Contents
Hire Slowly and Deliberately
Don’t Hire Jerks… Even if They’re Brilliant
Don’t Compromise
Have a Growth Mindset
Encord Blog
What I Learned Scaling a Start Up From 0 to 25 Employees
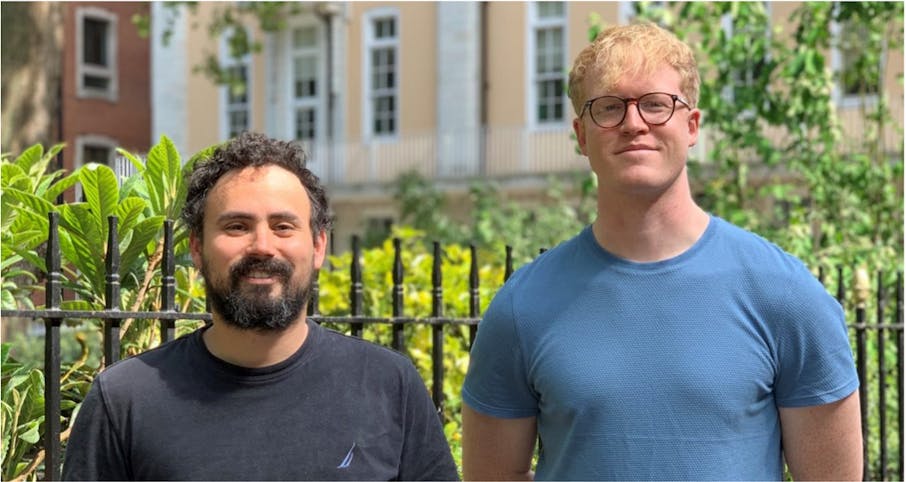
A lot changes when you transition from founding a startup to running a startup.
When starting Encord, my co-founder Ulrik and I spent nearly a year coding from our couches before we were accepted into Y Combinator. YC is an amazing experience, and it helped us focus on growing and scaling the business. However, the work environment still wasn’t very glamorous. We changed from coding on our couches in London to coding from my parent’s dining room in California. Our team expanded by one when Rio, my parents’ Akita, decided to start spending his days with us.
While Akitas are good for team morale, they don’t add that much value in terms of coding skill or strategic planning, so after three months at YC, we said goodbye to Rio and headed back to London where we began fundraising.
Those early days of running a startup can be stressful because you have to wear a lot of different hats at once. There’s a lot of dynamic problem solving that has to be done. However, when it’s just you and your co-founder, it’s always clear as to who is working on what problem, and the communications channels are very efficient. So, while there’s a lot of work to be done, it gets done in an efficient way. Even after a couple of hires, the work environment remains quite similar, but, as the business begins to scale and you hire more people, it starts to change.
Sourcing, interviewing, and hiring the right people for your team is challenging, and incorporating those new hires into the business also comes with its own unique set of growing pains.
Below are four things I’ve learned as we’ve scaled Encord from 0 to 25 people.
Hire Slowly and Deliberately
When I worked in finance, I interviewed a lot of people, but it’s very different when you are interviewing and hiring for your own company, especially if it’s at an early stage.
The success of the company is highly dependent on those first few hires. In a larger company with a hundred plus employees, a new hire won’t change the trajectory all that much. A hire only adds marginal incremental value whereas at a startup the early hires will have a tremendous impact on the course and future of the company.
We thought carefully about both the skills we needed most and the type of people we wanted to hire. We hired slowly and deliberately.
We began with the search for our founding software engineer. The decision to hire for this position first arose from our mutual agreement that we needed someone who was the best first complement to our skillset. Ulrik and I knew how to code, but we didn’t know how to design proper software systems. We needed someone who could take what we had built and properly architect it into a scalable system.
At Y Combinator, we learned that the first few people hired will be the ones in charge of hiring the next 50 people, so you shouldn’t underestimate the leverage effect of those early hires. This advice resonated with us and has already had a profound impact on our staffing at Encord.
So take your time. Don’t just hire indiscriminately. Make sure you get people who have the skills that you need and share your company’s values.
Don’t Hire Jerks… Even if They’re Brilliant
Netflix Co-Founder Reid Hastings once wrote that two factors largely shape the company culture: how the founders behave and how the team behaves on aggregate. When founding Encord, I realised that the culture of a startup does very much reflect the values of your first few hires and the way that these folks behave on a day-to-day basis.
