Contents
What is an image annotation tool?
Best Image Annotation Tools to Enhance Your Computer Vision Models
Best 5 free image annotation tools
Key Takeaways: Image Annotation Tools in 2024
Encord Blog
18 Best Image Annotation Tools for Computer Vision [Updated 2025]
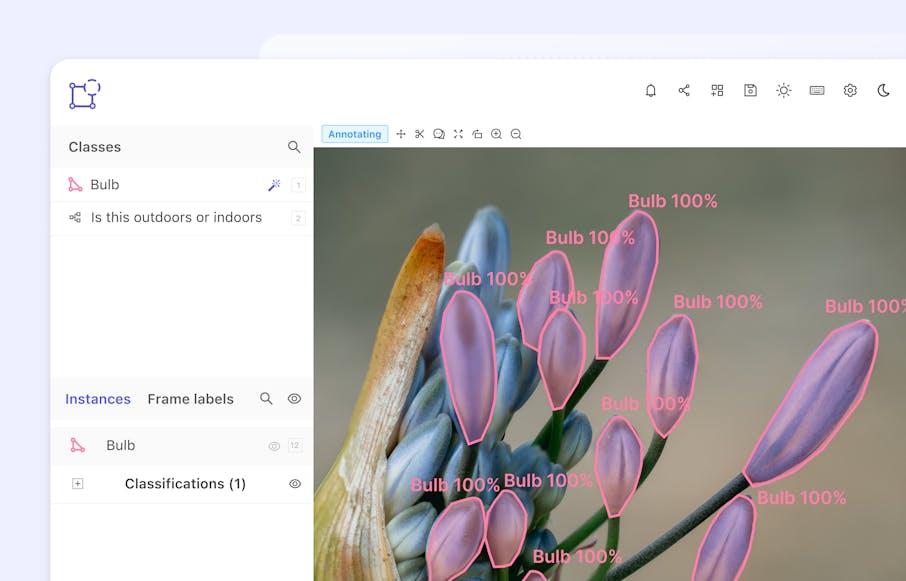
If you're looking for an image annotation tool, you have plenty of choices. The market is saturated, making it challenging to find the best tool for your needs.
To help you out, we did much of the research for you to streamline your buying process.
In this article, you will find a detailed overview of the most popular data annotation tools. If you want to try out Encord straight away, you can click the button below to try it now.
What is an image annotation tool?
An image annotation tool is used to label a set of visual data to ensure accuracy when training machine learning models. In other words, the quality of data used to train a computer vision model is directly correlated with the accuracy of its outputs. Correctly labeled data means that the computer vision model will be able to identify and classify objects. Therefore, feeding it high quality data is not only the key to success but also efficiency and cost savings.
Image annotation is applicable to a number of industries, from medical applications to retail, manufacturing and geospatial, to name a few. For example, DICOM, or Digital Imaging and Communications in Medicine, is a standard format used in the medical industry including X-rays, CT scans, MRI scans, and ultrasound images. Applications cover a wide range from medicine, to geo spacial imaging to the development of AI photo editor tools that automate photo editing. When annotated and trained, computer vision algorithms can interpret important metadata for image segmentation, object detection, image registration, disease diagnosis, and treatment planning.
While labeling data is the foundation for training machine learning models, errors can arise because it often relies on human annotators, which is why having a tool to streamline and improve this process is key.
Best Image Annotation Tools to Enhance Your Computer Vision Models
The sections below give an overview of the key features and user reviews for the above mentioned tools.
The summary table below compares all the tools based on supported data types, annotation types, ease of use, and automation.
