of both approaches. Active learning, a technique within semi-supervised labeling, involves the model selecting the most informative data points for labeling. This iterative process optimizes learning by focusing on areas where the model exhibits uncertainty or requires additional information. Combination labeling integrates labeled and unlabeled data, enhancing model performance with a more extensive dataset. Human-in-the-Loop (HITL) Human-in-the-loop (HITL) labeling acknowledges the strengths of both machines and humans. While machines handle routine labeling tasks, humans intervene when complex or ambiguous scenarios require nuanced decision-making. This hybrid approach ensures the quality and relevance of labeled data, particularly when automated systems struggle. Programmatic data labeling Programmatic data labeling involves leveraging algorithms to automatically label data based on predefined rules or patterns. This automated approach streamlines the labeling process, making it efficient for large-scale datasets. However, it requires careful validation to ensure accuracy, as the success of programmatic labeling depends on the quality of the underlying algorithms. Natural Language Processing Labeling Named Entity Recognition (NER) Named Entity Recognition involves identifying and classifying entities within text, such as names of people, locations, organizations, dates, and more. NER is fundamental in extracting structured information from unstructured text, enabling machines to understand the context and relationships between entities. Sentiment analysis Sentiment Analysis aims to determine the emotional tone expressed in text, categorizing it as positive, negative, or neutral. This technique is crucial for customer feedback analysis, social media monitoring, and market research, providing valuable insights into user sentiments. Text classification Text Classification involves assigning predefined categories or labels to textual data. This technique is foundational for organizing and categorizing large volumes of text, facilitating automated sorting and information retrieval. It finds applications in spam detection, topic categorization, and content recommendation systems. Audio Processing Labeling Audio processing labeling involves annotating audio data to train models for speech recognition, audio event detection, and various other audio-based applications. Here are some key types of audio-processing labeling techniques: Speed data labeling Speech data labeling is fundamental for training models in speech recognition systems. This process involves transcribing spoken words or phrases into text and creating a labeled dataset that forms the basis for training accurate and efficient speech recognition models. High-quality speech data labeling ensures that models understand and transcribe diverse spoken language patterns. Audio event labeling Audio event labeling focuses on identifying and labeling specific events or sounds within audio recordings. This can include categorizing events such as footsteps, car horns, doorbell rings, or any other sound the model needs to recognize. This technique is valuable for surveillance, acoustic monitoring, and environmental sound analysis applications. Speaker diarization Speaker diarization involves labeling different speakers within an audio recording. This process segments the audio stream and assigns speaker labels to each segment, indicating when a particular speaker begins and ends. Speaker diarization is crucial for applications like meeting transcription, which helps distinguish between different speakers for a more accurate transcript. Language identification Language identification involves labeling audio data with the language spoken in each segment. This is particularly relevant in multilingual environments or applications where the model must adapt to different languages. Benefits of Data Labeling The process of assigning meaningful labels to data points brings forth a multitude of benefits, influencing the accuracy, usability, and overall quality of machine learning models. Here are the key advantages of data labeling: Precise Predictions Labeled datasets serve as the training ground for machine learning models, enabling them to learn and recognize patterns within the data. The precision of these patterns directly influences the model's ability to make accurate predictions on new, unseen data. Well-labeled datasets create models that can be generalized effectively, leading to more precise and reliable predictions. Improved Data Usability Well-organized and labeled datasets enhance the usability of data for machine learning tasks. Labels provide context and structure to raw data, facilitating efficient model training and ensuring the learned patterns are relevant and applicable. Improved data usability streamlines the machine learning pipeline, from data preprocessing to model deployment. Enhanced Model Quality The quality of labeled data directly impacts the quality of machine learning models. High-quality labels, representing accurate and meaningful annotations, contribute to creating robust and reliable models. Models trained on well-labeled datasets exhibit improved performance and are better equipped to handle real-world scenarios. Use Cases and Applications As discussed before, for many machine learning applications, data labeling is the foundation that enables models to traverse and make informed decisions in various domains. Data points can be strategically annotated to facilitate the creation of intelligent systems that can respond to particular requirements and problems. The following are well-known use cases and applications where data labeling is essential: Image Labeling Image labeling is essential for training models to recognize and classify objects within images. This is instrumental in applications such as autonomous vehicles, where identifying pedestrians, vehicles, and road signs is critical for safe navigation. Text Annotation Text annotation involves labeling textual data to enable machines to understand language nuances. It is foundational for applications like sentiment analysis in customer feedback, named entity recognition in text, and text classification for categorizing documents. Video Data Annotation Video data annotation facilitates the labeling of objects, actions, or events within video sequences. This is vital for applications such as video surveillance, where models need to detect and track objects or recognize specific activities. Speech Data Labeling Speech data labeling involves transcribing spoken words or phrases into text. This labeled data is crucial for training accurate speech recognition models, enabling voice assistants, and enhancing transcription services. Medical Data Labeling Medical data labeling is essential for tasks such as annotating medical images, supporting diagnostic processes, and processing patient records. Labeled medical data contributes to advancements in healthcare AI applications. Challenges in Data Labeling While data labeling is a fundamental step in developing robust machine learning models, it comes with its challenges. Navigating these challenges is crucial for ensuring the quality, accuracy, and fairness of labeled datasets. Here are the key challenges in the data labeling process: Domain Expertise Ensuring annotators possess domain expertise in specialized fields such as healthcare, finance, or scientific research can be challenging. Lacking domain knowledge may lead to inaccurate annotations, impacting the model's performance in real-world scenarios. Resource Constraint Data labeling, especially for large-scale projects, can be resource-intensive. Acquiring and managing a skilled labeling workforce and the necessary infrastructure can pose challenges, leading to potential delays in project timelines. Label Inconsistency Maintaining consistency across labels, particularly in collaborative or crowdsourced labeling efforts, is a common challenge. Inconsistent labeling can introduce noise into the dataset, affecting the model's ability to generalize accurately. Labeling Bias Bias in labeling, whether intentional or unintentional, can lead to skewed models that may not generalize well to diverse datasets. Overcoming labeling bias is crucial for building fair and unbiased machine learning systems. Data Quality The quality of labeled data directly influences model outcomes. Ensuring that labels accurately represent real-world scenarios, and addressing issues such as outliers and mislabeling, is essential for model reliability. Data Security Protecting sensitive information during the labeling process is imperative to prevent privacy breaches. Implementing robust measures, including encryption, access controls, and adherence to data protection regulations, is vital for maintaining data security. Overcoming these challenges requires a strategic and thoughtful approach to data labeling. Implementing best practices, utilizing advanced tools and technologies, and fostering a collaborative environment between domain experts and annotators are key strategies to address these challenges effectively. Best Practices in Data Labeling Data labeling is critical to developing robust machine learning models. Your practices during this phase significantly impact the model's quality and efficacy. A key success factor is the choice of an annotation platform, particularly one with intuitive interfaces. These platforms enhance accuracy, efficiency, and the user experience in data labeling. Intuitive Interfaces for Labelers Providing labelers with intuitive and user-friendly interfaces is essential for efficient and accurate data labeling. Such interfaces reduce the likelihood of labeling errors, streamline the process, and improve the data annotation experience of users. Key features like clear instructions with ontologies, customizable workflows, and visual aids are integral to an intuitive interface. For instance, Treeconomy's use of Encord for tree counting illustrates how a user-friendly interface can facilitate efficient labeling and integrate well with existing systems. Read more about it in the case study Accurately Measuring Carbon Content in Forests Label Auditing Regularly validating labeled datasets is crucial for identifying and rectifying errors. It involves reviewing the labeled data to detect inconsistencies, inaccuracies, or potential biases. Auditing ensures that the labeled dataset is reliable and aligns with the intended objectives of the machine learning project. A robust label auditing practice should possess: Quality metrics: To swiftly scan large datasets for errors. Customization options: Tailor assessments to specific project requirements. Traceability features: Track changes for transparency and accountability. Integration with workflows: Seamless integration for a smooth auditing process. Annotator management: Intuitive to manage and guide the annotators to rectify the errors These attributes are features to look for in a label auditing tool. This process can be an invaluable asset in maintaining data integrity. Tractable's adoption of a QA and performance monitoring platform exemplifies how systematic auditing can maintain data integrity, especially in large, remote teams. See how they do it in this case study. Active Learning Approaches Active learning approaches, supported by intuitive platforms, improve data labeling efficiency. These approaches enable dynamic interaction between annotators and models. Unlike traditional methods, this strategy prioritizes labeling instances where the model is uncertain, optimizing human effort for challenging data points. This symbiotic interaction enhances efficiency, directing resources to refine the model's understanding in its weakest areas. Also, the iterative nature of active learning ensures continuous improvement, making the machine learning system progressively adept at handling diverse and complex datasets. This approach maximizes human annotator expertise and contributes to a more efficient, precise, and adaptive data labeling process. Quality Control Measures With Encord Encord stands out as a comprehensive solution, offering a suite of quality control measures designed to optimize every facet of the data labeling process. Here are a few quality measures: Active Learning Optimization Ensuring optimal model performance and facilitating iterative learning are paramount in machine learning projects. Encord's quality control measures include active learning optimization, a dynamic feature ensuring optimal model performance, and iterative learning. By dynamically identifying uncertain or challenging instances, the platform directs annotators to focus on specific data points, optimizing the learning process and enhancing model efficiency. Addressing Annotation Consistency Encord recognizes that consistency in annotations is paramount for high-quality labeled datasets. Addressing this, the platform meticulously labels data, have workflows to review the labels, and use label quality metrics for error identification. With a dedicated development team focus on minimizing labeling errors, Encord ensures annotations are reliable, delivering labeled data that is precisely aligned with project objectives. Ensuring Data Accuracy Validation and data quality assurance are the cornerstones of Encord's quality control framework. By implementing diverse data quality metrics and ontologies, our platform executes robust validation processes, safeguarding the accuracy of labeled data. This commitment ensures consistency and the highest standards of precision, fortifying the reliability of machine learning models.
Apr 14 2023
8 M
The Full Guide to Automated Data Annotation
Automated data annotation is a way to use AI-assisted tools and software to accelerate and improve the quality of creating and applying labels to images and videos for computer vision models. Automated data annotations and labels greatly impact the accuracy, outputs, and results that algorithmic models generate. Artificial intelligence (AI), computer vision (CV), and machine learning (ML) models require high-quality and large quantities of annotated data, and the most cost-effective and time-effective way of delivering that is through automation. Automated data annotation and labeling, normally using AI-based tools and software, makes a project run much smoother and faster. Compared to manual data labeling, automation can take manual, human-produced labels and apply them across vast datasets. This guide covers everything from the different types of automated data labeling, use cases, best practices, and how to implement automated data annotation more effectively with tools such as Encord. What is Data Annotation? Data annotation (or data labeling) is the task of labeling objects for machine learning algorithms in datasets, such as images or videos. As we focus on automation, AI-supported data labeling, and annotation for computer vision (CV) models, we will cover image and video-based use cases in this article. However, you can use automated data annotation and labeling for any ML project, such as audio and text files for natural language processing (NLP), conversational AI, voice recognition, and transcription. Data annotation maps the objects in images or videos against what you want to show in a CV model. Annotations and labels describe the objects in a dataset, including contextual information. Every label and annotation applied to a dataset should be aligned with a project's outcome, goals, and objectives. ML and CV models are widely used in dozens of sectors, with hundreds of use cases, including medical and healthcare, manufacturing, and satellite images for environmental and defense purposes. Labels are an integral part of the data that an algorithmic model learns. Quality and accuracy are crucial. If you put poor-quality data in, you’ll get inaccurate results. There are several ways to implement automated data annotation, including supervised, semi-supervised, in-house, and outsourcing. See Also: What is Data Labeling: The Full Guide. Now, let’s dive into how annotation, ML, and data ops teams can automate data annotation for computer vision projects. How to Automate Data Annotation Manual tasks, including data cleaning, annotation, and labeling, are the most time-consuming part of any computer vision project. According to Cognilytica, preparation absorbs 80% of the time allocated for most CV projects, with annotation and labeling consuming 25%. Automating data annotation tasks with AI-based tools and software greatly affects the time it takes to get a model production-ready. AI-supported data labeling is quicker, more efficient, cost-effective, and reduces manual human errors. However, picking the right AI-based tools is essential. As ML engineers and data ops leaders know, dozens of options are available, such as open-source, low-code and no-code, and active learning annotation solutions, toolkits, and dashboards, including Encord. There are also several ways you can implement automated data annotation to create the training data you need, such as: Supervised learning; Unsupervised learning; Semi-supervised learning; Human-in-the-Loop (HITL); Programmatic data labeling. We compare those in this article in more detail. Now, let’s consider one of the most important questions many ML and data ops leaders need to review before they start automating data annotation: “Should we build our own tool or buy?” Build vs. Buy Automated Data Annotation Tools Building an in-house tool takes 6 to 18 months ⏤ and usually costs anywhere in the 6 to 7-figure range. Even if you outsource the development work, it’s a resource-hungry project. Plus, you’ve got to factor in things like, “What if we need new features/updates?”, maintenance, and integration with up and downstream tools like those for managing data and evaluating models. The number of features and tools you’ll need correlates to the volume of data a tool will process, the number of annotators, and how many projects an AI-based tool will handle in the months and years ahead. On the other hand, buying an out-of-the-box solution means you could be up and running in hours or days rather than 6 to 18 months. In almost every case, it’s simply more time- and cost-effective. Plus, you can select a tool based on your use case and data annotation and labeling needs rather than any limitations of in-house engineering resources. Recommended: Buy vs Build for computer vision data annotation - what's better? Different Types of Automated Data Annotation in Computer Vision Computer vision uses machine learning models to extract commercial and real-world outputs and insights from image and video-based datasets. Some of the most common automated data annotation tasks in computer vision include: Image annotation; Video annotation; DICOM and medical image or video annotation. Let’s explore all three in more detail. Image Annotation Image annotation is an integral part of any image-based computer vision model. Especially when taking the data-centric AI approach or using an active learning pipeline to accelerate a model’s iterative learning. Although not as complex as video annotation, applying labels to images is more complex than many realize. Image annotation is the manual or AI-assisted process of applying annotations and labels to images in a dataset. You can accelerate this process with the right tools, improving a project's workflow and quality control. Video Annotation Video annotation is more complex and nuanced than image annotation and usually needs specific tools to handle native video file formats. Videos include more layers of data, and with the right video annotation tools, you ensure labels are correctly applied from one frame to the next. Sometimes, an object might be partially obscured or contain occlusions, and an AI-based tool is needed to apply the right labels to those frames. For more information, check out our guide on the 5 features you need in a video annotation tool. DICOM and Medical Image/Video Annotation Medical image file formats, such as DICOM and NIfTI, are even more complex and nuanced than images or even videos in many ways. The most common use cases in healthcare for automated computer vision medical image and video annotation include pathology, cancer detection, ultrasound, microscopy, and numerous others. The accuracy of an AI-based model depends on the quality of the annotations and labels applied to a dataset. To achieve this, you need human annotators with the right skills and tools to easily handle dozens of medical image file formats. In most cases, especially at the pre-labeling and quality control stage, you need specialist medical knowledge to ensure the right labels are correctly created and applied. High levels of precision are essential, with most projects having to pass various FDA guidelines. As for data security, and data compliance, any tool you use needs to adhere to security best practices such as SOC 2 and HIPAA (the Health Insurance Portability and Accountability Act). Project managers need granular access to every stage of the data annotation and labeling process to ensure that annotators do their job well. With the right tool, one designed with and alongside medical professionals and healthcare data ops teams, all of this is easier to implement and guarantee. Find out more with our best practice guide for annotating DICOM and NIfTI Files. Benefits of Automated Data Annotation Automated data annotation and labeling for computer vision and other algorithmic-based models include the following: Cost-effective Manually annotating and labeling large datasets takes time. Every hour of that work costs money. In-house annotation teams are more expensive. But outsourcing isn’t cheap either, and then you’ve got to consider issues such as data security, data handling, accuracy, expertise, and workflow processes. All of this has to be factored into the budget for the annotation process. With automated, AI-supported data annotation, a human annotation team can manually label a percentage of the data and then have an AI tool do the rest. And then, whichever approach you use for managing the annotation workflow ⏤ unsupervised, supervised, semi-supervised, human-in-the-loop, or programmatic ⏤ annotators and quality assurance (QA) team members can guide the labeling process to improve accuracy and efficiency. Either way, it’s far more cost-effective than manually annotating and labeling an entire dataset. Faster annotation turnaround time Speed is as important as accuracy. The quicker you start training a model, the sooner you can test theories, address bias issues, and improve the AI model. Automated data labeling and annotation tools will give you an advantage when training an ML model. Ensuring a faster and more accurate annotation turnaround time so that models can go from training to production-ready more easily. Consistent and objective results Humans make mistakes. Especially if you’re performing the same task for 8 or more hours. Data cleaning and annotation is time-consuming, and the risk of errors or bias creeping into a dataset and, therefore, into ML models increases over time. With AI-supported tools, human annotator workloads aren’t as heavy. Annotators can take more time and care to get things right the first time, reducing the number of errors that must be corrected. Applying the most suitable, accurate, and descriptive labels for the project's use case and goals manually will improve the automated process once an AI tool takes over. Results from data annotation tasks are more consistent and objective with the support of AI-based software, such as active learning pipelines and micro-models. Increased productivity and scalability Ultimately, automated annotation tools and software improve the team's productivity and make any computer vision project more scaleable. You can handle larger volumes of data, annotate, and label images and videos more accurately. Which Label Tasks Can I Automate? With the right automated labeling tools, you should be able to easily automate most data annotation tasks, such as classifying objects in an image. The following is a list of data labeling tasks that an AI-assisted automation software suite can help you automate for your ML models: Bounding boxes: Drawing a box around an object in an image and video and then labeling that object. Automation tools can then detect the same or similar object(s) in other images or frames of videos within a dataset. Object detection: Using automation to detect objects or semantic instances of objects in videos and images. Once annotators have created labels and ontologies for objects, an AI-assisted tool can detect those objects accurately throughout a dataset. Image segmentation: In a way, this is more detailed than detection. Segmentation can reach the granular, pixel-based level within images and videos. With segmentation, a label or mask is applied to specific objects, instances, or areas of an image or video. Then AI-assisted tools can identify identical collections of pixels and apply the correct labels throughout a dataset. Image classification: A way of training a model to identify a set of target classes (e.g., an object in an image) using a smaller subset of labeled images. Classifying images is a process that can also include binary or multi-class classification, where there’s more than one label/tag for an object). Human Pose Estimation (HPE): Tracking human movements in images or videos is a computer-intensive task. HPE tracking tools make this easier, providing images or videos of human movement patterns that have been labeled accurately and in enough detail. Polygons and polylines: Another way to annotate and label images is by drawing lines around static or moving objects in images and videos. Once enough of these have been applied to a subset of data (automated tools can implement those same labels accurately across an entire dataset. Keypoints and primitives: Also known as skeleton templates, these are data-labeling methods to templatize specific shapes, such as 3D cuboids and the human body. Multi-Object Tracking (MOT): A way to track multiple objects from frame to frame in videos. With automated labeling software, MOT becomes much easier, providing the right labels are applied by annotation teams, and a QA workflow keeps those labels accurate across a dataset. Interpolation: Another way to use data automation to fill in the gaps between keyframes in videos. Auto object segmentation and detection, including instance segmentation and semantic segmentation, perform a similar role to interpolation. Now, look at the features you need in an automated data annotation tool and best practices for AI-assisted data labeling. (Source) What Features Do You Need in an Automated Data Annotation Tool? Here are 7 features to look for in an automated data annotation tool. Supports Model or AI-Assisted Labeling Naturally, if you’ve decided that your project needs an automated tool, you must pick one that supports model or AI-assisted labeling. Assuming you’ve resolved the “buy vs. build” question and are opting for a customizable SaaS platform rather than open-source, then you’ve got to select the right tool based on the use case, features, reviews, case studies, and pricing. Make a checklist of what you’re looking for first using a checklist app. That way, data ops and ML teams can provide input and ideas for the AI-assisted labeling features a software solution should have. Supports Different Types of Data & File Formats Equally crucial is that the solution you pick can support the various file types and formats you’ll find in the datasets for your project. For example, you might need to label and annotate 2D and 3D images or more specific file formats, such as DICOM and NIfTI, for healthcare organizations. Depending on your sector and use case, you might even need a tool to handle Synthetic-Aperture Radar (SAR) images in various modes for computer vision applications. Ensure every base is covered and that the tool you pick supports images and videos in their native format without any issues (e.g., needing to reduce the length of videos). Easy-to-Use Tool With a Collaborative Dashboard Considering the number of people and stakeholders usually involved in computer vision projects, having an easy-to-use labeling tool with a collaborative dashboard is essential. Especially if you’ve outsourced the annotation workloads. With the right labeling tools, you can keep everyone on the same page in real time while avoiding mission creep. Data Privacy and Security DataOps teams must consider data privacy and security when sourcing image or video files for a computer vision project. In particular, whether there are any personally identifiable data markers or metadata within images or videos in datasets. Anything like that should be removed during the data cleaning process. Afterwards, you must make the right provisions for moving and storing the datasets. Especially if you’re in a sector with more stringent regulatory requirements, such as healthcare. You need to get this right if you’re outsourcing data annotation tasks. Only then can you move forward with the annotation process. Comprehensive platforms ensure you can maintain audit and security trails to demonstrate data security compliance with the relevant regulatory bodies. Automated Data Pipelines When a project involves large volumes of data, an easier way to automate data pipelines is to connect datasets and models using Encord’s Python SDK and API. This way, it’s even easier and faster to train an ML model continuously. Customizable Quality Control Workflows Make quality control (QC) or QA workflows customizable and easily managed. Validate labels and annotations being created. Check that the annotation teams are applying them correctly. Reduce errors and bias, and fix bugs in the datasets. You can automate this process using the right tool to check the AI-assisted labels applied from start to finish. Training Data and Model Debugging Every training dataset includes errors, inaccuracies, poorly-labeled images or video frames, and bugs. Pick an automated annotation tool that will help you fix those faster. Include this in your quality control workflows so annotators can correct mistakes and resubmit reformatted images or videos to the training datasets. Automated Data Annotation Best Practices Now let’s take a quick look at some of the most efficient automated data annotation best practices. Develop Clear Annotation Guidelines In the same way that ML models can’t train without accurately labeled data, annotation teams need guidelines before they start work. Create these guidelines and standard operating procedure (SOP) documents with the tool they’ll be using in mind. Align annotation guidelines with the product’s features and functionality and your organization's in-house data best practices and workflows. Design an Iterative Annotation Workflow Using the above as your process, incorporate an iterative annotation workflow. So this way, there are clear steps for processing data, fixing errors, and creating the right labels and annotations for the images and videos in a dataset. Manage Quality Assurance (QA) and Feedback via an Automated Dashboard In data-centric model training, quality is mission-critical. No project gets this completely right, as MIT research has found that even amongst best-practice benchmark datasets, at least 3.4% of labels are inaccurate. However, with a collaborative automated dashboard and expert review workflows, you can reduce the impact of common quality control headaches, such as inaccurate, missing, mislabeled images or unbalanced data, resulting in bias or insufficient data for edge cases. Read Also: 5 ways to improve the quality of your labeled data. Automated Data Annotation With Encord With Encord Annotate, Index, and Active, automated tools used by world-leading AI teams, you can accelerate data labeling workflows more effectively, securely, and at scale. Encord was created to improve the efficiency of automated image and video data labeling for computer vision projects. Our solution also makes managing a team of annotators easier, takes more time, and is cost-effective while reducing errors, bugs, and bias. With Encord, you can achieve production AI faster with ML-assisted labeling, training, and diagnostic tools to improve quality control, fix errors, and reduce dataset bias. Make data labeling more collaborative, faster, and easier to manage with an interactive dashboard and customizable annotation toolkits. Improve the quality of your computer vision datasets with Encord Index and enhance model performance with Active. Key Takeaways AI, ML, and CV models need high-quality and a large volume of accurately labeled and annotated data to train, learn, and go into production. It takes time to source, clean, and annotate enough data to reach the training stage. Automation, using AI-based tools, accelerates the preparation process. Automated data labeling and annotation reduce the time involved in one of the most crucial stages of any computer vision project. Automation also improves quality, accuracy, and the application of labels throughout a dataset, saving you time and money. Ready to accelerate the automation of your data annotation and labeling? Want to stay updated? Follow us on Twitter and LinkedIn for more content on computer vision, training data, and active learning. Join the Slack community to chat and connect.
