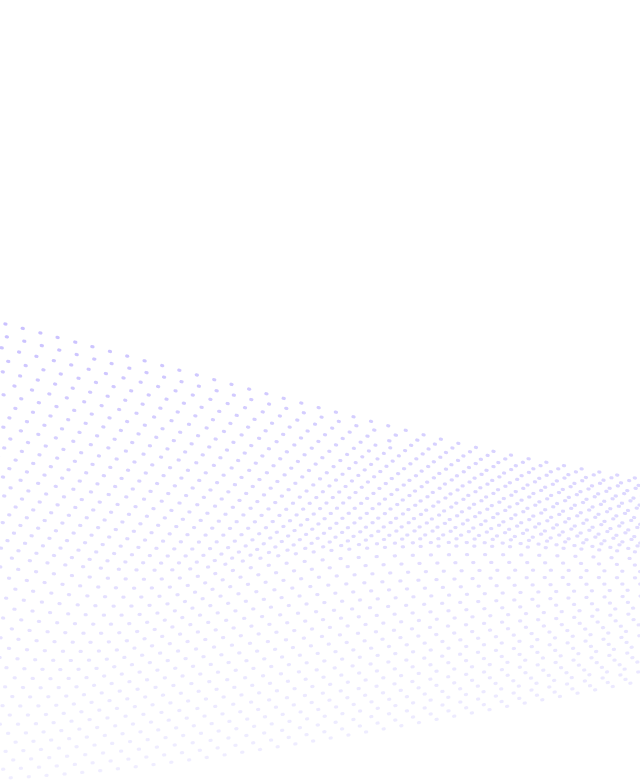
Encord Blog
Immerse yourself in vision
Trends, Tech, and beyond
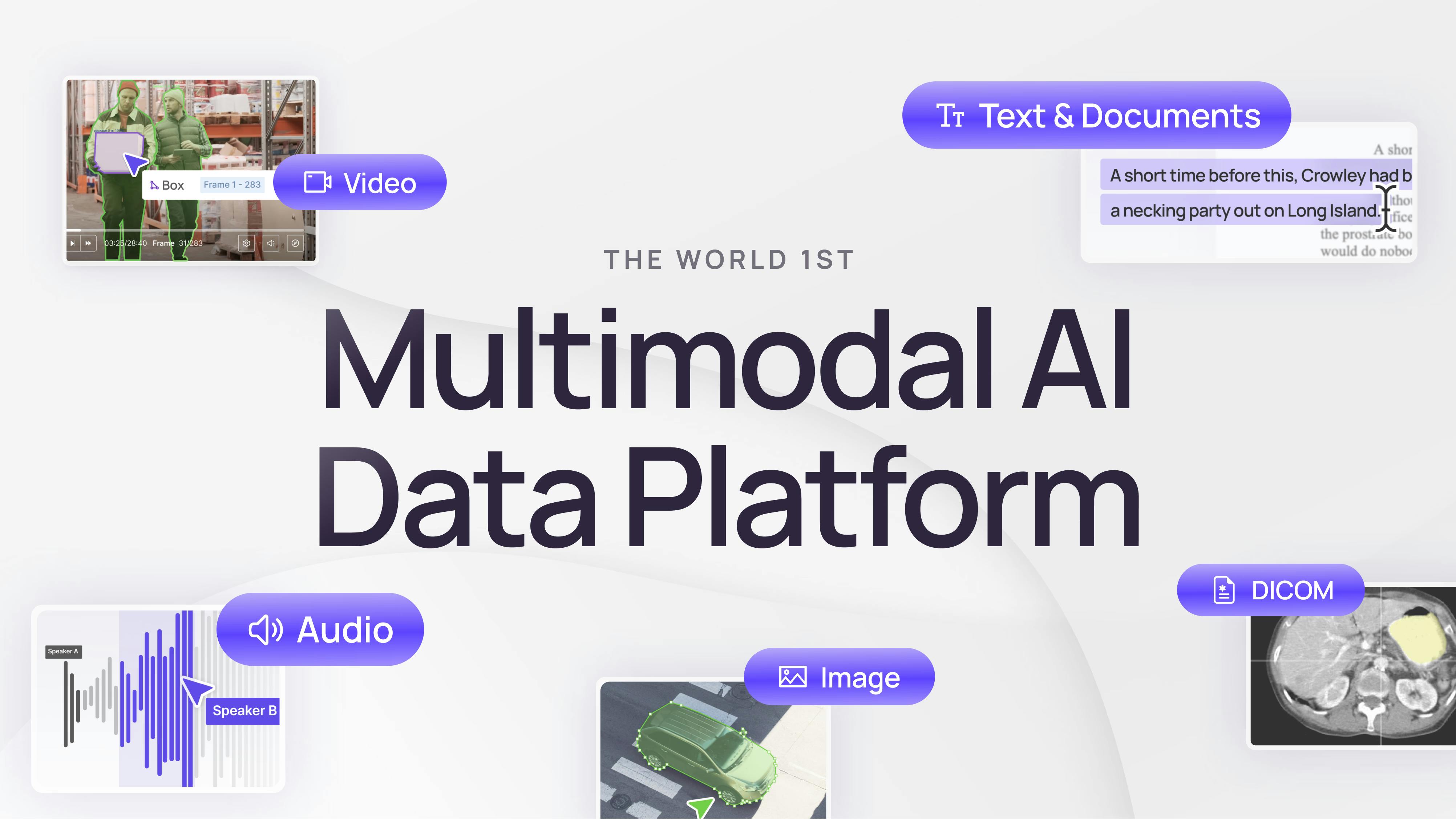
Encord is the world’s first fully multimodal AI data platform
Encord is the world’s first fully multimodal AI data platform Today we are expanding our established computer vision and medical data development platform to support document, text, and audio data management and curation, whilst continuing to push the boundaries of multimodal annotation with the release of the world's first multimodal data annotation editor. Encord’s core mission is to be the last AI data platform teams will need to efficiently prepare high-quality datasets for training and fine-tuning AI models at scale. With recently released robust platform support for document and audio data, as well as the multimodal annotation editor, we believe we are one step closer to achieving this goal for our customers. Key highlights: Introducing new platform capabilities to curate and annotate document and audio files alongside vision and medical data. Launching multimodal annotation, a fully customizable interface to analyze and annotate multiple images, videos, audio, text and DICOM files all in one view. Enabling RLHF flows and seamless data annotation to prepare high-quality data for training and fine-tuning extremely complex AI models such as Generative Video and Audio AI. Index, Encord’s streamlined data management and curation solution, enables teams to consolidate data development pipelines to one platform and gain crucial data visibility throughout model development lifecycles. {{light_callout_start}} 📌 Transform your multimodal data with Encord. Get a demo today. {{light_callout_end}} Multimodal Data Curation & Annotation AI teams everywhere currently use 8-10 separate tools to manage, curate, annotate and evaluate AI data for training and fine-tuning AI multimodal models. It is time-consuming and often impossible for teams to gain visibility into large scale datasets throughout model development due to a lack of integration and consistent interface to unify these siloed tools. As AI models become more complex, with more data modalities introduced into the project scope, the challenge of preparing high-quality training data becomes unfeasible. Teams waste countless hours and days in data wrangling tasks, using disconnected open source tools which do not adhere to enterprise-level data security standards and are incapable of handling the scale of data required for building production-grade AI. To facilitate a new realm of multimodal AI projects, Encord is expanding the existing computer vision and medical data management, curation and annotation platform to support two new data modalities: audio and documents, to become the world’s only multimodal AI data development platform. Offering native functionality for managing and labeling large complex multimodal datasets on one platform means that Encord is the last data platform that teams need to invest in to future-proof model development and experimentation in any direction. Launching Document And Text Data Curation & Annotation AI teams building LLMs to unlock productivity gains and business process automation find themselves spending hours annotating just a few blocks of content and text. Although text-heavy, the vast majority of proprietary business datasets are inherently multimodal; examples include images, videos, graphs and more within insurance case files, financial reports, legal materials, customer service queries, retail and e-commerce listings and internal knowledge systems. To effectively and efficiently prepare document datasets for any use case, teams need the ability to leverage multimodal context when orchestrating data curation and annotation workflows. With Encord, teams can centralize multiple fragmented multinomial data sources and annotate documents and text files alongside images, videos, DICOM files and audio files all in one interface. Uniting Data Science and Machine Learning Teams Unparalleled visibility into very large document datasets using embeddings based natural language search and metadata filters allows AI teams to explore and curate the right data to be labeled. Teams can then set up highly customized data annotation workflows to perform labeling on the curated datasets all on the same platform. This significantly speeds up data development workflows by reducing the time wasted in migrating data between multiple separate AI data management, curation and annotation tools to complete different siloed actions. Encord’s annotation tooling is built to effectively support any document and text annotation use case, including Named Entity Recognition, Sentiment Analysis, Text Classification, Translation, Summarization and more. Intuitive text highlighting, pagination navigation, customizable hotkeys and bounding boxes as well as free text labels are core annotation features designed to facilitate the most efficient and flexible labeling experience possible. Teams can also achieve multimodal annotation of more than one document, text file or any other data modality at the same time. PDF reports and text files can be viewed side by side for OCR based text extraction quality verification. {{light_callout_start}} 📌 Book a demo to get started with document annotation on Encord today {{light_callout_end}} Launching Audio Data Curation & Annotation Accurately annotated data forms the backbone of high-quality audio and multimodal AI models such as speech recognition systems, sound event classification and emotion detection as well as video and audio based GenAI models. We are excited to introduce Encord’s new audio data curation and annotation capability, specifically designed to enable effective annotation workflows for AI teams working with any type and size of audio dataset. Within the Encord annotation interface, teams can accurately classify multiple attributes within the same audio file with extreme precision down to the millisecond using customizable hotkeys or the intuitive user interface. Whether teams are building models for speech recognition, sound classification, or sentiment analysis, Encord provides a flexible, user-friendly platform to accommodate any audio and multimodal AI project regardless of complexity or size. Launching Multimodal Data Annotation Encord is the first AI data platform to support native multimodal data annotation. Using the customizable multimodal annotation interface, teams can now view, analyze and annotate multimodal files in one interface. This unlocks a variety of use cases which previously were only possible through cumbersome workarounds, including: Analyzing PDF reports alongside images, videos or DICOM files to improve the accuracy and efficiency of annotation workflows by empowering labelers with extreme context. Orchestrating RLHF workflows to compare and rank GenAI model outputs such as video, audio and text content. Annotate multiple videos or images showing different views of the same event. Customers would otherwise spend hours manually Customers with early access have already saved hours by eliminating the process of manually stitching video and image data together for same-scenario analysis. Instead, they now use Encord’s multimodal annotation interface to automatically achieve the correct layout required for multi-video or image annotation in one view. AI Data Platform: Consolidating Data Management, Curation and Annotation Workflows Over the past few years, we have been working with some of the world’s leading AI teams such as Synthesia, Philips, and Tractable to provide world-class infrastructure for data-centric AI development. In conversations with many of our customers, we discovered a common pattern: teams have petabytes of data scattered across multiple cloud and on-premise data storages, leading to poor data management and curation. Introducing Index: Our purpose-built data management and curation solution Index enables AI teams to unify large scale datasets across countless fragmented sources to securely manage and visualize billions of data files on one single platform. By simply connecting cloud or on prem data storages via our API or using our SDK, teams can instantly manage and visualize all of your data on Index. This view is dynamic, and includes any new data which organizations continue to accumulate following initial setup. Teams can leverage granular data exploration functionality within to discover, visualize and organize the full spectrum of real world data and range of edge cases: Embeddings plots to visualize and understand large scale datasets in seconds and curate the right data for downstream data workflows. Automatic error detection helps surface duplicates or corrupt files to automate data cleansing. Powerful natural language search capabilities empower data teams to automatically find the right data in seconds, eliminating the need to manually sort through folders of irrelevant data. Metadata filtering allows teams to find the data that they already know is going to be the most valuable addition to your datasets. As a result, our customers have achieved on average, a 35% reduction in dataset size by curating the best data, seeing upwards of 20% improvement in model performance, and saving hundreds of thousands of dollars in compute and human annotation costs. Encord: The Final Frontier of Data Development Encord is designed to enable teams to future-proof their data pipelines for growth in any direction - whether teams are advancing laterally from unimodal to multimodal model development, or looking for a secure platform to handle immense scale rapidly evolving and increasing datasets. Encord unites AI, data science and machine learning teams with a consolidated platform everywhere to search, curate and label unstructured data including images, videos, audio files, documents and DICOM files, into the high quality data needed to drive improved model performance and productionize AI models faster.