As a founder, your job is to behave in a way that you think is fitting for both yourself and your organisation. If you consistently keep yourself to this standard, the hope is that it will flow through to your employees and a great culture will arise via the combination of implicit behavioural cues and explicit values.
However, as a founder, your job is also to hire a starting team that will likewise behave in a way that benefits the organisation.
In other words, don’t hire jerks even if they're brilliant.
We hired someone very early on who was incredibly talented, but they weren’t easy to work with and their attitude didn’t reflect the values we wanted expressed in our organisation. Ultimately, it didn’t work out.
Because early hires have such an influence on the trajectory of the company, a bad hire early on can have an outsized impact on the company culture. Newly hired enthusiasts should love their job, and should be passionate enough to go the extra mile at work when it's needed. Learning that lesson early on was a good way for us to prevent potentially toxic cultures from taking over as we continue to scale.
While it can be tough to get a full sense of a person's personality during the interviewing process, there are always warning signs.
Ask yourself: Was this person flexible when we were scheduling the interview? Was their communication around hiring and interviewing effective and congenial? Did they brag during the interview? Was there a whiff of ego throughout the whole process?
Remember, people tend to be on their best behaviour as candidates, so any attribute or characteristic you notice during hiring will likely be amplified once they start working for you. Don’t ignore warning signs, even if the person has a pristine academic background or performed brilliantly on your hiring task. If you notice off putting behaviour during the interview process, you will 100 percent notice it every day that you interact with them and so will the rest of your team. Suddenly, when faced with the day-to-day reality of working with that person, all those impressive degrees and experiences won’t seem so impressive.
Don’t Compromise
Expert negotiator Chris Voss has a simple story that explains what compromising – or “splitting the difference”– often looks like:
A woman wants her husband to wear black shoes to match his suit. Her husband wants to wear his brown shoes because they are more comfortable. So they compromise, and he leaves the house wearing one brown shoe and one black shoe.
Either outcome– a pair of brown shoes or a pair of black shoes– would have been better than the compromise.
What this story illustrates is that we often think of compromising as the “morally right” thing to do, but in reality compromising often leads to unsatisfying outcomes that aren’t beneficial for either person involved in the negotiation or the business itself.
It’s important to be collaborative with your co-founder (or your senior leadership if you don’t have a co-founder), but collaboration doesn’t necessarily mean doing an average of things.
Everyone brings different expertise, knowledge, and opinions, and, in most cases, it’s wise to defer to the person with the stronger expertise and opinion. With hiring, for instance, everyone picks up on different signals and looks for different attributes. If I feel strongly that we should hire a certain candidate, and my co-founder feels strongly that we shouldn’t, then we should talk through the issues, and ultimately, defer to the decision of the person who has more expertise and stronger feelings.
Decision making should be weighted dynamically, not equally. Ask yourself: who is the person most qualified to make this call and how strongly do they feel about it?
Have a Growth Mindset
Starting a business means constantly solving new problems, which is exciting. However, it also means that you’re always bad at your job because you’re always new to it. (And once you start accelling at your job, it changes, so you’re bad at it again). You’re constantly learning, and, if you think you know the answers, your business will likely remain stagnant.
As a result, it’s important to have a growth mindset.
At Encord, we’re learning, and we’re willing to make mistakes as long as we evaluate and update our beliefs and processes based on what we learned from those mistakes. We try to reflect and course correct in incremental ways to get better answers.
When it comes to hiring and scaling, having a growth mindset has been especially important. Scaling creates a certain tension between trying to implement standardised processes to ensure the business operates smoothly and trying to stay nimble enough to be innovative and responsive.
Shortly after our Series A, we hired a few people, and our organisational dynamic subtly began to change. There wasn’t a binary demarcation– the change didn’t occur at a certain amount of new hires– rather we felt it continuously, and then we realised that we needed to start formalising processes and procedures. It’s a challenge because we don’t want to be too process-driven like a large, inflexible corporation, but we also don’t want to be so ad hoc or ambiguous that our employees experience chaos trying to figure out what they’re doing and who they’re reporting to.
At the moment, we’re figuring out how to design and build processes that can help the startup run itself and provide our people with guidance without hamstringing them. Striking that balance is difficult. There’s a lot of best practices out there, but you won’t know which ones apply to your company and its culture until you try it out and see if it fits. That trial and error process requires a growth mindset at every level of the organisation.
Power your AI models with the right data
Automate your data curation, annotation and label validation workflows.
Get startedWritten by

Eric Landau
Explore our products