Apr 14 2023
4 M
How to Use Low-Code and No-Code Tools for Computer Vision
The use of low-code and no-code environments, platforms, and active learning tools for computer vision is on the rise. Until recently, the only way to deploy software and algorithms for computer vision was through open-source applications or subscribing to proprietary tools (e.g., Software as a Service (SaaS) solutions), such as Encord. Now there’s a third option: low-code and no-code active learning platforms for active learning computer vision projects. You can build active learning tools and applications with zero technical knowledge and expertise with no-code solutions. Low-code solutions are similar, although a small amount of coding knowledge and experience is often useful. This article compares and contrasts no-code and low-code computer vision platforms. We look at why businesses and organizations are keen to deploy no-code and low-code software for computer vision projects. What is a No-Code Computer Vision Platform? Since the pandemic, the no-code and low-code development market has experienced even faster growth. In 2020, the market was worth over $10 billion and is expected to reach $94 billion in 2028, with a compound annual growth rate (CAGR) of 31.6%. At the time, businesses didn’t have the resources or budgets to commit to software development projects. So, one of the best solutions was to have teams without coding skills build websites, software, and apps, and the best way to achieve this was using no-code and low-code development platforms. No-code and low-code solutions were already popular in several sectors. However, given the time and resource limitations the pandemic imposed on organizations, it became necessary to look for solutions many wouldn’t have considered previously. Fortunately, the low-code/no-code software market was already active, with thousands of products and solutions on the market already. Many of which could be adapted and used for computer vision projects. For computer vision (CV) and machine learning (ML) projects, software developed using no-code tools means that people without coding experience can design and deploy them. Similarly, leveraging mobile app development services can streamline creating user-friendly applications, enhancing the overall project efficacy and customer interaction. There are numerous benefits to this, as we’ll soon cover. No-Code vs. Low-Code No-code and low-code software and development platforms are very similar. For practical purposes, the only significant difference is that low-code solutions require some coding knowledge. Whereas no-code are usually drag-and-drop interfaces. Unlike no-code website builders, non-technical people can simply select the features they want and move them into position. Organizations and teams building applications for computer vision projects can use these no-code and low-code app development platforms to accelerate AI (artificial intelligence) model training and deployment. Both types of solutions reduce the go-to-market time for new applications and make it easier for ML and data ops teams to start training computer vision models faster. The Benefits of Accelerated AI Model Training and Deployment Training and deploying an AI model involves several stages, including image or video-baseddata annotation work. Depending on your sector or specific use case ⏤ healthcare, retail, aerospace, defense, etc. ⏤ you might not be able to find the right tools for the project. It might be quicker to build your own; however, you don’t want to spend 9 to 12 months (or more) and 6 figures to achieve this. A more sensible, cost, and time-effective solution would be to use a low-code or no-code development platform to accelerate AI model training and development. Here are five reasons you might want to use a low-code or no-code platform for your next computer vision project: Collaborative, Accessible Tools for Teams As a rule, low-code/no-code tools are easy to use and more accessible for non-technical teams. Making them more collaborative when non-technical people are involved in computer vision projects, such as operations, marketing, sales, or medical professionals in the healthcare sector. Because these solutions often have pre-built AI models within them, there are already basic tasks many can perform before integrating low-code/no-code tools with more advanced CV models. Accelerated Time-to-Market With any computer vision project, the time-to-market when customized coding is needed within datasets, model development, or active learning platforms. When you use a low-code/no-code alternative, the time-to-market accelerates. One of the reasons for this is pre-built AI models and ready-made datasets templates. You might need to make some customizations for your project and use case, but doing so is easier when using low-code/no-code tools. Lower Costs, Better Results Because of the time and cost involved when implementing and deploying computer vision projects, anything you can do to reduce costs and improve results is an investment worth making. Naturally, developers and data science engineers aren’t necessary for low-code/no-code tools so this approach will save time and money. The more functionality you can automate, the quicker you can train and deploy an active learning computer vision model. Low-code and no-code development platforms make it easier for those managing computer vision projects to accelerate and automate numerous manual aspects of project workflows. Easier Diagnostics and Debugging As we’ve mentioned in a previous post, “Debugging deep learning models can be a complex and challenging task.” Debugging a computer vision model is very challenging. “The more advanced the neural network selected for the model, the more complex the issues it can have,” making debugging a headache. With low-code/no-code tools, it’s somewhat easier to debug models or the software that AI-based models run because there aren’t thousands of lines of code to scan. Making it significantly easier to quickly identify, diagnose, and debug the model when something isn’t working. Certified Data Security One of the final advantages is that low-code/no-code tools have high levels of data security built-in. Data security is mission-critical for many computer vision projects, especially when the datasets a model is being trained on are potentially sensitive, such as healthcare and medical images. For data compliance and security reasons, the last thing you can afford is a data breach or leak. It could affect the entire project, potentially wasting months of work. Having a computer vision platform that’s HIPAA and SOC 2 compliant is a distinct advantage, especially when you’re handling sensitive data. Accelerate AI Data Annotation With Active Learning for Computer Vision One of the most cost and time-effective ways to accelerate computer vision machine learning projects simply is by using Encord or Encord Active. Encord also has an Annotator Training Module that helps leading AI companies quickly bring their annotator team up to speed and improve the quality of annotations created. Whatever tool(s) you deploy or software you need to accelerate model development, training, deployment, and iterative learning, Encord has everything you need. Depending on your project and use cases, you can use aspects of our toolkit that don’t require coding skills, making them as easy to use as SaaS tools or low-code/no-code solutions. Most non-technical people feel completely comfortable using Encord, and others can implement anything that requires more technical skills on the team. At Encord, our active learning platform for computer vision is used by a wide range of sectors - including healthcare, manufacturing, utilities, and smart cities - to annotate human pose estimation videos and accelerate their computer vision model development. Encord is a comprehensive AI-assisted platform for collaboratively annotating data, orchestrating active learning pipelines, fixing dataset errors, and diagnosing model errors & biases. Try it for free today.
Mar 14 2023
3 M
How to Onboard 100s of Annotators for High-Quality Labels
Introduction Over the last 2 years, we have helped hundreds of computer vision companies onboard and train thousands of annotators for their data labeling projects. The main takeaway? It’s a time-consuming and tedious process. The same questions kept popping up: “How do we ensure the annotators deliver high-quality labels?” “Is there a most efficient way to onboard them onto annotation projects?” “How long does it typically take for annotators to be qualified and ready to start labeling training data?” "Should we be retraining our annotators?" Our answer was often "It depends" - which wasn't very satisfying to us, nor to our customers. And which is why, over the last year, our team has been at work building the Annotator Training platform we wish existed! Onboarding and training new annotators can be a daunting task, especially when dealing with complex datasets and specific use cases. But with Encord's Annotator Training Module, you can streamline the process, provide clear and concise training materials, and measure annotator performance before allowing them to annotate images that your models are trained on. Accurate labeling ensures that your models can properly identify and classify objects. However, producing high-quality labeling is challenging, especially when dealing with large datasets. In this article, we will explore you can onboard annotators using the Annotator Training Module to improve the speed and performance of your annotators and the speed and high-quality labels. If you like this post we know you’d also like these: Computer Vision Data Operations Best Practice Guide 9 Best Image Annotation Tools for Computer Vision [2023 Review] The Complete Guide to Data Annotation Why High-Quality Labels are Critical for Machine Learning Models As you know machine learning models rely on high-quality training data to make accurate predictions, and thus decisions. In computer vision applications, the quality of the training data is dependent on the quality of the annotations. Annotations are labels that are applied to images or videos to identify objects, regions, or other features of interest. For example, in an image of a street scene, annotations may include the locations of vehicles, pedestrians, and traffic signs and classifications on the time of day, weather, or action taking place in the image. Inaccurate or inconsistent annotations can lead to incorrect predictions and decisions, which can have serious consequences further down the line when you deploy your models to production To ensure high-quality annotations, it is essential to have well-trained and experienced annotators who follow best practices and guidelines. However, onboarding and training thousands of annotators can be a challenge, especially when dealing with multiple annotators (and ever changing personnel), complex domains, and different use cases. Existing Practices for Annotator Onboarding Traditional methods for annotator onboarding typically involve providing annotators with written guidelines and instructions, and then relying on them to apply those guidelines consistently. However, this approach can quickly lead to variations in annotation quality and inconsistencies between annotators. Another common approach is to have a small group of expert annotators who perform the annotations and then use their annotations as ground truth library for your annotators to refer to. The downside with this approach is that it can be expensive, time-consuming, and it doesn’t scale very well. To address these challenges, a growing number of companies are turning to specialized annotation tools that help ensure consistency and quality in the annotation training process. These tools provide a more structured and efficient way to onboard new annotator. Be aware though, with the majority if these tools, it can be difficult to efficiently onboard and train yours annotators. That’s where Encord’s Annotator Training Module comes in. Measuring Annotation Quality I think we can agree that High-quality annotation is critical for the success of your computer vision models. Therefore, measuring the quality of annotations is an essential step to ensure that the data is reliable, accurate, and unbiased. In this chapter, we will discuss the importance of measuring annotation quality and the different methods used to assess the quality of annotations. Skip ahead if you want to read about existing practices and the Annotator Training Module. Overview of Different Methods to Measure Annotation Quality There are different methods to measure the quality of annotations. Some of the most common methods are: Benchmark IOU: It measures the degree of agreement between two different labels. The most common method to measure Benchmark IOU agreement is through the use of intersection-over-union (IOU) scores. IOU measures the overlap between the bounding boxes created by different annotators. The higher the IOU score, the greater the agreement between the annotators. Accuracy: Accuracy measures the proportion of annotations that are correctly labeled. It is calculated by dividing the number of correctly labeled annotations by the total number of annotations. Ground truth Benchmark: The last approach is to have a small group of expert annotators who perform the annotations and then use their annotations as ground truth for to benchmark quality against. Ground truth Benchmark labels are the most reliable method for measuring annotation quality, but they can be time-consuming to create. Comparison of Different Methods Each method for measuring annotation quality has its strengths and weaknesses. Benchmark IOU is a good measure of the degree of agreement between annotations, but it can be affected by the size and shape of the object being annotated. Accuracy is a good measure of the proportion of annotations that are correct, but it does not take into account the degree of agreement between annotators. Ground truth Benchmark labels are the most reliable method for measuring annotation quality, but they can be time-consuming to create. Encord’s Annotator Training Module mixes all three methods into one and automates the evaluation process (Benchmark IOU ofcourse only applicable for cases with bounding boxes, polygons, or segmentation tasks). Introducing Encord's Annotator Training Module The Annotator Training Module has been designed to integrate seamlessly into your existing data operations workflows. The module can be customized to meet the specific needs and requirements of each use-case and project, with the ability to adjust the evaluation score for each project. With the Annotator Training Module, onboarding and evaluating annotators becomes a breeze. The module is designed to ensure that annotators receive the proper training and support they need to produce high-quality annotations consistently. The module includes the option to include Annotator training instructions directly in the UI. Such instructions can range from detailed instructions on how to use the annotation tool to best practices for specific annotation tasks. You can customize the training instructions according to your specific use cases and workflows, making it easier for your annotators to understand the project's requirements and guidelines. Your Data Operations team (or you) can monitor the performance of your annotators and identify areas for improvement. Step-by-Step Guide on How to Use the Module to Onboard Annotators Using Encord's Annotator Training Module is a straightforward and easy process. Here is a step-by-step guide on how to use the module to onboard annotators: If you want to view the full guide with a video and examples see this guide: Step 1: Upload Data First you upload the data to Encord and create a new dataset. This dataset will contain the data on which the ground truth labels are drawn. In order to do this, you needs to choose the appropriate dataset for your specific use case. Once the dataset is chosen, it needs to be uploaded to the annotation platform. This is done by selecting the dataset from your local folder or uploading it via your cloud bucket. Step 2: Set up Benchmark Project The next step in the process is to set up a benchmark project. The benchmark project is used to evaluate the quality of the annotations created by the annotators. It is important to set up the benchmark project correctly to ensure that the annotations created by the annotators are accurate and reliable. To set up the benchmark project, you needs to create a new standard project. Once the project is created, an ontology needs to be defined. The ontology is a set of rules and guidelines that dictate how the annotations should be created. This ensures consistency across all annotations and makes it easier to evaluate the quality of the annotations. Step 3: Create Ground Truth Labels After the benchmark project is set up, it is time to create the ground truth labels. This can be done manually or programmatically. The ground truth labels are the labels that will be used to evaluate the accuracy of the annotations created by the annotators. Manually creating the ground truth labels involves having subject matter experts use the annotation app to manually annotate data units, as shown here with the bounding boxes drawn around the flowers. Alternatively, one can use the SDK to programmatically upload labels that were generated outside Encord. Step 4: Set up and Assign Training Projects Once the ground truth labels are created, it is time to set up and assign a training project with the same ontology. Once the training project is created, the scoring functions need to be set up. These will assign scores to the annotator submissions and calculate the relative weights of different components of the annotations. With the module set up, you can now invite annotators to participate in the training. Encord provides a pool of trained annotators that can be added to your project, or you can invite your own annotators. Once the annotators have been added to the project, they will be provided with the training tasks to complete. Step 5: Annotator Training With the training project set up and the scoring functions assigned, it is time to train the annotators using the assigned tasks. Each annotator will see the labeling tasks assigned to them and how many tasks are left. The progress of the annotators can be monitored by the admin of the training module. This allows the admin to see the performance of the annotators as they progress through the training and to evaluate their overall score at the end. Step 6: Evaluate Annotator Performance After the annotators have completed their assigned tasks, it is time to evaluate their performance using the scoring function. This function assigns scores to the annotations created by the annotators and calculates the overall score. If necessary, modifications can be made to the scoring function to adjust the relative weights of different components of the annotations. This ensures that the scoring function accurately reflects the importance of each component and that the overall score accurately reflects the quality of the annotations. Finally, the annotators can be provided with feedback on their performance and given additional training if necessary. Conclusion Annotating large datasets is a complex and time-consuming process, but it is a crucial step in developing high-quality machine learning models. Without accurate and consistent annotations, machine learning algorithms will produce inaccurate or unreliable results. Encord's Annotator Training Module provides a powerful solution for data operation teams and computer vision engineers who need to onboard thousands of annotators quickly and efficiently. With the module, you can ensure that your annotators receive the proper training and support they need to produce high-quality annotations consistently. Want to stay updated? Follow us on Twitter and LinkedIn for more content on computer vision, training data, and active learning. Join the Slack community to chat and connect.
Mar 12 2023
5 M
Best Image Annotation Tools for Computer Vision [Updated 2024]
If you're looking for an image annotation tool, you have plenty of choices. The market is saturated, making it challenging to find the best tool for your needs. To help you out, we did much of the research for you to streamline your buying process. In this article, you will find a detailed overview of the most popular data annotation tools, including: Encord, Amazon SageMaker Ground Truth, Scale Rapid, Supervisely, CVAT, Labelbox, Playment, Appen, Dataloop, SuperAnnotate, V7, Hive, Label Studio, COCO Annotator, Make Sense, VGG Image Annotator, and, LabelMe Best Image Annotation Tools The sections below give an overview of the key features and user reviews for the above mentioned tools. The summary table below compares all the tools based on supported data types, annotation types, ease of use, and automation. Encord Encord is an end-to-end data development platform with an advanced image annotation tool for complex computer vision and multimodal use cases. The platform offers state-of-the-art model-assisted labeling and customizable workflows to accelerate image annotation projects and build production-ready models. Key Features AI-assisted labeling: Automate 97% of your image annotations with 99% accuracy by leveraging SOTA automated labeling capabilities such as Meta AI’s Segment Anything Model (SAM). Full suite of tools: Encord supports a range of labeling options, such as bounding boxes, rotatable boxes, polygons, polylines, key points, and classifications to support your model requirements. Accelerate with models-in-the-loop: Bring your own model to the Encord platform or leverage one of our Agents to pre-label datasets. Scalability: Encord lets you scale AI projects by supporting extensive datasets of up to 500,000 images. Build balanced datasets: Filter and slice datasets in a consolidated visual explorer and export for labeling in one click. Encord supports deep search, filtering, and metadata analysis. Complex ontologies: Build nested relationship structures in your data schema to improve the quality of your model output. Bulk classification: Leverage natural language or similarity search to select large datasets and label en masse, queue for review to accelerate labeling operations. Build reliable quality control workflows: Build robust workflows with multi-step review stages and consensus benchmarking for quality assurance. Find and fix label errors: Automatically surface labeling errors to shift your attention to the labels impacting model performance. Collaboration: Control user roles with permissions, manage task assignments and infinitely scale your MLOps workflows. Enterprise-grade security as standard: Encord Annotate complies with the General Data Protection Regulation (GDPR), System and Organization Controls 2 (SOC 2), and Health Insurance Portability and Accountability Act (HIPAA) standards while using advanced encryption protocols to ensure data privacy. Integrations: Encord allows you to retain total control of your data. Securely connect your native cloud storage buckets and programmatically control workflows. Advanced Python SDK and API access with easy export into JSON and COCO formats. Integrated Data Labeling Services: Outsource your labeling tasks to an expert workforce of vetted, trained, and specialized annotators. Encord G2 Review Summary Encord has a rating of 4.8/5 based on 60 reviews. Users prefer Encord’s powerful ontology feature, which lets them define rich taxonomy for all data sizes. In addition, the platform’s collaborative features and granular annotation tools help users improve annotation quality. Learn how Encord Annotate helps you create rich ontologies for an efficient labeling process. Curious? Try it out Amazon SageMaker Ground Truth Amazon SageMaker Ground Truth is a human-in-the-loop data labeling platform that offers features to label large datasets. It provides a self-serve and a managed service option to help you streamline your annotation workflow for multiple CV tasks. Amazon SageMaker Ground Truth Key Features Data Generation: The platform offers tools to fine-tune pre-trained models on a few data points to generate synthetic data samples for more diverse training. Model Evaluation: Sagemaker Ground Truth lets you evaluate foundation models based on multiple metrics such as accuracy, relevancy, toxicity, and bias through human feedback. Labeling Templates: It features over thirty labeling templates for multiple CV and NLP tasks, including image classification, object detection, text classification, and named entity recognition (NER). Interactive Dashboards: The tool offers intuitive dashboards and user-friendly interfaces to monitor labeling progress across multiple projects. G2 Review Summary Amazon SageMaker Ground Truth has a rating of 4.1/5 based on 19 reviews. Users like its ease of use and advanced annotation capabilities. However, they feel it is expensive, and tracking labeling performance is challenging. Scale Rapid Scale Rapid is a data and labeling services platform that supports computer vision use cases. It specializes in reinforcement learning with human feedback (RLHF), user experience optimization, large language models (LLMs), and synthetic data. Scale Rapid Key Features Supported Data Types: Scale lets you annotate text, images, video, audio, and point-cloud data. Customizable Workflows: Offers customizable labeling workflows tailored to specific project requirements and use cases. Data labeling services: Provides high-quality data labeling services for various data types, including images, text, audio, and video. Scalability: Capable of handling large-scale annotation projects and accommodating growing datasets and annotation needs. G2 Review Summary Scale Rapid has a rating of 4.4/5 based on 11 reviews. Users say it is easy to learn and does not require complex installation procedures. However, they feel the user interface is clunky and the tool’s pricing is complex. Find out about the top tools that help you perform reinforcement learning with human feedback (RLHF). Supervisely Supervisely is an end-to-end computer vision platform that offers multiple annotation tools for labeling images and videos. It features AI-based labeling that lets users automate labeling workflow through advanced ML models. Supervisely Key Features Versatile Annotation Tool: It supports multiple annotation types, including bounding boxes, polygons, polylines, points, and segmentation masks for precise labeling. Supported Data Types: Supervisely lets you label images, videos, point cloud, and medical image data. Smart Labeling Tools: Feature a class-agnostic smart tool based on customizable neural networks for capturing any object type, depending on your use case. Collaboration: The platform lets you collaborate with team members and assign relevant user roles to track issues and labeling performance. G2 Review Summary Supervisely has a rating of 4.7/5 based on ten reviews. Users like the tool’s integration with multiple apps within the Supervisely ecosystem, giving a smooth user experience. However, the number of options can be overwhelming, and the platform has latency issues. CVAT (Computer Vision Annotation Tool) CVAT is an open-source web-based image annotation tool by Intel. In 2022, CVAT’s data, content, and GitHub repository became a part of OpenCV, where CVAT continues to be open-source. Furthermore, CVAT can also help annotate QR codes within images, facilitating the integration of QR code recognition into computer vision pipelines and applications. CVAT Key Features Manual Annotation Tools: The tool supports various annotation types, including bounding boxes, polygons, polylines, points, and cuboids, catering to diverse annotation needs. Multi-platform Compatibility: Works on multiple operating systems such as Windows, Linux, and macOS, providing flexibility for users. Export Formats: CVAT supports numerous data formats, including JSON, COCO, and Pascal VOC, ensuring annotation compatibility with diverse tools and platforms. Automated Labeling: CVAT supports multiple algorithms, including the Segment Anything Model (SAM), YOLOv3, and Deep Extreme Cut (DEXTR). G2 Review Summary CVAT has a rating of 4.5/5 based on two reviews. Users like that the tool is free to use and requires no configuration and installation process because it is web-based. However, its slow performance and backend server failure are the most significant concerns. Labelbox Labelbox is a US-based data annotation platform founded in 2017 that provides a unified framework for curating and labeling datasets with collaboration and model evaluation tools. Besides a stand-alone image labeling platform, the tool offers managed annotation services with data labeling experts. Labelbox Key Features Data Management: Labelbox offers QA workflows and data annotator performance tracking. Customizable Labeling Interface: It features a user-friendly interface, providing easy-to-navigate editors for specific needs. Automation: Allows integration with AI models for automatic data labeling to accelerate the annotation process. Annotation Capabilities: It supports annotation for multiple data types beyond images, including text, video, audio, geospatial and medical images. G2 Review Summary LabelBox has a rating of 4.7/5 based on 33 reviews. Users find the tool’s data management features helpful. However, they feel that it does not perform well with high-resolution images. Playment Playment is an Indian-based end-to-end data annotation platform founded in 2015 and now operating under Telus’ ownership. It offers managed annotation services by employing computer vision teams to annotate training data for multiple use cases. Playment Key Features Data Labeling Services: Provides high-quality data labeling services for various data types, including images, videos, text, and sensor data. Support: Global workforce of contractors and data labelers. Scalability: Capable of handling large-scale annotation projects and accommodating growing datasets and annotation needs. Audio Labeling Tool: The tool features a speech recognition training platform that can handle over five hundred languages and dialects. G2 Review Summary Playment has a rating of 4.7/5 based on 11 reviews. Users find Playment’s annotation performance fast and accurate. However, they find the tool expensive and that it needs more improvement in automated labeling features. Appen Appen is a data labeling services platform founded in 1996, making it one of the first and oldest solutions in the market, offering data labeling services for various industries. In 2019, it acquired Figure Eight to expand its software capabilities and help businesses train and improve their computer vision models. Appen Key Features Data Labeling Services: Support for multiple annotation types (bounding boxes, polygons, and image segmentation). Data Collection: Data sourcing (pre-labeled datasets), data preparation, and real-world model evaluation. Natural Language Processing: Support for natural language processing (NLP) tasks such as sentiment analysis, entity recognition, and text classification. Image and Video Analysis: Analyzes images and videos for tasks such as object detection, image classification, and video segmentation. G2 Review Summary Appen has a rating of 4.2/5 based on 28 reviews. Users like that the tool is web-based and does not require specific installation procedures. However, the platform’s server crashes frequently, and the support team is slow to respond. Dataloop Dataloop is an Israel-based data labeling platform that provides a comprehensive solution for data management and annotation projects. The tool offers data labeling capabilities across images, text, audio, and video annotation, helping businesses train and improve their machine learning models. Dataloop Key Features Data Annotation: Supports multiple image annotation tasks, including classification, detection, and semantic segmentation. Collaboration Tool: It features tools for real-time collaboration among annotators, project sharing, and version control, allowing for efficient teamwork. Data Management: Offers data management capabilities, including data versioning, tracking, and organization for streamlined workflows. Model Management: Dataloop offers tools to manage different model versions and download SOTA models from the Model Marketplace. G2 Review Summary Dataloop has a rating of 4.4/5 based on 90 reviews. The tool’s plus points include its ease of use and annotation efficiency. However, users find it challenging to learn and face frequent performance issues. SuperAnnotate SuperAnnotate is an end-to-end AI platform that offers tools for data curation and automatic annotation with MLOps functionalities. It also lets you fine-tune LLMs using annotated data and RLHF. SuperAnnotate Key Features Multi-Data Type Support: Versatile annotation features for labeling videos, text, audio, and image data. AI Assistance: Integrates AI-assisted annotation to accelerate the labeling process and improve efficiency. Customization: Provides customizable annotation interfaces and workflows to tailor annotation tasks to specific project requirements. Export Formats: SuperAnnotate supports multiple data formats, including popular ones like JSON, COCO, and Pascal VOC. G2 Review Summary SuperAnnotate has a rating of 4.9/5 based on 137 reviews. Users find the tool’s feature set comprehensive and the interface intuitive. However, there have been complaints regarding its custom workflow setup and high price. V7 Labs V7 is a UK-based data annotation platform founded in 2018. The company enables teams to annotate image and video data using automated pipelines and custom workflows. The platform also offers model and data management tools to help users build high-quality training data for scalable AI projects. V7 Key Features Collaboration Capabilities: Project management and automation workflow functionality, with real-time collaboration and tagging. Data Management: The tool offers data management features, including functionalities to filter and sort data. It also helps organize and manage data classes at team and dataset levels. Auto-Annotate: Features auto-annotation that lets you use deep learning models to create pixel-perfect polygon masks. Auto-Track: V7 offers an auto-track feature for object tracking and instance segmentation in long videos. G2 Review Summary V7 has a rating of 4.8/5 based on 52 reviews. Users find its automation and collaboration features significantly helpful. However, they feel it lacks file manipulation options, and its sorting and filtering features do not perform well with large files. Hive Hive is a content-moderation platform that offers deep learning models to highlight harmful and explicit content in images, videos, text, and audio. It also features search and generative APIs to visualize similarities between images and videos and generate images based on textual prompts. Hive AI Key Features Ease of use: Hive offers an intuitive interface with multiple in-built image and text classification models. Embeddings: The platform lets you quickly create text embeddings to build retrieval augmented generation (RAG)-based LLMs. Search: Hive offers versatile web search functionality. You can use image prompts to retrieve relevant links to similar images. Generative Artificial Intelligence (Gen AI): Hive features APIs to generate text, images, and videos based on textual prompts. G2 Review Summary Hive has a rating of 4.6/5 based on 528 reviews. Users find its project management and collaboration features helpful. However, the interface is challenging to navigate and has a few glitches, which makes it complex to operate. Label Studio Label Studio is a popular open-source data labeling platform for annotating various data types, including images, text, audio, and video. It supports collaborative labeling, custom labeling interfaces, and integration with machine learning (ML) pipelines for data annotation tasks. Label Studio Key Features Customizable Labeling Interfaces: Label Studio lets you label data through flexible configurations that allow you to tailor annotation interfaces to specific tasks. Collaboration Tools: Real-time annotation and project-sharing capabilities for seamless collaboration among annotators. Export Formats: Label Studio supports multiple data formats, including JSON, CSV, TSV, and VOC XML like Pascal VOC, facilitating integration and annotation from diverse sources for machine learning tasks. ML Pipelines: Label Studio lets you connect the model development pipeline with the data labeling project. The method allows you to use ML models to predict labels, evaluate model performance, and perform human-in-the-loop labeling. G2 Review Summary G2 review not available. COCO Annotator COCO Annotator is a web-based labeling tool by Justin Brooks that is under the MIT license. The tool helps streamline the process of annotating images for object recognition, localization, and key point detection models. It also offers a range of features that cater to the diverse needs of machine learning practitioners, data scientists, and researchers. COCO Annotator Key Features Image Annotation: Supports annotation of images for object detection, instance segmentation, keypoint detection, and captioning tasks. Export Formats: The tool exports and stores annotations in the COCO format to facilitate large-scale object detection. Automation: The tool makes annotating an image easier by incorporating semi-trained models. It also provides access to advanced selection tools, including the Mask Region-based Convolutional Neural Network (MaskRCNN), Magic Wand, and Deep Extreme Cut (DEXTR) frameworks. Metadata Management: Users can create custom metadata for each instance or object. G2 Review Summary G2 review not available. Make Sense Make Sense AI is a user-friendly open-source annotation tool available under the GPLv3 license. It is accessible through a web browser and does not require advanced installations. The tool simplifies the annotation process for multiple image types. Make Sense Key Features Open Sourced: Make Sense AI stands out as an open-source tool, freely available under the GPLv3 license, fostering collaboration and community engagement for its ongoing development. Accessibility: It ensures web-based accessibility, operating seamlessly in a web browser without complex installations, promoting ease of use across various devices. Export Formats: It facilitates exporting annotations in multiple formats (YOLO, VOC XML, VGG JSON, and CSV), ensuring compatibility with diverse machine learning algorithms. Supported Annotation Types: The tool supports rectangles, lines, points, and polygons. G2 Review Summary G2 review not available. VGG Image Annotator VGG Image Annotator (VIA) is a versatile open-source tool by the Visual Geometry Group (VGG) for manually annotating image and video data. Released under the permissive BSD-2 clause license, VIA serves the needs of academic and commercial users, offering a lightweight and accessible solution for annotation tasks. VIA Key Features Lightweight and User-Friendly: VIA is a lightweight, self-contained annotation tool that uses HTML, Javascript, and CSS without external libraries. Offline Capability: The tool works offline, providing a full application experience within a single HTML file of less than 200 KB. Audio and Video Annotation: In addition to images, the tool lets users define temporal segments in audio and video data with textual descriptions. Supported Annotation Types: The tool allows you to draw rectangles, circles, ellipses, polygons, points, and polylines. G2 Review Summary G2 review not available. LabelMe LabelMe is an open-source web-based tool by the MIT Computer Science and Artificial Intelligence Laboratory (CSAIL) that allows users to label and annotate images for computer vision research. It provides a user-friendly interface for drawing bounding boxes, polygons, and semantic segmentation masks to label objects within images. LabelMe Key Features Web-Based: Accessible through a web-based interface, allowing you to perform annotation tasks in any modern web browser without requiring software installation. Supported Data Types: The tool supports image and video annotation. Supported Annotation Types: LabelMe lets you draw polygons, rectangles, circles, lines, and points. Export Format: It lets you export annotation in VOC and COCO format for semantic and instance segmentation. G2 Review Summary G2 review not available. Key Takeaways: Image Annotation Tools in 2024 As data volume and variety increase, businesses must invest in a suitable and reliable annotation tool to build high-quality datasets for training models. Below are a few key points regarding the top image annotation tools and tips for selecting an appropriate solution. Best Annotation Tools in 2024: Encord, Amazon Sagemaker Ground Truth, and Scale Rapid are the top annotation tools in 2024. Ease-of-use: Most G2 reviews highlight issues with the user interface. You should ensure that you select a tool that offers intuitive navigation and labeling features. Automation: Select a platform that offers state-of-the-art automation features, including pre-trained models and smart labeling tools. Open-source vs. Paid Platforms: While open-source tools offer a cost-effective solution, they have limited functionality. Paid tools provide a rich feature set with robust customer support to help you annotate multiple data types. So, streamline your CV operations with the annotation tool that best suits your needs.