Platform | Supported Data Types | Annotation Methods | Ease-of-use | Automation |
Encord | Image, video, and DICOM, SAR, documents | Bounding boxes, rotating boxes, polygons, polylines, keypoints, segmentation masks, frame classification | Easy-to-use, no code interface | Offers AI-based models for automated labeling and trainable micro-models for use-case specific labeling |
Amazon SageMaker Ground Truth | Image, video, text, and point-cloud | Bounding boxes, segmentation mask, named entity recognition, object tracking | Difficult to navigate | Limited Automation |
Scale Rapid | Image, video, text, documents, and audio | Bounding boxes, polygon, keypoint, ellipse, cuboid, line, named entity recognition | Difficult to navigate | Limited automation |
Supervisely | Image, video, point-cloud and DICOM | Bounding boxes, polygons, brush, pen, mask, keypoint, polylines, cuboids | Easy-to-use | Offers a smart tool based on trainable neural networks |
Labelbox | Image, video, text, audio | Bounding boxes, polygon, polyline, points, cuboid, segmentation mask, radio classification | Easy-to-use | Offers model-assisted labeling with a set ontology |
Playment | Image, video, point-cloud | Bounding boxes, ploygons, segmentation, polylines, object tracking | Easy-to-use | Offers a fully managed labeling service |
Appen | Text, image, audio, video | Bounding boxes, polygons, polylines, semantics segmentation, sentiment annotation | Easy-to-use | Offers a fully managed labeling service |
Dataloop | Image, video | Bounding boxes, polygons, ellipses, polylines, semantic segmentation, scene classification, object tracking | Easy-to-use | Offers AI-assisted magic tools and models for automated labeling |
SuperAnnotate | Image, text, video, audio | Bounding boxes, rotated box, polyline, polygon, ellipse, cuboid | Easy-to-use | Offers auto-track and AI-assisted magic tools to automated labeling |
V7 | Image, video, DICOM | Bounding boxes, key points, segmentation, keyframes | Easy-to-use | Offers AI-based auto-annotate and auto-track features to speed up image and video annotation |
Hive | Image, text, audio | Image, text, and audio classification to highlight explicit content | Difficult to navigate | Offers pre-trained models to flag harmful content |
Label Studio | Image, audio, text, video | Bounding boxes, polygons, circles, key points, segmentation, entity recognition, object tracking | Difficult to navigate | Offers ML-assisted labeling for predicting labels and using active learning pipelines |
COCO Annotator | Image | Bounding box, segmentation mask, key points | Easy-to-use | Offers deep learning models for automatic object selection |
Encord
Encord is an end-to-end data development platform with an advanced image annotation tool for complex computer vision and multimodal use cases. The platform offers state-of-the-art model-assisted labeling and customizable workflows to accelerate image annotation projects and build production-ready models.
Key Features
- AI-assisted labeling: Automate 97% of your image annotations with 99% accuracy by leveraging SOTA automated labeling capabilities such as Meta AI’s Segment Anything Model (SAM).
- Full suite of tools: Encord supports a range of labeling options, such as bounding boxes, rotatable boxes, polygons, polylines, key points, and classifications to support your model requirements.
- Accelerate with models-in-the-loop: Bring your own model to the Encord platform or leverage one of our Agents to pre-label datasets.
- Scalability: Encord lets you scale AI projects by supporting extensive datasets of up to 500,000 images.
- Build balanced datasets: Filter and slice datasets in a consolidated visual explorer and export for labeling in one click. Encord supports deep search, filtering, and metadata analysis.
- Complex ontologies: Build nested relationship structures in your data schema to improve the quality of your model output.
- Bulk classification: Leverage natural language or similarity search to select large datasets and label en masse, queue for review to accelerate labeling operations.
- Build reliable quality control workflows: Build robust workflows with multi-step review stages and consensus benchmarking for quality assurance.
- Find and fix label errors: Automatically surface labeling errors to shift your attention to the labels impacting model performance.
- Collaboration: Control user roles with permissions, manage task assignments and infinitely scale your MLOps workflows.
- Enterprise-grade security as standard: Encord Annotate complies with the General Data Protection Regulation (GDPR), System and Organization Controls 2 (SOC 2), and Health Insurance Portability and Accountability Act (HIPAA) standards while using advanced encryption protocols to ensure data privacy.
- Integrations: Encord allows you to retain total control of your data. Securely connect your native cloud storage buckets and programmatically control workflows. Advanced Python SDK and API access with easy export into JSON and COCO formats.
- Integrated Data Labeling Services: Outsource your labeling tasks to an expert workforce of vetted, trained, and specialized annotators.
Modalities Covered
- Image
- Video
- DICOM
- SAR
- Documents
- Audio
G2 Review Summary
Encord has a rating of 4.8/5 based on 60 reviews. Users prefer Encord’s powerful ontology feature, which lets them define rich taxonomy for all data sizes. In addition, the platform’s collaborative features and granular annotation tools help users improve annotation quality.
Amazon SageMaker Ground Truth
Amazon SageMaker Ground Truth is a human-in-the-loop data labeling platform that offers features to label large datasets. It provides a self-serve and a managed service option to help you streamline your annotation workflow for multiple CV tasks.