Nov 14 2024
m
Trending Articles
1
The Step-by-Step Guide to Getting Your AI Models Through FDA Approval
2
Introducing: Upgraded Project Analytics
3
18 Best Image Annotation Tools for Computer Vision [Updated 2025]
4
Top 8 Use Cases of Computer Vision in Manufacturing
5
YOLO Object Detection Explained: Evolution, Algorithm, and Applications
6
Active Learning in Machine Learning: Guide & Strategies [2025]
7
Training, Validation, Test Split for Machine Learning Datasets
Explore our...
What is Supply Chain Automation?
The global supply chain is more complex today than ever, with increasing demand for speed, accuracy, and efficiency. Businesses must move goods faster, while also reducing costs, minimizing errors and optimizing logistics. Traditional supply chain operations mainly rely on manual tasks and legacy systems and therefore, struggle to keep up with increasing demands. Supply chain automation uses artificial intelligence (AI), robotics and data-driven systems to streamline operations from warehouse management to delivery. As the adoption of automation grows, the companies face new challenges, particularly in handling unstructured data and optimizing AI models for real world applications. In this blog, we will explore supply chain automation, the data challenges companies face and how physical AI is rapidly transforming a number of industries to become more efficient, cost-effective, and accurate. Understanding Supply Chain Automation Supply chain automation refers to the use of AI and robotics to improve the efficiency in logistics, manufacturing, and distribution. By reducing manual intervention, businesses can improve speed, safety, accuracy and cost effectiveness. The automation can span across various stages like from real time inventory tracking to using robots to handle warehouse goods. How Supply Chain Automation Solution Works? The automation in the supply chain process generally involves: Robotic Process Automation (RPA): Using bots to handle repetitive tasks like data entry, order processing, and invoice management. Decision Making: Machine learning models analyze supply and demand patterns, and help businesses make better inventory and logistics decisions. Computer Vision & Robotics: Robots sort, pick, and pack goods in warehouses with precision, reducing human labor. IoT & Real-Time Tracking: Smart sensors track shipments, monitor warehouse conditions, and provide real-time updates on goods in transit. Autonomous Vehicles & Drones: Self-driving trucks and drones transport goods efficiently. It reduces dependency on human drivers. Key Benefits of Supply Chain Automation Increased Efficiency & Speed Automation technologies work 24/7 without fatigue. It ensures faster processing times for tasks like order fulfillment, inventory management, and warehouse operations. Efficient robotic systems also reduce manual errors, leading to smoother logistics operations. Workforce Optimization Labor costs in warehousing and logistics are high, and staffing shortages can disrupt operations. Automation reduces reliance on manual labor for repetitive and physically demanding tasks, allowing human workers to focus on higher-value activities such as supervising AI-driven systems or handling exceptions. Also, automation helps businesses ensure safety for the workforce. Improved Accuracy & Reduced Errors Human errors in inventory tracking, order fulfillment, and logistics management can cause costly delays and stock discrepancies. AI-powered automation ensures precise data entry, accurate order picking, and real-time tracking, reducing mistakes across the supply chain. Scalability & Flexibility Automated systems can scale up or down based on demand fluctuations. For example, during peak seasons like Black Friday or holiday sales, AI-driven fulfillment centers can process higher volumes of orders without requiring additional workforce hiring. Better Decision Making With AI-powered analytics, businesses can predict demand, optimize inventory levels, and streamline logistics. This data-driven approach helps companies make faster, smarter decisions, improving overall supply chain management. Why Supply Chain Automation is Critical Today? The global supply chain has faced many unexpected challenges in recent years like pandemic-related disruptions, labor shortages, increasing e-commerce demand, and rising logistics costs. Companies that fail to automate risk falling behind competitors that use the efficiency of automation. By implementing automation, businesses can future-proof their supply chains, ensuring agility, reliability, and scalability in an increasingly complex global market. Applications of Supply Chain Automation This is really transforming the industries by optimizing operations across warehousing, logistics, transportation, and fulfilment. Here are some of the key applications: Automated Logistics Warehouses nowadays are becoming fully automated environments where robotic systems handle tasks that require significant labor. This includes: Automated Picking & Sorting: Automated conveyor systems manage inventory movement, increasing the speed of fulfillment. Inventory Tracking: IoT sensors, RFID tags, and computer vision continuously track stock levels in real-time, reducing errors. Automated Storage & Retrieval Systems (AS/RS): These systems use robotic shuttles and cranes to optimize space utilization and ensure fast, efficient retrieval of items. Dynamic Order Processing: AI algorithms prioritize orders based on urgency, demand, and supply chain constraints. Example Massive fulfilment centers like Amazon use robotic arms to sort, pick and package millions of products daily. It reduces the need for manual labor and increases efficiency. Autonomous Freight and Delivery The transportation and logistics sector is integrating AI to improve efficiency, reduce delivery times, and minimize operational costs. This includes: Autonomous Vehicles & Drones: Self-driving trucks and delivery drones are being deployed for delivering products to customers, reducing dependence on human drivers. Route Optimization: Machine learning algorithms analyze traffic, weather, and delivery schedules to optimize routes. This helps in cutting fuel costs and improving on-time deliveries. Smart Freight Tracking: GPS and IoT sensors provide real-time shipment tracking, improving transparency and security in logistics. Example FedEx and UPS are testing autonomous delivery vehicles and AI route planning to speed up shipments and optimize delivery networks. Quality Control and Inspection Given the volume of the products handled by businesses, using AI models for quality control and inspection of the products at least the first line of inspection can be helpful. Defect Detection: Computer vision systems inspect goods in real-time, and identify defects or damages before they reach customers. Automated Sorting & Rejection: Robotics handle product sorting, and make sure defective items are removed from the supply chain before shipment. Predictive Maintenance for Equipment: AI systems monitor warehouse machinery and fleet vehicles, detecting potential failures before they occur. Example The Tesla factories use real time defect detection systems during the manufacturing and packaging process. Demand Forecasting Predictive analytics is helping businesses make better and data driven decisions by utilixing the huge amounts of supply chain data. Some of the applications are: Predicting Demand Spikes: Machine learning models analyze historical data, seasonal trends, and market conditions to optimize stock levels. Preventing Stock Shortages and Overstocking: Automated inventory systems adjust product procurement according to real-time visibility based on demand forecasts. Dynamic Pricing Adjustments: Data driven insights allow businesses to adjust pricing dynamically based on supply and demand fluctuations. Example Walmart uses forecasting models for inventory management across its global supply chain. It also analyses local demographics and purchasing patterns for cost savings associated with excess inventory, prevent stockouts and in general improve customer satisfaction. Warehouse Automation This makes the warehouse operations faster, safer and more efficient by automating one of the most physically demanding tasks in the supply chain businesses. Source Some of the applications are: Automated Unloading and Loading: Traditional trailer unloading is labor-intensive and slow. The robots automate the process, increasing speed while reducing physical strain on workers. Labor Optimization: By automating repetitive tasks, warehouse workers can shift to supervisory and higher-value roles, improving overall operational efficiency. Robotic Picking & Sorting: The robots can handle package sorting and placement with CV and ML models to minimize errors and maximize efficiency. Example Pickle Robot uses robotic arms to automate trailer unloading and package sorting. The robots are able to handle various package sizes with precision ensuring safety for workers and the products equally. Watch our full webinar with Pickle Robot: Data Challenges in Supply Chain Automation Supply chain automation relies heavily on AI, robotics, and real-time data processing to optimize operations. However, managing and utilizing supply chain data presents several challenges. From unstructured data inefficiencies to fragmented systems, these issues can slow down automation efforts and impact the decision making process. Unstructured Data Issues Supply chain data comes from various sources like video feeds, IoT sensors, GPS tracking, and robotic systems. Unlike structured databases, this data is unorganized, complex, and difficult to process using existing systems. But the AI models require structured, labeled datasets to function effectively, but supply chain environments generate raw, unstructured data that must be cleaned, annotated, and processed before use. Also, since the supply chain data sources vary so much, the data modalities also vary. Hence, a reliable data processing platform is essential which can handle different modalities. Example Surveillance cameras in warehouses capture footage of package movements, but extracting meaningful insights such as detecting misplaced items or predicting equipment failures requires advanced models trained on well annotated video data. Edge Cases & Variability Warehouses and logistics hubs are highly dynamic environments where AI systems must handle unexpected conditions, such as: Irregular package sizes and shapes that may not fit standard sorting models. Unstructured warehouse layouts where items are moved manually, making tracking difficult. Environmental factors like poor lighting, dust, or obstructions that can impact AI vision systems. Example A robotic arm needs to be trained to pick all different shapes and sizes of boxes. Otherwise the arms would pick uniformly shaped boxes and may struggle when faced with irregular or damaged packages, leading to errors and delays. Lack of High-Quality Labeled Data Training AI models for supply chain automation requires large volumes of accurately labeled data. A process that is both time-consuming and expensive. Data annotation for robotics and computer vision requires human expertise to label objects in warehouse environments e.g., differentiating between package types, identifying conveyor belt anomalies, or classifying damaged goods.Without high-quality annotated datasets, AI models struggle with real-world deployment due to poor generalization. Example A self-driving forklift needs detailed labeled data of warehouse pathways, obstacles, and human movement patterns to navigate safely—without this, its performance remains unreliable. Data Silos and Fragmentation Supply chain data is often stored in disconnected systems across different