Feb 08 2023
10 M
5 Ways to Improve The Quality of Labeled Data
Computer vision models are improving in sophistication, accuracy, speed, and computational power every day. Machine learning teams are training computer vision models to solve problems more effectively, making the quality of labeled data more important than ever. Poor quality labeled data, or errors and mistakes within image or video-based datasets can cause huge problems for machine learning teams. Regardless of the sector or problem that needs solving, if computer vision algorithms don’t have access to the quality and volume of the data they need they won’t produce the results organizations need. In this article, we take a closer look at the common errors and quality issues within labeled data, why organizations need to improve the quality of datasets, and five ways you can do that. Common Data Error and Quality Problems in Computer Vision? Data scientists spend a lot of time — too much time, many would say — debugging data and adjusting labels in datasets to improve model performance. Or if the labels that have been applied aren’t up to the standard required, part of the dataset needs to go back to annotators to be re-labeled. Despite annotation automation and AI-assisted labeling tools and software, reducing errors and improving quality in datasets is still time-consuming work. Often, this is done manually, or as close to manual as possible. However, when there are thousands of images and videos in a dataset, sifting through every single one to check for quality and accuracy becomes impossible. As we’ve covered in this article, the top three causes of errors and quality problems in computer vision datasets are: Inaccurate labels; Mislabeled images; Missing labels (unlabeled data); Unbalanced data and corresponding labels (e.g. too many images of the same thing), resulting in data bias, or insufficient data to account for edge cases. Depending on the quality of the video or image annotation work and AI-supported annotation tools that are used, and the quality control process, you could end up with all three issues throughout your dataset. Inaccurate labels cause an algorithm to struggle to identify objects in images and videos correctly. Common examples of this include loose bounding boxes or polygons, labels that don’t cover an object, or labels that overlap with other objects in the same image or frame. Applying the wrong label to an object also causes problems. For example, labeling a “cat” as a “dog” would generate inaccurate predictions once a dataset was fed into a computer vision model. MIT research shows that 3.4% of labels are wrong in best practice datasets. Meaning, there’s an even greater chance there are more inaccurate labels in datasets most organizations use. Missing labels in a ground truth dataset also contribute to computer vision models producing the wrong predictions and outcomes. Naturally, the aim of annotation work should be to provide the best, most accurate labels and annotations possible for image and video datasets. According to the relevant use case and problems you are trying to solve. Why Do You Need To Improve The Quality of Your Datasets? Improving the quality of a dataset that’s being fed into a machine learning or computer vision model is an ongoing task. Quality can always be improved. Every change made to the annotations and quality of the labels in a dataset should generate a corresponding improvement in the outcomes of your computer vision projects. For example, when you first give an algorithmic model a training dataset, you might get a 70% accuracy score. Getting that up to 90%+ or even 99% for the production model involves assessing and improving the quality of the labels and annotations. Here’s what you need from a dataset that should produce the results you’re looking for: Accurately labeled and annotated objects within images and videos; Data that’s not missing any labels; Including labels and annotations that cover data outliers, and every edge case; Balanced data that covers the distribution of images and videos in the deployment environment, such as different lighting conditions, times of day, seasons, etc.); A continuous data feedback loop, so that data drift issues are reduced, quality keeps increasing, bias reduces, and accuracy improves to ensure that a model can be put into production. Now let’s consider five ways you can improve the quality of your labeled data. Five Ways To Improve The Quality of Your Labeled Data Use Complex Ontological Structures For Your Labels Machine learning models require high-quality data annotation and labels as a result of your project’s labeling process. Achieving the results you want often involves using complex ontological structures for your labels, providing that's what is required — not simply for the sake of it. Simplified ontological structures aren’t very helpful for computer vision models. Whereas, when you use more complex ontological structures for the data annotation labeling process, it’s easier to accurately classify, label, and outline the relationship between objects in images and videos. With clear definitions, applied through the ontological structure, of objects within images and videos, those implementing the data annotation labeling process can produce more accurate labels. In turn, this produces better, more accurate outcomes for production-ready computer vision models. Example of a complex ontology in Encord AI-Assisted Labeling A wholly manual data labeling process is a time-consuming and exhausting task. It can cause annotators to make mistakes, burn out (especially when they’re applying the same labels over and over again), and for quality to go down. One of the best ways to accelerate the timescale it takes to label and annotate a dataset is to use artificial intelligence (AI-assisted) labeling tools. AI-assisted labeling, such as the use of automation workflow tools in the data annotation process is an integral part of creating training datasets. AI-assisted labeling tools come in all shapes and sizes. From open source out-of-the-box software, to proprietary, premium, AI-based tools, and everything in between. AI solutions save time and money. Efficiency and quality increase when you use AI-assisted tools, producing high-quality datasets more consistently, reducing errors, and improving accuracy. One such tool is Encord’s micro-models, that are “annotation specific models overtrained to a particular task or particular piece of data.” Encord also comes with a wide range of AI-assisted labeling tools and solutions, and we will cover those in more detail at the end of this article. Identify Badly Labeled Data Badly labeled, mislabelled, or data with missing labels will always cause problems for computer vision models. The best way to avoid any of these issues is to ensure labels are applied accurately during the data annotation process. However, we know that isn’t always possible. Mistakes happen. Especially when a team of outsourced annotators are labeling tens of thousands of images or videos. Not every annotator is going to do a perfect job every single day. Some will be better than others. Quality will vary, even when annotators have access to AI-assisted labeling tools. Consequently, to ensure your project gets the highest-quality annotated and labeled datasets possible, you need to implement an expert review workflow and quality assurance system. An additional way to ensure label and data quality is to use Encord Active, an open-source active learning framework to identify errors and poorly labeled data. Once errors and badly labeled images and videos have been identified, the relevant images or videos (or entire datasets) can be sent back to be re-annotated, or your machine learning team can make the necessary changes before introducing the dataset to the computer vision model. Identifying badly labeled images in Encord Active Improve Annotator Management Reducing the number of errors at the quality assurance end of the data pipeline involves improving annotator management throughout the project. Even when you’re working with an outsourced team in another country, distance, language barriers, and timezones shouldn’t negatively impact your project. Poor management processes will produce poor dataset quality outcomes. Project leaders need continuous visibility of inputs, outputs, and how individuals on the annotation team are performing. You need to assess the quality of data annotations and labels coming out of the annotation work, so that you can see who’s achieving key performance indicators (KPIs), and who isn’t. With the right AI-assisted data labeling tools, you should have a project dashboard at your fingertips. Not only should this provide access control, but it should give you a clear overview of how the annotation work is progressing, so that changes can be made during the project. This way, it should be easier to judge the quality of the labels and annotations coming from the annotation team, to ensure the highest quality and accuracy possible. Use Encord to Improve The Quality of Your Computer Vision Data Labels Encord is a powerful platform that pioneering AI teams across numerous sectors use to improve the quality, accuracy, and efficiency of computer vision datasets. Encord comes with everything, from advanced video annotation to an easy-to-use labeling interface, and automated object tracking, interpolation, and AI-assisted labeling. It comes with a dashboard, and a customizable toolkit to equip an annotation and machine learning team to label images and videos, and then implement a production-ready computer vision model. With Encord, you can find and fix machine learning models and data problems. Reduce the number of errors that come out of an annotation project, and then further refine a dataset to produce the results you need. We are transforming the speed and ways in which businesses are getting their models into production faster. And there we go, the 5 ways you can improve the quality of your labeled data.
Jan 20 2023
8 M
Best Practice Guide for Computer Vision Data Operations Teams
In most cases, successful outcomes from training computer vision models, and producing the results that project leaders want, comes down to a lot of problem-solving, trial-and-error, and the unsung heroes of the profession, data operations teams. Data operations play an integral role in creating computer vision artificial intelligence (AI) models that are used to analyze and interpret image or video-based datasets. And the work of data ops teams is very distinct from that of machine learning operations (MLOps). Without high-quality data, ML models won’t generate results, and it’s data operations and annotation teams that ensure the right data is being fed into CV models and the process for doing so runs smoothly and efficiently. In this article, we review the importance of data operations in computer vision projects; the role data ops teams play, and 10 best practice guidelines and principles for effective data operations teams. What’s the Role of Data Operations in Computer Vision Projects? Data operations for computer vision projects oversee and are responsible for a wide range of roles and responsibilities. Every team is configured differently, of course, and some of these tasks could be outsourced with an in-house team member to manage them. However, generally speaking, we can sum up the work of data operations teams in several ways: Dataset sourcing. Depending on the project and sector, these could be free, open-source datasets or proprietary data that is purchased or sourced specifically for the organization. Data cleaning tasks. Although this might be done by a sub-team or an outsourced provider for the data ops team, data ops are ultimately responsible for ensuring the datasets are “clean” for computer vision models. Clean visual data must be available before annotation and labeling work can start. Data cleaning involves removing corrupted or duplicate images and fixing numerous problems with video datasets, such as corrupted files, duplicate frames, ghost frames, variable frame rates, and other sometimes unknown and unexpected problems. Read more about: How To: Data Cleaning For Computer Vision Machine Learning Implementing and overseeing the annotation and labeling of large-scale image or video datasets. For this task, most organizations either have an in-house team or outsource data labeling for creating machine learning models. It often involves large amounts of data, so is time-consuming and labor-intensive. As a result, making this as cost-effective as possible is essential, and this is usually achieved through automation, using AI-powered tools, or strategies such as semi-supervised or self-supervised learning. Once the basic frameworks of a data pipeline are established (sourcing the data, data cleaning, annotation, data label ontologies, and labeling), a data operations team manages this pipeline. Ensuring the right quality control (QC), quality assurance (QA), and compliance processes are in place is vital to maintaining the highest data quality levels and optimizing the experimentation and training stages of building a CV model. During the training stage, maintaining a high-quality, clean, and efficient data pipeline is essential. Data ops teams also need to ensure the right tools are being used (e.g., open-source annotation software, or proprietary platforms, ideally with API access), and that storage solutions are scalable to handle the volume of data the project requires. Data operations teams also check models for bias, bugs, and errors, and see which perform in line with or above expectations, use data approximation or augmentation where needed, and help prepare a model to go from the training stage into production mode. Does Our Computer Vision Project Need a Data Operations Team? In most cases, commercial computer vision projects need and would benefit from creating a data operations team. Any project that’s going to handle large volumes of data, and will involve an extensive amount of cleaning, annotation, and testing, would benefit from a data ops team handling everything ML engineers and data scientists can’t manage. Remember, data scientists and ML engineers are specialists. Project managers don’t want highly-trained specialists invoicing their time (or requesting overtime) because you’ve not got the resources to take care of everything that should be done before data scientists and ML engineers get involved. High-performance computer vision (and other AI or ML-based) models that data science teams are training and putting into production are only as effective as the quality and quantity of the data, labels, and annotations that it’s being given. Without a team to manage, clean, annotate, automate, and perfect it, the data will be of poorer quality, impacting a model’s performance and outputs. Managing the pipeline and processes to ensure new training data can be sourced and fed into the model is essential to the smooth running of a computer vision project, and for that, you need a data operations team. Read more about: 5 Strategies To Build Successful Data Labeling Operations How Data Operations Improve & Accelerate CV Model Development and Training Data operations play a mission-critical role in model development because they manage labor-intensive, manual, and semi-automatic tasks between the ML/data science and the annotation teams. DataOps perform a cross-functional bridge, handling everything that makes a project run smoothly, including managing data sourcing (either open-source or proprietary image and video datasets, as required for the CV project), cleaning, annotation, and labeling. Otherwise, data admin and operations would fall on the shoulders of the machine learning team. In turn, that would reduce the efficiency and bandwidth of that team because they’d be too busy with data admin, cleaning, annotations, and operational tasks. 10 Data Operations Principles & Best Practices for Computer Vision Here are 10 practical data operations principles and best practice guidelines for computer vision. Build Data Workflows & Active Learning Pipelines before a Project Starts Implementing effective data workflow processes before a project starts, not during, is mission-critical. Otherwise, you risk having a data pipeline that falls apart as soon as data starts flowing through it. Have clear processes in place. Leverage the right tools. Ensure you’ve got the team(s), assets, budget, senior leadership support, and resources ready to handle the project starting. DataOps, Workflow, Labeling, and Annotation Tools: Buy Don’t Build When it comes to data operations and annotation tools, the cost of developing an in-house solution compared to buying is massive. It can also take anywhere from 6 to 12 months or more, and this would have to be factored in before a project could start. It’s several orders of magnitude more expensive to build data ops and annotation tools, especially when there are so many powerful and effective options on the market. Some of those are open-source; however, many don’t do everything that commercial data ops teams require. Commercial tools are massively more cost-effective, scalable, and flexible than building your own in-house software while delivering what commercial data ops teams need better than open-source options. It’s also worth noting that several are specifically tailored to achieve the needs of certain use cases, such as collaborative annotating tooling for clinical data ops teams and radiologists. Having a computer vision platform that’s HIPAA and SOC 2 compliant is a distinct advantage, especially when you’re handling sensitive data. We go into more detail about selecting the right tool, software, or platform for the project further down this article. Implement DataOps Using Software Development Lifecycle Strategies One of the most effective ways to build a successful and highly-functional data operation is to use software development lifecycle strategies, such as: Continuous integration and delivery (CI/CD); Version control (e.g., using Git to track changes); Code reviews; Unit testing; Artifacts management; Release automation. Plus, any other software development strategies and approaches that make sense for the project, the software/tools you’re using, and datasets. For data ops teams, using software development principles is a smart strategic and operational move, especially since data engineers, scientists, and analysts are used to code-intensive tasks. Automate and Orchestrate Data Flows The more a data ops team can do to automate and orchestrate data flows, annotation, and quality assurance workflows, the more effectively a computer vision project can be managed. Read more about: How to Create Workflows in Encord One of the best ways to achieve this is to automate deployments with a Continuous integration and delivery (CI/CD) pipeline. Numerous tools can help you do this while reducing the amount of manual data wrangling required. Continuous Testing of Data Quality & Labels Testing the accuracy and quality of image or video-based labels and annotations is essential throughout computer vision projects. Having a quality control/quality assurance workflow will ensure that projects run more smoothly and label outputs meet the project's quality metrics. Data operations teams can put systems and processes in place, such as active learning pipelines and debugging tools, to continually assess the quality of the labels and annotations an annotation team creates. Read more about: An Introduction to Quality Metrics in Computer Vision Ensure Transparent Observability As part of the quality control and assurance process, having transparent metrics and workflows is important for everyone involved in the project. This way, leaders can oversee everything they need, and other data stakeholders can observe and provide input as required. One of the best ways to do that is with a powerful dashboard, giving data ops leaders the tools they need to implement an effective quality control process and active learning workflows. Deliver Value through Data Label Semantics For DataOps to drive value quickly, and to ensure that annotation teams (especially when they’re outsourced), it helps everyone involved to build a common and shared data, metadata, and c. In other words, make sure everyone is on the same page when it comes to the labels and annotations being applied to the datasets. Providing this is done early into a computer vision project, you can even pre-label images and videos so that when batches of the datasets are assigned to annotation teams, they’re clearer on the direction they need to take. Create Collaboration Between Data Stakeholders Another valuable principle is to establish collaboration between cross-functional data stakeholders. Similar to the agile principle in software development, when data and workflows are embedded throughout, it removes bottlenecks and ensures that everyone works together to solve problems more effectively. This way, data operations can ensure the computer vision project is aligned with overall operational and business objectives while ensuring every team involved works well together. Data quality summary in Encord Treat Data as an Intellectual Property (IP) Asset Data ops, machine learning, and computer vision teams need to treat datasets as an integral part of your organizations and project's intellectual property (IP). Rather than treating it as an afterthought or simply material that gets fed into an AI model. The datasets you use, and annotations and labels applied to the images and videos, make them unique; integral to the success of your project. Take every step to protect this IP, safeguarding it from data theft and ensuring data integrity and compliance is maintained throughout. Have a clear data audit trail so that you know who’s worked on every image or video, with timestamps and metadata. An audit trail also makes data regulation and compliance easier to achieve, especially in healthcare, if you’re aiming to achieve FDA compliance. Pick the Most Powerful, Feature-rich, and Functional Labeling & Annotation Tools Picking the most powerful labeling and annotation tools is integral to the success of data ops teams and, therefore, the whole project. There are open-source tools, low-code/no-code solutions, and powerful commercial platforms. In some cases, the tool you use depends on the use case. However, in most cases, the best tools are use case agnostic and accelerate the success of projects with extensive and powerful automation features. Encord and Encord Active are two such solutions. Encord improves the efficiency of labeling data and managing a team of annotators. Encord Active is an open-source active learning framework for computer vision: a test suite for your labels, data, and models. Having the right tools is a big asset for data operations teams. It’s the best way to ensure everything runs more smoothly and the right results are achieved within the timescale that project leaders and senior stakeholders require. Conclusion: Advantages of an Effective Data Operations Team A data operations team that’s performing well is organized, operationally efficient, and focused on producing high-quality and accurate image or video-based datasets, labels, and annotations. Beyond overseeing the annotation workflows, quality control, assurance, and data integrity and compliance are usually within the remit of a data ops team. To achieve the best results, data ops teams need to ensure those doing the annotation work have the right tools. Software that comes with a range of tools for applying labels and annotations, a collaborative dashboard to oversee the work, and an efficient data audit, security, and compliance framework are essential too. Ready to improve the performance of your computer vision models? Sign-up for an Encord Free Trial: The Active Learning Platform for Computer Vision, used by the world’s leading computer vision teams. AI-assisted labeling, model training & diagnostics, find & fix dataset errors and biases, all in one collaborative active learning platform, to get to production AI faster. Try Encord for Free Today. Want to stay updated? Follow us on Twitter and LinkedIn for more content on computer vision, training data, and active learning. Join our Discord Channel to chat and connect.