Key Features
- Data Generation: The platform offers tools to fine-tune pre-trained models on a few data points to generate synthetic data samples for more diverse training.
- Model Evaluation: Sagemaker Ground Truth lets you evaluate foundation models based on multiple metrics such as accuracy, relevancy, toxicity, and bias through human feedback.
- Labeling Templates: It features over thirty labeling templates for multiple CV and NLP tasks, including image classification, object detection, text classification, and named entity recognition (NER).
- Interactive Dashboards: The tool offers intuitive dashboards and user-friendly interfaces to monitor labeling progress across multiple projects.
Modalities Covered
- Image
- Video
- Text
- Point-cloud
Pros & Cons
Pros:
- Automated labeling
- Support for multiple data types
- Customizable labeling workflow
- Integration with Amazon SageMaker
Cons:
- Using it in combination with non-AWS services may introduce friction
- Limited control over labelers
- Setup requires familiarity with AWS’s IAM policies, permissions, and general AWS environment
- May not have the depth of customization some niche or complex projects require
G2 Review Summary
Amazon SageMaker Ground Truth has a rating of 4.1/5 based on 19 reviews. Users like its ease of use and advanced annotation capabilities. However, they feel it is expensive, and tracking labeling performance is challenging.
Scale Rapid
Scale Rapid is a data and labeling services platform that supports computer vision use cases. It specializes in reinforcement learning with human feedback (RLHF), user experience optimization, large language models (LLMs), and synthetic data.
Key Features
- Supported Data Types: Scale lets you annotate text, images, video, audio, and point-cloud data.
- Customizable Workflows: Offers customizable labeling workflows tailored to specific project requirements and use cases.
- Data labeling services: Provides high-quality data labeling services for various data types, including images, text, audio, and video.
- Scalability: Capable of handling large-scale annotation projects and accommodating growing datasets and annotation needs.
Modalities Covered
- Image
- Video
- Test
- Documents
- Audio
Pros & Cons
Pros:
- High-quality annotations with human-in-the-loop labeling
- Optimized for speed, providing fast delivery times even on large datasets
- Supports a range of complex data types, including 3D point clouds and LiDAR data
- Built-in quality control measures
Cons:
- May not offer the depth of customization needed for highly specific or unconventional labeling tasks
- Not as deeply integrated with automated labeling as some competitors
- Does not integrate directly into machine learning pipelines
G2 Review Summary
Scale Rapid has a rating of 4.4/5 based on 11 reviews. Users say it is easy to learn and does not require complex installation procedures. However, they feel the user interface is clunky and the tool’s pricing is complex.
Supervisely
Supervisely is an end-to-end computer vision platform that offers multiple annotation tools for labeling images and videos. It features AI-based labeling that lets users automate labeling workflow through advanced ML models.
Key Features
- Versatile Annotation Tool: It supports multiple annotation types, including bounding boxes, polygons, polylines, points, and segmentation masks for precise labeling.
- Supported Data Types: Supervisely lets you label images, videos, point cloud, and medical image data.
- Smart Labeling Tools: Feature a class-agnostic smart tool based on customizable neural networks for capturing any object type, depending on your use case.
- Collaboration: The platform lets you collaborate with team members and assign relevant user roles to track issues and labeling performance.
Modalities Covered
- Image
- Video
- Point-Cloud
- DICOM
Pros & Cons
Pros:
- Interface is intuitive and highly visual
- Offers specialized tools for advanced annotation types, such as semantic segmentation
- Incorporates AI-assisted labeling tools
- Users can create custom plugins and scripts
Cons:
- Does not provide a built-in labeling workforce
- Lacks some advanced workflow automation features
G2 Review Summary
Supervisely has a rating of 4.7/5 based on ten reviews. Users like the tool’s integration with multiple apps within the Supervisely ecosystem, giving a smooth user experience. However, the number of options can be overwhelming, and the platform has latency issues.
Labelbox
Labelbox is a US-based data annotation platform founded in 2017 that provides a unified framework for curating and labeling datasets with collaboration and model evaluation tools.
Besides a stand-alone image labeling platform, the tool offers managed annotation services with data labeling experts.
Labelbox
Key Features
- Data Management: Labelbox offers QA workflows and data annotator performance tracking.
- Customizable Labeling Interface: It features a user-friendly interface, providing easy-to-navigate editors for specific needs.