departments, vendors, and third-party logistics providers, making it difficult to get a unified view of operations. Example A warehouse may use one system for inventory tracking, another for shipment logistics, and a separate platform for robotic operations. Without integrating and connecting all of these systems, AI models cannot make real-time, data-driven decisions across the entire supply chain. Improving Data for Effective Supply Chain Automation High quality data helps build reliable AI models which is essential in supply chain automation. From unstructured data processing to better annotation workflows and system integration, improving data quality can significantly improve AI logistics. Structuring Unstructured Data The data in the supply chain pipeline comes from various sources and in large amounts. It is mainly unstructured and needs to be processed, annotated and in general converted into a usable format so that AI models can be trained on it. This will help the AI models to make accurate and automate the process. Comprehensive data platforms like Encord help organize, label and extract valuable insights from video or sensor data. Handling Edge Cases AI models must adapt to unexpected warehouse conditions such as damaged packages, irregular stacking, or poor lighting. During data curation for building automated supply chain models, it is essential to curate a diverse and well balanced dataset. Annotation tools allow the teams to label complex scenarios and also visualize the whole dataset and help curate a balanced training data. Efficient Data Annotation AI models for supply chain automation need large, high-quality labeled datasets, but manual annotation is slow and costly. AI-assisted annotation speeds up labeling while ensuring accuracy. Data platforms like Encord help identify, label, and visualize warehouse data, enabling teams to curate balanced training datasets for improved AI performance. Accurately label and curate physical AI data to supercharge robotics with Encord. Learn how Encord can transform your Physical AI data pipelines. Conclusion Supply chain automation is revolutionizing how businesses manage logistics, warehouses, and transportation. AI, robotics, and real-time data analytics are improving the customer experience. However, bottlenecks such as unstructured data, edge cases, and fragmented systems must be addressed to access the automation’s full potential. High-quality, structured data is essential for training reliable AI models. Advanced annotation tools and intelligent data management solutions streamline data labeling, improve model accuracy, and ensure seamless system integration. With the use of these data platforms like Encord, business processes can build smarter, more scalable automation tools for supply chains. As automation adoption continues to grow, companies that effectively manage their data and AI workflows will gain a competitive edge. Future-ready supply chains will not only optimize efficiency but also enhance resilience, adaptability, and overall decision-making. To learn how to overcome key data-related issues when developing physical AI and critical data management practices, download our Robotics e-book: The rise of intelligent machines.
Feb 14 2025
5 M
How Speech-to-Text AI Works: The Role of High Quality Data
Imagine a world where every spoken word is immediately recorded as clear, actionable text by your very own digital scribe that never gets tired. Imagine yourself in a lively meeting or in an inspiring lecture full of great ideas that come fast and every insight matters. With Speech-to-Text (STT) AI this dream is now reality. Speech-to-Text or Automatic Speech Recognition (ASR) uses artificial intelligence (AI) to convert spoken words into written text. It uses audio signal processing and machine learning (ML) algorithms to detect speech patterns in the audio and transform it into accurate transcriptions. How Speech-to-Text AI Works (By Author) Steps of Speech-to-Text AI Systems Following are the key components or steps of Speech-to-Text AI systems. Audio Processing In this step, the audio input is processed. The background noise is removed and normalization (i.e. adjustment of volume levels for consistency) is performed. Finally, the sampling (i.e. converting analog audio signals to digital signals) and segmentation is done to segment audio signals into smaller parts for processing. Feature Extraction In this step, the preprocessed audio is transformed into a set of features which represent the speech characteristics. There are some common techniques such as Mel-Frequency Cepstral Coefficients (MFCC), log-mel spectrograms, or filter banks which are used to extract audio features. These methods capture various details of the speech signal which helps the system to analyze and understand these speech signals. Acoustic Modeling This involves feeding the extracted features into an acoustic model (a deep neural network), which learns to map these features to primitive sound units (i.e. phonemes or sub-word units). NVIDIA has developed multiple models that utilize convolutional neural networks for acoustic models, including Jasper and QuartzNet. Language Modeling The system uses statistical methods (such as n-gram) or neural networks(such as Transformer based models like BERT) to understand the context and predicted word sequences. This helps in accurately converting phonetic sounds into meaningful words and sentences. Decoding Finally, the AI combines the output from acoustic and language models to produce the text transcription of the spoken words. How Speech-to-Text Works (Source) Applications of Speech-Text-AI When most people think of Speech-to-Text their minds go to having a chat with Siri or Alexa about the weather or to set an alarm reminder. For many of us, this was our first, or most salient, touchpoint with AI. Speech-to-Text has several applications across various domains. Some key applications of Speech-to-Text AI are discussed here. Virtual Assistants As mentioned above, a virtual assistant is one of the most popular applications of Speech-to-Text AI. It allows virtual assistants to interpret spoken language and respond appropriately, such as asking the time, weather, or to start a call It converts users voice commands into text that the backend systems process which as a result enable interactive, hands-free operation. Some examples of virtual assistants that you are likely familiar with are Amazon Alexa and Google Assistant. A user may ask, “What is the weather today?”. While this might seem like a simple query to those of us asking the question.The assistant converts the spoken query into text and processes the request by accessing weather data, and responds with the forecast. This integration of speech recognition enhances user convenience and accessibility. But the role of visual assistants does not stop here. They are also used in many applications such as home automation as shown in figure below. How Alexa Works for Home Automation (Source) The image above illustrates how speech-to-text AI enables home automation using Alexa. When a user gives a command, "Alexa, turn on the kitchen light," the Amazon Echo captures the speech and converts it into text. The text is processed by Alexa's Smart Home Skill API which identifies the intent through natural language processing. Alexa generates a directive which is sent to a smart home skill. The smart home skill then communicates with the device cloud. The device cloud relays the command to the smart device, such as turning on the kitchen light. Meeting and Conference Tools Have you ever been on a work-from-home call and accidentally lost focus? It happens to the best of us. In collaborative environments such as online meeting and conferencing tools, Speech-to-Text AI helps improve productivity by transcribing spoken words. Speech-to-Text AI enables accurate records, searchable archives, and real-time captioning for remote participants. For example, Microsoft Teams uses Speech-to-Text AI to generate live transcriptions during meetings. After the meeting, the transcript is saved and searchable in the chat history. This helps participants to focus on discussion without taking manual notes. MS Teams Microsoft Teams Transcription and Captioning (Source) Tools like notta.ai can help in real-time translations in meetings. You can automate the process of real-time translation for meetings with the help of this tool. It also helps in transcribing the meeting recordings into multiple languages. Live translation and transcription using notta.ai (Source) Customer Support Chatbots Customer support can be a never-ending stream of queries. Therefore, in customer support systems, Speech-to-Text AI is used to convert speech into text. Speech-to-Text AI works in intelligent chatbots and voice assistants to handle inquiries without human intervention in such a system. Many banks deploy customer service chatbots that accept voice commands, for example Customers can use these chatbots to acquire banking information using speech commands. Customer Support Assistant ICICI Bank UK (Source) Healthcare Applications Speech-to-Text AI is also used in healthcare applications. One of the most important uses is transcribing doctor-patient interactions to automate the documentation process for hands-free operation in sterile environments. An example application is Nuance Dragon Medical One. This cloud-based speech recognition solution helps physicians to document patient records. Doctors can dictate notes during or immediately after consultations which helps in reducing the administrative burden and allowing more time for patient care. Nuance Dragon Medical One (Source) Automated Transcription Services Automated transcription service is the process of converting spoken language (audio or video recordings) into written text using Speech-to-Text AI. These services are designed to create accurate, readable, and searchable text versions of spoken content. Automated transcription service is used for creating written records of interviews, lectures, podcasts, and more. It can be used for documentation, analysis, accessibility, or compliance purposes. For example, if you are using a long YouTube video for research, having it automatically transcribed will help distill the information into text rather than sitting and watching the entire video. The Otter.ai is an example of a transcription service for generating transcripts from meetings, lectures, or interviews. It allows users to upload recordings and provides transcription. Users can generate summaries and search through text to review meeting details and retrieve important information. Generating Transcription from Meeting using Otter AI (Source) Accessibility Tools There are applications available as accessibility tools which use Speech-to-Text AI to provide real-time captions and transcripts services. These accessibility tools help individuals with hearing impairments in conversation by translating and transcribing text in real time. For example, Live Transcribe is a real-time captioning app developed by Google for Android devices in collaboration with Gallaudet University. This application transcribes conversations in real time which helps deaf or hard-of-hearing users to follow conversations in a range of settings such as from classrooms to busy public spaces. Live Transcribe (Source) Language Learning Apps Many of us have taken a stab at learning a new language on Duolingo. Language learning platforms use Speech-to-Text to help learners improve their pronunciation, fluency, and comprehension. These apps analyze spoken input and offer feedback to help users correct their spoken words. For example, speaking exercises offered by Duolingo assists users to practice a new language by speaking into the app. The AI transcribes