Contents
What is Audio AI?
Capabilities of Audio AI
Applications of Audio AI
How Audio AI Works
Audio AI Model Architectures
Challenges of Audio AI
Encord for Audio AI
Audio AI: Key Takeaways
Encord Blog
Exploring Audio AI: From Sound Recognition to Intelligent Audio Editing
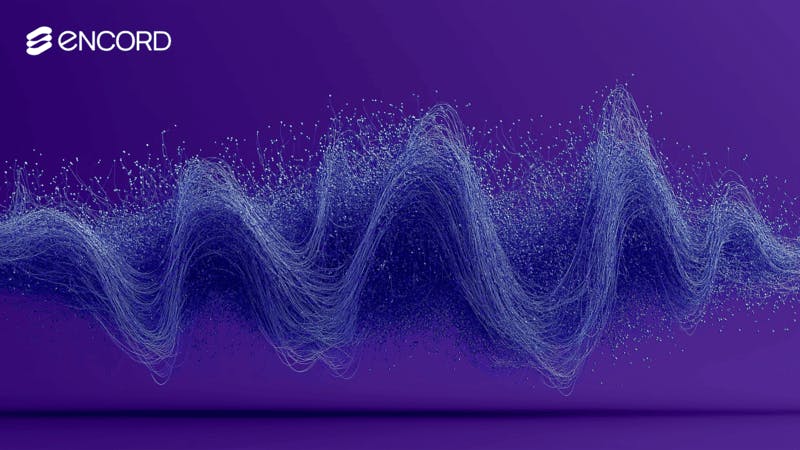
The global speech and voice recognition market was worth USD 12.62 billion in 2023. Moreover, estimates suggest it will be worth USD 26.8 billion by 2025.
The rising popularity of voice assistants results from sophisticated generative audio artificial intelligence (AI) tools that enhance human-machine interaction.
Yet, the full potential and diverse applications of audio AI remain underexplored, continually evolving to meet the shifting needs and preferences of businesses and consumers alike.
In this post, we will discuss audio AI, its capabilities, applications, inner workings, implementation challenges, and how Encord can help you curate audio data to build scalable audio AI systems.
What is Audio AI?
Audio AI refers to deep neural networks that process, analyze, and predict audio signals. The technology is witnessing significant adoption in various industries like media, healthcare, security, and smart devices.
It enables organizations to build tools like virtual assistants with advanced functionalities such as automated transcription, translation, and audio enhancement to optimize human interactions with sound.
Capabilities of Audio AI
Audio AI is still evolving, with different AI algorithms and frameworks emerging to allow users to produce high-quality audio content. The list below highlights audio AI’s most powerful capabilities, which are valuable in diverse use cases.
- Text-to-Speech (TTS): TTS technology converts written text into lifelike speech. Modern TTS systems use neural networks to produce highly natural and expressive voices, enabling applications in virtual assistants, audiobooks, and accessibility tools for individuals with visual impairments.
- Voice Cloning: Voice cloning replicates a person’s voice with minimal training data. AI models can create AI voices that closely mimic the original speaker by analyzing speech patterns and vocal characteristics. The method is valuable in personalized customer experiences, voiceover work, and preserving voices for historical or sentimental purposes.
- Voice Generation: AI-driven synthesis generates new voices, often used for creative projects or branding. Experts can tailor these AI-generated voices for tone, emotion, and style, opening opportunities in marketing, gaming, and virtual character creation.
- Voice Dubbing: Audio AI facilitates seamless dubbing by synchronizing translated speech with original audio while maintaining the speaker's tone and expression. The approach enhances the accessibility of movies, TV shows, and educational content across languages.
- Audio Editing and Generation: AI-powered tools simplify audio editing by automating background noise reduction, equalization, and sound enhancement. Generative deep-learning models create music and sound effects. They serve as versatile tools for content creators and musicians, helping them produce unique and immersive auditory experiences to captivate audiences.