Dec 21 2022
10 M
How to Improve the Accuracy of Computer Vision Models
Accuracy is crucial when training computer vision models. Accuracy rests on three core pillars: The quality, volume, and cleanliness (how clean it is) of the imaging or video datasets that will be annotated, labeled, and used in a computer vision model; The experimentation and training process used to train a computer vision (CV) or machine learning (ML) model; The workflow, annotation tools, automation features, dashboard, quality control (QC), and quality assurance (QA) processes can have a huge positive impact on iterative training outcomes when building an algorithmic-based model. In this article, we bring together the most effective best practice guidelines for those who are training computer vision models and are tasked with improving the accuracy and performance of the models, to get them from proof of concept (POC) to a working production-ready model. How to Source Datasets for Computer Vision Models? As we’ve covered in previous articles, there are numerous ways to source datasets for computer vision models. You can use your own data if you have it, or you can go out and find an open-source dataset that is ready to feed into a CV or ML-based model. If you are looking for open-source image or video datasets, there’s a wealth of options for an extensive range of sectors in this article: where to find the best open-source datasets for machine learning models, including where you can find them depending on your sector/use cases. Tutorial: What Are The Best Datasets For Machine Learning? Open-source datasets for computer vision models aren’t difficult to source, and they’re free! It’s more challenging finding proprietary datasets that you can buy or source cheaply, especially when large volumes of data are needed to train an artificial intelligence model. It’s even more difficult to get these in the medical and healthcare sector because even though hospitals and medical providers sell data, it needs to meet data compliance requirements and be free from individual patient identifiers (or these need to be scrubbed from the images or videos during the cleaning process). However, once you’ve got data you can use, there’s a detailed process to work through before those datasets can get anywhere near a production-ready computer vision model. The process involves: Data cleaning; Labeling and annotating the images or videos in the dataset (automation and having the right tools can accelerate this crucial part of the process); Experiments and training using the annotated datasets; Sufficient iterations on the datasets and during the model training process to put a POC model into production, to start generating the results and outcome the project needs. Here’s more information about how to improve datasets for computer vision models. Before any of that can happen, the first part of the process is data cleaning. A thankless and labor-intensive task that every dataset needs to go through before annotation and labeling work can start. Even if you use an open-source dataset, a certain amount of cleaning is usually required. Why is Data Cleaning Crucial for Machine Learning Experiments and Training? Clean data is essential for successful computer vision and machine learning experiments, training, and models. Unclean data is expensive, costing time and money. According to an IBM estimate published in the Harvard Business Review (HBR), unclean and poor-quality data costs the world $3.1 trillion. Cleaning data contained within spreadsheets costs tens of thousands of dollars. Whereas, cleaning image and video-based data costs even more, as the work is considerably more time-consuming, and getting it right the first time is essential if you want to produce an accurate computer vision model. To avoid challenges further down the road, you need to clean your video or image data before using it to train your machine learning model. One way you can do this is by matching your dataset against a well-known open-source dataset that includes images of similar objects. When your data has been bought or sourced for a project a certain amount of data cleaning is usually necessary. The trick is to automate this as much as possible to reduce the time and cost of data cleaning. Here’s a tutorial about how to do data cleaning for computer vision models. Cleaning images involves removing duplicate or corrupt files, and enhancing or reducing the brightness and pixelation of images. Medical images are more complex to clean as there are numerous layers to file formats (such as DICOM). And then when it comes to videos, you’ve got to remove and tidy up corrupted files, duplicate frames, ghost frames, variable frame rates, and other sometimes unknown and unexpected problems. Once the images or videos are ready, and the annotation and labeling work has started, a quality control (QC) and quality assurance (QA) workflow process are mission-critical to ensure the quality and accuracy of the labels before you can start training a computer vision model. How to Improve Dataset Annotation and Labels for Greater Accuracy In computer vision, dataset annotation and labeling are critical part of the process. It’s often said that you can have the best algorithm in the world but if your dataset lacks quality and volume then your machine-learning model will suffer. When creating datasets ready for training and machine learning experiments, you need to ensure they’re diverse enough to reflect every aspect of the variety of objects within the dataset, to reduce bias For example, if you want to create an annotation label for types of cars, don't just include pictures of Lamborghinis and Ferraris — you need images with numerous different and relevant makes, models, and colors so that your algorithm can learn how to identify cars accurately regardless of their color, make, or model. Having the right tools for dataset annotation and labeling improves the accuracy, annotation process, and project outcomes. Tools such as Encord gives data annotation teams the label and annotation formats they need, the ability to upload files in a native format and give project leaders the overview and workflow features they require to ensure a project runs smoothly. It’s especially useful in medical imaging or other specialist settings to have a tool that is built for and works well with native file formats, such as DICOM and NIfTI. Encord has developed our medical imaging dataset annotation software in close collaboration with medical professionals and healthcare data scientists. For those in the medical profession, here’s how to improve medical imaging machine-learning experiments. Labels and annotations need to be run through a quality control process before experiments and training can start. Otherwise, you risk putting poor-quality data into a model that will only generate poor-quality results. Next, you need to run experiments to train your computer vision model to improve performance and accuracy. Why Do You Need to Run Experiments for Computer Vision Models? Experiments are an integral part of creating and building working computer vision models. Experiments are used to: Improve performance: You will need to improve model performance by running experiments and analyzing its results. Improve the model: You can use an experiment to improve your model by gathering data about its behavior and changing it accordingly, making it more accurate, robust, or efficient at solving a particular problem. Improve the training dataset: By running an experiment on a range of labeled images with different classes (e.g., cats vs dogs), one could gather information about how well each annotation and label class works when given different datasets as training inputs. For example, you might need more images under different light conditions, showing daytime and nighttime images, and different breeds of cats and dogs. How to Train Your Model to Increase Performance and Accuracy The next step is to train your model and assess its performance. When you’re training a model, it will learn from the data you feed into it. Failure is an inevitable and necessary part of the training process for machine learning and computer vision models. To start with, expect an accuracy rating of around 70%. Don’t worry. The aim is to keep iterating, keep improving the volume of data, and labels and annotations within the images and videos until the accuracy rating reaches 90%+. It will happen. It takes time, but your ML or data ops team will get there. You can also use a benchmarking dataset for evaluation purposes—this means that after training your model, you run it against a benchmark dataset to see how well your computer vision model performs compared with what was expected for accuracy and the false positive rate. Do You Need to Create Artificial Images or Video Content? Artificially-generated content can help test the algorithm because it allows you to see how well it performs when presented with different situations or scenarios in which there are no (or not enough) real-world examples available from which it can learn from. For example, you might not have enough images or videos of car crashes, and yet that’s what you need for your ML model. What can you do? You can source artificially-generated content in several ways. It’s especially useful when the volume of images or videos for a particular use case won’t be enough to accurately train a computer vision model. Computer-generated images (CGI) 3D games engines — such as Unity and Unreal — and Generative adversarial networks (GANs) are ideal sources for creating images or videos that are high-quality enough to train a CV model. Quality and quantity are important factors; hence the need to use artificial or synthetic images and videos to train computer vision models. For more information, here’s An Introduction to Synthetic Training Data Now let’s take a closer look at how to improve computer vision model experiment workflows. How to Improve Computer Vision Model Experiment Workflows Improving the accuracy of your computer vision model is not only about understanding what works, but also how to improve the process of experimenting with different machine learning models and parameters. The best way to do this is by using tools that allow you to quickly try out new ideas and test them on a dataset. With tools such as Encord and Encord Active, you can quickly improve the quality of labels and annotations, and the associated workflow and quality process management. Using a dashboard, data ops managers can oversee annotation and training workflows more effectively, ask for more accurately labeled datasets, introduce data augmentation, and reduce bias. Now it’s simply a case of training and re-training the model until the desired results are being achieved consistently, and then you can put a working model into production to solve the problem that needs solving. Ready to improve your computer vision workflows? Sign-up for an Encord Free Trial: The Active Learning Platform for Computer Vision, used by the world’s leading computer vision teams. AI-assisted labeling, model training & diagnostics, find & fix dataset errors and biases, all in one collaborative active learning platform, to get to production AI faster. Try Encord for Free Today. Want to stay updated? Follow us on Twitter and LinkedIn for more content on computer vision, training data, and active learning.
Dec 19 2022
5 M
The Complete Guide to Data Annotation [2024 Review]
Data annotation is integral to the process of training a machine learning (ML) or computer vision model (CV). Datasets often include many thousands of images, videos, or both, and before an algorithmic-based model can be trained, these images or videos need to be labeled and annotated accurately. Creating training datasets is a widely used process across dozens of sectors, from healthcare to manufacturing, to smart cities and national defense projects. In the medical sector, annotation teams are labeling and annotating medical images (usually delivered as X-rays, DICOM, or NIfTI files) to accurately identify diseases and other medical issues. With satellite images (usually delivered in the Synthetic Aperture Radar format), annotators could be spending time identifying coastal erosion and other signs of human damage to the planet. In every use case, data labeling and annotation are designed to ensure images and videos are labeled according to the project outcome, goals, objectives, and what the training model needs to learn before it can be put into production. In this article, we cover the complete guide to data annotation, including the different types of data annotation, use cases, and how to annotate images and videos. What is Data Annotation? Data annotation is the process of taking raw images and videos within datasets and applying labels and annotations to describe the content of the datasets. Machine learning algorithms can’t see. It doesn’t matter how smart they are. We, human annotators and annotation teams, need to show AI models (artificial intelligence) what’s in the images and videos within a dataset. Annotations and labels are the methods that are used to show, explain, and describe the content of image and video-based datasets. This is the way models are trained for an AI project; how they learn to extrapolate and interpret the content of images and videos across an entire dataset. With enough iterations of the training process (where more data is fed into the model until it starts generating the sort of results, at the level of accuracy required), accuracy increases, and a model gets closer to achieving the project outcomes when it goes into the production phase. At the start, the first group of annotated images and videos might produce an accuracy score of around 70%. Naturally, the aim is to increase and improve that, and therefore more training data is required to further train the model. Another key consideration is data-quality - the data has to be labeled as clearly and accurately as possible to get the best results out of the model. Image segementation in Encord What’s AI-assisted Annotation? Manual annotation is time-consuming. Especially when tens of thousands of images and videos need to be annotated and labeled within a dataset. As we’ve mentioned in this article, annotation in computer vision models always involves human teams. Fortunately, there are now tools with AI-labeling functionality to assist with the annotation process. Software and algorithms can dramatically accelerate annotation tasks, supporting the work of human annotation teams. You can use open-source tools, or premium customizable AI-based annotation tools that run on proprietary software, depending on your needs, budget, goals, and nature of the project. Human annotators are often still needed to draw bounding boxes or polygons and label objects within images. However, once that input and expertise is provided in the early stages of a project, annotation tools can take over the heavy lifting and apply those same labels and annotations throughout the dataset. Expert reviewers and quality assurance workflows are then required to check the work of these annotators to ensure they’re performing as expected and producing the results needed. Once enough of a dataset has been annotated and labeled, these images or videos can be fed into the CV or ML model to start training it on the data provided. What Are The Different Types of Data Annotation? There are numerous different ways to approach data annotation for images and videos. Before going into more detail on the different types of image and video annotation projects, we also need to consider image classification and the difference between that and annotation. Although classification and annotation are both used to organize and label images to create high-quality image data, the processes and applications involved are somewhat different. Classification is the act of automatically classifying objects in images or videos based on the groupings of pixels. Classification can either be “supervised” — with the support of human annotators, or “unsupervised” — done almost entirely with image labeling tools. Alongside classification, there is a range of approaches that can be used to annotate images and videos: Multi-Object Tracking (MOT) in video annotation for computer vision models, is a way to track multiple objects from frame to frame in videos once an object has been labeled. For example, it could be a series of cars moving from one frame to the next in a video dataset. Using MOT, an automated annotation feature, it’s easier to keep track of objects, even if they change speed, direction, or light levels change. Interpolation in automated video annotation is a way of filling in the gaps between keyframes in a video. Once labels and annotations have been applied at the start and end of a series of videos, interpolation is an automation tool that applies those labels throughout the rest of the video(s) to accelerate the process. Auto Object Segmentation and detection is another type of automated data annotation tool. You can use this for recognizing and localizing objects in images or videos with vector labels. Types of segmentation include instance segmentation and semantic segmentation. Model-assisted labeling (MAL) or AI-assisted labeling (AAL) is another way of saying that automated tools are used in the labeling process. It’s far more complex than applying ML to spreadsheets or other data sources, as the content itself is either moving, multi-layered (in the case of various medical imaging datasets) or involves numerous complex objects, increasing the volume of labels and annotations required. Human Pose Estimation (HPE) and tracking is another automation tool that improves human pose and movement tracking in videos for computer vision models. Bounding Boxes: A way to draw a box around an object in an image or video, and then label that object so that automation tools can track it and similar objects throughout a dataset. Polygons and Polylines: These are ways of drawing lines and labeling either static or moving objects within videos and images, such as a road or railway line. Keypoints and Primitives (aka skeleton templates): Keypoints are useful for pinpointing and identifying features of countless shapes and objects, such as the human face. Whereas, primitives, also known as skeleton templates are for specialized annotations to templatize specific shapes, e.g. 3D cuboids, or the human body. Of course, there are numerous other types of data annotations and labels that can be applied. However, these are amongst some of the most popular and widely used CV and ML models. How Do I Annotate an Image Dataset For Machine Learning? Annotation work is time-consuming, labor intensive, and often doesn’t require a huge amount of expertise. In most cases, manual image annotation tasks are implemented in developing countries and regions, with oversight from in-house expert teams in developed economies. Data operations and ML teams ensure annotation workflows are producing high-quality outputs. To ensure annotation tasks are complete on time and to the quality and accuracy standards required, automation tools often play a useful role in the process. Automation software ensures a much larger volume of images can be labeled and annotated, while also helping managers oversee the work of image annotation teams. Different Use Cases for Annotated Images Annotated images and image-based datasets are widely used in dozens of sectors, in computer vision and machine learning models, for everything from cancer detection to coastal erosion, to finding faults in manufacturing production lines. Annotated images are the raw material of any CV, ML, or AI-based model. How and why they’re used and the outcomes these images generate depends on the model being used, and the project goals and objectives. How Do I Annotate a Video Dataset For Machine Learning? Video annotation is somewhat more complicated. Images are static, even when there’s a layer of images and data, as is often the case with medical imaging files. However, videos are made up of thousands of frames, and within those moving frames are thousands of objects, most of which are moving. Light levels, backgrounds, and numerous other factors change within videos. Within that context, human annotators and automated tools are deployed to annotate and label objects within videos to train a machine learning model on the outputs of that annotation work. Different Use Cases for Annotated Videos Similar to annotated images, videos are the raw materials that train algorithmic models (AI, CV, ML, etc.) to interpret, understand, and analyze the content and context of video-based datasets. Annotated videos are used in dozens of sectors with thousands of practical commercial use cases, such as disease detection, smart cities, manufacturing, retail, and numerous others. At Encord, our active learning platform for computer vision is used by a wide range of sectors - including healthcare, manufacturing, utilities, and smart cities - to annotate 1000s of images and accelerate their computer vision model development. Experience Encord in action. Dramatically reduce manual video annotation tasks, generating massive savings and efficiencies. Sign-up for an Encord Free Trial: The Active Learning Platform for Computer Vision, used by the world’s leading computer vision teams. AI-assisted labeling, model training & diagnostics, find & fix dataset errors and biases, all in one collaborative active learning platform, to get to production AI faster. Try Encord for Free Today. Want to stay updated? Follow us on Twitter and LinkedIn for more content on computer vision, training data, and active learning. Join our Discord channel to chat and connect. FAQs: How to Annotate and Label Different Image and Video Datasets for Machine Learning How are DICOM and NIfTI Images Annotated for Machine Learning? DICOM and NIfTI images are two of the most widely used medical imaging formats. Both are annotated using human teams, supported by automated annotation tools and software. In the case of DICOM files, labels and annotations need to be applied across numerous layers of the images, to ensure the right level of accuracy is achieved. How are Medical Images Used in Machine Learning? In most cases, medical images are used in machine learning models to more accurately identify diseases, and viruses, and to further the medical professions' (and researchers') understanding of the human body and more complex edge cases. How are SAR (Synthetic Aperture Radar) Images Annotated for Machine Learning? SAR images (Synthetic Aperture Radar) come from satellites, such as the Copernicus Sentinel-1 mission of the European Space Agency (ESA) and the EU Copernicus Constellation. Private satellite providers also sell images, giving projects that need them a wide variety of sources of imaging datasets of the Earth from orbit. SAR images are labeled and annotated in the same way as other images before these datasets are fed into ML-based models to train them. What Are The Uses of SAR Images for Machine Learning? SAR images are used in machine learning models to advance our understanding of the human impact of climate change, human damage to the environment, and other environmental fields of research. SAR images also play a role in the shipping, logistics, and military sectors.
Dec 08 2022
10 M
Complete Guide to Open Source Data Annotation
Open-source annotation tools and software are widely used in the computer vision and machine learning sectors across hundreds of projects. In some cases, it can be an advantage to use an open-source tool, especially if a project or company is in the startup phase and there’s a limited budget for annotation work. Academic projects often find open-source tools useful, alongside the hundreds of open-source datasets (such as COCO). However, for commercial projects and use cases, there are downsides too. Open-source doesn’t always come with the tools and features machine learning and data operations teams need to manage projects effectively, efficiently, or at scale. In this article, we look at what open-source annotation tools are used for, provide more details on 5 of the most popular open-source tools, and then weigh up the pros and cons of using open-source tools, before comparing this to the option of using something more advanced. What is an Open-source Data Annotation Tool? An open-source data annotation tool is a piece of software that’s specifically designed for image labeling and data annotation for image and video datasets. Annotation is an essential part of training computer vision models, as labels and data annotations are required to train models to produce the results/outcomes that organizations need. Open-source tools are free to use. Anyone can download and use them, so there’s no license fee or monthly subscription to pay, unlike Software as a Service (SaaS) products. Open-source tools are usually maintained by a foundation, similar to a charity, through community donations, or with sponsorship from tech companies. What Would You Use an Open-source Labeling Tool For? Finding the right open-source tool isn’t always easy. It depends on what you need it for, whether this is for image labeling, video labeling, or both. Or whether you need an open-source labeling tool with specific functionality for certain use cases, such as annotating medical imaging datasets. As we’ve covered that topic in previous articles, in this post we are focusing on more widespread computer-vision-based image and video use cases, such as smart cities, manufacturing, security, and sports analytics. Open-source labeling tools are used for everything from image segmentation to drawing bounding boxes, polylines, object detection, and numerous other annotations and labels on images and videos. You can use open-source tools for human pose estimation (HPE), and dozens of other computer vision (CV) project use cases. Image annotation in Encord Now let’s take a look at 5 of the most popular open-source data annotation tools for computer vision projects. What Are The Main Open-source Data Annotation Tools? CVAT The Computer Vision Annotation Tool (CVAT) started as an internal Intel project in 2017. Now it’s an independent company and foundation, with over 1 million downloads of their open-source image and video annotation software, and a passionate community of supporters and contributors. With CVAT, you can annotate images and videos by creating classifications, segmentations, 3D cuboids, and skeleton templates. CVAT is used across the healthcare, retail, manufacturing, sports, automotive, and aerial observation (drones) sectors. CVAT is an open-source project supported by Intel, under the OpenCV umbrella, and is free to use commercially, thanks to the permissive MIT license. CVAT’s core team will work with the OpenCV team to support the project, and OpenCV will support those migrating from the original CVAT.org to its new home, at CVAT.ai. MONAI Label MONAI Label is an open-source image annotation tool that uses AI to automate annotation work. Although it’s primarily used in the medical and healthcare sectors, MONAI Label can be used for any kind of image annotation project. It’s an ecosystem that’s easy to install and can run locally on a machine with single or multiple GPUs. Both the server-side and client-side can work on the same or different machines, depending on what you need. LabelMe LabelMe is an open-source “online annotation tool to build image databases for computer vision research” that emerged from the MIT Computer Science and Artificial Intelligence Laboratory. LabelMe comes with the downloadable source code, a toolbox, an open-source version for 3D images, image datasets you can use for computer vision training projects, and the ability to outsource data labeling through Amazon Mechanical Turk. RIL-Contour RIL-Contour is another open-source annotation tool that accelerates annotation projects using iterative deep learning (IDL). It was primarily designed for medical imaging datasets but can be used for any kind of image-based dataset for computer vision and machine learning projects. RIL-Contour is an open-source project with over 1000 contributors, with the schema and framework originating from ELIXIR, the European Infrastructure for Biological Information. Sefexa Sefexa is an open-source image segmentation tool. Sefexa was created by Ales Fexa, a software engineer in Prague with a passion for computer vision and mathematics. With Sefexa, you can use it to semi-automate image segmentation in image-based datasets, analyze images and export the findings into Excel, and create ground truth data from the images in a dataset. Now let’s look at the pros and cons of using open-source annotation tools. What Are The Pros and Cons of Using Open-source Annotation Tools? Unfortunately, open-source tools come with several downsides, and here they are: Cons of Open-Source Annotation Tools Buying vs. Building: Sunk cost fallacy turned upside down As most founders know, there’s an advantage to buying instead of building as it ensures your engineering team is devoted to developing your product. Otherwise, your developers could spend far too much time building non-core in-house tech solutions when there are hundreds of options on the market. In the video and image annotation space, open-source solutions represent a potential answer to the challenge of annotating and labeling thousands of images and video datasets. However, this is one area where the ‘sunk cost fallacy’ gets turned on its head. Some companies use these open-source tools ‘straight out the box’, or as the basis for building an in-house version. Unfortunately, as we outline below, open-source tools come with far too many downsides compared to the advantages of buying off-the-shelf and customizable premium annotation solutions that aren’t weighed the disadvantages of open-source annotation tools. Difficult to scale annotation projects One of the foremost challenges is scaling annotation projects. Image and video annotation projects usually involve annotating thousands of images and videos. Every single one needs labels and suitable annotations, such as bounding boxes, polygons, polylines, object detection, HPE, and anything else required. Annotation tools automate this process as much as possible. Open-source tools often come with technical limitations. They can operate slower, making projects take longer, and even when open-source tools come with automation features, those features from commercial vendors are often faster, more efficient, and more effective. However, automation is only possible once human annotators have given annotation software something to work with. With commercial and feature-packed annotation tools, scaling these projects is much easier and less time-consuming. Everyone can see the whole team’s work and more importantly, project leaders can monitor annotators and scale up and down accordingly. With open-source software, annotation teams can only share image and video datasets via cloud-storage solutions such as Dropbox. Making it more difficult to scale annotation projects, and right now you don't need any more headaches when managing an annotation project. Weak or limited data security, no audit trails Data security and audit trails are integral to computer vision and machine learning projects. With open-source tools, there are no audit trails, and data security is weak or non-existent. Ensuring your project stays compliant with relevant data protection laws, such as GDPR in Europe, CE certification, or CCPA in the US is difficult without the ability to track and monitor a basic audit trail and timestamps on images and videos. Project leaders can’t monitor annotation teams Open-source tools don’t give annotators the ability to monitor the work of annotation teams as cost-effectively as premium software. Because open-source tools aren’t cloud-based, project leaders can’t monitor the progress of annotators in real-time. There are no dashboards, so you can’t see who’s done what, who is performing well, and who isn’t. Benchmarking performance takes a lot more time and effort. Collaboration is reliant on annotators sending completed batches of images and videos through cloud-based shared folders, such as Dropbox and Box. Annotation projects often take more time, especially if re-annotation and re-labeling are required, or accuracy is low. When projects are on a tight deadline and accurate training data is needed quickly, using an open-source tool could cost your team time you can’t afford to waste. Pros of Open-source annotation tools Free to download and use! One of the best, and main reasons to use open-source annotation tools is the price: they’re free! Annotation work is time-consuming. Getting your hands on any kind of tool that accelerates this work is a bonus, even more so if you don’t have to pay for it. For startups and academic projects, an open-source tool could be the right solution, especially when you’ve got to cover the budget for a team of annotators, and machine learning, computer vision, or data ops engineers to pay for too. When annotation budgets are tight, every penny helps. Adaptable and editable software Another advantage of open-source tools is they’re adaptable and editable. Open-source tools usually publish their source code and documentation, so if the tool doesn’t align with exactly what you need there are ways to adapt and modify it accordingly. Plus, you can use plugins, APIs, and other technical adaptations and workarounds to modify open-source software to your exact requirements. Community support Unlike proprietary and premium annotation software, where the support comes from the company, open-source projects are often surrounded by large and active communities. These are people who are either software users or have contributed to the development of the software. You can always count on these communities to answer any questions you might have, as others are likely to have encountered similar challenges during annotation and labeling projects. However, given the nature of the support from commercial tools, many would argue that this usually beats answers a community can provide, especially when you’re on a deadline and need a solution to a problem fast. When Should You Look at Using Commercial Annotation Tools? When we factor in the challenges of using open-source tools effectively, and efficiently, with the workflow oversight required, collaboratively, and at scale, there’s a good reason many project leaders turn to and prefer commercial software solutions. With solutions such as Encord, you benefit from an easy-to-use, collaborative interface. You need to be able to manage annotators in different countries and work with other teams as required. You can’t do this as easily when annotators have their own local version of the software and are sharing files using services such as Dropbox. Automation features are equally important. Automation features can save annotation teams a massive amount of time. For example, interpolation, which can match pixel data from one image to the next and ensure that annotators can draw interpolation labels in any direction is a huge time saver. Let’s face it, anything that can save annotation teams time is worth doing! A project dashboard with built-in quality control processes and features is equally useful. It’s essential for the smooth running of any annotation project. For project managers, this can make the difference between the success or failure of an annotation project. Audit trails and data compliance are equally valuable, especially in sectors with stringent levels of regulatory compliance to align with, such as healthcare and anything to do with defense. Wrapping up There are numerous advantages to using open-source tools. Especially for startups and academic projects. In a commercial scenario, an open-source tool could be a good starting point for developing your own in-house proprietary annotation solution or deciding what you need when buying an off-the-shelf solution. Although if you want to save time, buying is always the quickest route, compared to building! Despite certain downsides, open-source annotation tools will continue to be popular and evolve to adapt to the changing needs of the market, businesses, and organizations that require annotation software for video and imaging datasets. Ready to automate and improve the quality of your data labeling? Sign-up for an Encord Free Trial: The Active Learning Platform for Computer Vision, used by the world’s leading computer vision teams. AI-assisted labeling, model training & diagnostics, find & fix dataset errors and biases, all in one collaborative active learning platform, to get to production AI faster. Try Encord for Free Today. Want to stay updated? Follow us on Twitter and LinkedIn for more content on computer vision, training data, and active learning.
