Contents
Use Case: Sports Analytics
Problem: Scaling In-house Tools
Solution: Using API Access and Object Detection
Results: Start Collecting Data Immediately
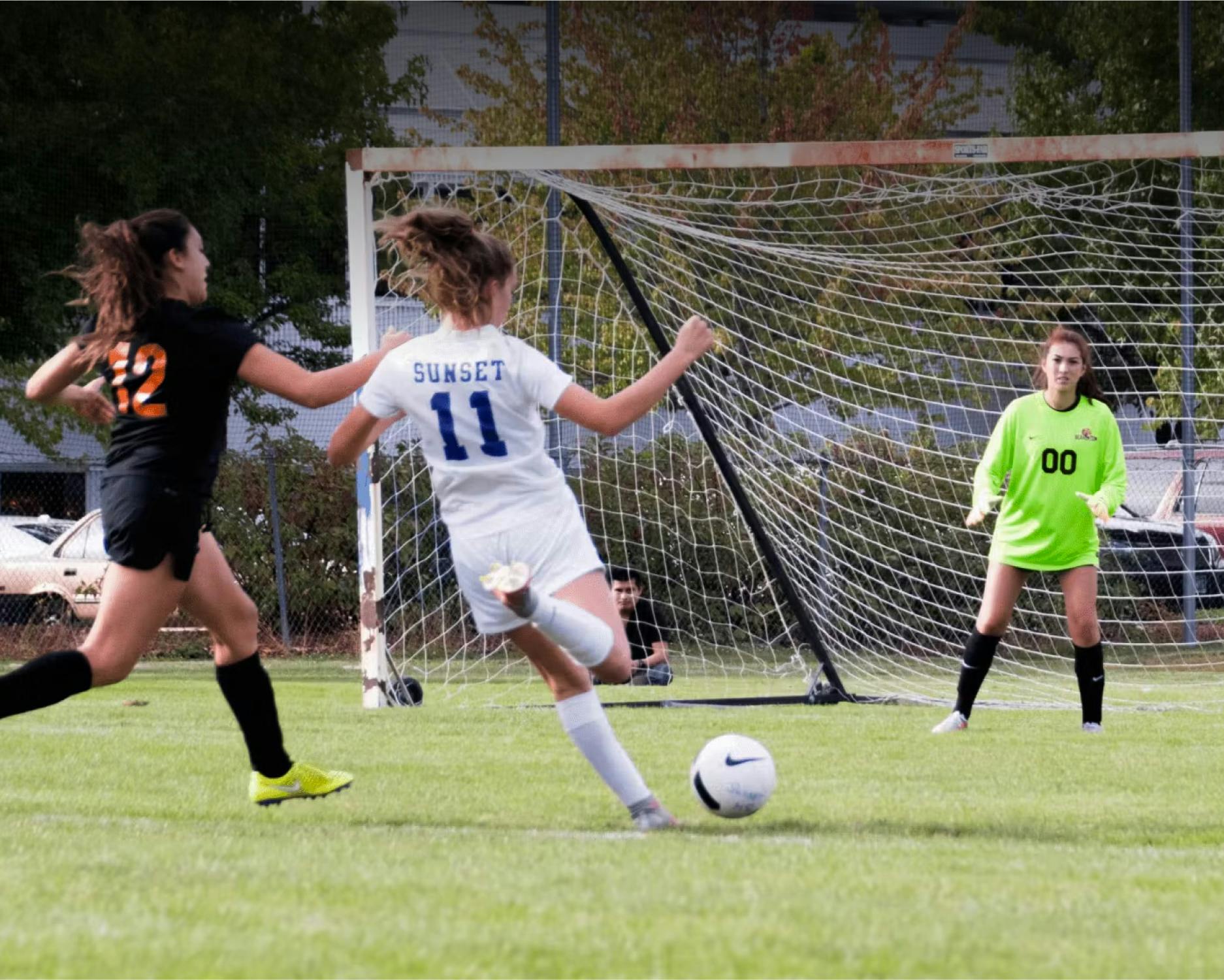
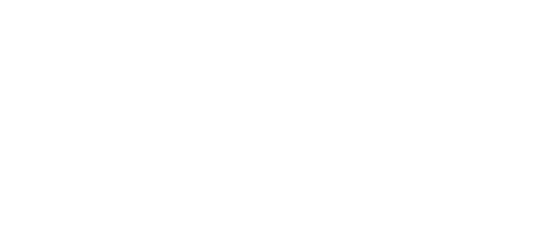
Rapid Annotation & Flexible Ontology for a Sports Tech Startup
A leading sports analytics company needed to label large amounts of video footage to train its models to identify and analyze key moments in matches. Labeling data quickly is key, but when the company’s rapid in-house annotation tool limited its ML team’s ability to iterate and develop new features, they turned to Encord to annotate at speed and build new ontologies quickly.