and analyzes their pronunciation and offers feedback and adjustments to help them improve their language skills. Duolingo’s Speaking Exercises (Source) Entertainment and Media Speech-to-Text AI is also very widely used in media production to create subtitles and generate searchable texts from audio or video. Speech-to-Text AI also enables interactive voice-controlled experiences in gaming and other entertainment sectors. Platforms like Netflix use speech recognition technology to automatically generate subtitles for movies and TV shows. Generating Subtitles in Netflix (Source) Challenges in Speech-to-Text AI The performance of speech-to-text AI systems depends upon the quality, richness, and accuracy of the training data. Therefore, failure may occur when these models are trained on inaccurate or low-quality data. Following are some key challenges: Limited or Unrepresentative Data Many speech recognition systems are trained on speech data with standard or common accents. If the training data does not include variety in speech data such as regional accents, dialects, or non-native speech patterns, the system may fail to understand speakers who do not have common ascent. This type of training data can cause errors in the system. It may also be possible that there is limited speech data in some languages for which there are fewer speakers or limited online data available. When a model is trained on this kind of little data for these languages, its performance will be lower in those languages than the languages with more data. Data Quality and Annotation Training data for speech recognition systems often contains "non-verbatim" transcriptions where the transcriber may skip certain words or correct mispronunciations. It means that sometimes the transcriber may change what was actually said. For example, excluding the words like "um" or "uh," to fix mistakes in how someone spoke, or rephrase sentences to make it sound better. This means that the written text does not match spoken words in the audio. When the system is trained on this kind of data, it gets confused because it learns from mismatched examples. These small errors can cause the system to make mistakes in understanding real speech. Training data is also recorded in quiet and controlled environments where there is no noise. It may also be possible that training data has a lot of background noise and is not cleaned or annotated properly. Models trained without enough examples of noisy or echo-filled environments often struggle when used in real situations. Domain and Context Mismatch In fields like medicine or law, the language that is used contains very technical and specific terms. If the training data does not have enough examples of the use of these specialized and technical terms, the trained model may struggle to understand or accurately transcribe them. To fix this, it is important to collect examples of training data which have these specialized word lists used in the field. Data Quantity and Imbalance Speech-to-Text AI systems need a lot of data for training so that it can learn how people speak. Systems trained on less data do not perform well and are not able to understand a variety of voices. If the training data include only specific types of voice (like male voices, or voices of specific age groups, or particular languages), the system will become biased toward those examples. This means that the system will not work well for voices or languages that are less represented in the data. Data Augmentation and Synthetic Data When there is not enough training data, the data augmentation techniques (like adding background noise or changing speech speed etc.) are applied or synthetic data is generated to increase training samples. While these techniques help, they fail to capture the complexity of real-world sounds. Relying too much on these techniques can make the system perform well on test data (because the test data may also contain these artificial samples) but the system may not perform in real world situations. Role of High Quality Data The foundation of any great Speech-to-Text AI system lies in the quality of data. The quality of data used during training decides the performance (I.e. accuracy, robustness, and generalization) of a Speech-to-Text AI model. Here is why high-quality data is essential. Improving Model Accuracy Clear, high-quality audio helps the model focus on the speech instead of background noise. This makes the model understand the words and translate it accurately into text. High-quality data does not mean quality of audio sample but how accurate the transcriptions are. It means that the transcribed text exactly matches what is spoken in the audio. Accurate annotations improve the accuracy of the model. Enhancing Model Robustness and Generalization To make a Speech-to-Text AI system work well in real-world situations, it is important that the training data must include a wide variety of accents, dialects, speaking styles, and sound environments. High-quality data makes sure that the trained model works well in all types of speakers or settings. The training data must also contain domain specific vocabulary and speech patterns to train Speech-to-Text AI in that field. This kind of data enhances models' robustness for all kinds of speech environments and models can generalize well. Efficient and Stable Model Training The model performs better when it is trained on clean and well-organized data. High-quality data reduces the chances of overfitting. Augmentation techniques like adding artificial noise or changing speech speed can help, but these steps are not required if the original data is already high-quality. This makes training simple and results in better performance by the trained model in real-world situations. Impact on Decoding and Language Modeling High-quality data helps the system to understand the relationship between sounds and words. This means it can make more accurate predictions about the spoken words. When these predictions are used during decoding, the final transcript is more accurate. High-quality data allows the AI system to understand the context of spoken words. This helps the model to handle the situations where there are words that sound the same but they have different meanings (e.g., "to," "too," and "two"). The high quality data makes the model make sense of such situations. High-quality data is very important for building a speech-to-text AI system. It improves accuracy, makes training faster and more reliable, and helps the system work well for different speakers, accents, and settings. How Encord Helps in Data Annotation Encord is a powerful data‐annotation platform that helps in preparing high-quality training data for training Speech-to-Text AI models. Following are key features how Encord helps annotate audio data for Speech-to-Text AI applications: Flexible, Precise Audio Annotation Encord’s Audio annotation tool allows users to label audio data with high accuracy. For example,annotators can accurately mark the start and end of spoken words or phrases. This precise timestamping is essential to produce reliable transcriptions and to train models that are sensitive to temporal nuances in speech. Support for Complex Audio Workflows Speech data often contains overlapping speakers, background noise, or varying speech patterns, making it a complex modality to train models on Encord addresses this complexity with these features: Overlapping Annotations: It allows multiple speakers or concurrent sounds to be annotated within the same audio file. This is useful for diarization (identifying who is speaking when) and for training models to differentiate speech from background sounds. Layered Annotation: In Layered annotations, annotators can add several layers of metadata to a single audio segment (e.g. speaker identity, emotion, or acoustic events). This layered annotation helps in preparing high quality data to improve model performance. AI-Assisted Annotation and Pre-labeling Encord supports SOTA AI models like OpenAI’s Whisper and Google’s AudioLM in its workflow to accelerate the annotation process.These supported SOTA models can automatically generate draft transcriptions or pre-label parts of the audio data. Annotators then review and correct these labels which reduces the manual effort required for annotating large data. Collaborative and Scalable Platform Encord offers a collaborative environment where multiple annotators and reviewers can work on the same project simultaneously in large-scale speech-to-text projects. The platform includes: Real-Time Progress Tracking: This feature enables teams to monitor annotation quality and consistency. Quality Control Tools: This feature allows built-in review and validation to make sure that annotations meet the required standards. Data Management and Integration Encord supports various audio file formats (e.g., WAV, MP3, FLAC) and easy integration with several cloud storage solutions (like AWS, GCP, or Azure). This flexibility means that large speech datasets can be stored, organized, and annotated efficiently. Take an example of a contact center application that uses Speech-to-Text AI for understanding customer queries and provides responses. The process for building application is illustrated in the diagram below. In this process, raw audio recordings from a contact center are first converted into text using existing speech-to-text AI models. The resulting text is then curated and enhanced to remove errors and improve clarity. Encord plays an important role by helping annotators annotate this curated data with metadata such as sentiment, call topics, and outcomes, and by verifying the accuracy of these annotations. This high-quality annotated data is used to train and fine-tune the Speech-to-Text AI model for contact center. The deployed system is continuously monitored and feedback is received to further refine the data preparation process. This whole process ensures that the Speech-to-Text AI operates with improved performance and reliability. An Example of Contact Center Application Key Takeaways: Speech-to-Text AI Annotating data for Speech-to-Text AI projects can be challenging. There are several issues like varied accents, background noise, and inconsistent audio quality which make it difficult to annotate such data. With the help of right tools, like Encord, and proper strategy the data annotations can be effectively done. Following are some key takeaways from this blog: Speech-to-Text AI transforms spoken language into text through a series of steps such as audio processing, feature extraction, acoustic and language modeling, and decoding. Various applications such as virtual assistants, meeting transcription tools, customer support chatbots, healthcare documentation, accessibility tools, language learning apps, and media subtitle generation uses Speech-to-Text AI. To build an effective Speech-to-Text AI system, high-quality training data is must. Issues like limited accent diversity, imperfect annotations, and domain-specific jargon can significantly reduce system performance. High-Quality audio data not only improves model accuracy and also enhances robustness and generalization. It also ensures that the trained Speech-to-Text AI system gives reliable performance across various speakers, accents, and real-world conditions. Advanced audio annotation tools like Encord streamline the data preparation process with precise, collaborative audio annotation and AI-assisted pre-labeling. Such tools ensure that Speech-to-Text models are trained on high-quality, well-organized datasets. If you're extracting images and text from PDFs to build a dataset for your multimodal AI model, be sure to explore Encord's Document Annotation Tool—to train and fine-tune high-performing NLP Models and LLMs.