- Automation: Allows integration with AI models for automatic data labeling to accelerate the annotation process.
- Annotation Capabilities: It supports annotation for multiple data types beyond images, including text, video, audio, geospatial and medical images.
Modalities Covered
- Image
- Video
- Text
- Audio
Pros & Cons
Pros:
- Has quality assurance tools, such as consensus scoring and annotation reviews
- Includes tools for AI-assisted labeling
- Integrates with popular machine learning frameworks and platforms
Cons:
- May not offer the depth of customization required for highly specialized workflows
- Heavily cloud-based, which may pose challenges in industries with strict data governance
- Working with high-resolution images or video data may sometimes impact platform performance
G2 Review Summary
LabelBox has a rating of 4.7/5 based on 33 reviews. Users find the tool’s data management features helpful. However, they feel that it does not perform well with high-resolution images.
Playment
Playment is an Indian-based end-to-end data annotation platform founded in 2015 and now operating under Telus’ ownership. It offers managed annotation services by employing computer vision teams to annotate training data for multiple use cases.
Key Features
- Data Labeling Services: Provides high-quality data labeling services for various data types, including images, videos, text, and sensor data.
- Support: Global workforce of contractors and data labelers.
- Scalability: Capable of handling large-scale annotation projects and accommodating growing datasets and annotation needs.
- Audio Labeling Tool: The tool features a speech recognition training platform that can handle over five hundred languages and dialects.
Modalities
- Image
- Video
- Point-cloud
Pros & Cons
Pros:
- Strong in complex use cases like autonomous driving, geospatial analysis, and indoor mapping
- Includes human-in-the-loop processes with quality control mechanisms
- Multiple quality control layers, including auditing, consensus scoring, and feedback loops
- Allows for some customization in workflows
Cons:
- Lacks some of the advanced AI-assisted labeling features
- Primarily a cloud-based platform, which may pose challenges for organizations with strict data privacy
G2 Review Summary
Playment has a rating of 4.7/5 based on 11 reviews. Users find Playment’s annotation performance fast and accurate. However, they find the tool expensive and that it needs more improvement in automated labeling features.
Appen
Appen is a data labeling services platform founded in 1996, making it one of the first and oldest solutions in the market, offering data labeling services for various industries. In 2019, it acquired Figure Eight to expand its software capabilities and help businesses train and improve their computer vision models.
Key Features
- Data Labeling Services: Support for multiple annotation types (bounding boxes, polygons, and image segmentation).
- Data Collection: Data sourcing (pre-labeled datasets), data preparation, and real-world model evaluation.
- Natural Language Processing: Support for natural language processing (NLP) tasks such as sentiment analysis, entity recognition, and text classification.
- Image and Video Analysis: Analyzes images and videos for tasks such as object detection, image classification, and video segmentation.
Modalities Covered
- Text
- Image
- Audio
- Video
Pros & Cons
Pros:
- Employs quality control processes like consensus scoring, accuracy monitoring, and annotation reviews
- Can integrate with popular machine learning tools and platforms
- Expertise in multilingual data labeling
Cons:
- Not fully designed for real-time feedback or iterative adjustments
- Relies on its own managed workforce and may lack the integration options needed for companies wanting to use their own in-house teams
G2 Review Summary
Appen has a rating of 4.2/5 based on 28 reviews. Users like that the tool is web-based and does not require specific installation procedures. However, the platform’s server crashes frequently, and the support team is slow to respond.
Dataloop
Dataloop is an Israel-based data labeling platform that provides a comprehensive solution for data management and annotation projects. The tool offers data labeling capabilities across images, text, audio, and video annotation, helping businesses train and improve their machine learning models.
Key Features
- Data Annotation: Supports multiple image annotation tasks, including classification, detection, and semantic segmentation.
- Collaboration Tool: It features tools for real-time collaboration among annotators, project sharing, and version control, allowing for efficient teamwork.
- Data Management: Offers data management capabilities, including data versioning, tracking, and organization for streamlined workflows.
- Model Management: Dataloop offers tools to manage different model versions and download SOTA models from the Model Marketplace.
Modalities Covered
- Image
- Video
Pros & Cons
Pros:
- Supports a wide variety of annotation types
- AI-assisted labeling features
- Has quality control mechanisms, including annotation reviews and consensus checks
- Integrates with popular machine learning tools and platforms
Cons:
- Highly specific workflows or niche annotation requirements may require additional customization
- Relatively limited tools and support for natural language processing (NLP) or audio data
G2 Review Summary
Dataloop has a rating of 4.4/5 based on 90 reviews. The tool’s plus points include its ease of use and annotation efficiency. However, users find it challenging to learn and face frequent performance issues.