- Speech-to-text transcription: Audio AI converts spoken language into accurate written text. The ability helps automate tasks like transcribing meeting minutes, generating video subtitles, and assigning real-time captions.
- Voice Assistants and Chatbots: Users can leverage audio AI to develop intelligent voice assistants and chatbots to enable seamless, conversational interactions with end customers. These systems handle tasks like setting reminders, answering queries, and assisting with customer support.
- Emotion Recognition in Speech: Deep learning audio architectures can analyze vocal tone, pitch, and rhythm to detect emotions in speech. This technology is valuable in customer service to gauge satisfaction, mental health monitoring to assess well-being, and entertainment to create emotionally aware systems.
- Sound Event Detection: Experts can use audio AI to identify specific sounds, such as alarms, footsteps, or breaking glass, in real time. This capability is crucial for security systems, smart homes, and industrial monitoring.
- Music Recommendation: Intelligent audio systems can generate personalized music recommendations by analyzing listening habits, preferences, and contextual data.
Applications of Audio AI
Audio AI advancements are empowering businesses to leverage the technology across a wide range of applications. The following sections mention a few popular use cases where audio AI’s capabilities transform user experience.
Audio AI in the Film Industry
Audio AI in the film industry helps film-makers in the following ways:
- Dubbing: Audio AI makes the dubbing process more efficient and accurate, allowing natural lip-syncing and emotion-rich translations and making films accessible to global audiences.
- Animated movies: AI-generated voices can bring characters to life in animated films. They offer diverse vocal styles without requiring extensive audio recording sessions.
- Music: Audio AI assists in composing original scores. This helps improve background soundscapes and automates audio mixing for immersive experiences.
Audio AI for Content Generation
Audio AI streamlines content creation workflows across platforms by automating and enhancing audio production.
Below are a few examples:
- Podcasts: Audio AI helps reduce background noise, balance audio levels, and even generate intro music scores according to the creator’s specifications. Creators can also use AI to simulate live editing, making real-time adjustments during recording, such as muting background disruptions or improving voice clarity.
- YouTube and TikTok videos: AI-powered tools enable creators to effortlessly add voiceovers in deepfakes, captions, and sound effects. This can make content more engaging and professional for different target audiences.
- Audiobooks: Text-to-speech (TTS) technology delivers lifelike narrations, reducing production time while maintaining high-quality storytelling. AI can also adapt narrations for diverse listener needs, such as adjusting speaking speed or adding environmental sounds, for more personalization and inclusivity.
Audio AI in Healthcare
Healthcare professionals can improve patient care and documentation through audio AI automation. Some common use cases include:
- Patient engagement: AI-powered voice assistants can interact with patients to provide appointment reminders, medication alerts, and health education, ensuring better adherence to care plans.
- Managing Documentation: Audio AI automates documentation by transcribing doctor-patient conversations and generating accurate medical records in real-time. This approach reduces administrative burdens on healthcare providers and allows them to provide personalized care according to each patient’s needs.
Audio AI in the Automotive Industry
The automotive sector uses audio AI to make vehicles smarter and more user-friendly. A few innovative applications include:
- Auto diagnostics: Audio AI can analyze engine or mechanical sounds to detect anomalies. This helps identify potential issues early and reduces maintenance costs.
- In-car entertainment: With Audio AI, drivers can use voice to control a vehicle’s audio systems, personalizing music playlists, adjusting audio settings, and enhancing sound quality for an immersive experience.
- Smart home integration: Users can control their vehicles from home devices like Alexa or Google Home via voice commands. With a stable internet connection, they can start the engine, lock or unlock doors, check fuel levels, and set navigation destinations.
Audio AI in Education
Education offers numerous opportunities where Audio AI can enhance the learning experience for both students and teachers. The most impactful applications include:
- Lecture Transcription: Instead of manually taking notes, students can feed a teacher’s recorded lecture into an audio AI model to transcribe the recording into a written document.