Dec 07 2022
5 M
How to use Action Classifications In Video Annotation
In almost every video some objects move. A car could be moving from frame to frame, but static annotations limit the amount of data machine learning teams can train a model on. Hence the need for action classifications in video annotation projects. With action, dynamic or events-based classification, video annotation teams can add a richer layer of data for computer vision machine learning models. Annotators can label whether a car is accelerating or decelerating, turning, stopping, starting, or reversing, and apply numerous other labels to a dynamic object. In this post, we will explain action classifications, also known as dynamic or event-based classification in video annotation in more detail, why this is difficult to implement, how it works, best practices, and use cases. {{try_encord}} What are Action Classifications in Video Annotation? Action, dynamic or event-based classification (also known as activity recognition) in video annotation is a time-dependent approach to annotation. Annotators need to apply action classifications to say what an object is doing and over what timescale those actions are taking place. With the right video annotation tool, you can apply these annotation labels so that an algorithm-generated machine-learning model has more data to learn from. This helps improve the overall quality of the dataset, and therefore, the outputs the model generates. For example, a car could be accelerating in frames 100 to 150, then decelerate in frames 300 to 350, and then turn left in frames 351 to 420. Dynamic classifiers contribute to the ground truth of a video annotation, and the video data a machine learning model learns from. Action or dynamic classifications are incredibly useful annotation and labeling tools, acting as an integral classifiers in the annotation process. However, dynamic classifications and labels are difficult to implement successfully. Very few video annotation platforms come with this feature. Encord does, and that’s why we’re going into more detail as to why dynamic or event classifications matter, how it works, best practices, and use cases. Action Classification vs. Static Classification: What’s the Difference? Before we do, let’s compare action with static classifications. With static classifications, annotators use an annotation tool to define and label the global properties of an object (e.g. the car is blue, has four wheels, and slight damage to the drivers-side door), and the ground truth of video data an ML is trained on. You can apply as much or as little detail as you need to train your computer vision model algorithm using static classifications and labels. On the other hand, action, or dynamic classifications, describe what an object is doing and when those actions take place. Action classifications are labels that are always inherently time and action-orientated. An object needs to be in motion, whether that’s a person, car, plane, train, or anything else that moves from frame to frame. An object’s behavior — whether that’s a person running, jumping, walking; a vehicle in motion, or anything else — defines and informs the labels and annotations applied during video annotation work and the object detection process. When annotated training datasets are fed into a computer vision or machine learning model, those dynamic labels and classifications influence the model’s outputs. Why are Action Classifications in Video Datasets Difficult to Implement? Action classifications are a truly innovative engineering achievement. Despite decades of work, academic research, and countless millions in funding for computer vision, machine learning, artificial intelligence (AI), and video annotation companies, most platforms don’t offer dynamic classification in an easy-to-implement format. Static classifications and labels are easier to do. Every video annotation tool and platform comes with static labeling features. Dynamic classification features are less common. Hence the advantage of finding an annotation tool that does static and dynamic, such as Encord. Action classifications require special features to apply dynamic data structures of object descriptions, to ensure a computer vision model understands this data accurately so that a moving car in one frame is tracked hundreds of frames later in the same video. How Does Action Classification for Video Data Work? Annotating and labeling movements aren’t easy. When an object is static, annotators give objects descriptive labels. Object detection is fairly simple for annotation tools. Static labels can be as simple as “red car”, or as complicated as describing the particular features of cancerous cells. On the other hand, dynamic labels and classifications can cover everything from simple movement descriptors to extremely detailed and granular descriptions. When we think about how people move, so many parts of the body are in motion at any one time. Hence the advantage of using keypoints and primitives (skeleton templates) when implementing human pose estimation (HPE) annotations; this is another form of dynamic classification when the movements themselves are dynamic. Therefore, annotations of human movement might need to involve an even higher level of granular detail. In a video of tennis players, notice the number of joints and muscles in action as a player hits a serve. In this one example, we can see that the players’ feet, legs, arms, neck, and head are all in motion. Every limb moves, and depending on what you’re training a computer vision model to understand, it means ensuring annotations cover as much detail as possible. How to Train Computer Vision Models on Action Classification Annotations? Answering this question comes down to understanding how much data a computer vision model needs, and whether any AI/ML-based model needs more data when the video annotations are dynamic. Unfortunately, there’s no clear answer to that question. It always depends on a number of factors, such as the model's objectives and project outcomes, interpolation applied, the volume, and quality of the training datasets, and the granularity of the dynamic labels and annotations applied. Any model is only as accurate as the data provided. The quality, detail, number of segmentations, and granularity of labels and annotations applied during the stage influence how well and fast computer vision models learning. And crucially, how accurate any model is before more data and further iterations of that data need to be fed into the model. As with any computer vision model, the more data you feed it, the more accurate it becomes. Providing a model with different versions of similar data — e.g. a red car moving fast in shadows, compared to a red car moving slowly in evening or morning light — the higher the accuracy of the training data. With the right video annotation tool, you can apply any object annotation type and label to an object that’s in motion — bounding boxes, polygons, polylines, keypoints, and primitives. Using Encord, you can annotate the localized version of any object — static and dynamic — regardless of the annotation type you deploy. Everything is conveniently accessible in one easy-to-use interface for annotators, and Encord tools can also be used through APIs and SDKs. Now let’s take a look at the best practices and use cases for action classifications in video annotation projects. Best Practices for Action Classifications in Video Use clean (raw) data Before starting any video-based annotation project, you need to ensure you’ve got a large enough quantity and quality of raw data (videos). Data cleansing is integral and essential to this process. Ensure low-quality or duplicate frames, such as ghost frames, are removed. Understand the dynamic properties video dataset annotations are trying to explain Once the videos are ready, annotation and ML teams need to be clear on what dynamic classification annotations are trying to explain. What are the outcomes you want to train a computer vision model for? How much detail should you include? Answering these questions will influence the granular level of detail annotators should apply to the training data, and subsequent requests ML teams make when more data is needed. Annotators might need to apply more segmentation to the videos or classify the pixels more accurately, especially when comparing against benchmark datasets. Understand the dynamic properties video dataset annotations are trying to explain Next, you need to ensure the labels and annotations being used align with the problem the project is trying to solve. Remember, the quality of the data — from the localized version of any object to the static or dynamic classifications applied — has a massive impact on the quality of the computer vision model outcomes. Projects often involve comparing model outcomes with benchmark video classification datasets. This way, machine learning team leaders can compare semantic metrics against benchmark models and machine learning algorithm outcomes. Go granular with annotation details, especially with interpolation, object detection, and segmentation Detail and context are crucial. Start with the simplest labels, and then go as granular as you need with the labels, annotations, specifications, segmentations, protocols, and metadata, right down to classifying individual pixels. This could involve as much detail as saying a car went from 25kmph to 30kmph in the space of 10 seconds. What Are The Use Cases for Action Classification in Video Annotation? Action classification in video annotation is useful across dozens of sectors, with countless practical applications already in use. In our experience, some of the most common rights now include computational models for autonomous driving, sports analytics, manufacturing, and smart cities. Key Takeaways for Using Action Classification in Video Annotation Any sector where movement is integral to video annotation and computer vision model projects can benefit from dynamic or events-based classifications. Action classifications give annotators and ML teams a valuable tool for classifying moving and time-based objects. Movement is one of the most difficult things to annotate and label. A powerful video annotation tool is needed, with dynamic classification features, to support annotators when events/time-based action needs to be accurately labeled. At Encord, our active learning platform for computer vision is used by a wide range of sectors - including healthcare, manufacturing, utilities, and smart cities - to annotate 1000s of videos and accelerate their computer vision model development. Speak to sales to request a trial of Encord Want to stay updated? Follow us on Twitter and LinkedIn for more content on computer vision, training data, and active learning. Join our Discord channel to chat and connect.
Nov 11 2022
8 M
4 Questions to Ask When Evaluating Training Data Pipelines
Building a scalable and secure data pipeline involves a lot of decision making. However, for many machine learning and data science teams, the first step in the process is deciding where to store their datasets. While the major cloud providers such as Google and AWS offer a lot of benefits, a computer vision project’s individual privacy and security considerations will determine the best storage solution for them. Whereas a medical artificial intelligence company operating in the United States might use a major cloud provider, a medical AI company working with EU patient data will only be able to use EU-based cloud providers to store that data. Likewise, companies that work with highly sensitive data, such as defense contractors, will have more specific storage requirements, often needing to store data on their own hard drives kept on the premises. At the same time, all data pipelines need to be secure with very high encryption standards. Storing on-premise comes with its own challenges because while the major cloud storage providers have best-in-class teams dedicated to security, a company with an on-premise system will need to have a top-notch, in-house IT team that stays up-to-date on security and system maintenance. Otherwise, the company’s in-house storage system could be vulnerable to cyber attacks. It’s a tough decision, influenced by many factors such as cost and compliance. However, as the first decision in building a secure and compliant data pipeline and related workflows, deciding where to store your data has implications for many other data-related decisions that follow, including which data products a company can use. Here are four questions that data scientists and machine learning teams working on machine learning models should ask when determining whether a data product fits with the data pipelines for their particular use case. Is the product agnostic about where data is stored? For machine learning teams working with sensitive datasets, data storage remains top-of-mind throughout the entire model development process. As the teams put together a data pipeline to feed their algorithms and train their models, there are a lot of off-the-shelf data products that can make the process easier. However, teams need to know that a data product can work seamlessly with their datasets regardless of where the data is stored. Encord’s customers often ask, “What do you do with our data? Where do you store it?” 6x Faster Model Development with Encord Encord helps you label your data 6x faster, create active learning pipelines and accelerate your model development. Try it free for 14 days They want to ensure that the data remains stored in the location of their choice while they use our product. That’s not a problem because our product is storage agnostic. A storage agnostic data product can integrate with any storage facilities, enabling machine learning teams to have the same seamless experience as if the data was stored in the product’s own cloud facility. With a storage agnostic product, a company can use the same product with multiple data storage providers. It doesn’t matter if a company is storing data on nich or regional cloud providers, such as Germany’s Open Telekoms, or on a global provider such as AWS. Similarly, it doesn’t matter if a computer vision company working with healthcare images stores some of those datasets on a PACS viewer and some at an on-prem facility. A storage agnostic data product can integrate with all of these systems. For most computer vision companies, building a multi-region, multi-cloud strategy is essential for long-term business growth. Working across different regions can provide companies with access to more clients and their machine learning teams with access to more and varied data. When a model trains on more data, it learns to make better predictions. In a similar vein, a model training for deployment in different regions will be better able to generalize to those specific regions when trained on the appropriate training datasets. Of course, gaining access to such regional datasets requires maintaining compliance with the data privacy and regulations of the governing jurisdiction, including those regarding data storage. That’s why storage agnostic products are so important. Storage-agnostic products make implementing multi-region, multi-cloud strategies possible. With these products, a company that works in multiple locations with multiple different storage buckets can build integrations for each storage location and maintain granular access to the data. By enabling a company to use the same product across multiple teams and localities, these products also save companies time, effort, and money by eliminating the need to search for new tools or train staff members on multiple tools. What if the product doesn’t already have integrations with one of my data storage providers? Once you’ve found a storage agnostic data product, the most important question a company can ask is: “How quickly can that tool be integrated with new kinds of clouds or storage facilities?” One benefit of storing data with a major cloud provider is that it’s easy for products to integrate with those platforms because so many companies use them. However, if your company opted for a regional or on-prem solution, integration may not already exist. Building end-to-end integrations can be difficult and time consuming. In general, the greater the uniqueness of a storage facility, the more complex the deployment, and the greater number of engineering hours required to build integrations. The greater the number of hours needed, the greater the cost. The aim of any data product should be to integrate as seamlessly as possible with all places that a company might store data for their computer vision applications. If an integration doesn’t already exist, then it should be easy for the data product’s team to build it and add it into the repertoire of integrations that the product offers and facilitates. We designed Encord to be storage agnostic, and we also architected the system so that we can build new integrations quickly and at a low cost. For instance, to stay compliant with data privacy laws, one of our customers needed to store their data on the German cloud provider Open Telekom. Our developers could build those integrations for the provider within a couple of days so that Encord fit seamlessly with their existing data pipeline while enabling the machine learning team to take full advantage of our platform and its features. Having a storage-agnostic product that can be altered quickly to integrate with multiple storage providers allows companies to build an expandable data pipeline. As their security and privacy needs change, they can continue to collect and store data at multiple locations– running the spectrum from Big Cloud to on-premise– without having to worry about whether the data product will work with new datasets stored in new locations. How does the product securely access my datasets for my computer vision model? Nothing is more important than data pipeline security. The data needs to be encrypted to a high standard and inaccessible except to authorized users. When companies pick a data tool, they need to know how they can grant the tool access to their data in a secure manner. A good solution to this problem is using a signed URL. With a signed URL a company can keep public access to the data shut off while allowing specific and approved external users to access and temporarily render the data without actually storing it. If our customer uses their own private cloud storage, our product never actually has to store the data, which means that our customers remain compliant with data privacy laws and their data remains secure. Another benefit of using granular data access control is that it only grants access to the specific data items that a data product needs to have access to. For instance, if a computer vision company is working across multiple hospitals, but they currently only need to label images from patients at one hospital, then they can grant Encord’s product access to only images from that one hospital as opposed to granting blanket access to every hospital in which they work. Granting permissions to datasets with this level of specificity helps further ensure data compliance and protection. Does the product allow the machine learning team to work with the datasets in a granular manner? Whenever possible, companies should buy off-the-shelf data tools rather than build them internally. However, off-the-shelf data products must work as well with a company’s data as if the company had built the product internally. Data products must have a flexible API that allows teams working on ML models to work with the data in the same ways as if the tool were built in-house for a custom purpose and as if the data were stored internally. Users need to be able to perform all the basic CRUD operations, manipulating the data and still allowing it to flow continuously and seamlessly through the pipeline. A flexible API that allows you to work with the data pipelines in this granular manner is an essential component for any data product. In addition to having a flexible API, Encord also has a Python SDK. By wrapping the Python SDK around the API, we’ve made certain operations easier for Python developers. By providing an open source SDK, Encord enables developers to customize the tool until it fits perfectly with their machine learning and data pipeline needs. With the right data products in place, data will flow fluidly through your data pipeline. With a strong data pipeline in place, you can more efficiently train your deep learning model, evaluate data quality, automate labelling and set up active learning pipelines, all of which ultimately decreases the time needed to build and deploy your models, getting you to production AI faster. Get in touch to see Encord in action and try it out for yourself! Where to next? “I want to start annotating” - Get a free trial of Encord here. "I want to get started right away" - You can find Encord Active on Github here or try the quickstart Python command from our documentation. "Can you show me an example first?" - Check out this Colab Notebook. If you want to support the project you can help us out by giving a Star on GitHub ⭐ Want to stay updated? Follow us on Twitter and LinkedIn for more content on computer vision, training data, and active learning. Join the Slack community to chat and connect.
Nov 11 2022
5 M
The Full Guide to Training Datasets for Machine Learning
Training data is the initial training dataset used to teach a machine learning or computer vision algorithm or model to process information. Algorithmic models, such as computer vision and AI models (artificial intelligence), use labeled images or videos, the raw data, to learn from and understand the information they’re being shown. These models continue to refine their performance ⏤ , improving their decision-making and confidence ⏤ as they encounter new data and build upon what they learned from the previous data. High-quality training data is the foundation of successful machine learning because the quality of the training data has a profound impact on any model’s development, performance, and accuracy. Training data is as crucial to the success of a production-ready model as the algorithms themselves because the quality and volume of the labeled training data directly influence the accuracy with which the model learns to identify the outcome it was designed to detect. Training data guides the model: it’s the textbook and raw material from which the model gains its foundational knowledge. It shows the model patterns and tells it what to look for. After data scientists train the model, it should be able to identify patterns in never-before-seen datasets based on the patterns it learned from the training data. Machine learning and AI-based models are the students. In this scenario, the teachers are human data scientists, data ops teams, and annotators. They’re turning the raw data into labeled data using data labeling tools. Like human students, machines perform better when they have well-curated and relevant examples to practice with and learn from. If a computer vision model is trained on unreliable or irrelevant data, well-designed models can become functionally useless. As the old artificial intelligence adage goes: “garbage in, garbage out”. How do we use a training dataset to train computer vision models? Two common types of machine learning models are supervised and unsupervised. Unsupervised learning is when annotation and data science teams feed data into a model without providing it specific instructions or feedback on its progress. The training data is raw, meaning there are no annotations or identifying labels within the images and videos provided. So, the computer vision model trains without human guidance and discovers patterns independently. Unsupervised models can cluster and identify patterns in data, but they can’t perform tasks with a desired outcome. For instance, a data scientist can’t feed unsupervised model images of animals and expect the model to group them by species: the model might identify a different pattern and group them by color instead. Machine learning engineers build supervised learning models when the desired outcomes are predetermined, such as identifying a tumor or changes in weather patterns. In supervised learning, a human provides the model with labeled data and then supervises the machine learning process, providing feedback on the model’s performance. Human-in-the-loop (HILT) is the process of humans continuing to work with the machine and help improve its performance. The first step is to curate and label the training data. One of the best ways to achieve this is by using data labeling tools, active learning pipelines, and AI-assisted tools to turn the raw material into a labeled dataset. Labeling data allows the data science and ops team to structure the data in a way that makes it readable to the model. Within the training data, specialists identify a target ⏤ outcome that a machine learning model is designed to predict ⏤ , and they annotate objects in images and videos by giving them labels. By labeling data, humans can point out important features in the images and videos (or any type of data) and ensure that the model focuses on those features rather than drawing incorrect conclusions about the data. Applying well-chosen labels is critical for guiding the model’s learning. For instance, if humans want a computer vision model to learn to identify different types of birds, then every bird that appears in the image training data needs to be labeled appropriately with a descriptive label. After data scientists begin training the model to predict the desired outcomes by feeding it the labeled data, the “humans-in-the-loop” check its outputs to determine whether the model is working successfully and accurately. Active learning pipelines take a similar, albeit more automated, approach. In the same way that teachers help students prepare for an exam, the annotators and data scientists make corrections and feed the data back to the model so that it can learn from any inaccuracies. By constantly validating the model’s predictions, humans can ensure that its learning moves in the correct direction. The model improves its performance through this continuous loop of feedback and practice. Once the machine has been sufficiently trained, data scientists will test the model’s performance at returning real-world predictions by feeding it never-before-seen “test data.” Test data is unlabelled because data scientists don’t use it to tune the model: they use it to confirm that the model is working accurately. If the model fails to produce the right outputs from the test data, then data scientists know it needs more training before predicting the desired outcome. What makes a good machine learning training dataset? Because machine learning is an interactive process, it’s vital that the training data is applicable and appropriately labeled for the use case. The curated data must be relevant to the problem the model is trying to solve. For instance, if a computer vision model is trying to identify bicycles, then the data must contain images of bicycles and, ideally, various types of bicycles. The cleanliness of the data also impacts the performance of a model. The model will make incorrect predictions if trained on corrupt or broken data or datasets with duplicate images. Lastly, as already discussed, the quality of the annotations has a tremendous effect on the quality of the training data. It’s one of the reasons labeling images is so time-consuming, and annotation teams are more effective when they have access to the right tools, such as Encord. Encord specializes in creating high-quality training data for downstream computer vision models with various powerful AI-backed tools. When organizations train their models on high-quality data, they increase the performance of their models in solving real-world business problems. Our platform has flexible ontology and easy-to-use annotation tools, so computer vision companies can create high-quality training data customized for their models without spending time and money building these tools in-house. What’s the best way to create an image or video-based dataset for machine learning? Creating, evaluating, and managing training data depends on having the right tools. Encord’s computer vision-first toolkit lets customers label any computer vision modality in one platform. We offer fast and intuitive collaboration tools to enrich your data so that you can build cutting-edge AI applications. Our platform automatically classifies objects, detects segments, and tracks objects in images and videos. Computer vision models must learn to distinguish between different aspects of pictures and videos, which requires them to process labeled data. The types of annotations they need to learn to vary depending on the task they’re performing. Let’s take a look at some common annotation tools for computer vision tasks. Image Classification: For single-label classification, each image in a dataset has one label, and the model outputs a single prediction for each image it encounters. In multi-label classification, each image has multiple labels which are not mutually exclusive. Bounding boxes: When performing object detection, computer vision models detect an object and its location, and the object’s shape doesn’t need to be detailed to achieve this outcome, which makes bounding boxes the ideal tool for this task. With a bounding box, the target object in the image is contained within a small rectangular box accompanied by a descriptive label. Polygons/Segments: When performing image segmentation, computer vision models use algorithms to separate objects in the image from both their backgrounds and other objects. Mapping labels to pixel elements belonging to the same image helps the model break down the digital images into subgroups called segments. The shape of these segments matters, so annotators need a tool that doesn’t restrict them to rectangles. With polygons, an annotator can create tight-knit outlines around the target object by plotting points on the image vertices. Encord’s platform provides annotation tools for a variety of computer vision tasks, and our tools are embedded in the platform, so users don’t have to jump through any hoops before accessing model-assisted labeling. Because the platform supports various data formats, including images, videos, SAR, satellite, thermal imaging, and DICOM images (X-Ray, CT, MRI, etc.), it works for a wide range of computer vision applications. Labeling training data for machine learning in Encord How to create better training datasets for your machine learning and computer vision models While there’s no shortage of data in the world, most of it is unlabelled and thus can’t actually be used in supervised machine learning models. Computer vision models, such as those designed for medical imaging or self-driving cars, need to be incredibly confident in their predictions, so they need to train on vast amounts of data. Acquiring large quantities of labeled data remains a serious obstacle to the advancement of AI. There are dozens of open-source datasets out there: Here’s a curated list of 10 of the best for computer vision projects. Because every incorrect label has a negative impact on a model’s performance, data annotators play a vital role in the process of creating high-quality training data. Hence the importance of quality assurance in the data labeling process workflow. Ideally, data annotators should be subject-matter experts in the domain for which the model is answering questions. In this scenario, the data annotators ⏤ , because of their domain expertise, ⏤ understand the connection between the data and the problem the machine is trying to solve, so their labels are more informative and accurate. Data labeling is a time-consuming and tedious process. For perspective, one hour of video data can take humans up to 800 hours to annotate. That creates a problem for industry experts who have other demands on their time. Should a doctor spend hundreds of hours labeling scans of tumors to teach a machine how to identify them? Or should a doctor prioritize doctor-human interaction and spend those hours providing care to the patients whose scans clearly showed malignancies? Data labeling can be outsourced, but doing so means losing the input of subject-matter experts, which could result in low-quality training data if the labeling requires any industry-specific knowledge. Another issue with outsourcing is that data labeling jobs are often in developing economies, and that scenario isn’t viable for any domain in which data security and privacy are important. When outsourcing isn’t possible, teams often build internal tools and use their in-house workforces to label their data manually, which leads to cumbersome data infrastructure and annotation tools that are expensive to maintain and challenging to scale. The current practice of manually labeled training data isn’t sufficient or sustainable. Using a unique technology called micro-models, Encord solves this problem and makes computer vision practical by reducing the burden of manual annotation and label review. Our platform automates data labeling, increasing its efficiency without sacrificing quality. Using micro-models to automate data labeling for machine learning Encord uses an innovative technology solution called micro-models to build its automation features. Micro-models allow for quick annotation in a “semi-supervised fashion”. In semi-supervised learning, data scientists feed machines a small amount of labeled data in combination with a large amount of unlabelled data during training. The micro-model methodology comes from the idea that a model can produce strong results when trained on a small set of purposefully selected and well-labeled data. Micro-models don’t differ from traditional models in terms of their architecture or parameters, but they have different domains of applications and use cases. A knee-jerk reaction from many data scientists might be that this goes against “good” data science because a micro-model is an overfit model. In an overfit model, the algorithm can’t separate the “signal” (the true underlying pattern data scientists wish to learn from the data) from the “noise” (irrelevant information or randomness in a dataset). An overfit model unintentionally memorizes the noise instead of finding the signal, meaning that it usually makes poor predictions when it encounters unseen data. Overfitting a production model is problematic because if a production model doesn't train on a lot of data that resembles real-world scenarios, then it won’t be able to generalize. For instance, if data scientists train a computer vision model on images of sedans alone, then the model might not be able to identify a truck as a vehicle. However, Encord’s micro-models are purposefully overfitted. They are annotation-specific models intentionally designed to look at one piece of data, identify one thing, and overtrain that specific task. They wouldn’t perform well on general problems, but we didn’t design them to apply to real-world production use cases. We designed them only for the specific purpose of automating data annotation. Micro-models can solve many different problems, but those problems must relate to the training data layer of model development. Comparing traditional and micro models for creating machine learning training data Because micro-models don’t take much time to build, require huge datasets, or need weeks to train, the humans in the loop can start training the micro-models after annotating only a handful of examples. Micro-models then automate the annotation process. The model begins training itself on a small set of labels and removes the human from much of the validation process. The human reviews a few examples, providing light-touch supervision, but mostly the model validates itself each time it retrains, getting better and better results. With automated data labeling, the number of labels that require human annotation decreases over time because the system gets more intelligent each time the model runs. When automating a comprehensive annotation process, Encord strings together multiple micro-models. It breaks each labeling task into a separate micro-model and then combines these models. For instance, to classify both airplanes and clouds in a dataset, a human would train one micro-model to identify planes, create and train another to identify clouds, and then chain them together to label both clouds and planes in the training data. Production models need massive amounts of labeled data, and the reliance on human annotation has limited their ability to go into production and “run in the wild.” Micro-models can change that. With micro-models, users can quickly create training data to feed into downstream computer vision models. Encord has worked with King’s College London (KCL) to make video annotations for computer vision projects 6x faster than previous methods and tools. Clinicians at KCL wanted to find a way to reduce the amount of time that highly-skilled medical professionals spent annotating videos of precancerous polyps for training data to develop AI-aided medical diagnostic tools. Using Encord’s micro-models and AI-assisted labeling tools, clinicians increased annotation output speeds, completing the task 6.4x faster than when manual labeling. In fact, only three percent of the datasets required manual labeling from clinicians. Encord’s technology not only saved the clinicians a lot of valuable time but also provided King’s College with access to training data much more quickly than had the institution relied on a manual annotation process. This increased efficiency allowed King’s College to move the AI into production faster, cutting model development time from one year to two months. Encord is a comprehensive AI-assisted platform for collaboratively annotating data, orchestrating active learning pipelines, fixing dataset errors, and diagnosing model errors & biases. Try it for free today.