SuperAnnotate
SuperAnnotate is an end-to-end AI platform that offers tools for data curation and automatic annotation with MLOps functionalities. It also lets you fine-tune LLMs using annotated data and RLHF.
Key Features
- Multi-Data Type Support: Versatile annotation features for labeling videos, text, audio, and image data.
- AI Assistance: Integrates AI-assisted annotation to accelerate the labeling process and improve efficiency.
- Customization: Provides customizable annotation interfaces and workflows to tailor annotation tasks to specific project requirements.
- Export Formats: SuperAnnotate supports multiple data formats, including popular ones like JSON, COCO, and Pascal VOC.
Modalities Covered
- Image
- Text
- Video
- Audio
Pros & Cons
Pros:
- Supports a wide range of annotation types
- Includes AI-assisted labeling features
- Integrates with popular machine learning frameworks
Cons:
- Doesn’t provide built-in annotators
- Not as specialized for natural language processing (NLP) tasks as other platforms
- Challenges with large video datasets or high-resolution media
G2 Review Summary
SuperAnnotate has a rating of 4.9/5 based on 137 reviews. Users find the tool’s feature set comprehensive and the interface intuitive. However, there have been complaints regarding its custom workflow setup and high price.
V7 Labs
V7 is a UK-based data annotation platform founded in 2018. The company enables teams to annotate image and video data using automated pipelines and custom workflows. The platform also offers model and data management tools to help users build high-quality training data for scalable AI projects.
Key Features
- Collaboration Capabilities: Project management and automation workflow functionality, with real-time collaboration and tagging.
- Data Management: The tool offers data management features, including functionalities to filter and sort data. It also helps organize and manage data classes at team and dataset levels.
- Auto-Annotate: Features auto-annotation that lets you use deep learning models to create pixel-perfect polygon masks.
- Auto-Track: V7 offers an auto-track feature for object tracking and instance segmentation in long videos.
Modalities Covered
- Image
- Video
- DICOM
Pros & Cons
Pros:
- Advanced AI-assisted labeling features
- Supports a variety of annotation types including bounding boxes, polygons and key points
- Integrates with popular machine learning frameworks and cloud storage providers
Cons:
- Primarily focuses on image and video data and is not optimized for NLP or audio tasks
- Some of the AI-assisted features and integrations may be limited in on-premises deployments, as these rely on cloud infrastructure
G2 Review Summary
V7 has a rating of 4.8/5 based on 52 reviews. Users find its automation and collaboration features significantly helpful. However, they feel it lacks file manipulation options, and its sorting and filtering features do not perform well with large files.
Hive
Hive is a content-moderation platform that offers deep learning models to highlight harmful and explicit content in images, videos, text, and audio. It also features search and generative APIs to visualize similarities between images and videos and generate images based on textual prompts.
Key Features
- Ease of use: Hive offers an intuitive interface with multiple in-built image and text classification models.
- Embeddings: The platform lets you quickly create text embeddings to build retrieval augmented generation (RAG)-based LLMs.
- Search: Hive offers versatile web search functionality. You can use image prompts to retrieve relevant links to similar images.
- Generative Artificial Intelligence (Gen AI): Hive features APIs to generate text, images, and videos based on textual prompts.
Modalities Covered
- Image
- Text
- Audio
Pros & Cons
Pros:
- Can perform real-time moderation on images
- Allows users to create custom moderation categories and rules
- Includes robust API options for seamless integration into existing workflows
Cons:
- Complex cases still need human oversight
- May not be as effective in niche areas or for highly specific content categories
- Some customization challenges, such as specialized moderation workflows.
G2 Review Summary
Hive has a rating of 4.6/5 based on 528 reviews. Users find its project management and collaboration features helpful. However, the interface is challenging to navigate and has a few glitches, which makes it complex to operate.
Label Studio
Label Studio is a popular open-source data labeling platform for annotating various data types, including images, text, audio, and video. It supports collaborative labeling, custom labeling interfaces, and integration with machine learning (ML) pipelines for data annotation tasks.
Key Features
- Customizable Labeling Interfaces: Label Studio lets you label data through flexible configurations that allow you to tailor annotation interfaces to specific tasks.