- Automated Note-taking: AI-based audio applications can generate notes by listening to lectures in real-time. This allows the student to focus more on listening to the lecturer.
- Real-time Translation: Instructors can use AI-powered translation tools to break language barriers, making educational content accessible to a global audience.
- Audio and Video Summarization: Audio AI software allows students to condense lengthy materials into concise highlights, saving time and improving comprehension.
- Captioning of Virtual Classes: Students with hearing impairments or those in noisy environments can use audio AI to caption online lectures for better understanding.
How Audio AI Works
As mentioned earlier, Audio AI uses machine learning algorithms to analyze sounds. It understands sound datasets through waveforms and spectrograms to detect patterns.
A waveform represents sound as amplitude across time. The amplitude is the height of a wave indicating a specific sound’s loudness. Waveforms can consist of extensive data points containing amplitude values for each second. The dataset can range from 44,000 to 96,000 samples.
Spectrogram: Color Variations Represent Amplitudes
In contrast, a spectrogram is a much richer representation that includes a sound’s amplitude and frequency against time. Since each data point in a spectrogram contains more information than a point in a waveform, analyzing spectrograms requires fewer samples and computational power.
The choice of using spectrograms or waveforms as inputs to generative models depends on the desired output and raw audio complexity. Waveforms are often helpful when you need phase information to process multiple sounds simultaneously. Phases indicate the precise timing of a point in a wave.
Audio AI Model Architectures
Besides output and raw audio type, the model architecture is a crucial component of audio AI systems. Several architectures are available to help generate sounds and voices for the use cases discussed in the previous section.
The list below discusses the most popular frameworks implemented in modern audio AI tools.
Variational Autoencoders (VAEs)
VAEs are deep learning models comprising encoder and decoder modules. The encoder converts data samples into a latent distribution, while the decoder module samples from this distribution to generate the output.
Experts train VAEs by minimizing a reconstruction loss. They compare the decoder's generated output with the original input. The goal is to ensure the decoder accurately reconstructs an original sound sample by randomly sampling from the latent distribution.
Generative Adversarial Networks (GANs)
GANs consist of a generator and a discriminator component. The generator creates random noise, such as sound, voice, or music, and sends it to the discriminator. The discriminator tries to tell whether the generated noise is real or fake.
The training process involves the generator producing multiple samples and the discriminator trying to distinguish between real and fake samples. Training stops once the discriminator cannot categorize the generator’s output as fake.
Transformers
Transformers are one of the most revolutionary and sophisticated deep learning architectures. They use the well-known attention mechanism to generate or predict an output. The architecture powers most modern large language models (LLMs) we know today.
The attention mechanism works by understanding the relationships between different existing data points to predict or generate a new sample. It breaks down a soundwave or any other data type into smaller chunks or embeddings to detect relations. It uses this information to identify which part of data is most significant to generate a specific output.
Challenges of Audio AI
Although audio AI models’ predictive and generative power is constantly improving, developing them is challenging. The following section highlights the most common issues in building audio AI solutions.
Data Preparation
High-quality data is essential for training effective audio AI systems. However, preparing audio data means cleaning, labeling, and segmenting large datasets. This can be time-consuming and resource-intensive.
Variations in accents, noise levels, and audio quality further complicate data management. It requires robust preprocessing techniques to ensure models use diverse and representative data for optimal performance.
Data Privacy
Audio data often contains sensitive personal information, such as the voices of real individuals or confidential conversations. Ensuring data privacy is a significant challenge, as improper handling could lead to breaches or misuse.
Companies must comply with strict regulations, implement anonymization techniques, and adopt secure storage and processing methods to protect user data and build trust.
Accuracy and Bias
Audio AI systems can struggle with accuracy due to diverse accents, languages, or environmental noise. Additionally, biases in training data can lead to uneven performance across demographics, potentially creating disadvantages for certain groups.