Nov 11 2022
10 M
How to Automate Video Annotation for Machine Learning
Automated video labeling saves companies a lot of time and money by accelerating the speed and quality of manual video labeling, and eventually taking over the bulk of video annotation work. Once you start using machine learning and AI-based algorithms for video annotation — using large amounts of labeled videos — and ensuring those videos are accurately labeled is crucial to the success of the project. Generating labels manually during video annotation is highly laborious, time-consuming, costs a lot of money, and requires a whole team of people. Businesses and organizations often outsource this work to save costs. However, this rarely makes the task any quicker and can often cause problems with quality. Automated video annotation can solve most of these problems, reducing manual inputs, saving time and money, and ensuring you can annotate and label much larger datasets while maintaining consistent quality. In this post, we look at four ways to automate video annotation while ensuring the quality and consistency of your labels #1: Multi-Object Tracking (MOT) to Ensure Continuity from Frame to Frame Tracking objects automatically is a powerful automated video annotation feature. Once you’ve labeled an object, you want to ensure it’s tracked correctly and consistently from one frame to the next, especially if it’s moving and changing direction or speed. Or if the background and light levels change, such as a shift from day to night. Not only that but if you’ve labeled multiple objects, you need an AI-based video annotation tool capable of tracking every single one of them. The most powerful automated video labeling tool tracks pixels within an annotation from one frame to the next. This shouldn't be a problem even if you are tracking multiple objects with automatic annotation. Multi-object tracking is especially useful when processing videos through a machine learning automation tool and an asset when analyzing drone footage, surveillance videos, and in the healthcare and manufacturing sectors. Healthcare companies often need to annotate and analyze surgical or gastroenterology videos, whereas manufacturers need clearer, annotated videos of assembly lines. Automated object tracking for video annotation in Encord #2: Use Interpolation to Fill in the Gaps In automated video annotation or labeling, interpolation is the act of propagating labels between two keyframes. Say an annotation team has already manually labeled objects within hundreds of keyframes, using bounding boxes or polygons — at the start and end of a video. Interpolation accelerates the annotation process, filling in the details within the unannotated frames. However, you must use interpolation carefully, at least when starting out with a video annotation project. There’s always a trade-off between speed and quality. Dependent, of course, on the quality of the labels applied and the complexity of the labeling agents used during the model training stage. For example, a polygon applied to a complex multi-faceted object that’s moving from one frame to the next might not interpolate as easily as a simple object with a bounding box around it that’s moving slowly. As annotators know, this entirely depends on how much is changing in the video from one frame to the next. When polygons are drawn on an object in a video, supported by a proprietary algorithm that runs without a representational model, it can tighten the perimeter of the polygon, interpolate, and track the various segments (in this case, clothes) within a moving object, e.g., a person. Interpolation to support video annotation in Encord #3: Use Micro-Models to Accelerate AI-assisted Video Annotation In most cases, machine learning (ML) models and AI-based algorithms need vast amounts of data before they can produce meaningful results. Not only that, but the data going in should be clean and consistent. Otherwise, you risk the whole project taking much longer than anticipated or having to start over again. Automated video labeling and annotation are complicated. This method is also known as model-assisted labeling (MAL), or AI-assisted labeling (AAL). This type of labeling is far more complex than annotating static images or applying ML to vast Excel spreadsheets and other data sources. Conversely, micro-models are powerful, tightly-scoped approaches that over-fit data models to bootstrap your video annotation tasks. Training machine learning algorithms using micro-models is an iterative process that requires manual annotation and labeling at the start. However, you don’t need nearly as much manual work or time spent training the model as you would with other video annotation platforms. In some cases, you can train micro-models on as few as five labeled frames. As we outline in another post, “micro-models are annotation-specific models that are overtrained to a particular task or particular piece of data.” Micro-models are best applied to a narrow domain, e.g., automatically annotating particular objects throughout a long video, and the training data required is minimal. It can take minutes to train a micro-model and only minutes or hours to run through the development cycle. Micro-models save vast amounts of time and money for organizations in the healthcare, manufacturing, or research sectors, especially when annotating complex moving objects. #4: Auto Object Segmentation to Improve the Quality of Object Segments Auto-segmentation is drawing an outline around an object and then using an algorithm to automatically “snap” to the contours of the object, making the outline tighter and more accurately aligned with the object and label being tracked from one frame to the next. Annotators can do this using polygons. You might, for example, need to segment clothes a person is wearing in a surveillance video so that you can see when a suspect takes off an item of clothing to put something else on. With the right video annotation tool, auto object segmentation is applicable for almost any use case across dozens of sectors. It works on arbitrary shapes, and interpolation can track object segments across thousands of frames. In most cases, the outcome is a massive time and cost saving throughout a video annotation project, resulting in much faster and higher quality segmentations. Automated object segmentation in Encord The power of automated video annotation In our experience, there are very few cases where automatic video annotation can’t play a useful role during video annotation projects. Automation empowers annotators to work faster, more effectively, and deliver higher-quality project outputs. Experience Encord in action. Try out our automated video annotation features (including our proprietary micro-model approach). Sign-up for an Encord Free Trial: The Active Learning Platform for Computer Vision, used by the world’s leading computer vision teams. AI-assisted labeling, model training & diagnostics, find & fix dataset errors and biases, all in one collaborative active learning platform, to get to production AI faster. Try Encord for Free Today. Want to stay updated? Follow us on Twitter and LinkedIn for more content on computer vision, training data, and active learning. Join our Discord channel to chat and connect.
Nov 11 2022
6 M
5 Important Video Annotation Features
Labeling and annotating images is easy. Video annotation is not. Too many platforms focus on image annotation, throwing in video as an additional suite of features rather than implementing video-native tools for annotators. In this post, we outline the 5 features you need to maximize video annotation ROI and efficiencies so you can choose the right video annotation tool for your needs. Image vs. Video Annotation Video annotation is not the same as image annotation. You need a completely different — specialist, video-centric — a suite of tools and features to handle videos. Otherwise, data and video analyst teams are juggling multiple annotation platforms (which is something we see more often than you’d imagine) to achieve their objectives. As a leader or manager within an organization that needs a video annotation and labeling solution, you must ensure that the platform can effectively handle the specificities of video and image annotation. For example, within a large video — with a long runtime — you need to ensure the correct coordinates of objects that move from one frame to the next are aligned with the frame and timestamp the object first appeared. For several reasons, this doesn’t always happen with other tools, forcing companies to discard months’ worth of incorrectly labeled data. Let’s review the five most important features you need when considering which video annotation tool/platform to use. 5 Essential Video Labeling Software Features Advanced Video Handling Video annotation comes with dozens of challenges, such as variable frame rates, ghost frames, frame synchronization issues, and numerous others. To avoid these issues and ensure you don’t lose days of labeling activity, there are two things your video annotation platform needs: No limit to video length: Most video annotation software limits the length of videos, forcing you to cut them into shorter videos before annotation can start. With the best video annotation tools, you won’t have this problem - they should be able to handle arbitrarily long videos. Video pre-processing: Frame synchronization issues are a massive headache for video annotation teams, and there are numerous causes, such as the types of browsers being used for annotation work or variable frame rates at different points in a video. Effective pre-processing solves these challenges, ensuring a video is displayed properly and ready for annotation. Pre-processing means you avoid needing to re-label everything if there’s an issue with the video (e.g., sync frame issues, video not displayed properly, annotations are not matched with the proper frames, etc.), saving your annotation team countless hours and a lot of budgets at the start of a project. Easy-to-use Annotation Interface An easy-to-use video annotation and labeling interface ensures that annotators are productive. Video labeling and annotation shouldn’t take months, especially when annotating long videos. With this in mind, here are the key features you need to look out for to ensure your chosen annotation tool is easy to use: Navigation: When annotating long videos, a simple navigation tool is really important. Annotators need to be able to quickly find individual objects, move back and forth, and use labels to track specific objects as they move from frame to frame. Efficient manual annotation work: With an intuitive interface, annotators aren’t spending weeks getting to know the software. It should be easy to use by default. Hotkeys and other features make manual annotation work easier. Organizations can benefit from massive time, resource, and budget savings when annotators aren’t spending months on manual video labeling. Powerful annotation tooling: Annotation becomes a lot easier if you’ve got the right annotation types available to you. The main ones a video labeling tool should have are: Bounding Boxes: Drawing a bounding box is one way to label or classify an object in a video. It’s integral to the process of video annotation. With the best annotation tools, you should have the ability to draw a box around the object you want to label. For example, city planners designing a smart city could label moving cars and vehicles in videos when analyzing traffic movement around urban areas. A powerful and effective annotation tool should make it easy to maintain the same bounding box from frame to frame, tracking multiple objects in motion. Polygons are another annotation type, one you can draw free-hand. Add the relevant label and make polygons static or dynamic, depending on the annotated object. Static polygon annotations are useful when labeling cells or tumors in medical images. Polylines are equally useful, especially if you’re labeling something that is static itself, but moves from frame to frame, such as a road, railway line, or waterway. Keypoints outline or pinpoint the landmarks of specific shapes, such as a human face. Keypoint annotation is versatile and useful across countless shapes. Once you’ve highlighted the outline of a specific object, it can be tracked from frame to frame, making it easier for AI-based systems or manual annotation of the same object throughout the rest of a video or series of images. Primitives, also known as skeleton templates, are highly-useful for specialized annotations to templatize shapes (e.g., 3D cuboids, pose estimation skeletons, rotated bounding boxes, etc.). Annotation teams can use primitives or skeleton templates to outline an object, empowering them to track the object from one frame to the next. Primitives are especially useful in medical video annotation. Object tracking is a simple and powerful way of labeling a specific object, giving it a unique ID that you can use to track it throughout a video. Pixels from the object that’s been labeled are matched to pixels in the frames that come next, allowing a moving object — such as a car or person running — to be automatically tracked. Navigation features in the video annotation section of Encord Dynamic and Event-Based Classifications Another important feature of a great video annotation tool is the ability to classify frames and events. This gives you additional data for your model to work from - whether it was nighttime in the video or what the labeled object was doing at the time. Dynamic classifications are often called action or “event-based” classifications. The clue is in the name - they tell you what the object is doing - whether the car that you’re tracking is turning from left to right over a specific number of frames; hence these classifications are dynamic. It depends on what’s going on in the video and the granular level of detail you need to label. Dynamic or event-based classifications are a powerful feature that the best video annotation platforms come with, and you can use them regardless of the annotation type used to originally label the object in motion. Frame Classifications are different from specific object classifications. Instead of labeling or classifying an object, you use an annotation tool to organize a specific frame within a video. Hotkeys and video labeling menus can make it simple to select the start and end of a frame and then give that frame a label while annotating. A frame classification is used to highlight something happening in the frame itself - whether it is day or night or raining or sunny, for example. Automated Object Tracking, Interpolation & AI Assisted Labeling Annotation is a time-consuming, manual, data-intensive task. Especially when videos are long, complicated, or there are hundreds of videos to annotate. A solution is to automate video annotations. Automation leverages the skills of your annotation teams. It saves time and money while increasing the efficiency and the quality of the annotation work. Micro-Models are “annotation-specific models that are overtrained to a particular task or particular piece of data.” Encord’s video annotation tool is the only one that uses the micro-model approach, and it is ideal for bootstrapping automated video annotation projects. What’s special about micro-models is that they don’t need huge amounts of data. Quite the opposite; you can train micro-models within a few minutes. Once you’ve labeled the object or specific thing, person, or action within a video you want to track, powerful AI-generated algorithms do the rest. Active learning is often the best approach with micro-models, as it may take a few iterations for an algorithm to get it right. Organizations with large video annotation projects have found that micro-models give them a massive advantage. Automated Object Tracking is an evolution of the ability to label specific objects while doing video annotation. This might be challenging when using older or less powerful software. However, when you use software that comes with a proprietary algorithm that runs without using a representational model, you will save time when implementing automated object tracking. Interpolation can be implemented automatically when the right software comes with a linear interpolation algorithm designed with practical use cases in mind. Simply draw object vertices in arbitrary directions (e.g., clockwise, counterclockwise, and otherwise), and the algorithm will still track the same object as it moves from one frame to the next. Auto Object Segmentation is when you divide an object into multiple regions or a series of pixels without any constraints on the shape of those regions/pixels. For example, if an annotator has drawn a label boundary around a specific object — e.g., a cellular cluster being analyzed — the goal of auto-object segmentation is to tighten the edges so it fits more closely around the image in question. Algorithms can also track this image throughout the video automatically. Example of automated labeling using interpolation in Encord Annotation Team and Project Management Large annotation teams are difficult to manage. Whether you’re a Head of Machine Learning or Data Operations leader, you’ve got to juggle team management, budgets, operational timelines, and project outputs. Project leaders need visibility on what’s going on, being processed, and being analyzed. You need a clear understanding of the state of the project in real-time, giving you the ability to react fast if anything changes. When big-budget and long-timescale annotation projects are underway, it’s often useful to leverage external annotation teams to implement labor-intensive aspects of the project. But working with external providers creates the need for advanced team and project management features, such as: Access control is essential when video data is confidential, such as medical video annotations. As a project leader, you need to set clear rules and restrictions on who has access to specific data assets, especially when this could breach GDPR in Europe or healthcare data security legislation in the US (e.g., HIPAA). Performance dashboards, giving project leaders real-time visibility on video annotation project progress. Performance dashboards need to be granular. Giving you an overview for each annotator, reviewer, and annotation object (e.g., time spent, quality of annotation/rejection rate, and as much detail as you need to manage the process and project outputs effectively). On a higher level, you need to know the total number of annotations done (compared to the project total, so you can track progress) and which kind of annotations, alongside dozens of other details. User management in Encord And there we go, the 5 features every video annotation tool needs. At Encord, our active learning platform for computer vision is used by a wide range of sectors - including healthcare, manufacturing, utilities, and smart cities - to annotate videos and accelerate their computer vision model development. Experience Encord in action. Dramatically reduce manual video annotation tasks, generating massive savings and efficiencies. Try it for free today.
Nov 11 2022
10 M
The Full Guide to Video Annotation for Computer Vision
Computer vision has numerous cool applications like self-driving cars, pose estimation and many others in the field of medical imaging which uses videos as their data. Hence, video annotation plays a crucial part in training computer vision models. Annotating images is a relatively simple and straightforward process. Video data labeling on the other hand is an entirely different beast! It has an added layer of complexity but you can extract more information from it if you know what you are doing and use the right tools. In this guide, we’ll start with understanding video annotation, its advantages and use cases. Then we’ll look at the fundamental elements of video annotation and how to annotate a video. We’ll then look at video annotation tools and discuss best practices to improve video annotation for your computer vision projects. Video Labeling for Computer Vision Models In order to train computer vision AI models, video data is annotated with labels or masks. This can be carried out manually or, in some cases with AI-assisted video labeling. Labels can be used for everything from simple object detection to identifying complex actions and emotions. Video annotation tools help manage these large datasets while ensuring high accuracy and consistency in the process of labeling. Video annotation vs. Image annotation As one might think, video and image annotation are similar in many aspects. But there are considerable differences as well between the two. Let’s discuss the three major aspects: Data Compared to images, video has a more intricate data structure which is also the reason it can provide more information per unit of data. For example, the image shown doesn’t provide any information on the direction of movement of the vehicles. A video on the other hand would provide not only the direction but provide information to estimate its speed compared to other objects in the image. Annotation tools allow you to add this extra information to your dataset to be used for training ML models. Video data can also use data from previous frames to locate an object that might be partially obscured or contains occlusion. In an image, this information would be lost. Annotation process Comparing video annotation to image annotation, there is an additional level of difficulty. While labeling one must synchronize and keep track of objects in various states between frames. This process can be made quicker by automating it. Accuracy While labeling images, it is essential to use the same annotations for the same object throughout the dataset. This can be difficult and prone to error. Video on other hand provides continuity across frames, limiting the possibility of errors. In the process of annotation, tools can help you remember context throughout the video, which in turn helps in tracking an object across frames. This ensures more consistency and accuracy than image labeling, leading to greater accuracy in the machine learning model’s prediction. Computer vision applications do rely on images to train machine learning. While in some use cases, like object detection or pixel-by-pixel segmentation, annotated images are preferred. But considering image annotation is a tedious and expensive process, if you are building the dataset from scratch, let’s look at some of the advantages of video annotation instead of image data collection and annotation. Advantages of annotating video Video annotation can be more time-consuming than image annotation. But with the right tool, it can provide added functionalities for efficient model building. Here are some of the functionalities that annotated videos provide: Ease of data collection As you know a few seconds of the video contains several individual images. Hence, a video of an entire scene contains enough data to build a robust model. The process of annotation also becomes easier as you do not need to annotate each and every frame. Labeling the first occurrence of the object and the last frame the object occurs is enough. The rest of the annotation of in-between frames can be interpolated. Temporal context Video data can provide more information in form motion which static images cannot help the ML models. For example, labeling a video can provide information about an occluded object. It provides the ML model with temporal context by helping the ML model understand the movement of objects and how it changes over time. This helps the developer to improve network performance by implementing techniques like temporal filters and Kalman filters. The temporal filters help the ML models to filter out the misclassifications depending on the presence or absence (occlusion) of specific objects in adjacent frames. Kalman filters use the information from the adjacent frames to determine the most likely location of an object in the subsequent frames. Practical functionality Since the annotated videos provide fine-grained information for the ML models to work with, they lead to more accurate results. Also, they depict real-world scenarios more precisely than images and hence can be used to train more advanced ML models. So, video datasets are more practical in terms of functionality. Video annotation use cases Now that we understand the advantages of annotated video datasets, let’s briefly discuss how it helps in real-world applications of computer vision. Autonomous vehicles The ML models for autonomous vehicles solely rely on labeled videos to understand the surrounding. It’s mainly used in the identification of objects on the street and other vehicles around the car. It is also helpful in building collision braking systems in vehicles. These datasets are not just used in building autonomous vehicles, they can also be used to monitor driving in order to prevent accidents. For example, monitoring the driver’s condition or monitoring unsafe driving behavior to ensure road safety. Pose estimation Robust pose estimation has a wide range of applications like tracking body parts in gaming, augmented and virtual reality, human-computer interaction, etc. While building a robust ML model for pose estimation one can face a few challenges which arise due to the high variability of human visual appearance when using images. These could be due to viewing angle, lighting, background, different sizes of different body parts, etc. A precisely annotated video dataset, allows the ML model to identify the human in each frame and keep track of them and their motion in subsequent frames. This will in turn help in training the ML model to track human activities and estimate the poses. Traffic surveillance Cities around the world are adapting to rely on smart traffic management systems to improve traffic conditions. Given the growing population, smart management of traffic is becoming more and more necessary. Annotated videos can be used to build ML models for traffic surveillance. These systems can monitor accidents and quickly alert the authorities. It can also help in navigating the traffic out of congestion by routing the traffic into different routes. Medical Imaging Machine learning is making its way into the field of medical science. Many diagnoses rely on videos. In order to use this data for diagnosis through ML models, one needs to annotate. For example, in endoscopy, the doctors have to go through videos in order to detect abnormalities. This process can be fast-forwarded by annotating these videos and training ML models. ML models can run live detection of abnormalities and act as the doctor’s assistant. This will also ensure higher accuracy as there is a second method of filtration for the detection. For deeper insight into how video annotation helps doctors in the field of gastroenterology, take a look at our blog Pain Relief for Doctors Labeling Data. In the field of medical diagnostics, high-precision annotations of medical images are crucial for building reliable machine learning models. In order to understand the importance of robust and effective medical image annotation and its use in the medical industry in detail, please read our blog Introduction to medical image labeling for machine learning. Though the use cases discussed here mainly focus on the object detection and segmentation tasks in the field of computer vision, it is to be noted that use cases of video datasets are not limited to just these tasks. While there are several benefits to annotating videos rather than images and many use cases of video datasets alone, the process is still laborious and difficult. The person responsible for annotating these videos must understand the use of the right tools and workflows. What is the role of a video annotator? The role of a video annotator is to add labels and tags to the video dataset that has been curated for the specific task. These labeled datasets are used for training the ML models. The process of adding labels to data is known as annotation and it helps the ML models in identifying specific objects or patterns in the dataset. The best course of action if you are new to the process is to learn about video annotation techniques. This will help in understanding and using the ideal type of annotation for the specific task. Let’s first understand the different processes of annotating videos and then dive deeper into different methods to annotate a video. Video annotation techniques There are mainly two different methods one could annotate the videos: Single frame annotation This is more of a traditional method of labeling. The video is separated or divided into distinct frames or images and labeled individually. This is chosen when the dataset contains videos with less dynamic object movement and is smaller than the conventional publicly available datasets. Otherwise, it is time consuming and expensive as the videos one has to annotate a huge amount of image data, given a large video dataset. Multiframe or stream annotation In this method, the annotator labels the objects as video streams using data annotation tools, i.e the object and its coordinates have to be tracked frame-by-frame as the video plays. This method of video annotation is significantly quicker and more efficient, especially when there is a lot of data to process. The tagging of the objects is done with greater accuracy and consistency. With the growing use of video annotation tools, the multi-frame approach has grown more widespread. The continuous frame method of video labeling tools now features to automate the process which makes it even easier and helps in maintaining continuity. This is how it’s done: Frame-by-frame machine learning algorithms can track objects and their positions automatically, preserving the continuity and flow of the information. The algorithms evaluate the pixels in the previous and next frames and forecast the motion of the pixels in the current frame. This information is enough for the machine learning algorithms to accurately detect a moving object that first appears at the beginning of the video before disappearing for a few frames and then reappearing later. The task for which the dataset has been curated is essential to understand in order to pick the right annotation methods. For example, in human pose estimation, you need to use the keypoint method for labeling the joints of humans. Using a bounding box for it would not provide the ML model with enough data to identify each joint. So let’s learn more about different methods to annotate your videos! Different methods to annotate Bounding boxes Bounding boxes are the most basic type of annotation. With a rectangular frame, you surround the object of interest. It can be used for objects for which some elements of the background will not interfere in the training and interpretation of the ML model. Bounding boxes are mainly useful in the task of object detention as they help in identifying the location and size of the object of interest in the video. For rectangular objects, they provide precise information. If the object of interest is of any other shape, then polygons should be preferred. Annotating an image with bounding boxes in the Encord platform Polygons Polygons are used to annotate when the object of interest is of irregular shape. This can also be used when any element of background is not required. This process of annotating through polygons can be tiresome for large datasets. But with automated segmentation features in the annotation tools, this can get easier. Polylines Polylines are quite essential in video datasets to label the objects which are static by nature but move from frame to frame. For example, in autonomous vehicle datasets, the roads are annotated using polylines. Polygon and polyline annotation in the Encord platform Keypoints Keypoints are helpful for annotating objects of interest whose geometry is not essential for training the ML model. They outline or pinpoint the landmarks of the objects of interest. For example, in pose estimation, keypoints are used to label the landmarks, or the joints, of the human body. These keypoints here represent the human skeleton and can be used to train models to interpret or monitor the motion of humans in videos. Keypoint annotation in Encord Primitives They are also known as skeleton templates. Primitives are used for specialized annotations for template shapes like 3D cuboids, rotated bounding boxes, etc. It is particularly useful in labeling objects whose 3D structure is required from the video. Primitives are very helpful for annotating medical videos. Creating a skeleton template (primitives) in Encord Now that we have understood the fundamentals of video annotation, let us see how to annotate a video! How to annotate a video for computer vision model training Even though video annotations are efficient, labeling them can still be tedious for the annotator given the sheer amount of videos in datasets. That’s why designing the video annotation pipeline streamlines the task for the annotators. The pipeline should include the following components: 1. Define Objectives Before starting the annotation process, it is essential to explicitly define the project’s goal. The curated dataset and the objective of the ML model should be accounted for before the start of the annotation process. This ensures that the annotation process supports the building of a robust ML model. 2. Choose the right tool or service The type of dataset and the techniques you are going to use should be considered while choosing the video annotation tool. The tool should contain the following features for ease of annotation: Advanced video handling Easy-to-use annotation interface Dynamic and event-based classification Automated object tracking and interpolation Team and project management To learn more about the features to look for in a video annotation tool, you can read the blog 5 features you need in a video annotation tool. Label classifications in Encord 3. Review the annotation The process of reviewing the annotations should be done from time to time to ensure that the dataset is labeled as per the requirement. While annotating large datasets, it is possible that a few things are annotated wrongly or missed. Reviewing the annotation at intervals would ensure it doesn’t happen. Annotation tools provide operation dashboards to incorporate this into your data pipeline. These pipelines can be automated as well for continuous and elastic data delivery at scale. Video annotation tools There are a number of video annotation platforms available, some of them are paid whereas some of them are free. The paid annotation platforms are mainly used by machine learning and data operations teams who are working on commercial computer vision projects. In order to deal with large datasets and manage the whole ML lifecycle, you need additional support from all the tools you are using in your project. Here are some of the features Encord offers which aren’t found in free annotation tools: Powerful ontology features to support complex sub-classifications of your labels Render and annotate videos and image sequences of any length Support for all annotation types, boxes, polygons, polylines, keypoints and primitives. Customizable review and annotation pipelines to monitor the performance of your annotators and automatically allocate labeling tasks Ability to automate the annotation using Encord’s micro- model approach There are also video annotation tools which are free. They are suitable for academics, ML enthusiasts, and students who are looking for solutions locally and have no intention of scaling the solution. So, let’s look at a few open-source video annotation tools for labeling your data for computer vision and data science projects. CVAT CVAT is a free and open-sourced, web-based annotation tool for labeling data for computer vision. It supports primary tasks for supervised learning: object detection, classification and image segmentation. Features Offers four basic annotation techniques: boxes, polygons, polylines and points Offers semi-automated annotation Supports interpolation of shapes between keyframes Web-based and collaborative Easy to deploy. Can be installed in a local network using Docker but is difficult to maintain as it scales LabelMe LabelMe is an online annotation tool for digital images. It is written in Python and uses Qt for its graphical interface. Features Videos should be converted into images for the annotation Offers basic annotation techniques: polygon, rectangle, circle, line, and point Image flag annotation for classification and cleaning Annotations can only be saved in JSON format (supports VOC and COCO formats which are widely used for experimentation) Diffgram Diffgram is an open-sourced platform providing annotation, catalog and workflow services to create and maintain your ML model. Features Offers fast video annotation with high resolution, high frame rate and multiple sequences with their interface Annotations can be automated Simplified human review pipelines to increase training data and project management efficiency Store the dataset virtually; unlimited storage for their enterprise product Easy ingest of the predicted data Offers automated error highlighting to ease the process of debugging and fixing issues. Best practices for video annotation In order to use your video datasets to train a robust and precise ML model, you have to ensure that the labels on the data are accurate. Choosing the right annotation technique is important and should not be overlooked. Other than this, there are a few things to consider while annotating video data. So, how do you annotate effectively? For those who want to train their computer vision models, here are some tips for video annotators. Quality of the dataset The quality of the dataset is crucial for any ML model building. The dataset curated should be cleaned before starting the annotation process. The low quality and duplicate data should be identified and removed so that it doesn’t affect your model training adversely. If an annotation tool is being used then you have to ensure that it uses lossless frame compression so that the tool doesn’t degrade the quality of the dataset. Using right labels The annotators need to understand how the dataset is going to be used in the training of the ML model. If the project goal is object detection, then they need to be labeled using bounding boxes or the right annotation technique to get the coordinates of the object. If the goal is to classify objects, then class labels should be defined previously and then applied. Organize the labels It is significant to use customized label structures and use accurate labels and metadata to prevent the objects from being incorrectly classified after the manual annotation work is complete. So the label structures and the class it would belong to should be predefined. Use of interpolation and keyframes While annotating videos, you may come across objects that move predictably and don’t change shape throughout the video. In these cases, identifying the frames which contain important data which is enough is important. By identifying these keyframes, you do not need to label the whole video, but use them to interpolate and annotate. This speeds up the process while maintaining quality. So the sooner you find these keyframes in your video, the faster the annotation process. User-friendly video annotation tool In order to create precise annotations which will, later on, be used for training ML models, annotators require powerful user-friendly annotation tools. The right tool would make this process easier, cost-effective and more efficient. Annotation tools offer many features which can help to make the process simpler. For example, tools offering auto-annotation features like auto segmentation. Annotating the segmentations in video datasets manually is more time consuming than labeling classes or drawing bounding boxes for object detection. The auto-segmentation feature allows the annotator to just draw an outline over the object of interest, and the tool automatically “snaps' to the contours of the object saving the annotator's time of annotator. Similarly, there are many features a video annotation tool has which are built to help the annotators. While choosing the tool it is also essential to look at features such as automation, which align with the goal of the annotation and would make the process more efficient. Conclusion Computer vision systems are built around images and videos. So, if you are working on a computer vision project, it is essential to understand your data and how it has been created before building the model. I’ve discussed the difference between using images and videos, and the advantages of using videos for your ML model. Then we took a deeper dive into video annotation, and the techniques, and discussed briefly the tools available. Lastly, we looked at a few of the best practices for your video annotation. If you’re looking for an easy to use video annotation tool that offers complex ontology structures and streamlined data management dashboards, get in touch to request a trial of Encord.