- Collaboration Tools: Real-time annotation and project-sharing capabilities for seamless collaboration among annotators.
- Export Formats: Label Studio supports multiple data formats, including JSON, CSV, TSV, and VOC XML like Pascal VOC, facilitating integration and annotation from diverse sources for machine learning tasks.
- ML Pipelines: Label Studio lets you connect the model development pipeline with the data labeling project. The method allows you to use ML models to predict labels, evaluate model performance, and perform human-in-the-loop labeling.
Modalities Covered
- Image
- Audio
- Text
- Video
Pros & Cons
Pros:
- The platform is open-source allowing users to download, customize, and run it without licensing fees
- Supports multiple data types and annotation types
- Customizable UI that allows users to create unique annotation interfaces tailored to specific project requirements
- Integration with machine learning workflows and other platforms
Cons:
- Users who need pre-labeling or AI-powered annotation support will need to integrate third-party tools or custom models
- Not optimized for high-throughput, real-time labeling at scale
- Limited support for advanced project management features such as workflow automation and task prioritization
G2 Review Summary
G2 review not available.
COCO Annotator
COCO Annotator is a web-based labeling tool by Justin Brooks that is under the MIT license. The tool helps streamline the process of annotating images for object recognition, localization, and key point detection models. It also offers a range of features that cater to the diverse needs of machine learning practitioners, data scientists, and researchers.
Key Features
- Image Annotation: Supports annotation of images for object detection, instance segmentation, keypoint detection, and captioning tasks.
- Export Formats: The tool exports and stores annotations in the COCO format to facilitate large-scale object detection.
- Automation: The tool makes annotating an image easier by incorporating semi-trained models. It also provides access to advanced selection tools, including the Mask Region-based Convolutional Neural Network (MaskRCNN), Magic Wand, and Deep Extreme Cut (DEXTR) frameworks.
- Metadata Management: Users can create custom metadata for each instance or object.
Modalities Covered
- Image
Pros & Cons
Pros:
- Easy to work with COCO datasets or other systems that use this format
- Supports various annotation types
- Open source which allows users to meet specific project requirements
Cons:
- Lacks support for audio, video, and text annotations
- Does not include advanced quality control tools like consensus scoring and review workflows
- May not perform optimally with very large datasets or a high volume of concurrent users
- Does not have built-in AI-assisted labeling tools
G2 Review Summary
G2 review not available.
Best 5 free image annotation tools
The sections below provide an overview of the best free image annotation tools, including the features they offer and how they’re reviewed.
The summary table below compares our top picks.
Platform | Supported Data Types | Annotation Methods | Ease-of-use | Automation |
CVAT | Image, video | Bounding boxes, polygons, polylines, points, ellipses, cuboids, skeleton | Difficult to navigate | Offers integration with deep learning models for semi-automatic and automatic annotation |
LabelMe | Image, video | Polygons, bounding boxes, circles, polylines, key points | Easy-to-use | Limited automation |
Make Sense | Image | Bounding boxes, polylines, polygons, key points | Easy-to-use | Offers integrated pre-trained models to automatically draw bounding boxes and estimate pose |
VGG Image Annotator | Image, audio, video | Bounding boxes, circles, ellipses, polygons, key points, polylines, speech segmentation | Easy-to-use | Limited automation |
VoTT (Visual Object Tagging Tool) | Image, video | rectangle and polygon bounding boxes | Easy-to-use | AI-assisted labeling capabilities |
LabelMe
LabelMe is an open-source web-based tool by the MIT Computer Science and Artificial Intelligence Laboratory (CSAIL) that allows users to label and annotate images for computer vision research. It provides a user-friendly interface for drawing bounding boxes, polygons, and semantic segmentation masks to label objects within images.
Key Features
- Web-Based: Accessible through a web-based interface, allowing you to perform annotation tasks in any modern web browser without requiring software installation.
- Supported Data Types: The tool supports image and video annotation.
- Supported Annotation Types: LabelMe lets you draw polygons, rectangles, circles, lines, and points.
- Export Format: It lets you export annotation in VOC and COCO format for semantic and instance segmentation.
Modalities Covered
- Image
- Video
Pros & Cons
Pros:
- Free to use and offers flexibility for teams and researchers with limited budgets
- Can be self-hosted, giving users complete control over data privacy and security
- Developers can modify to add features or customize its functionality
Cons:
- Lacks AI-assisted labeling features like auto-segmentation or object tracking
- Lacks quality control and collaboration features
- Requires manual data uploading and exporting
G2 Review Summary
G2 review not available.