Feb 13 2025
5 M
Data Collection: A Complete Guide to Gathering High-Quality Data for AI Training
Organizations today recognize data as one of their most valuable assets, making data collection a strategic priority. As generative AI (GenAI) adoption grows, the need for accurate and reliable data becomes even more critical for decision-making. With 72% of global organizations using GenAI tools to enhance their decisions, the demand for robust data collection pipelines will continue to rise. However, accessing quality data is challenging because of its high complexity and volume. In addition, low quality data, consisting of inaccuracies and irrelevant information, can cause 85% of your AI projects to fail, leading to significant losses. These losses may increase for organizations that rely heavily on data to build artificial intelligence (AI) and machine learning (ML) applications. Improving the data collection process is one way to optimize the ML model development lifecycle. In this post, we will discuss data collection and its impact on AI model development, its process, best practices, challenges, and how Encord can help you streamline your data collection pipeline. Data Collection Essentials Data collection is the foundation of any data-driven process. It ensures that organizations gather accurate and relevant datasets for building AI algorithms. Effective data collection strategies are crucial for maintaining training data quality and reliability, particularly as more and more businesses rely on AI and analytics. Experts typically classify data as structured and unstructured. Structured data includes organized formats like databases and spreadsheets, while unstructured data consists of images, audio, video, and text. Semi-structured data, such as JSON and XML files, falls between these categories. Modern machine learning models involving computer vision (CV) and natural language processing (NLP) typically use unstructured data. Organizations can collect such data from various sources, including APIs, sensors, and user-generated content. Surveys, social media, and web scraping also provide valuable data for analysis. A typical data lifecycle Gathering data is the first stage in the data lifecycle, followed by storage, processing, analysis, and visualization. This highlights the importance of data collection to ensure downstream processes, such as machine learning and business intelligence, generate meaningful insights. Poor data collection can affect the entire lifecycle, leading to inaccurate models and flawed decisions. Establishing strong quality control practices is necessary to prevent future setbacks. Why Is High-Quality Data Collection Important? Being the first step in the ML development process, optimizing data collection can increase AI reliability and boost the quality of your AI applications. Enhanced data collection: Reduces Bias: Bias in AI data can lead to unfair or inaccurate model predictions. For instance, an AI-based credit rating app may always give a higher credit score to a specific ethnic group. Organizations can minimize biases and improve fairness by ensuring diversity and representation during data collection. Careful data curation helps prevent skewed results that could reinforce stereotypes, ensuring ethical AI applications and trustworthy decision-making. Helps in Feature Extraction: Feature extraction relies on raw data to identify relevant patterns and meaningful attributes. Clean and well-structured data enables more effective feature engineering and allows for better model interpretability. Poor data collection leads to irrelevant or noisy features, making it harder for models to generalize to real-world use cases. Improves Compliance: Regulatory frameworks require organizations to collect and handle large datasets responsibly. An optimized collection process ensures compliance by maintaining data privacy, accuracy, and transparency right from the beginning. It builds customer trust and supports ethical AI development to prevent costly fines and reputational damage. Determines Model Performance: High-quality data directly impacts the performance of AI systems. Clean, accurate, and well-labeled data helps improve model training, resulting in better predictions and insights. Poor data quality, including missing values or outliers, can degrade model accuracy and lead to unreliable outcomes and loss of trust in the AI application. How AI Uses Collected Data? Let’s discuss how machine learning algorithms use collected data to gain deeper insights into the data requirements for effective ML model development. A simple learning process of a neural network Annotated Data Goes as Input AI models rely on annotated data as input to learn patterns and make accurate predictions. Labeled datasets help supervised learning algorithms map inputs to outputs, improving classification and regression tasks. High-quality annotations enhance model performance, while poor labeling can lead to errors and reduce AI reliability. Parameter Initialization Before training begins, deep learning AI models initialize parameters such as weights and biases, often using random values or pre-trained weights. Proper initialization prevents issues like vanishing or exploding gradients, ensuring stable learning. The quality and distribution of collected data influence initialization strategies, affecting how efficiently the model learns. Forward Pass During the forward pass, the AI model processes input data layer by layer, applying mathematical operations to generate predictions. Each neuron in the network transforms the data using learned weights and activation functions. The quality of input data impacts how well the model extracts features and identifies meaningful patterns. Prediction Error Using a loss function, the model compares its predicted output with actual labels to calculate prediction error. This error quantifies how far the predictions deviate from the ground truth. High-quality training datasets reduce noise and inconsistencies. They ensure the model learns meaningful relationships rather than memorizing errors or irrelevant patterns. Backpropagation Backpropagation calculates gradients by propagating prediction errors backward through the network. It determines how much each parameter contributed to the error, allowing the model to adjust accordingly. Clean, well-structured data ensures stable gradient calculations, while noisy or biased data can lead to poor weight updates and slow convergence. Parameter Updates The model updates its parameters using optimization algorithms like stochastic gradient descent (SGD) or Adam. These updates refine the weights and biases to minimize prediction errors. High-quality data ensures smooth and meaningful updates, while poor data can introduce inconsistencies, making the learning process time-consuming and unstable. Validation After training, data scientists evaluate the model on a validation dataset to assess its performance on unseen data. This step helps fine-tune hyperparameters and detect overfitting. A well-curated validation set ensures a realistic assessment. In contrast, poor validation data can mislead model tuning, leading to suboptimal generalization. Testing The final testing phase evaluates the trained model on a separate test dataset to measure its real-world performance. High-quality test data, representative of actual use cases, ensures accurate performance metrics. Incomplete, biased, or low-quality test data can provide misleading results, affecting deployment decisions and trust in AI predictions. Steps in the Data Collection Process Data collection is the backbone of the entire process, from providing AI models with annotated data to conducting final model testing. Organizations must carefully design their data collection strategies to achieve optimal results. While the exact approach may vary by use case, the steps below offer a general guideline. 1. Define Objectives Clearly defining objectives is the first step in data collection. Organizations must outline specific goals, such as improving model accuracy, understanding customer behavior, or optimizing operations. Well-defined objectives ensure data collection efforts are relevant and align with business needs. 2. Identify Data Sources Identifying reliable data sources is crucial for collecting relevant data. Organizations should determine whether data science teams will collect data from internal systems, external databases, APIs, sensors, or user-generated content. Correctly identifying sources minimizes the risk of collecting biased data, which can skew results. 3. Choose Collection Methods Selecting the proper data collection methods depends on the type of data, objectives, and sources. Standard methods include surveys, interviews, web scraping, and sensors for real-time data. The choice of method affects data accuracy, completeness, and efficiency. Combining methods often yields more comprehensive and reliable datasets. 4. Data Preprocessing Data preprocessing includes cleaning and transforming raw data into a usable format. This step includes handling missing values, removing duplicates, standardizing units, and dealing with outliers. Proper preprocessing ensures the data is consistent, accurate, and suitable for analysis. It improves model performance and reduces the risk of inaccurate results. 5. Data Annotation Data annotation labels raw data to provide context for AI models. This step is essential for supervised learning, where models require labeled examples to learn. Accurate annotations are crucial for training reliable models, as mistakes or inconsistencies in labeling can reduce model performance and lead to faulty predictions. 6. Data Storage Storing collected data securely and efficiently is essential for accessibility and long-term analysis. Organizations should choose appropriate storage solutions like databases, cloud storage, or data warehouses. Effective data storage practices ensure that large amounts of data are readily available for analysis and help maintain security, privacy, and regulatory compliance. 7. Metadata Documentation Metadata documentation describes the collected data's context, structure, and attributes. It provides essential information about data sources, collection methods, and formats. Proper documentation ensures data traceability and helps teams understand its usage. Clear metadata makes it easier to manage, share, and ensure the quality of datasets over time. 8. Continuous Monitoring Quality assurance requires continuous monitoring, which includes regularly tracking the accuracy and relevance of collected data. Organizations should set up automated systems to identify anomalies, inconsistencies, or outdated information. Monitoring ensures that data remains accurate, up-to-date, and aligned with objectives. It provides consistent input for models and prevents errors arising from outdated data. Learn how to master data cleaning and preprocessing Best Practices for High-Quality Data Collection The steps outlined above provide a foundation for building a solid data pipeline. However, you can further enhance data management by adopting the best practices below. Data Diversity: Ensure the collected data is diverse and representative of all relevant variables, groups, or conditions. Diverse data helps reduce biases and leads to fairer predictions across different demographic segments or scenarios. Ethical Considerations: Follow ethical guidelines to protect privacy, obtain consent, and ensure fairness in data collection. You must be transparent about data usage, avoid discrimination, and safeguard sensitive information. The practice will help maintain trust and compliance with data protection regulations. Scalability: Design your data collection process with scalability in mind. As data needs grow, your system should be able to handle increased volumes, sources, and complexity without compromising quality. Collaboration: Foster collaboration across teams, including data scientists, engineers, and domain experts, to align data collection efforts with business objectives. Cross-functional communication addresses all perspectives and helps teams focus on the most valuable insight. Automation: Automate repetitive tasks within the data collection process to increase efficiency and reduce errors. Automated tools can handle data gathering, preprocessing, and annotation. It allows teams to focus on higher-value tasks instead of spending time on tedious procedures. Data Augmentation: Use data augmentation techniques to enhance existing datasets, especially when data is scarce. Generating new data variations through methods like rotation, flipping, or adding noise can improve model robustness and create more balanced datasets. Data Versioning: Implement data versioning to track changes and updates to datasets over time. Version control ensures reproducibility and helps prevent errors due to inconsistent data. It also facilitates collaboration and provides a clear record of data modifications. Learn more about data versioning Data Collection Challenges Despite the abovementioned best practices, some challenges still remain. The most common issues relate to: Data Accessibility: Organizations often struggle with accessing the right data, especially when it is spread across multiple sources or stored in incompatible formats. The issue worsens for highly technical domains such as legal and scientific research, where finding relevant data may be challenging. Data Privacy: Collecting and using personal or sensitive data raises privacy concerns. Organizations must ensure compliance with data protection regulations to safeguard individuals' privacy. This is especially true for domains like healthcare, where even the slightest data breach can have severe consequences. Data Bias: Bias in data occurs when collected information misrepresents certain groups. Despite being careful, organizations can inadvertently introduce bias during collection, annotation, or sampling. Addressing bias is essential to developing equitable AI models and ensuring that predictions do not reinforce discriminatory practices. Resource Constraints: Data collection often demands significant time, expertise, and financial resources, especially with large or complex datasets. Organizations may face budgetary or staffing limitations, hindering their ability to gather data effectively. Encord for Data Collection You can mitigate the challenges mentioned earlier using specialized tools for handling complex AI datasets. Encord is one such solution that can help you curate extensive data. Encord is an end-to-end AI-based multimodal data curation platform that offers robust data curation, labeling, and validation features. It can help you detect and resolve inconsistencies in your collected data to increase model training efficiency. Encord Key Features Curate Large Datasets: Encord helps you develop, curate, and explore extensive multimodal datasets through metadata-based granular filtering and natural language search features. It can help you explore multiple types, including images, audio, text, and video, and organize them according to their contents. Data Security: The platform adheres to globally recognized regulatory frameworks, such as the General Data Protection Regulation (GDPR), System and Organization Controls 2 (SOC 2 Type 1), AICPA SOC, and Health Insurance Portability and Accountability Act (HIPAA) standards. It also ensures data privacy using robust encryption protocols. Addressing Data Bias: With Encord Active, you can assess data quality using comprehensive performance metrics. The platform’s Python SDK can also help build custom monitoring pipelines and integrate them with Active to get alerts and adjust datasets according to changing environments. Scalability: Encord can help you overcome resource constraints by ingesting extensive multimodal datasets. For instance, the platform allows you to upload up to 10,000 data units simultaneously as a single dataset. You can create multiple datasets to manage larger projects and upload up to 200,000 frames per video at a time. Get in-depth data management, visualization, search and granular curation with Encord Index. Data Collection: Key Takeaways With AI becoming a critical component in data-driven decisions, the need for quality data collection will increase to ensure smooth and accurate workflows. Below are a few key points to remember regarding data collection. High-quality Data Collection Benefits: Effective data collection improves model performance, reduces bias, helps extract relevant features, and boosts regulatory compliance. Data Collection Challenges: Access to relevant data, bias in large datasets, privacy concerns, and resource constraints are the biggest hindrances to robust data collection. Encord for Data Collection and Curation: Encord’s AI-based data curation features can help you remove the inconsistencies and biases present in complex datasets.