Nov 11 2022
15 M
How Automated Data Labeling is Solving Large-Scale Challenges
We are on the verge of a computer vision revolution. It’s reminiscent of the early days of the internet: the promise of technology is clear, but society hasn’t yet seen widespread adoption of it. When we do, computer vision will touch every aspect of our lives. Consider our daily commutes. Car manufacturers are promising a future in which autonomous vehicles and robotics remove the cognitive and temporal burdens that come with driving. That future depends on computer vision algorithms. Thanks to computer-vision-powered “smart carts”, our everyday shopping experience is evolving rapidly. No more waiting in lengthy queues at the grocery store. Shoppers can scan, register, and pay for their items without having to visit the checkout counter. And what about our health? Recent advancements in deep learning and computer vision have increased the quality and capabilities of medical imaging so that computers can help doctors spot and diagnose abnormalities such as tumours and stroke indicators. Encord's DICOM tool can help doctors spot and diagnose abnormalities While it’s hard to predict all of the ways that computer vision will affect our day-to-day lives, it’s even harder to predict the ways in which computer vision will help humans tackle some of the world's most pressing problems. As computing power decreases in cost, machine learning models increase in accuracy, and data becomes more plentiful, organisations are thinking more and more about how best to use computer vision to address large-scale challenges. As an example, the recent proliferation of satellite imaging has created enormous opportunities to develop computer-vision-based approaches for responding to global challenges, such as coping with natural disasters and changing weather patterns. Every day, space technology companies are recording thousands of square kilometres of satellite imaging. When a natural disaster strikes, computer vision models can read this data and assess the damage, providing real-time intelligence of what’s happening on the ground from the moment the disaster begins. Quickly determining the extent of the damage can help international development agencies provide the appropriate amount of emergency disaster relief. Computer vision can help these agencies better understand the resource scarcity caused by a disaster and the types of aid most urgently needed to prevent human suffering. By automating image identification, computer vision models can likewise identify groups of people in satellite imaging, thereby helping search and rescue teams quickly locate people in crisis situations. The alternative method– having humans manually sift through aerial images and identify people in distress– is a time-consuming process that doesn’t align with the urgent action required to save lives after a natural disaster. Using computer vision to analyse the extent of the infrastructure damage in the event of a natural disaster also provides insight into the total amount of money needed for reconstruction, helping insurance companies efficiently estimate the cost of repair. As a result, insurance claims can be made and paid in a more timely manner than when they rely on a surveyor providing an on-foot assessment of the damage. When it comes to long-term strategies for coping with natural disasters and weather patterns, computer vision models can help scientists predict the changes that will occur in a particular area– such as increased flooding– as a result of climate change. With this knowledge, governments can make assessments about whether to prohibit people from building in and inhabiting areas where future disasters are likely. Computer vision could be a game changer for creating new approaches to tackling large-scale challenges such as providing disaster relief. Unfortunately, the artificial intelligence industry’s reliance on manually labelling data is hindering the technology’s progress. To make the most of this technology, companies need to curate high-quality datasets suited to the model’s use case and label them appropriately. Doing so requires moving away from outdated data management practices. Data, Data, Everywhere, and Not a Bit to Read The world has a surplus of data, and it’s increasing all the time. Ninety percent of the world’s data has been generated since 2016. This increase can create incredible opportunities for computer vision applications; however, most of the world’s data is unlabelled, so computer vision models can’t read it. These models need to train on well-curated and appropriately labelled training data sets. If not properly trained, well-designed models can become useless. These models also need to train on vast amounts of labelled data so that they can become incredibly confident in their predictions. (Remember these models will run self-driving cars and inform disaster relief strategies.) Acquiring large quantities of high-quality training data is the greatest obstacle for the advancement of computer vision. Unfortunately, most AI companies still rely on the practice of manual data labelling. Manually labelling data isn’t sufficient, scalable, or sustainable; furthermore, the escalation of data generation means the number of human labellers available will soon be outpaced by the amount of data that needs to be labelled. The Benefits of Automated Data Labeling Data labelling is a slow, tedious process that’s prone to human error. With a purely manual approach, annotating minutes of video and image data takes many hours. Many companies provide data labelling services in which they outsource the data to human labellers. However, such outsourcing means losing the input of subject-matter experts into the labelling process, which could result in low-quality training data and compromise the accuracy of computer vision model performance. Also, because these jobs often go to people living in developing economies, outsourcing data labelling isn’t a viable scenario for companies operating in any domain where security and data privacy are important, such as healthcare, education, and government. Some data labelling services offer ML model assisted labelling, but, to access these services, developers have to jump through a lot of hoops, including running their production models on the data they want to label before they can create and apply labels, which results in time-consuming operational burdens. Because of the issues associated with data labelling services, many teams build internal tools and use their in-house workforce to manually label their data. However, building these tools in-house often leads to cumbersome data infrastructure and annotation tools that are expensive to maintain and challenging to scale. So what’s to be done? For starters, we’ve got to acknowledge that the current manual approach to data labelling isn’t working. Breaking Away From Manual Labelling Data labelling cannot remain a manual process if machine learning in general, and computer vision in particular, are to become ubiquitous technologies Crowdsourcing, outsourcing, in-house labelling – none of these stop-gap approaches will clear the data bottleneck and unlock the power of AI for solving large-scale challenges. Their shortcoming is that they try to improve upon an inherently flawed system of manual labelling. They are effectively better wrenches when what’s needed is a power drill. Artificial intelligence’s looming needs for new data require new tools, ones capable of scaling. That’s why we designed Encord. Encord’s computer-vision first platform uses a unique technology called micro-models to automate data labelling. Our platform enables companies to break away from a system of data annotation dependent on manual labour. It automates labelling, running micro-models with only a few pieces of hand annotated data. Then, the micro-model begins to train itself to label the rest. Micro-models can be trained on just 5 images Encord also embeds its data annotation tools into the platform, so users can access model-assisted labelling without jumping through any hoops or placing any operational burdens on developers. In addition, companies retain 100 percent control of their data, making Encord an ideal solution for companies with data privacy and security considerations. Flexible ontology–defining a set of features that you are looking for in the data and mapping the relationships between those features– is necessary for using computer vision to solve large-scale problems. Encord enables flexible label ontology, which also allows users to target each micro-model to individual features in the ontology. With Encord, users can define multiple ontologies and then build a separate micro-model to label each different feature in the ontology. Supporting flexible ontology results in more advanced computer vision capabilities because it allows models to express greater complexity. To design complex computer vision models, users need to be able to construct a rich ontology. For instance, when determining the amount of damage caused by a natural disaster, a computer vision model needs to be able to identify the type of infrastructure and then identify whether the infrastructure has suffered damage. To determine the number of houses damaged by the disasters, users would build an “infrastructure damage” model and build a “house detector” for that specific feature. By combining many micro-models together, data engineers can ask nested questions and obtain granular ontologies that increase the usefulness of models for real-world use cases. For example, after building a micro-model to determine whether a natural disaster damaged a building, a data engineer could construct a micro-model to determine whether street flooding occurred nearby. By linking these two micro-models together, the engineer could gain a better sense of the overall infrastructure damage for that particular area. By combining model training and data-centric AI, companies can transform the promise of computer vision into reality. They can build smart cities, streamline manufacturing, and develop cancer detecting devices. They can build models that monitor climate change, predict natural disasters, and help increase food security. But to achieve that reality, companies must break with their unsustainable and unscalable labelling practices and embrace new tools designed for the future of AI.
Nov 11 2022
6 M
Data Annotation Tooling: Build vs. Buy: Lessons from Practitioners
Until recent years, any organization that wanted to scale data annotation, machine learning (ML), computer vision (CV) and other artificial intelligence-based (AI) projects had to build their own data annotation and labeling tools. Or failing that, use a combination of in-house tools and open-source annotation software to attempt to implement computer vision projects. Now technical leaders have a wide range of off-the-shelf data labeling, annotation, and active learning platforms to choose from. Whether you’re a CTO at an early-stage or growth-stage startup, or a Head of AI, Head of Computer Vision, or Data Operations leader at a larger organization, there’s a lot of choice in this market. And yet, the question is still something technical and ML leaders think about: “Should we build or buy an annotation tool?” This article aims to answer this question with insights from data annotation team leaders and practitioners. Why do data annotation teams need a labeling tool? Even now, with every technical advantage we have, annotating and labeling images or video-based datasets is a massively time-consuming part of any computer vision project. The quality and accuracy of data annotation labels are crucial. Poor-quality labeled data can cause huge problems for machine-learning teams. One of the best and fastest ways to improve the quality and accuracy of your labeled data is to use artificial intelligence (AI-assisted) labeling tools. AI solutions save time and money. Now comes the question, “Can we build our own or get an out-of-the-box solution?” Let’s see what data annotation leaders and practitioners have to say . . . Does your software engineering team have the time/resources to build a data annotation solution? Building an in-house solution is time-consuming and expensive. It can take anything from 9 to 18 months, costing 6 to 7 figures of in-house resources and taking over the working schedules of several engineers. As one sports analytics Encord customer found (before they came to us), “An in-house tool and interface for data annotation had limitations: it took months to build and refine, and the result was a single-purpose tool.” “When they needed new functionality, it took the in-house engineers months to redesign and reconfigure the tool. On the other hand, “Encord can build out a new ontology in a matter of minutes. Spending months building an in-house tool for each specific annotation task wasn’t a feasible, sustainable, or scalable strategy.” That client confirms that in-house resources were better spent elsewhere: “Before using Encord, the ML team had to take the safe route because of the high cost of pursuing new ideas that failed. With a multi-purpose, cost-effective annotation tool, they can now iterate on ideas and be more adventurous in developing new products and features.” How long would it take to build a data annotation tool for your project(s)? Building an in-house annotation tool can take months. It all depends on: The volume of an image or video-based datasets you need to annotate; The functionality the platform needs; The number of annotators who are going to use the platform, The time you’ve got, as an ML or data ops leader, to get this solution to market, so you can start using it to annotate images and videos (before beginning the process of training a data model); How scaleable this tool needs to be: What other projects will it be needed on in the future? With that in mind, an engineering team can start estimating project build time. Or, if you’ve got the budget, the outsourcing costs of having a third-party software development company complete the project. Either way, we are talking months of work, a large capital budget’s required, and a project leader is needed to oversee it. Once complete, you’ll need in-house developers familiar with the annotation software to fix bugs, maintain it, and implement any upgrades and new features/functionality it needs. Would it make more sense to outsource development to a third party? In some cases, outsourcing development to low-cost regions, such as Central & Eastern Europe (CEE), can cost less than building in-house. Especially when you compare the cost of engineers and data scientists in those regions vs. US or Western European professionals with those same skills. However, the challenges are similar to building in-house. The project still needs managing. Once ready, an in-house team must look after, debug, maintain the tool, and implement new features and functionality. Advantages of Buying a Data Annotation Tool Instead of going the in-house or outsourced build route, many organizations are making the financially and time-based decision to buy an out-of-the-box solution, such as Encord. Dr. Hamza Guzel, a Turkish Ministry of Health radiologist, explains the advantages of using Encord for medical image data annotation. Dr. Guzel also works with Floy, a medical AI company developing technology that assists radiologists in detecting lesions, helping them prepare the medical imaging data used to train their machine learning models. Floy had numerous problems with other commercial off-the-shelf solutions and didn’t consider building one because of the time and cost involved. So, the solution was to switch to Encord for CT & MRI annotation and labeling. “The organizational issue was not a problem in Encord, and with Encord’s freehand annotation tool, we can label data however we want. We can decrease the distance between the dots on boundaries to work at the millimeter scale that we need to label lesions and other objects precisely. Labeling is also a smooth experience– it’s very easy to draw on the image and move from one image slice to another.” “It’s also fast. I didn’t realize how slow the other platforms were, or how fast labeling could be until we switched to Encord.” “Using Encord, we reduced the labeling time for CT series by 50 percent and 25 percent for MRI series.” Read more about how Encord is Reducing Experiment Duration for Stanford Medicine. In Conclusion: Should I Build or Buy a Data Annotation Tool? Depending on your data annotation needs, here are five features that the best out-of-the-box solutions have, such as Encord. If all of those features sound familiar (and we’ve introduced more since then, such as Encord Active and the Annotator Training Module), you have to ask yourself, do we have the in-house time/resources to build something similar ourselves? Or would it be easier to avoid capital outlays and project management headaches and simply buy an off-the-shelf data annotation solution? In every way, buying a data annotation tool is: Far less expensive than building Less time consuming (you can be set up in minutes instead of months) Significantly faster for getting machine learning, and computer vision models production ready More flexible (better functionality, including APIs and SDKs) As one G2 review says: “Encord has helped us streamline our data pipelines and get our training data into one place. We've managed to build fairly seamless integrations using the flexible API.” “We've also been using some of the customizable dashboards & reports in our reporting, which has been a plus. The user interface is easy to navigate, and the object detection annotation tools (bounding box, etc.) are very expansive in functionality as we can define rich ontologies supported in the platform.” Benjamin, Data Scientist at a Mid-market company using Encord. Another review says: “Encord's DICOM annotation solution is solving the problem of inefficient and time-consuming image annotation and workflow management for building training datasets for medical AI. By streamlining these processes, it is saving our team a lot of time and increasing our overall productivity.” “Additionally, the quality control features ensure that all images are of the highest quality, providing peace of mind for both radiologists and our ML research team which has helped with going through FDA clearance. Overall, this product is greatly benefiting our team by making our annotation work more efficient and organized.” Thomas, Clinical Machine Learning Engineer. At Encord, our active learning platform for computer vision is used by a wide range of sectors - including healthcare, manufacturing, utilities, and smart cities - to annotate human pose estimation videos and accelerate their computer vision model development. Here's more Encord customer stories. Encord is a comprehensive AI-assisted platform for collaboratively annotating data, orchestrating active learning pipelines, fixing dataset errors, and diagnosing model errors & biases. Try it for free today.
Nov 11 2022
4 M
How to Label a Dataset (with Just a Few Lines of Code)
The purpose of this tutorial is to demonstrate the power of algorithmic labelling through a real world example that we had to solve ourselves. In short, algorithmic labelling is about harvesting all existing information in a problem space and converting it into the solution in the form of a program. Here is an example of a algorithmic labelling that labels a short video of cars: a) Raw Data b) Data Algorithm c) Labelled data Our usual domain of expertise at Encord is in working with video data, but we recently came across a problem where the only available data was in images. We thus couldn’t rely on the normal spatiotemporal correlations between frames that are reliably present in video data to improve the efficiency of the annotation process. We could, however, still use principles of algorithmic labelling to automate labelling of the data. Before we get into that, the problem was as follows: Company A wants to build a deep learning model that looks at a plate of food and quantifies the calorie count of that plate. They have an open source dataset that they want to use as a first step to identify individual ingredients on the plate. The dataset they want to use is labelled with an image level classification, but not with bounding boxes around the “food objects” themselves. Our goal is to re-label the dataset such that every frame has a correctly placed bounding box around each item of food. Example Food Item with Bounding Box Instead of drawing these bounding boxes by hand we will label the data using algorithmic labelling. Why Algorithmic Labelling? So before we talk about solving this with algorithmic labelling, let’s look at our existing options to label this dataset. We can: go ahead and hand label it ourselves. It takes me about six seconds to draw a bounding box, and with ~3000 images, it will take me about five hours to label all the images manually. send the data elsewhere to be labeled. An estimated outsourced cost will likely be around $0.15 per image with total cost about $450. It will additionally take some time to write a spec and get a round trip of the data through to an external provider. Big Data Jobs If we look at the cost/time tradeoffs of algorithmic labelling against our first two options, it might not seem like a slam dunk. Writing a good program will take time, maybe initially even more time than you would be spending annotating the data yourself. But it comes with very important benefits: Once you have an algorithm working, it is both reusable for similar problems, and extensible to fit slightly altered problems. The initial temporal cost of writing a program is fixed, it does not increase with the amount of data you have. Writing a good label algorithm is thus scalable. Most importantly, writing label algorithms improves your final trained models. The data science process does not start once you have a labelled dataset, it starts once you have any data at all. Going through the process of thinking through an algorithm to label your data will give you insight into the data that you will be missing if you just send it to an external party to annotate. With algorithmic labelling there is a strong positive externality of actually understanding the data through the program that you write. The time taken is fixed but the program, and your insight, exists forever. With all this in mind, let’s think through a process we can use to write a program for this data. The high level steps will be Examine the dataset, Write and test a prototype Run the program out of sample and review Examine the dataset The first step to any data science process should be to get a look at the data and take an inventory of its organisational structure and common properties and invariants that it holds. This usually starts with the data’s directory structure: We can also inspect the images themselves: Sample images Let’s go ahead and note down what we notice: The data is organised in groups of images of photographs of individual items of food on a plate. The title of each folder containing the images is the name of the piece of food that is being photographed There is only one piece of food per image and the food is the most prominent part of the image The food tends to be on average around the centre of the frame The colour of the food in most images stands out since the food is always on a white plate sitting on a non colourful table There is often a thumb in the picture that the photographers likely used for a sense of size scaling There are some food items that look more challenging than others. The egg pictures, for instance, stand out because the colour profile is white on white. Maybe the same program shouldn’t be used for every piece of food. The next step is to see if we can synthesise these observations into a prototype program. Write a prototype There are a few conclusions we can draw from our observations and a few educated guesses we can make in writing our prototype: Definites -We can use the title of the image groups to help us. We only need to worry about a particular item of food being in an image group if the title includes that food name. If we have a model for a particular item of food we can run it on all image groups with that title. -There should only be one bounding box per frame and there should be a bounding box in every single frame. We can write a function that enforces this condition explicitly. -We should add more hand annotations to the more “challenging” looking food items. Educated Guesses -Because the food location doesn’t jump too much from image to image, we might want to try an object tracker as a first pass to labelling each image group -Food items are very well defined in each picture so a deep learning model will likely do very well on this data -The colour contrasts within the pictures might make for good use of a semantic segmentation model Let’s synthesise this together more rigorously into a prototype label algorithm. Our annotation strategy will be as follows: Use the Python SDK to access the API and upload the data onto the Encord annotation platform using the directory structure to guide us. We will use a Encord data function to concatenate the separate images into a video object so that we can also make use of object tracking Hand annotating two examples for a piece of food, one on the first image and one on halfway point image. For what we think are going to be trickier food items like eggs, we will try ten annotations instead of two. Run a CSRT object tracker across images. Again this dataset is not a video dataset, but with only one object per image that is around the same place in each frame, an object tracking algorithm could serve as a decent first approximation of the labels. Train a machine learning model with transfer learning for each item of food using tracker-generated labels. We can start with a segmentation model. The objects have a stark contrast to the background. Training a model with the noisy labels plus the stark contours might be enough to get a good result. We already converted our bounding boxes to polygons in the previous step, so now the Encord client can train a segmentation model. Run the model on all image groupings with that piece of food in the title. Convert the polygonal predictions back to bounding boxes and ensure that there is only box per image by taking the highest confidence prediction. That’s it.The data function library and full SDK are still in private beta, but if you wish to try it for yourself sign up here. Let’s now run the program on some sample data. We will choose bananas for the test: It seems to do a relatively good job getting the bounding boxes. Run the algorithm “out of sample” and review Now that we have a functioning algorithm, we can scale it to the remaining dataset. First let’s go through and annotate the few frames we need. We will add more frames for the more difficult items such as eggs. Overall we only hand annotate 90 images out of 3000.We can now run the program and wait for the results to come back with all the labels. Let’s review the individual labels. We can see for the most part it’s done a very good job. The failure modes are also interesting here because we get a “first-look” of where our eventual downstream model might have trouble. For these “failures” I can go through and count the total number that I need to hand correct. That’s only 50 hand corrections in the entire dataset. Overall, the label algorithm requires less than 5% of hand labels to get everything working. And that’s the entire process. We made some relatively simply observations about the data and converted those into automating labelling of 95% of the data. We can now use the labelled dataset to build our desired calorie model, but critically, we can also use many of the ideas we had in the algorithmic labelling process to help us as well. Real world examples are always better than concocted examples in that they are messy, complex, and require hands-on practical solutions. In that vein, you exercise a different set of problem-solving muscles than would normally not be used in concocted examples with nice closed formed type solutions.
Nov 11 2022
9 M
Why You Should Ditch Your In-House Training Data Tools
At Encord, we’ve spent weeks interviewing data scientists, product owners, and distributed workforce providers. Below are some of our key learnings and takeaways for successfully establishing and scaling a training data pipeline. If you’ve ever dabbled in anything related to machine learning, chances are you’ve used labeled training data. And probably lots of it. You might even have gone through the trouble of labeling training data yourself. As you have most likely discovered, spending time creating and managing training data sucks — and it sucks even more if you can’t find an open-source tool that fits your specific use case and workflow. Building custom tools might seem like the obvious choice, but making the first iteration is typically just the tip of the iceberg. More start- and scale-ups than we can count end up spending an insurmountable amount of time and resource building and maintaining internal tools. Making tools is rarely core to their business of building high-quality machine learning applications. Here are things to consider when establishing your training data pipeline and when you might want to ditch your in-house tools. Is It Built To Scale? You’ve produced the first couple of thousand labels, trained a model, and put it into production. You begin to discover that your model does poorly in specific scenarios. It could be that your food model infers a tomato as an orange in dim lighting conditions, for example. You decide to double or even triple your workforce to keep up with your model’s insatiable appetite for data to help solve these edge cases. If your tool is built on top of CVAT — like most of the machine vision teams we’ve worked with — it quickly starts to succumb to the increased workload and comes down crashing faster than you can say Melvin Capital. Cost Grows with Complexity Machine learning is an arms race. Keeping up with the latest and greatest models require you to re-evaluate and update your training data. That typically means that the complexity of your label structure (ontology) and data grows, requiring you to add new features to your in-house tools continuously. New features take time to build and will be around to maintain long after, eating up precious resources from your engineering team and dragging down your expensive workforce’s productivity. This cost is not immediately apparent when you are first building out a pipeline but can become a considerable drag on your team as your application grows. I/O Is Key to Success A robust pipeline should give you a complete overview of all of your training data assets and make it easy to pipe them between different stakeholders (annotators, data scientists, product owners, and so on). Adequate piping necessitates that the data resides in a centralized repository and that there is only a single source of truth to keep everyone synced. Building a series of well-defined APIs that allows for effective pushing and pulling data is no small feat. Additionally, making a good API is often complicated by attempting to mould training labels produced by open-source tools into queryable data assets. Label I/O should be as simple as calling a function Starting from Scratch When establishing a training data pipeline, the perennial mistake teams make when they spend money on a workforce is starting the annotation process from scratch. There are enough pre-trained pedestrian and car models to cut initial annotation costs drastically. Even if you are working on something more complex, using transfer learning on a pre-trained model fed with a few custom labels can get you far. An additional benefit is that it allows you to understand where a model might struggle down the line and immediately kickstart the data science process before sinking any money into an expensive workforce. At Encord, we applied this exact method in our collaboration with the gastroenterology team at King’s College London, helping them speed up their labeling efficiency by 16x, which you can read more about here. Labeling Pre-Cancerous Polyps Case Study: Marginal cost per label with and without utilizing pre-trained models & data algorithms Doesn’t Get Smarter With Time In addition to using pre-trained models, intelligently combining heuristics and other statistical methods (what we like to call ‘data algorithms’) to label, sample, review, and augment your data can drastically increase the ROI on human-produced labels. Existing software doesn’t apply these intelligent ‘tricks’, which means that the marginal cost per produced label remains constant. It shouldn’t. It should fall, even collapse, as your operation scales. We’ve seen teams attempt baking in some of these methods in their existing pipelines. However, each data algorithm can take days, if not weeks, to implement and often lead to nasty dependency headaches. The latter can be a substantial time suck — we know first-hand how frustrating it can be to line up the exact version of CUDA matching with PyTorch, matching with torchvision, matching with the correct Linux distribution… you get the idea. Conclusion If any of the above points resonate with you, it might be time to start looking for a training data software vendor. While the upfront cost of buying or switching might seem steep relative to building on top of an open-source tool, the long-term benefits most often outweigh the costs by orders of magnitude. Purpose-built training data software ensures that all of your stakeholders’ needs are satisfied, helping you cut time to market and increase ROI. If you’re a specialist AI company or a company investing in AI, training data is at the core of your business and forms a vital part of your IP. It is best to make the most of it.