CVAT (Computer Vision Annotation Tool)
CVAT is an open-source web-based image annotation tool by Intel. In 2022, CVAT’s data, content, and GitHub repository became a part of OpenCV, where CVAT continues to be open-source. Furthermore, CVAT can also help annotate QR codes within images, facilitating the integration of QR code recognition into computer vision pipelines and applications.
Key Features
- Manual Annotation Tools: The tool supports various annotation types, including bounding boxes, polygons, polylines, points, and cuboids, catering to diverse annotation needs.
- Multi-platform Compatibility: Works on multiple operating systems such as Windows, Linux, and macOS, providing flexibility for users.
- Export Formats: CVAT supports numerous data formats, including JSON, COCO, and Pascal VOC, ensuring annotation compatibility with diverse tools and platforms.
- Automated Labeling: CVAT supports multiple algorithms, including the Segment Anything Model (SAM), YOLOv3, and Deep Extreme Cut (DEXTR).
Modalities Covered
- Image
- Video
Pros & Cons
Pros:
- Free to use and highly customizable
- Specialized support for video annotations with features like frame-by-frame annotation and object tracking
- Includes quality control features
- Integrates with machine learning models to provide semi-automated labeling
Cons:
- Running CVAT, especially for video annotations or large datasets, can consume considerable CPU and memory resources
- While it offers basic task assignment and review workflows, it lacks sophisticated project management features
- Doesn’t offer native integration with popular cloud storage services
G2 Review Summary
CVAT has a rating of 4.5/5 based on two reviews. Users like that the tool is free to use and requires no configuration and installation process because it is web-based. However, its slow performance and backend server failure are the most significant concerns.
Make Sense
Make Sense AI is a user-friendly open-source annotation tool available under the GPLv3 license. It is accessible through a web browser and does not require advanced installations. The tool simplifies the annotation process for multiple image types.
Key Features
- Open Sourced: Make Sense AI stands out as an open-source tool, freely available under the GPLv3 license, fostering collaboration and community engagement for its ongoing development.
- Accessibility: It ensures web-based accessibility, operating seamlessly in a web browser without complex installations, promoting ease of use across various devices.
- Export Formats: It facilitates exporting annotations in multiple formats (YOLO, VOC XML, VGG JSON, and CSV), ensuring compatibility with diverse machine learning algorithms.
- Supported Annotation Types: The tool supports rectangles, lines, points, and polygons.
Modalities Covered
- Image
Pros & Cons
Pros:
- Entirely free and open source, making it an attractive option for users on a budget, small research teams, and students
- Supports several annotation types
- Can be self-hosted, allowing users to maintain complete control over their data
Cons:
- Does not offer AI-assisted labeling features
- Lacks project management and scaling features, such as task assignment and annotation tracking
- Limited to image data only and does not support video, audio, or text annotation
G2 Review Summary
G2 review not available.
VGG Image Annotator
VGG Image Annotator (VIA) is a versatile open-source tool by the Visual Geometry Group (VGG) for manually annotating image and video data. Released under the permissive BSD-2 clause license, VIA serves the needs of academic and commercial users, offering a lightweight and accessible solution for annotation tasks.
Key Features
- Lightweight and User-Friendly: VIA is a lightweight, self-contained annotation tool that uses HTML, Javascript, and CSS without external libraries.
- Offline Capability: The tool works offline, providing a full application experience within a single HTML file of less than 200 KB.
- Audio and Video Annotation: In addition to images, the tool lets users define temporal segments in audio and video data with textual descriptions.
- Supported Annotation Types: The tool allows you to draw rectangles, circles, ellipses, polygons, points, and polylines.
Modalities Covered
- Image
- Audio
- Video
Pros & Cons
Pros:
- Entirely free and open source
- Allows users to define custom attributes for regions, which enables more detailed and structured annotations
- Annotations can be exported in JSON format
Cons:
- Lacks project management tools
- Does not support AI-assisted labeling features like automatic object detection or segmentation
- Does not natively integrate with external storage solutions or cloud platforms, so users must manually upload and manage their images
G2 Review Summary
G2 review not available.