Feb 13 2025
5 M
Recap: AI After Hours - Physical AI (Special Edition)
On January 25, Encord wrapped up our second AI After Hours at the GitHub HQ for a special edition on Physical AI. The 6x over the subscribed evening was the place where AI leaders could hear from disruptors in the Physical AI space. Here’s a quick recap of what you missed and your opportunity to watch the sessions on demand. Don’t want to miss out on a future AI After Hours? Look for future happenings here. The Scene Up until this year, the latest craze in AI has largely focused on understanding and generating digital data like text, images, and video. We have seen advancements in areas like chatbots, image generation, and language understanding. But in 2025, that changed. CES pushed Physical AI into the limelight. The reality is that the real world is more than pixels and words. It has motion, sound, temperature, force, and more, and it is a rather complex, dynamic system that traditional AI struggles to understand. This is where Physical AI comes in. Instead of relying entirely on vision-based LLMs, physical AI uses sensor data to analyze, predict, and interact with the physical human world. It powers applications beyond traditional robotics in fields like manufacturing, healthcare, and industrial automation. So what’s the TL’DR on the key advancements in Physical AI that were discussed at this special edition of AI After Hours? The first talk, given by Rish Gupta & Dunchadhn Lyons from Spot AI, explored AI-powered video processing, demonstrating how cameras can transition from passive surveillance tools to active automation agents. In the second talk, Kevin Chavez from Dexterity explored the use of physical AI in warehouse operations by combining perception, control, and actuation. The final talk, by Ivan Poupyrev, Dr., CEO & Co-founder of Archetype AI, an Encord customer, focused on their Physical AI Model, which uses sensor data to build an understanding of physical systems beyond robotics. Are you curious to learn more? Here’s a summary of these talks and the playbacks Transforming Security Cameras into Automated AI Agents Traditional security cameras are passive recording tools that require human monitoring to extract insights. Spot AI is changing this by converting cameras into automation tools for industries like healthcare, retail, logistics, and manufacturing. Their platform helps businesses analyze, search for, and automate actions based on real-time video insights. Key Highlights Unified Camera Infrastructure: Spot AI connects various camera brands to a centralized, on-premise server for seamless integration. Massive Video Indexing: Processes 3 billion minutes of fresh video per month, surpassing YouTube’s monthly ingestion. AI-Driven Automation: Combines rule-based AI with LLMs to minimize human intervention in safety and security tasks. Zero-Shot Text Prompting: Enables AI models to identify new objects or behaviors without additional training. Hybrid AI with Gemini: Uses LLMs for contextual understanding, reducing false positives in forklift safety monitoring. Use Cases and AI Agent Development Security & Retail: Detecting unattended vehicles in drive-thrus, preventing unauthorized entry (tailgating detection). Manufacturing & Warehouses: Forklift monitoring, enforcing safety compliance (detecting missing hard hats or vests). Healthcare: Identifying patients vs. staff in restricted areas using a lightweight classifier trained on semantic embeddings. Physical AI for Warehouse Automation Dexterity is building a robotics platform to automate complex warehouse tasks. It integrates multimodal sensing and advanced motion planning to optimize logistics efficiency. Their hardware-software ecosystem enables dexterous manipulation and long-horizon reasoning for real-world applications like truck loading and order fulfillment. Key Highlights Dexterity’s Robotics Stack: A three-layered platform combining hardware (multi-arm mobile robots), DexAI software (bundles of robotic capabilities as skills), and task-specific applications. Multimodal Physical AI: Uses force, torque, vision, and proprioceptive data for real-time perception, planning, and control. Hybrid AI Model: Combines transformer-based trajectory prediction with real-time force control for precise manipulation. Industry Deployments: Actively working with FedEx and UPS to automate truck loading, reducing wasted space and improving packing stability. Use cases Truck Loading & Packing: AI-driven tight packing and 3D bin packing optimize space utilization and package stability. Order Fulfillment & Sorting: Intelligent robotic handling for depalletizing, order picking, and package routing. Dexterous Manipulation: Advanced motion planning enables squeezing, tucking, and precise object handling in dynamic environments. Understanding the Physical World Without Robotics Instead of training AI for specific robotic tasks, Archetype AI’s model, Newton, learns the fundamental rules of physics i.e., how objects move, how energy flows, and how environments change over time. Key Highlights You can process sensor data like vibrations, sound, pressure, and temperature to make sense of the world. Physical Reasoning & Semantic Interpretation: The model uses reinforcement learning to predict real-world behaviors and then translates these predictions into human-understandable insights. Zero-Shot Generalization: Newton can predict physical events it has never seen before, a key step toward general-purpose AI for industrial applications. Use Cases Energy Grid Monitoring: Detecting inefficiencies and preventing failures in power systems. Healthcare & Safety: Identifying falls in elderly care facilities using motion sensors. Manufacturing: Predicting defects in industrial processes using non-visual data. Conclusion The three talks highlight a major shift in AI. Hardware, AI, and data are converging, with the goal of creating AI that understands and interacts more effectively with the physical world. Key takeaways: AI is moving beyond digital data to multimodal sensing and physical interaction. Security cameras are evolving into intelligent AI agents that automate monitoring and safety. Physical AI is revolutionizing warehouse automation through dexterous manipulation and real-time perception. AI models like Newton can generalize physical understanding across industries beyond robotics. The next frontier of AI isn’t just generative AI, it’s machines and intelligent spaces that can understand and navigate the real world. Contact us to learn how Encord can streamline your data to be Physical AI-ready.
Feb 11 2025
5 M
What is LLM as a Judge? How to Use LLMs for Evaluation
Generative AI (Gen AI) is revolutionizing how we interact with computers today. A recent McKinsey survey reports that over 65% of organizations use Gen AI tools to optimize operations. Large Language Models (LLMs) are the backbone of such Gen AI solutions as they allow machines to produce human-quality text, translate languages, and create different types of content. However, evaluating the outputs of LLMs can be challenging, especially when it comes to ensuring coherence, relevance, and accuracy. This is where the concept of LLM-as-a-judge emerges. The LLM-as-a-judge framework addresses these challenges by using one LLM to evaluate the output of another - AI scrutinizing AI. One study suggests LLM judgments match about 80% of human evaluations, indicating that two LLMs agree on judgments at the same rate as human experts. The research concludes that LLM-as-a-judge is a scalable, explainable method compared to hiring human judges.. In this post, we will discuss why LLM-as-a-judge can be valuable in augmenting human reviews, how to use LLMs for evaluation, and how Encord can improve text data quality for LLM. We will also discuss the importance of AI alignment and strategies to improve LLM-based evaluation performance, particularly in the context of chatbot development, large-scale deployments, and real-time decision-making. Why Use LLMs to Judge other LLMs? LLMs can help assess the performance of other LLM systems at greater scale and speed than people with near similar accuracy. When judging LLMs with only human reviewers, there are several factors that can cause failures in the process specifically associated with changing behavior resulting from: Prompt modifications Input method adjustments Adjusting LLM API request parameters Model switching (e.g., from GPT-3 to Llama or Claude) Changes to training data With so many variables, manually checking for improvements or regressions each time a change occurs is simply not feasible. LLM-as-a-Judge (LaaJ) augments the human review aspect by introducing automation to the process of inspecting and judging while still allowing human-in-the-loop evaluations to occur to meet business or regulatory requirements. The goal of using LaaJ is to verify if the LLM system functions as expected within specified parameters as quickly as possible with some high confidence in accuracy. This approach evaluates thousands of LLM outputs without significant dependence on human evaluators, saving time and cost. Moreover, LLM judges ensure consistent evaluation criteria and help minimize subjectivity due to multiple human judges. The approach also helps enhance interpretability and observability in the evaluation process of the model. How LLM-as-a-Judge Works As a part of the LaaJ process, the model conducting the reviews is evaluating the performance of other models, grading and moderating educational content, and benchmarking AI systems. There are four baseline requirements inorder to set-up LLM judging system which can be classified as DDPA, define, design, present, and analyize: Define the judging task Design the evaluation prompt Present the content for evaluation Analyze the LLM and generate judgements evaluating the LLM Judge Let’s explore each of these in more detail. LLM-as-a-Judge evaluation pipelines Define the Judging Task The first step is to clearly define the task for which the LLM will act as a judge. This includes determining the type of content or output to be evaluated. For instance, the task could be assessing the quality of written responses, determining the accuracy of information, comparing multiple outputs, or rating performance based on certain criteria. LLMs are capable of judging various attributes The definition of the task is the foundation for all subsequent steps in the LLM judging process. Here are some examples of task definitions: "Judge the following responses based on their clarity, fluency, and coherence." "Compare the following two summaries and determine which one best captures the main points of the original article." "Rate the following machine translation on a scale of 1 to 5, with 5 being the most accurate and fluent." Design the Evaluation Prompt The next step is to design a prompt for the LLM. Effective prompting improves the accuracy of the assessment while reducing bias from creeping in. What’s are the key components to build a strong evalution prompt? The context of the task (the what): Provide background information to help the LLM clearly understand the evaluation scenario. Specific criteria for evaluation (the who): Defines specific guidelines for judgment, including rating scales, rubrics, or key qualities such as accuracy, coherence, and tone. Instructions on how to format the judgment (the how): Specifies how the LLM should evaluate, whether as a numerical score, a text label, or a more detailed written assessment. Necessary background information (the additional context): Any additional context or data, including reference texts or other relevant materials, that may be needed to fully assess the content. Presenting Content for Evaluation At this stage, judgement begins.Present the content to be judged by the LLM (e.g., LLM-generated content), this is typically done as part of the prompt and the content can be in various formats, such as text, code snippets, or other types of outputs. The presentation might include: A single piece of content for direct assessment. Multiple items for comparison, where the LLM needs to evaluate each separately or in relation to the other. Contextual information, such as a reference document when evaluating for hallucinations or other properties that depend on external information. LLM Analysis and Judgment Generation Upon receiving the prompt and content, the LLM processes the information using its pre-trained knowledge to analyze the input. During the analysis, the LLM understands the context, identifies key elements, and applies the evaluation criteria specified in the prompt. This extremely is important as