Addressing these issues requires datasets from several groups to ensure fair, consistent, and relevant results across all user profiles.
Continuous Adaptation
Languages evolve and differ across generations, having various slang, acronyms, and conversational styles. Continuously adapting audio AI tools to match new user requirements is tricky, and failing to keep up can result in outdated or irrelevant outputs.
Continuous learning, model updates, and retraining are essential but demand significant resources, technical expertise, and robust infrastructure to maintain system relevance and effectiveness over time.
Multimodal Support and Integration
Applications like TTS, transcription, narrations, and translation require multimodal models that simultaneously understand different data modalities, such as text, speech, and images.
However, integrating audio AI with such modalities presents technical challenges. Seamless multimodal support requires sophisticated architectures capable of processing and aligning diverse data types.
Ensuring interoperability between systems while maintaining efficiency and accuracy adds complexity to implementation, especially for real-time systems like virtual assistants or multimedia tools.
Encord for Audio AI
Addressing all the above challenges can be overwhelming, requiring different approaches, expertise, and infrastructure. However, a business can take a more practical route using cost-effective audio annotation tools that streamline data management and model development workflows.
Encord’s audio annotation tool is a comprehensive multimodal AI data platform that enables the efficient management, curation and annotation of large-scale unstructured datasets including audio files, videos, images, text, documents and more. Encord supports a number of audio annotation use cases such as speech recognition, emotion detection, sound event detection and whole audio file classification. Teams can also undertake multimodal annotation such as analyzing and labeling text and images alongside audio files.
Key Features
- Flexible Classification: Allows for precise classification of multiple attributes within a single audio file down to the millisecond.
- Overlapping Annotations: Supports layered annotations, enabling the labeling of multiple sound events or speakers simultaneously.
- Collaboration Tools: Facilitates team collaboration with features like real-time progress tracking, change logs, and review workflows.
- Efficient Editing: Provides tools for revising annotations based on specific time ranges or classification types.
- AI-Assisted Annotation: Integrates AI-driven tools to assist with pre-labeling and quality control, improving the speed and accuracy of annotations.
Strength
The platform’s support for complex, multilayered annotations, real-time collaboration, and AI-driven annotation automation, along with its ability to handle various file types like WAV and an intuitive UI with precise timestamps, makes Encord a flexible, scalable solution for AI teams of all sizes preparing audio data for AI model development.
Audio AI: Key Takeaways
Audio AI technology holds great promise, with exciting opportunities to improve user experience and business profitability in different domains. However, implementing audio AI requires careful planning and robust tools to leverage its full potential.
Below are some key points to remember regarding audio AI.
- Audio AI Applications: Businesses can use audio AI to streamline film production, generate podcasts and videos, manage vehicles, improve patient engagement, and make education more inclusive.
- Audio AI Challenges: Audio AI’s most significant challenges include preparing data, maintaining security, ensuring accuracy and unbiased output, continuously adapting to change, and integrating with multimodal functionality.
- Encord for Audio AI: Encord’s versatile data curation features can help you quickly clean, preprocess, and label audio data to train high-performing and scalable AI models.
Better Data, Better AI
Enhance your AI with automated data curation, annotation, and validation.
Try it todayWritten by

Haziqa Sajid
- AI processes audio data through waves or spectrograms to detect voice tones, styles, and dialects. It uses the information to generate unique sounds and voices.
- Audio AI can transform text into speech, clone voices, edit audio, dub voices in other languages, and generate music.
- Yes, advanced AI models can generate lifelike voiceovers, replicating human tone, emotion, and inflection.
- AI Text-to-Speech (TTS) converts written text into natural-sounding speech using deep learning models.
- The most common challenges include data preparation, ensuring privacy, addressing bias, achieving accuracy, continuous adaptation, and integrating audio with multimodal systems.
Explore our products