VoTT (Visual Object Tagging Tool)
VoTT is an open-source image annotation tool that can be installed directly from Github for free. It was created by Microsoft to build object detection models from image and video data. VoTT uses active learning, a supervised approach to machine learning that uses training data optimization cycles to continuously improve the performance of an ML model. Within this feature, users can choose between a Predict Tag and Auto Detect.
Key features
- Multiformat Support: can export in Azure Custom Vision Service, CSV, CNTK, Pascal VOC, Tensorflow Records, and VoTT Json.
- Tagging Capabilities: Users can tag and annotate both Image Directories and Stand alone videos.
- Computer-Assisted Tagging & Tracking: Objects can be tagged using the Camshift tracking algorithm.
- Tag Exporting Ability: The tool allows for tags and assets to be exported to CNTK or YOLO formats
- Data and Model Validation: Trained CNTK object detection models can be validated
Modalities Covered
- Image
- Video
Pros & Cons
Pros:
- Completely free and open source
- Integrates smoothly with Microsoft Azure services, including Azure Blob Storage and Azure Machine Learning
- Includes AI-assisted labeling capabilities
Cons:
- Limited to rectangle and polygon annotations
- Lacks advanced project management features, collaboration tools, and built-in quality control
G2 Review Summary
G2 review not available.
Key Takeaways: Image Annotation Tools in 2024
As data volume and variety increase, businesses must invest in a suitable and reliable annotation tool to build high-quality datasets for training models.
Below are a few key points regarding the top image annotation tools and tips for selecting an appropriate solution.
- Best Annotation Tools in 2024: Encord, Amazon Sagemaker Ground Truth, and Scale Rapid are the top annotation tools in 2024.
- Ease-of-use: Most G2 reviews highlight issues with the user interface. You should ensure that you select a tool that offers intuitive navigation and labeling features.
- Automation: Select a platform that offers state-of-the-art automation features, including pre-trained models and smart labeling tools.
- Open-source vs. Paid Platforms: While open-source tools offer a cost-effective solution, they have limited functionality. Paid tools provide a rich feature set with robust customer support to help you annotate multiple data types.
So, streamline your CV operations with the annotation tool that best suits your needs.
Better Data, Better AI
Enhance your AI with automated data curation, annotation, and validation.
Try it todayWritten by
Nikolaj Buhl
- There are various options, including open-source, low-code or no-code, and active learning annotation solutions like Encord. Encord is the leading annotation tool to build better models, faster. Accelerate the creation of training data with pixel-perfect AI-assisted labeling to develop high quality training data and build product-ready models up to 10x faster.
- Image annotation involves several stages. Image and image-based datasets need to be sourced (either bought or downloaded from open-source databases), cleaned, and uploaded into annotation tools and software.
- Automatically annotate images with active learning annotation platforms, like Encord. Traditional computer vision models require extensive data for robustness and generalizability. You can leverage the power of the Segment Anything Model to complete single one-click annotations and in just minutes, train Encord’s micro-models starting from a small set of labels.
- Automatically annotate images with active learning annotation platforms, like Encord. Traditional computer vision models require extensive data for robustness and generalizability. You can leverage the power of the Segment Anything Model to complete single one-click annotations and in just minutes, train Encord’s micro-models starting from a small set of labels.
- You should look for versatility in annotation types (bounding boxes, polygons), user-friendly interfaces, collaboration support, scalability, automation features, and compatibility with various annotation formats for seamless integration into your workflow.
- Model-assisted labeling involves using pre-trained models to assist in the annotation process, improving efficiency by automating certain tasks and reducing manual effort.
- Encord offers a complimentary trial, followed by straightforward per-user pricing. Consider notable options like CVAT, MakeSense, and VGG Annotator, known for being open-source, web-based, supporting diverse annotation types, and offering export flexibility in various formats.
- Choose your preferred annotator, upload the image, select the annotation type (bounding box, polygon), annotate, and export labels in your chosen format. Tools like Encord offer automated annotation features, streamlining the process for added convenience.
- Auto annotation features, like the one by Encord Annotator, assist in the annotation process by leveraging semi-trained models to automatically suggest annotations, enhancing efficiency in various deep learning tasks like object detection, instance segmentation, object recognition, and localization.
- Image annotation tools facilitate collaboration by enabling multiple users to annotate images simultaneously, fostering real-time communication and feedback. They streamline the process of labeling objects within images, enhancing efficiency and accuracy in object detection and classification tasks.
Explore our products