Use Case: Sports Analytics
This leading sports analytics company’s computer vision technology allows teams to record, live stream, and analyze professional quality videos of their matches. It detects key events, such as free kicks and goals, and player positions. Players and coaches can interact with the recording, look at stats, and review critical moments from the match to improve their skills.
Problem: Scaling In-house Tools
When labeling video datasets, the company’s annotation team must click within each frame to mark players and objects.
At first, the company built its own in-house tool and interface for data annotation. While the tool worked well for labeling at speed, it had limitations: it took months to build and refine, and the result was a single-purpose tool.
Having been built for a specific annotation task, the tool could only label certain objects, which restricted the machine learning team from trying new models, working on new problems, and building out new products and features. Spending months building an in-house tool for each specific annotation task wasn’t a feasible, sustainable, or scalable strategy.
Solution: Using API Access and Object Detection
The company’s annotation team has a workload that consists of approximately two million clicks, so speed matters. They needed a data labeling tool that allowed them to label objects rapidly as they moved quickly from frame to frame.
Before finding Encord, the team tried a couple of high-profile off-the-shelf annotation tools, but these products didn’t have a multi-frame object detection feature. Most of them focused on fancy annotation features without prioritizing the importance of annotating at speed. For this sports analytics company, spending several seconds to click and select an object – as opposed to spending only one second to click and select an object – significantly and negatively affected the rate at which they could train and develop models.
Speed matters most to the company because if the annotation team can label 10 times faster, then they can annotate 10 times as many frames. In other products, it wasn’t possible to quickly track an object across frames with a single click per frame, but Encord built out a feature to do exactly what the company needed.
The team could easily add action classifications to the labeled data, providing the ML team with important information about cameras and frame timestamps.
Another benefit of working with Encord was that its API allowed the company to take the datasets already annotated with the in-house tool and upload them into the platform. That meant that the company’s previous annotation effort was not wasted because the ML team could continue to use and expand upon the old data using Encord.
Because Encord’s user-friendly interface allows for multiple annotators to use the platform at the same time, the company could also continue to use the external annotation team with whom they had worked and built trust for years.
Annotating a video of a soccer match in Encord
Results: Start Collecting Data Immediately
While building a new annotation-specific tool in-house takes months, Encord can build out a new ontology in a matter of minutes. Having this flexible ontology enables this company’s ML teams to try out different ontologies, work with new data types, and explore different ways of annotating to learn what works best. Before using Encord, the ML team had to take the safe route because of the high cost of pursuing new ideas that failed. With a multi-purpose, cost-effective annotation tool, they can now iterate on ideas and be more adventurous in developing new products and features.
As the company continues to build analysis features for additional sports, using Encord’s flexible, multi-purpose annotation tools will help them move with speed to create new training datasets for identifying key sports events such as jump balls, faceoffs, and touchdowns. By harnessing the power of data-centric AI, this computer vision technology is on the path to giving players around the world the AI-powered tools they need to improve their games.
Explore our products
Yes. In addition to being able to train models & run inference using our platform, you can either import model predictions via our APIs & Python SDK, integrate your model in the Encord annotation interface if it is deployed via API, or upload your own model weights.
At Encord, we take our security commitments very seriously. When working with us and using our services, you can ensure your and your customer's data is safe and secure. You always own labels, data & models, and Encord never shares any of your data with any third party. Encord is hosted securely on the Google Cloud Platform (GCP). Encord native integrations with private cloud buckets, ensuring that data never has to leave your own storage facility.
Any data passing through the Encord platform is encrypted both in-transit using TLS and at rest.
Encord is HIPAA&GDPR compliant, and maintains SOC2 Type II certification. Learn more about data security at Encord here.Yes. If you believe you’ve discovered a bug in Encord’s security, please get in touch at security@encord.com. Our security team promptly investigates all reported issues. Learn more about data security at Encord here.
Yes - we offer managed on-demand premium labeling-as-a-service designed to meet your specific business objectives and offer our expert support to help you meet your goals. Our active learning platform and suite of tools are designed to automate the annotation process and maximise the ROI of each human input. The purpose of our software is to help you label less data.
The best way to spend less on labeling is using purpose-built annotation software, automation features, and active learning techniques. Encord's platform provides several automation techniques, including model-assisted labeling & auto-segmentation. High-complexity use cases have seen 60-80% reduction in labeling costs.
Encord offers three different support plans: standard, premium, and enterprise support. Note that custom service agreements and uptime SLAs require an enterprise support plan. Learn more about our support plans here.