it determines the LLM's comphrension and output of what it was evaluating - in otherwords, it’s assessing the quality of the judge. Once it analyzes the content, the LLM generates its judgment. This output can take various forms, depending on the instructions in the prompt. For example, it can include: A numerical score from 1-10 that reflects the level of quality or performance. A qualitative assessment provides a descriptive judgment with added context. A comparison between multiple inputs that may involve ranking or selecting a preferred option based on the predefined paramaters Detailed feedback or explanations that justify the LLM's evaluation. This is especially important for identifying areas for improvement in the evaluated content and you’re trying to understand how the Judge came to it’s conclusion Evaluating the LLM Judge The final step is to validate the evaluation process of the LLM judge and give it a grade. Reminder, a human is invovled through every step of the process so far. This involves comparing the LLM's judgments with human evaluations or other benchmarks, such as a "golden dataset," to measure performance and accuracy. Typically at this stage there are only two possible outcomes: 1/move to production becuase it passed, 2/needs to be modified because it failed. It’s important to note, there are several different ways to structure the prompt and evaluate LLM-as-a-Judge. There are two core strategies and you should use the best that aligns with your objectives: Pairwise Comparison: The LLM is presented with two model responses and asked to determine which is better. This is valuable for comparing different models, prompts, or configurations. Single Output Scoring (Pointwise): The LLM evaluates a single response and assigns a score, often using a Likert scale, to assess specific qualities like tone, clarity, and correctness. You can also combine these techniques with the chain of thought (CoT) prompting to improve scoring quality. Types of LLM Evaluation Metrics Relevant LLM evaluation metrics are necessary to assess the performance and quality of LLMs in various tasks. These metrics help determine how well an LLM aligns with intended objectives, ethical standards, and safety requirements. Common LLM evaluation metrics include: Relevance: Evaluate if the LLM's response relates to the given query and whether it addresses the user's question. Relevance is often assessed using human evaluation or automated metrics like BLEU, BERTScore, or cosine similarity. Hallucinations: Checks if the LLM's output includes false information not rooted in the provided context or reference text. A hallucination occurs when the LLM generates answers based on assumptions not found in the reference text. By fine-tuning models and using evaluation datasets, developers can reduce the occurrence of hallucinations. Question-Answering Accuracy: Assesses how well the LLM can answer domain-specific questions correctly and accurately. This metric compares the LLM's response to a ground truth or reference answer. An LLM judge can evaluate the answer by comparing it to the reference answer to ensure that the response conveys the same meaning. Streamlining LLM Data Workflows: A Deep Dive into Encord's Unified Platform. LLMs Metrics for Retrieval-Augmented Generation (RAG) Application Evaluations These metrics are specifically used when evaluating Retrieval-Augmented Generation (RAG) systems. The RAG model retrieves information before generating a response. Common RAG evaluation metrics are: Contextual Relevance Relevance assesses how closely retrieved documents match the original query. Maintaining contextual relevance is crucial for quick response time and high accuracy. An LLM judge can evaluate this by checking if the reference text contains the information needed to answer the question. Faithfulness Faithfulness, known as groundedness, is a metric that assesses how well the LLM's response aligns with the retrieved context. It checks that the answer relies on the retrieved context to prevent hallucinations. Improving LLMs as Judge Performance Engineering practices can help improve the performance of LLMs as judges prompt. You can employ Chain-of-Thought (CoT) prompting. It involves prompting the LLM to articulate its reasoning process, improving explainability and accuracy. Providing clear instructions in the prompt to the LLM judge is crucial for effective evaluations. Instructions should specify the task context, evaluation criteria, and judgment format. There are different approaches to implementing CoT prompting. Two of them include: Zero-Shot CoT: A zero-shot CoT prompt can be implemented by appending a phrase such as "Please write a step-by-step explanation of your score" to the judge’s prompt. Appended prompts let the LLM explain its reasoning. Auto-CoT: Auto-CoT takes zero-shot CoT a step further, where the LLM itself generates the steps for evaluation rather than having them explicitly included in the prompt. Building on these techniques, the G-Eval method also provides a structured evaluation framework for LLM. G-Eval uses auto-CoT prompting to generate evaluation steps from the original criteria, effectively guiding the LLM through a structured evaluation process. For instance, G-Eval might guide the LLM to evaluate "Factual accuracy," "Coherence," and finally "Fluency" before determining an overall score. This structured approach enhances the consistency and explainability of LLM-based evaluations. Beyond CoT prompting, other strategies for improving LLM judge performance include: Few-shot learning: Provide the LLM with several high-quality evaluation examples to clarify the desired criteria and style. Include sample responses, evaluation rubrics, or short dialogues that demonstrate effectiveness. Iterative Refinement: Continuously analyze the performance of the LLM judge and refine the prompts based on its outputs. This iterative process allows for ongoing enhancement in evaluation accuracy and consistency. Structured Output Formats: Using formats such as JSON can make the evaluation results easier to analyze, compare, and share. AI Alignment and Its Importance in LLM-as-Judge Using LLMs as judges provides a solution to the challenges of human evaluation. However, users must ensure that the LLM’s evaluations align with human preferences. When discussing LLMs as judges, such alignment refers to how closely an LLM's judgments match human evaluations. In the above section, we discussed how to enhance LLM performance as evaluators. However, without proper alignment, automated evaluation may yield inaccurate assessments. Here is why AI alignment is critical for LLM-as-Judge: Reliability of Evaluations: The primary goal of using LLMs as judges is to create a scalable and cost-effective alternative to human evaluation. However, if an LLM judge's assessments don't align with human evaluations, the results become unreliable and cannot be trusted. Understanding Strengths and Weaknesses: By comparing LLM judge evaluations with human evaluations, users can identify any failure cases and areas where the judges struggle. Guiding Improvements: Understanding the failure modes and biases of LLMs as judges helps users focus on how to improve them. Research by OpenAI shows that incorporating human feedback via Reinforcement Learning from Human Feedback (RLHF) can improve alignment by up to 30%. Validating LLM-as-Judge: Alignment is important for validating the LLM-as-judge approach itself. If LLMs can't reliably mimic human judgment, their usefulness as automatic evaluators is questionable. AI alignment in LLM-as-judge is not just about matching scores. Instead, it is about ensuring that the LLMs as judges provide reliable evaluations as a proxy for human evaluators, allowing for fair and accurate assessment of LLMs. Challenges of LLM-as-a-Judge LLM-as-a-Judge is a handy technique for evaluating AI systems, but it presents several challenges, including: Data Quality Concerns: LLMs base their evaluation judgments on patterns in text, not on a deep understanding of real-world contexts. Poor data quality can mislead LLM, resulting in inaccurate scores. Data Variety Limitations: LLMs struggle with grading responses to contextual or cultural questions, needing a deep understanding of specific social norms. Inconsistency: While generally consistent, LLMs can sometimes provide inconsistent judgments for complex evaluation tasks, particularly for edge cases or when prompts are slightly changed. Potential Biases: LLMs may have biases from training data, affecting their judgments related to race, gender, culture, or other sensitive attributes. One type is self-enhancement bias, where LLM judges may favor responses generated by themselves. For example, OpenAI GPT-4 may assign higher scores to its own outputs. Encord's Approach for Enhancing Text Data Quality for LLM It's essential to tackle issues like inaccurate scores caused by low-quality or incorrect training data to improve data quality when using LLMs as judges. A solution like Encord can help by simplifying access to relevant raw data and then enhancing the data’s accuracy and reliability. Poor data quality arises when the training data on which the LLM is trained is not using all the relevant and correctly annotated data. Therefore, using a curation and highly-percise annotation tool is key to ensuring that the LLM can make more accurate judgments that are inline with the desired outcome. While many open-source text annotation tools are available, they tend to lack the advanced capaiblities necessary to ensure high-quality text data for training LLMs. Easy to use, scalable, and secure tools such as Encord, are a better alternative as they offer more functionality to deal with complex data management tasks. Encord’s feature rich annotation tool can enhance labeling accuracy and consistency through its powerful AI-assisted labeling workflows with HITL for diverse use cases. Below are a few key features of Encord that can help you with text and document annotation to boost training data quality for LLM judges. Key Features Text Annotation: The Encord annotation tool can help you label large documents and text datasets in different ways. It makes annotation faster and easier through customizable hotkeys, intuitive text highlighting, pagination navigation, and free-form text labels. Encord supports various annotation methods for different use cases, including named entity recognition (NER), sentiment analysis, text classification, translation, and summarization. This variety allows accurate labeling of text data, which is important for training powerful LLMs. Encord RLHF: Encord’s RLHF platform enables the optimization of LLMs through human feedback. This process helps to ensure that model outputs are aligned with human preferences by incorporating human input into the reward function. Model-Assisted Annotation: Encord integrates advanced models such as GPT-4o and Gemini Pro 1.5 into data workflows to automate and accelerate the annotation process. This integration enhances the quality and consistency of annotations by enabling auto-labeling or pre-classification of text content, thereby reducing manual effort. Collaborative Features: Encord facilitates team collaboration by providing customizable workflows that allow distributed teams to work on data annotation, enhancing efficiency. Data Security: Encord complies with key regulations such as GDPR, SOC 2 Type 1, AICPA SOC, and HIPAA. It employs advanced encryption protocols for data privacy compliance. A Complete Guide to Text Annotation. LLM as a Judge: Key Takeaways The concept of LLM-as-a-Judge is a transformative to augment the traditional human review process to evaluating LLMs. It offers scalability, consistency, and cost-efficiency, while still ensuring human engagement remains to the level your business requires. Below are some key points to remember when using LaaJ: Best Use Cases for LLM-as-a-Judge: LaaJ is highly effective for grading educational content, moderating text, benchmarking AI systems, and evaluating RAG applications. It excels in assessing relevance, faithfulness, and question-answering accuracy. LLM-as-a-Judge Challenges: LaaJ can face challenges such as data quality concerns, inconsistency in complex evaluations, and potential biases inherited from training data. Addressing these issues is critical for reliable and fair judgments. Encord for LLM-as-a-Judge: Encord’s advanced tools, including text annotation, RLHF, and model-assisted labeling, enhance the quality of training data for LLMs. These features ensure better alignment with human preferences and improve the accuracy of LLM judges.
