Contents
📜 Top Picks for Computer Vision Papers This Month
🧑💻 Developer Resources You’d Find Useful
📰 Computer Vision In the News
Encord Blog
Encord Monthly Wrap: June Industry Newsletter
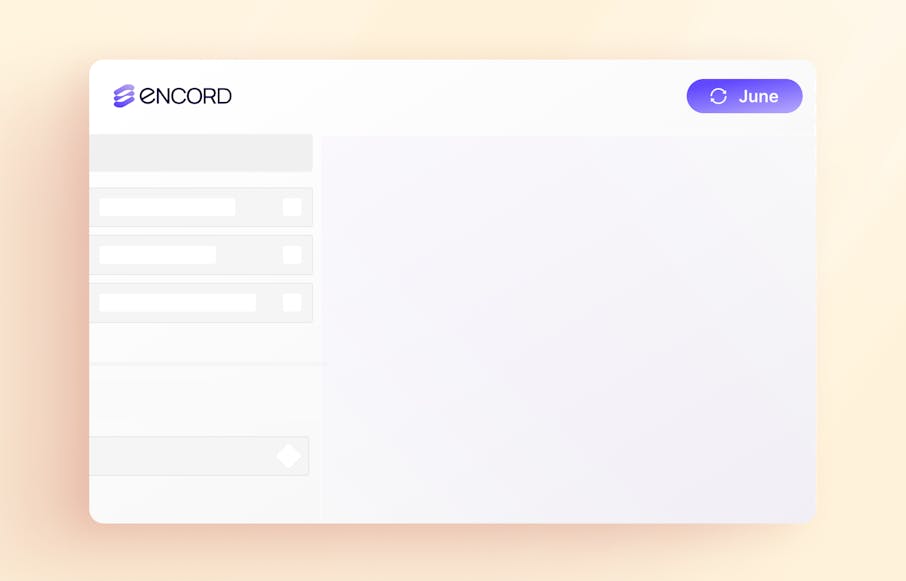
Hi there,
Welcome to the Computer Vision Monthly Wrap for June 2024!
Here’s what you should expect:
- 🎁 Automatic Data Curation for Self-Supervised Learning: A Clustering-Based Approach
- 📽️ Top CVPR 2024 papers, including the poster sessions
- ⚒️ Developer resources to use for your next vision AI application
- 🤝 New model releases in the computer vision and multimodal AI world
Let’s go! 🚀
📜 Top Picks for Computer Vision Papers This Month
Automatic Data Curation for Self-Supervised Learning: A Clustering-Based Approach
Researchers at Meta AI released a paper introducing an automatic data curation method for self-supervised learning that can create large, diverse, and balanced datasets without manual effort.
The approach in the paper uses hierarchical k-means clustering and balanced sampling to curate high-quality datasets from raw data.
The method addresses imbalanced data representation in web-based collections, ensuring a more uniform distribution of diverse concepts.
What’s impressive? 🤯
- The approach enables training self-supervised models on automatically curated datasets, which alleviates the need for costly manual labeling and curation
- Hierarchical k-means clustering obtains uniform data clusters representing different concepts
- Balanced sampling from the clusters ensures the curated dataset has an even distribution of concepts
- Experiments on images, satellite data, and text show features trained on the auto-curated datasets match or exceed the performance of features from manually curated data
How can you apply it? ⚒️
- Curate your own high-quality datasets from large raw data sources for self-supervised pre-training
- Scale up model training by avoiding the bottleneck of manual data curation
- Improve model robustness and generalization by training on diverse and balanced datasets
- Apply the technique to various domains like computer vision, earth observation (remote-sensing), and natural language processing
Frederik Hvilshøj, Lead ML Engineer at Encord, spoke to the paper's first author and distilled (yes, I don’t excuse the pun 😁) insights from the paper and conversations. Watch the video on LinkedIn.
Top Papers and Poster Sessions from CVPR 2024
CVPR 2024 was quite an experience for many researchers, stakeholders, and engineers working on computer vision and multimodal AI problems. At Encord, we even released a fun game to get you battling it out with AI to win amazing prizes! 😎.
This article reviews the top papers presented at CVPR 2024, including the research highlights. Frederik also reviewed some of the papers that were presented during the poster session:
- YOLO-World: Real-Time Open-Vocabulary Object Detection
- Putting the Object Back into Video Object Segmentation
- Panda-70M: Captioning 70M Videos with Multiple Cross- modality Teachers
- InternVL: Scaling up Vision Foundation models and Aligning for Generic Visual-Linguistic Tasks
🧑💻 Developer Resources You’d Find Useful
- Building Multi-Modal RAG Systems → Frederik Hvilshøj shared insights on a new model that could be just what you need to integrate multimodal RAGs in your apps.
- [WATCH] Interactive Tutorial On Using Gemini in Data Pipelines → Learn how to use Gemini 1.5 Pro to extract structured data from visual content with hands-on examples in this Colab notebook.
- Notebook for Fine-tune Florence-2 → The Hugging Face team and community members shared a notebook, HF Space, and a walkthrough blog on fine-tuning Florence-2 on DocVQA dataset. Check them out. New to Florence-2 from Microsoft? See this explainer blog post.
- How to Pre-Label Your Data with GPT-4o → Multimodal AI models are increasingly useful for bulk classification and pre-labeling datasets. That blog walks you through the principles behind this and shows you how to set up your own AI Agents to automate labeling
📰 Computer Vision In the News
- DeepMind’s new AI generates soundtracks and dialogue for videos → A new video-to-audio model that DeepMind developed can use a video and a soundtrack description (such as "jellyfish pulsating underwater, marine life, ocean") to produce audio that matches the video's mood, characters, and plot.
- Sensor Perception with NVIDIA’s Omniverse Cloud Sensor RTX at CVPR 2024 → At CVPR, NVIDIA showcased Omniverse microservices, including techniques and algorithms to simulate perception-based activities in realistic virtual environments before real-world deployment.
- From TechCrunch → All the rage has been about Runway’s new video-generating AI, Gen-3, which offers improved controls and more high-fidelity video generation results.
Till next month, have a super-sparkly time!
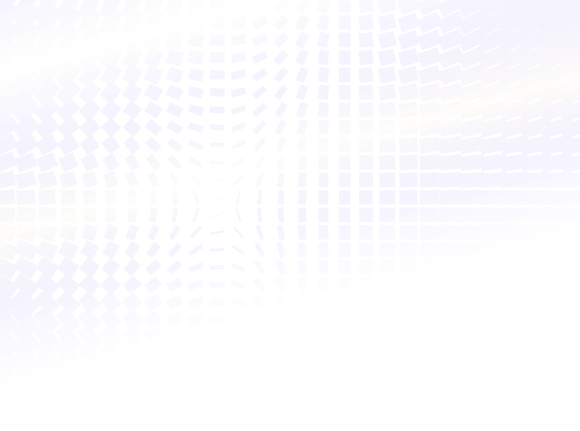
Power your AI models with the right data
Automate your data curation, annotation and label validation workflows.
Get startedWritten by

Stephen Oladele
Explore our products