Nov 11 2022
5 M
Playing videos is easy, pausing them is actually very difficult
The core of Encord's offering is building fast and intuitive object detection, segmentation, and classification video annotation tools to build training datasets for computer vision models. Just as items can move across different frames in the video, we have to make sure that we store the correct coordinates of individual labels corresponding to the correct frame number in the video. You might think, “duh,” but this is a serious and complicated problem to solve. We consistently have companies switching to our platform after they have had to throw away months' worth of manual annotation from other commercial tools not purpose built for video or from expensive labeling teams on open-source tooling. All because they realized that labels were misaligned from the corresponding frames due to a myriad of issues casued by rendering video data in modern web browsers. This is how we approached the problem: We embed videos via the HTML <video> element. When a user pauses the video to draw a label, we query the current frame number of the video from the relevant DOM element and store the data in our backend. Whenever the client needs the label for a review process, to train or apply machine learning algorithms, or to download the labels for their internal tools, they get the correct data. That is the point when we realized we had solved all our client’s problems and retired to the Caribbean forever. While this scenario is what we wished for, this is unfortunately not the way that the <video> element works. The problem is that it does not allow you to seek a specific frame but only a specific timestamp. This should not be a problem if you think that seeking a specific frame would be seeking to timestamp_x = frame_x * (1 / frames_per_second) which works unless: There is a variable frame rate in the video. There are other unknown complications. Variable frame rates in a video file When talking about videos with variable frame rates one might think that only time travelers or magicians might need those. While they are uncommon, there seems to be a use case, especially with dashcams producing them. We assume that dashcams are trying to save frames as fast as they can; with different processes grabbing the dashcam CPU’s attention, this might sometimes mean faster and sometimes slower writes. Okay, these exist, let’s see later if we can support those. Other unknown complications with annotating video content When we looked into what else can go wrong, we opened up a can of worms so unpleasant that we decided against adding an appropriately graphical gif here (you’re welcome). While we thought we could apply our magic formula of timestamp_x = frame_x * (1 / frames_per_second) whenever there is no variable frame rate, we quickly realized that different media players can in some circumstances show a different amount of frames or stretch/shorten the length of specific frames. This is especially true for the media player behind Chromium based browsers (e.g. Google Chrome). With the help of our friend FFmpeg, we can check the metadata of a video and the metadata of each individual frame in the video on our server. That way we can actually verify the true timestamp at which a frame is meant to be played. However, we cannot access this metadata from the <video> element. We also cannot reproduce the same timestamp heuristics in our server as there are in the browser. Therefore, there is a frame synchronization issue between the frontend and our backend. Possible Solution What we know so far is: In Chrome, we can only seek the frame of a video by providing a timestamp. We found out that Chrome will sometimes move between frames at incorrect timestamps. We do need to ensure that labels are stored with the correct frame number (as shown by FFmpeg on our server). In short, there is a problem to solve. Let’s look at possible solutions. Unpacking a video into images One trivial solution is to use our friend FFmpeg to unpack a video into a directory of images with the frame number as part of the image. A command like $ ffmpeg -i my-video.mp4 my-video-images/$filename%03d.jpg would make that possible. Then we could upload the images in the frontend, and when the user navigates around frames, we display the corresponding image. This would mean we might have no proper support for video playback on our frontend, which can make videos feel clunky. The bigger issue is that we would blow up the storage size of videos on our platform. The whole point of videos is the compression of images. A 10-minute video such as this one in full HD takes about 112MB of storage as a video but needs 553MB of storage as extracted images. We see the storage requirements of clients hitting terabytes and continuing to grow, so we decided against this approach. Using web features that allow seeking specific frames We were not the first developer team that had this problem. You can read more discussions here. Given the frustration of other developers, there are now experimental web features, all with their own advantages and disadvantages. Some of them are The HTMLMediaElement.seekToNextFrame() callback function. Using WebCodecs for more fine-grained control. The HTMLVideoElement.requestVideoFrameCallback() callback function. If you are reading this article and any of those has landed to become properly supported with API backward compatibility guarantees in the browser, we suggest you do one of the following: Celebrate, close this article, and use one of those to solve frame synchronization issues. Celebrate and continue reading this article as a history lesson. We decided against using experimental features due to possible API breakages in newer browser versions. Aligning labels with the correct frames is at the core of our platform, and we felt a more robust solution would be appropriate. Finding out when the browser media player acts up and reacting accordingly Spoiler alert: This is what we decided to do to solve issues. We felt that by finding out which type of videos are causing problems, we would a.) gain valuable experience in the world of video formats and media players in our developer team and b.) be able to offer an informed solution to our clients to guarantee frame synchronization in our platform. To find problems quicker, we have built an internal browser-based testing tool that takes two inputs: the frames per second (fps) of the video the video itself It then embeds the video with the <video> element and increments the timestamp with very small steps. On each step, it takes a screenshot of the currently displayed frame and compares this to the previous screenshot. If the images differ we can guarantee that the browser is displaying a new frame. We then record the timestamp at which that happened. The tool flags whether the timestamps of the start of each frame are as expected, given the fps of the video. Videos with audio One pattern that we consistently saw with our testing tool was that in many videos, the very first frame was displayed for longer than it should have been. A frame rate of about 23.98 fps is a common video standard. This means every frame would last for about 0.0417 seconds, but in many videos, the first frame was 0.06305 long. Just over 1.5x the expected length. If the first frame is stretched, that means that all the labels of the entire video will be off by one frame. We inspected the packets with this command: $ ffprobe -show_packets -select_streams v -of json video.mp4 And found that for the problematic videos, we would see audio frames with a negative timestamp. “Timestamp” here refers to the time that is reported from the metadata of the video from FFmpeg tools - not the timestamp that we seek in the <video> element. If the media player decides to play at least part of the negative audio frames (and Chromium decides to do so), it is forced to stretch the first frame for longer than its usual display time to display a frame while those negative audio frames are playing. The <video> element has a muted attribute which we tried enabling in our video player, so Chrome would not have to stretch the first frame. Unfortunately, while the audio was being ignored, the first frame was still stretched. We then tried removing the audio frames from the video with $ ffmpeg -i video-with-audio.mp4 -c:v copy -an video-no-audio.mp4 All this does is copy the video frames and drop the audio frames into a new video called `video-no-audio.mp4`. We then uploaded the video to our testing tool, and voila - the problem was fixed, and the frames were displayed with the same timestamps as in the “Expected” row. Ghost frames We coined this spooky term when stumbling upon a video where we found video frames with a negative timestamp in the metadata. Why do such videos exist? It could come from trimming a video where key frames or “infra frames” are kept around from negative timestamps. You can read more about the different types of frames in a video here. When playing back this video with different media players, we noticed that they all would decide to display anywhere between none and all of the frames with negative timestamps. Given that there was no way to deterministically say how many of those were displayed, we decided that we would need to remove all those frames by re-encoding the video. Re-encoding is the process of unpacking a video into all of its image frames and packing it up into a video again. While doing that, you can also choose to drop corrupted frames, such as frames with negative timestamps. With mp4 files, this command could look like: $ ffmpeg -err_detect aggressive -fflags discardcorrupt -i video.mp4 -c:v libx264 -movflags faststart -an -tune zerolatency re-encoded-video.mp4 -err_detect aggressive reports any errors with the videos to us for debugging purposes -fflags discardcorrupt removes corrupted packets -c:v libx264 encodes the video with the H.264 coding format (used for mp4) -movflags faststart is recommended for videos being played in the browser; it puts video metadata to the start of the video so playback can start immediately as the video is buffering. -an to remove audio frames - in case they would be problematic -tune zerolatency is recommended for fast encoding. Re-encoding the video with this command would remove all of the frames with negative timestamps, removing this problem. Variable frame rates Coming back to the videos with variable frame rates, we saw two options to deal with them: Pass a map of the frame number to the timestamp from the backend to the frontend to seek the correct time. Re-encode the video forcing a constant frame rate. Given our experience with some unexpected behavior around videos with audio frames or videos with ghost frames, we did not want to speculate anything about the browser not stretching/squeezing frame lengths in variable frame rate videos. Therefore, we ended up going with option 2. We used a similar re-encoding FFmpeg command as from above, just with the -vsync cfr flag added. This would ensure that FFmpeg figures out a sensible constant frame rate given the frames that it has seen before. Given that it needs to squeeze frames of a variable rate into a constant rate, this means that some frames will then be duplicated or dropped altogether. A tradeoff we felt was fair given that we can ensure data integrity. Closing thoughts To recap, we found a frame synchronization issue in how we see the number of frames in a video. In our backend, we could reliably look into the metadata of the video using FFmpeg to find out the exact timestamp of a frame. In the browser, we could only seek a frame via a timestamp, but we needed to deal with multiple issues where the browser would not be reliable in translating a timestamp into the exact frame number within that video. In retrospect, we are glad that we decided to look into the individual frame synchronization issues that would arise from different videos instead of just unpacking the video into images. Our dev team has now built a solid understanding of video encoding standards, different behaviors of media players, possible problems with those, and how to provide the right solutions. We now report to clients any potential issues ahead of annotation time and offer them a solution with the click of a button. We can also give them enough context on why exactly they have to re-encode certain videos.
Nov 11 2022
5 M
3 Key Considerations for Regulatory Compliance in AI systems
There’s nothing worse than putting in the time, effort, and resources into building an artificial intelligence (AI), machine learning (ML), or a computer vision (CV) model only to find out you can’t use it. Failing regulatory compliance is one of those mission-critical factors, especially in sectors such as healthcare, that you can’t afford to overlook. It’s even worse if what you’re missing is operationally crucial, such as ensuring the whole data management, labeling, annotation, and model training, and production process should have been geared to align with regulatory compliance practices. When it comes to building artificial intelligence systems (AI), you’ve got to take data compliance considerations into account from day one; otherwise, your project will be finished before it even begins. What is the importance of regulatory compliance? Compliance regulations exist for good reason, especially when it comes to handling any kind of potentially sensitive data, including images and videos. Data compliance regulations exist to ensure that companies, governments, and researchers handle data responsibly and ethically. However, developing machine learning models and emerging technologies that derive meaningful information from imagery is a challenging task. Compliance regulations can create additional headaches when designing these systems for AI application use cases, including computer vision models in healthcare and clinical operations. Production models run in the real world on out-of-sample data. They evaluate never-before-seen data to make predictions and generate outcomes, and they can only make predictions based on the training a model receives, based on the datasets they were trained on. Even the smartest ML or CV models can’t reason and infer how a human can when encountering new data without a frame of reference. To ensure the highest performance possible, algorithmic models must train on a vast amount and variety of data. However, different legal frameworks govern data in different ways. When building and training a model, the data used must be compliant with the regulatory framework where the data originated, even if the model is being built or deployed elsewhere. For example, some jurisdictions have stricter laws protecting citizens' identifiable information than others. Models trained on data collected in these jurisdictions might not be able to be shipped elsewhere. Similarly, healthcare AI systems trained on US data must often meet HIPAA regulations with unique criteria for patients’ medical data, creating constraints around where the model can be deployed. Machine learning engineers must successfully navigate the inherent tension between acquiring as much data as possible and abiding by compliance regulations. With that in mind, here are three compliance considerations to take into account when building production AI technologies. What are the three key considerations for regulatory compliance? In this article, we cover the following top 3 considerations for regulatory compliance: Partitioning Training Data For Data Privacy Auditability for Data Annotations Data Compliance Throughout The Release Lifecycle: From Annotation to CV Model Deployment Partitioning Training Data For Data Privacy To follow best practices for data-centric AI, you should train a model on large volumes of diverse and high-quality labeled datasets. However, you can’t just mix and match data as needed to fill out your training dataset. Data operations teams have got to be sure that the data you're using complies with the regulatory requirements of its country, state, or region of origin. Within each country, state, or region, different institutions and governing bodies will have different requirements for handling data, achieving regulatory compliance, and broader risk management. For instance, let’s say you’re building a computer vision model for medical imaging. You’ve obtained a million images from various hospitals to train the model. However, one-third of the images originated in the US, so that data is subject to HIPAA regulations. In contrast, another third originated in Europe (specifically within the European Union), so it’s subject to GDPR. Meanwhile, the last third is open-source and, therefore, freely licensed. Unfortunately, training one model on all these images would be difficult while ensuring the outputs remain compliant. For regulatory compliance reasons, it would be better to partition the data into separate buckets and build three distinct models so that each one is compliant with the appropriate regulatory framework as determined by the data’s origins. Documenting and showing your workflows and processes will also be important to prove that you followed the respective compliance rules from the start. So, keep a clear record of the training data used for each computer vision model. Traceability can create a significant challenge from an engineering perspective. It’s a cumbersome and difficult task but also a serious consideration when building production AI. If you spend resources building a model only to realize later that one piece of data in the training dataset wasn’t compliant, you’ll have to scrap that model. Thanks to the non-compliant data, you’d have to go through the entire building process again, retraining the model without it. Unfortunately, this is similar to a judge throwing out an entire court case because a crucial piece of evidence was obtained illegally. It happens, and data scientists must meet exacting requirements, especially in sectors with strict compliance requirements. Auditability for Data Annotations When putting an AI model into production, you’ve got to consider the auditability of the data, not just the models. Make sure there’s an exact audit trail of how each piece of training data and its label was generated because both the labels and data must comply with the process you’re trying to optimize. For example, when it comes to developing medical AI, some regulatory bodies have implemented an approval process for algorithms, which requires independent expert reviews. These procedures are in place to ensure that the model learns to make predictions from training data that has either been labeled or reviewed by a certified professional. As such, when medical companies build production AI, a designated number of medical specialists must review the labeled training data before the company can use it in downstream model-building applications. They must also keep a record of how each piece of data was labeled, who it was reviewed by, and how many times it was reviewed. With Encord, you can do all of this, thanks to our regulatory-compliant and auditable dashboard, so you’ve got a record of the entire flow of data, from raw images or videos, through to a production-ready model. Encord's DICOM labeling tool in action Data Compliance Throughout The Release Lifecycle: From Annotation to CV Model Deployment Before building the model, it’s wise to consider the localities that will be involved in each stage of the production cycle. Ask yourself: Where is the model being trained? Is it being trained in the same jurisdiction as where the labels and training data were generated? Where is the model being deployed after training? From a production and model deployment viewpoint, the answers to these questions are important for preventing issues down the road. For instance, if your training data is in the US, but your model training infrastructure is established in the UK, you need to know if you’re allowed to process that data by sending it to the UK. Even if you have no intention of storing data in the UK, you still have to establish whether you’re allowed to process that data ⏤ e.g., train the model and perform various types of experiments over the model ⏤ in the UK. It gets even more complex if you’ve got an outsourced data annotation team elsewhere in the world, such as South East Asia. Data operations leaders need to know they can store, send, and share datasets with outsourced collaboration teams without compromising the entire project's regulatory compliance. The practical implication for the AI companies, and organizations using computer vision models, is that they either have to have model infrastructure deployed in different jurisdictions so that they can process data locally, or they have to ensure that they have data processing agreements in place with customers, which clearly state whether and where they intend to process the data. Some jurisdictions have much more stringent rules around data processing and storage than others, and it’s important to know the regulations around data collection, usage, processing, and storage, for all the relevant jurisdictions. Compliance regulations can create headaches for building production AI by adding operational overhead when making the model work in practice. However, it’s best to know the rules from the start and decrease the potential high-risk situation of having to abandon a model for falling afoul of AI regulations. At Encord, we’ve worked with multiple customers from different jurisdictions and different data requirements. With our user-friendly, computer-vision-first platform and in-house expertise, we help companies develop their training data pipeline while removing their compliance headaches. Encord is a comprehensive AI-assisted platform for collaboratively annotating data, orchestrating active learning pipelines, fixing dataset errors, and diagnosing model errors & biases. Sign-up for an Encord Free Trial: The Active Learning Platform for Computer Vision, used by the world’s leading computer vision teams. AI-assisted labeling, model training & diagnostics, find & fix dataset errors and biases, all in one collaborative active learning platform, to get to production AI faster. Try Encord for Free Today. Want to stay updated? Follow us on Twitter and LinkedIn for more content on computer vision, training data, and active learning. Join our Discord channel to chat and connect.
Nov 11 2022
4 M
5 Steps to Build Data-Centric AI Pipelines
Data-centric AI is a positive emerging trend in the machine learning (ML) and computer vision (CV) community. Simply put, data-centric AI is the notion that the most relevant component of an AI system is the data that it was trained on rather than the model or sets of models that it uses. The data-centric AI concept recommends an attentional shift from finding improvements to model architectures and hyper-parameters to finding ways to improve the data. With the idea that better data will produce more accurate model outcomes. While this is fine in the abstract, it leaves a little to be desired concerning the actions necessary for a real-world AI practitioner. Data scientists and data ops teams are right to wonder: How exactly do you transition your workload from iterating over models to over data? Model accuracy on ImageNet is leveling off over time In this article we will go over a few of the practical steps for how to properly think about and implement data-centric AI. Specifically, we will investigate how data-centric AI differs from model-centric AI with respect to creating and handling training data. For more information, here's our article on 5 Strategies To Build Successful Data Labeling Operations What is a Data-centric approach to AI (artificial intelligence)? Data-centric shifts the focus when training computer vision models, or any algorithmically-generated model, from the model to the data. Unleashing the true potential of AI means sourcing, annotating, labeling, and building better datasets. The accuracy and output quality can and will improve dramatically with higher-quality data going into a model. Any data-centric approach is only as good as your ability to source, annotate, and label the right data to put into your model. In a previous article, we explore: The importance of finding the best training data How to prioritize what to label How to decide which subset of data to start training your model on How to use open-source tools to select data for your computer vision application With that in mind, we can now turn to the benefits of a data-centric approach and 4 ways to implement a data-centric strategy. What are the benefits of a data-centric approach to AI? Adopting a data-centric approach for AI, ML, and computer vision models gives organizations numerous advantages when training and implementing production-ready models. As we’ve seen from working with companies in dozens of sectors, a data-centric approach, when supported by an AI-driven active learning platform for labeling and model training, produces the following advantages: Build and train computer vision models faster; Improve the quality of the data, and therefore, the accuracy and outputs of the model; Reducing the time it takes to train a model to deployment; Enhanced iterative learning cycles, improving the production-ready model's accuracy and outputs. 5 Steps for implementing a data-centric approach to AI, ML, and Computer Vision: Sourcing, Managing, Annotating, Reviewing, and Training (SMART) Here are the five steps you need to take to develop a data-centric approach to AI, using the SMART model. Sourcing the right data Includes: Finding data, collecting it, cleaning it, sanitizing (for regulatory/compliance purposes) Model-centric approach: Use ImageNet or an open-source dataset, that’ll be fine! Data-centric AI model approach: Make every effort to source proprietary datasets that align with the goals and use case of the computer vision project. Although a seemingly unimportant concern, the first and most crucial step for data-centric AI is securing a high-quality source of data or access to a proprietary data pipeline that aligns with the project goals and use case. In our experience, the main way to predict whether a computer vision project will succeed is the team's ability to source the best datasets possible (best in combining both quantity and quality). Sometimes through partnerships or more creative methods, such as sophisticated data scraping, structural advantages (e.g., access to Google datasets), or sheer force of will. From the clients Encord has worked with, we’ve seen that the investment in sourcing the best dataset was always worth the outcome. Sourcing high-quality data also creates positive externalities because better data attracts more skilled data scientists, data engineers, and ML engineers. Once you’ve got the datasets, whether image- or video-based, it needs to be cleaned and cleansed so it’s ready for the annotation and labeling part of the process. Raw unprocessed data often violate legal, privacy, or other regulatory restrictions. Most data operations leaders are prepared to handle these challenges. A team is assembled, either internally or externally, to clean the data and prepare it for annotation and labeling. Training Datasets for Machine Learning: The Complete Guide Managing image and video-based datasets Includes: Storage, querying, sampling, augmenting, and curating datasets. Model-centric approach: Querying and slicing data in efficient ways is not necessary, I will use a fixed set of data and labels for everything because my focus will be on improving my model parameters. Data-centric AI model strategy: Data retrieval and manipulation need to occur frequently and efficiently as we will be iterating through many permutations and transformations of the data. Once you’ve sourced the right datasets, the next step is finding a way to manage them effectively. Data management is an undervalued part of computer vision because it’s a messy engineering task rather than mathematical formulations and algorithms. We find data scientists, not data engineers often design data systems. More times than we would like, we’ve seen annotations in text files dumped into random Amazon S3 folders alongside an unstructured assortment of images or videos. This is mainly due to the philosophy that if the data is accessible somehow, it should be fine. Unfortunately, this inflexibility slows down the data-centric development process because of inefficient data access. A data-centric approach maps out management solutions from the beginning of the projects and ensures all valuable utilities are included. Sometimes, that might be finding ways to create more data through augmentations and synthetic data creation. Other times, it will involve removing data (images, videos, and other data as needed) through sampling and pruning. Within the Large Hadron Collider( probably the most sophisticated data collection device on the planet), for instance, over 99.99% of the data is thrown away and never analyzed. This is not a random decision, of course, but it is part of the careful management of a system that produces around 100 petabytes yearly. From a practical perspective, this means investing in data engineering early. This can be in talent or in external solutions; just make sure to future-proof your data systems, and don’t leave it to the hands of a mathematics Ph.D. (said by a former physics Ph.D.). Open-source Large Hadron Collider data from CERN Source Annotating and Reviewing Datasets Using Artificial Intelligence (This is effectively two stages: Annotating and reviewing; however, we've grouped them together as they usually move swiftly from one to the next in the SMART data-centric pipeline) Includes: Schema specification, pipeline design, manual and automated labeling, label, and model evaluation Model-centric approach: Get to model development quicker by using an open source labeled dataset, or, if one is not available for your problem, pay a bunch of people to label stuff, and now you have labels you can use forever. Data-centric AI model approach: Annotation is a continuous iterative workflow process and should be informed by model performance. One of the biggest misconceptions about annotation is that it’s a one-off process. The model-centric view is you create a static set of labels for a project and then build a production model by optimizing parameters and hyper-parameters through permutations of train, testing, and validating these labels and annotations. It’s clear where this perception originates. This is the standard operating procedure for academic AI work. Academics tend lean on benchmark datasets to compare their results against a body of existing work run on the same datasets. For practical applications and business use cases, this approach doesn’t work. The real-world, unfortunately, doesn’t look like ImageNet. It’s a mess of dynamic and imperfect datasets that can be tailored for various projects and use cases. The solution to the messiness of real-world datasets is maintenance. Continuous annotation is the maintenance layer of AI. Robust data annotation pipelines and workflows are iterative and contain processes that include annotation, labeling, quality control, and assurance to ensure ground truth quality and input from existing models and intelligence. This ensures that AI models can adapt to the flow of new labels and data. The most maintainable AI systems are designed to accommodate these continuous processes and make the most of these active learning pipelines. For industrial AI and any computer vision model that’s being designed and built by an organization is that intellectual property can be developed during the labeling process itself. In the world of data-centric AI, the label structures you use are in themselves architectural design choices that may give your system competitive advantages. Using common ontologies or open-source labels removes this potential advantage. These choices often require some empirical analysis to get right. Similar to how data annotation pipelines should be iterative, converging on the right label structure should itself also be an iterative process guided by experimentation. Training Computer Vision Models with a data-centric approach Includes: Data splitting, efficient data loading, training and re-training, and active learning pipelines. Model-centric AI: I trained my model and see the results in weights and biases! Hmm, they don’t look good, let me write some code to fix it. Data-centric AI & CV models: I trained my model and see the results in weights and biases! Hmm, they don’t look good, let me check my dataset to see what’s wrong. The model training and validation processes look very similar for both model-centric and data-centric approaches. The major difference is the first place a data scientist looks when they go to improve performance. A model-centric view will unsurprisingly check the model. Is there a bug in the model code? Did I use a wide enough scope of hyperparameters? Should I turn on batch normalization? A data-centric view will (also unsurprisingly) focus on the data. Did I train on the right data? Is this failing for specific subsets of the data? Are there errors in my annotations and labels? Using the data-centric approach, start with the datasets when looking for performance improvements post-training. Poor performance and accuracy outputs can originate from a wide range of potential issues, but the strategy behind taking a data-centric AI approach is that to build high-performance AI systems, much more care needs to go into getting the data layer right. Failure modes in this domain can be quite subtle, so careful thought is often required and can lead to deeper insight and understanding of the problems a model is encountering. Because it’s subtle, debugging your data after training also requires lining up all of the above steps of the SMART pipeline correctly. And like most of the other steps, training is not a one-off process in the pipeline, but dynamic and iterative and feeding the other steps. Training is not the end of a linear pipeline, only the middle of a circular one. Key Takeaways: Advantages of the data-centric approach to AI For those wanting to take a more effective data-centric AI approach, here are the steps you need to follow: Find clever ways to source your high-quality proprietary datasets Invest in good data engineering resources for dataset management Setup continuous annotation generating and monitoring pipelines Think about debugging your data first, before your models While seemingly obvious, there is no shortage of companies that we have seen that fail to think about many of the points above. They don’t realize that they don’t necessarily need to have smarter or more sophisticated models than their competitors, they just need better data than they do. While probably not as ostensibly fun as reading a paper about the latest model that improved on an open-source benchmark, a data-centric approach is our best bet to make AI a practical reality for the everyday world. Ready to accelerate and automate your data annotation and labeling? Sign-up for an Encord Free Trial: The Active Learning Platform for Computer Vision, used by the world’s leading computer vision teams. AI-assisted labeling, model training & diagnostics, find & fix dataset errors and biases, all in one collaborative active learning platform, to get to production AI faster. Try Encord for Free Today. Want to stay updated? Follow us on Twitter and LinkedIn for more content on computer vision, training data, and active learning. Join the Slack community to chat and connect.
Nov 10 2022
4 M
Software To Help You Turn Your Data Into AI
Forget fragmented workflows, annotation tools, and Notebooks for building AI applications. Encord Data Engine accelerates every step of taking your model into production.