Feb 07 2025
5 M
Best Practices for Video Annotation in Multi-Object Tracking
Multi object tracking (MOT) is an essential application of computer vision commonly used in autonomous driving, sports analytics, and surveillance. It involves identifying and tracking multiple objects across video frames while maintaining their unique features. Accurate video labeling is important for training robust MOT models, but the annotation process is complex. Challenges like occlusion, motion blur, and annotation inconsistencies can degrade the MOT model’s performance. High-quality data annotation helps ensure reliable tracking, reducing errors in downstream applications. What is Multi-Object Tracking? It is a computer vision task where you detect and track multiple objects across a video while maintaining their unique features. Unlike single object tracking, which focuses on only a single entity, MOT uses robust algorithms to follow multiple objects simultaneously, even as they interact, overlap, or disappear from the view. MOT is widely used in real-world applications like: Driving autonomous vehicles – Identifying and tracking vehicles, pedestrians, and cyclists in traffic. Surveillance and security – Monitoring people and objects in crowded areas for anomaly detection. Sports analytics – Tracking players and equipment like balls for performance analysis. Robotics – Helping robots navigate dynamic environments by recognizing and following moving objects. By accurately tracking multiple entities over time, MOT enables AI systems to understand motion patterns, predict trajectories, and make informed decisions in real time. How Multiple Object Tracking Works It is a multi step process which combines object detection, feature extraction and tracking algorithms to maintain consistency across video frames. The key stages of an MOT algorithm include: Object Detection The first step is to identify the object in each frame. Object detection models like YOLO, EfficientDet, and more identify objects by drawing bounding boxes around them. These models serve as the starting point for tracking by providing the initial locations of the objects. When combined with action recognition, these computer vision models can help analyze object movements and behaviors, enabling more context-aware tracking in video sequences. This is helpful when tracking humans or animals. For more information on object detection, read the blog Object Detection: Models, Use Cases, Examples Feature Extraction In this step, the distinct features are extracted to distinguish each object that is tracked. The models extract visual and spatial features such as color, shape, texture, and motion patterns. This helps in maintaining object identities when there are multiple similar looking objects present. Data Association This step involves linking the detections from the object detection models for each object across individual frames. The tracking algorithms assign unique IDs to the detected objects and update their positions over time. Common approaches for data association include: Kalman Filters - Predicting the next position of an object based on its previous trajectory. SORT (Simple Online and Realtime Tracker) – A lightweight tracking method that combines Kalman Filters with detection-based updates. DeepSORT – An improved version of SORT that integrates deep learning-based appearance features to reduce identity switches. Transformer-based Trackers – Using self-attention mechanisms to model long-range dependencies for more robust tracking. For more information, read the blog Top 10 Video Object Tracking Algorithms in 2025 Track MAnagement Objects can enter, exit, or temporarily disappear from the scene over time. A robust MOT system must manage: New Tracks – Assigning IDs to newly detected objects. Lost Tracks – Handling objects that become occluded or leave the frame. Merging & Splitting – Adjusting for situations where objects move close together or separate. MOT remains a challenging task due to factors like occlusions, identity switches, and variations in object motion. However, advancements in deep learning, transformer-based trackers, and AI-assisted annotation tools are improving tracking accuracy and efficiency, making MOT increasingly reliable for real-world applications. Key Challenges in Multi-Object Tracking Annotation Occlusion and Object Overlap Objects frequently cross behind obstacles or overlap with each other in a video, making it difficult to maintain accurate tracking. While annotating, you must decide whether to interpolate object positions or to manually adjust the labels. Identity Swaps This happens when there are multiple objects with similar appearances. The tracking model may configure them and assign identity incorrectly. This issue is particularly problematic in crowded scenes, where objects interact frequently. Motion Blur Motion blur occurs when objects move rapidly across frames, causing streaking effects that make detection and tracking difficult. High-speed objects, sudden accelerations, or low-frame-rate videos exacerbate this issue, leading to missed detections or inaccurate bounding boxes. Changes in Object Appearances Across a video, the object’s appearance may also change due to lighting variations, occlusions, transformations, or objects may move closer or farther from the camera. This can lead to incorrect or lost track assignments. Annotation Consistency Maintaining consistent labels across frames is critical for high-quality video datasets. Variability in object positions, bounding box sizes, or identity assignments can introduce noise into the dataset, impacting model performance. Scalability MOT projects often involve thousands of frames and multiple objects per frame, making data labeling time consuming. Without automation, manual tracking can become impractical. Best Practices for Multi-Object Tracking Annotation Use Interpolation Manually annotating every frame is time consuming and more likely to be inconsistent. Interpolation allows annotators to label keyframes, and the system automatically predicts object positions for intermediate frames. This significantly speeds up the annotation process while maintaining accuracy. Define Clear Object Classes and Attributes A well-structured ontology is essential for consistent annotation. Clearly defining object categories, attributes, and tracking rules prevents misannotations and ensures high-quality datasets. Key considerations include: Consistent class definitions – Ensure all annotators understand the differences between object categories. Attribute standardization – Define object attributes like color, motion type, or occlusion status for better classification. Handling ambiguous cases – Establish rules for scenarios like partial occlusions or object merging/splitting. Use AI Assisted Tools AI-assisted annotation tools can track objects across frames, reducing the need for manual intervention. Video annotation tools combine automation with human review to ensure high accuracy. Here are few ways you can use the tools: Pre-trained AI models – Automate initial tracking and let human annotators refine results. Active learning – AI suggests likely object tracks, allowing annotators to accept or modify predictions. Automated identity tracking – Reduces identity switches by using deep learning-based re-identification (ReID) techniques. Ensure Frame-by-Frame Consistency The annotations must be consistent across frames to avoid errors like bounding box jitters, abrupt size changes, or losing track of an object after occlusion. This can be ensured by regularly reviewing annotations and validating as many frames as possible. The use of algorithms like Kalman filters to smooth out object trajectories also help. You should also handle occlusions properly. The occluded objects should be marked minted of removal from frame to maintain tracking integrity. Implement Validation and QA Strategies Quality assurance (QA) ensures the accuracy of multi-object tracking annotations before model training. QA workflows help refine annotations and reduce errors in downstream applications. Effective validation strategies include: Spot-checking – Randomly reviewing frames to detect errors. Consensus-based review – Multiple annotators validate tracking results to reduce biases. Error detection algorithms – Using automated tools to flag anomalies like missing objects or identity swaps. By following these best practices, annotators can create high-quality multi-object tracking datasets that enhance model performance and reduce tracking errors in real-world applications. How Encord Simplifies Multi-Object Tracking Annotation Encord is an annotation platform that streamlines the process of multi-object tracking with its AI assistant tools, streamlined annotation workflows and quality metrics to validate the quality of the annotations. It is designed to handle large-scale tracking projects efficiently. Here is how Encord simplifies MOT annotation process: AI Assisted Tracking The manual annotation of every frame is inefficient, especially when tracking multiple objects across thousands of frames. Encord’s AI-assisted tracking uses the SAM 2.0 model to automatically follow objects across frames. It improves tracking accuracy by adjusting to dynamic object movements and interactions in real-time reducing manual input while ensuring consistent object localization. Be sure to read our blog on SAM 2 to learn more about Encord’s SAM 2 integration. Automated Bounding Box Adjustments Encord’s interpolation feature enables annotators to label keyframes while the system fills in intermediate frames with high accuracy. This ensures smooth object tracking without requiring frame-by-frame manual adjustments. This also prevents annotation drift, where objects gradually shift away from accurate bounding box annotations. Handling Occlusions and Complex Motion Encord allows the annotators to mark occluded objects instead of deleting them. It also uses predictive motion modeling to maintain tracking accuracy even when objects temporarily leave the frame. Video Quality Metrics Encord ensures high-quality annotations for multi-object tracking by providing tools to assess and improve video quality during the annotation process. With the video quality metrics, annotators can identify and address potential issues that may impact tracking accuracy, such as low resolution, motion blur, or frame inconsistencies. Scalable Workflow for Building Large Datasets MOT projects often involve thousands of frames and multiple objects per frame. Encord’s scalable annotation workflow optimizes efficiency by supporting collaborative annotation with multiple reviewers and annotators. By combining AI-powered tracking, automation, and scalable annotation workflows, Encord significantly reduces the time and effort required for multi-object tracking annotation. Step-by-Step Guide: Annotating Multi-Object Tracking in Encord Encord provides a streamlined workflow for annotating multi-object tracking (MOT) projects, reducing manual effort while ensuring high-quality annotations. With the introduction of SAM 2 and SAM 2 Video Tracking, annotation is now even more efficient, allowing for automatic object tracking and segmentation across frames. Here is how you can efficiently track multiple objects in a video using Encord: Step 1: Upload Video Data & Set Up Ontology Log in to Encord Annotate and create a new annotation project. Upload video files or connect external cloud storage. Define a detailed ontology, including object classes, attributes, and relationships, to maintain annotation consistency. Ensure that your ontology includes Polygon, Bounding Box, or Bitmask annotation types to utilize SAM 2. Step 2: Annotate Objects in the First Few Frames Use the bounding box, polygon, or keypoint tools to label objects in the first frame. Assign a unique ID to each object for tracking continuity. Add relevant attributes (e.g., object type, occlusion status, motion category). Step 3: Enable AI-Assisted Object Tracking with Encord’s SAM 2 Integration Activate AI-assisted tracking with SAM 2.0, which automatically follows objects of interest across frames using motion estimation. SAM 2 brings state-of-the-art segmentation and tracking capabilities to video annotation, significantly accelerating the process. Activating SAM 2: Go to Encord Labs in the Settings menu and turn on SAM 2 and SAM 2 Video Tracking (currently in beta). Open an annotation task and select the wand icon next to the Polygon, Bounding Box, or Bitmask annotation tools. Use Shift + A to toggle SAM mode. Using SAM 2 for Object Tracking: Click the object in the frame to enable automatic segmentation and tracking. SAM 2 uses motion estimation to track objects across frames, adapting to occlusions and changes in appearance. If necessary, manually refine object placement in frames where tracking needs adjustments. SAM 2.0 uses advanced motion estimation to predict and track the object’s path. It adapts to complex movements, occlusions, and changes in appearance, ensuring continuous tracking. If needed, manually adjust the tracking in specific frames where the model may need refinement (e.g., during occlusions or sharp changes in movement). Step 4: Use Interpolation to Speed Up Annotation To accelerate the annotation process, use Encord’s interpolation feature to automatically generate object trajectories between keyframes. Follow these steps: Annotate Keyframes: Start by manually annotating the object positions in keyframes, typically at the beginning, middle, and end of an object's motion sequence. These keyframes serve as reference points for interpolation. Activate Interpolation: Once the keyframes are set, Encord’s AI-powered interpolation will automatically generate the object's path in the intermediate frames, smoothly predicting the object’s movement between keyframes. Validate: Examine the interpolated frames to ensure the predicted movement matches the actual motion of the object. If any drift or inaccuracies are identified in the interpolation (e.g., object misalignment or incorrect trajectory), adjust the object’s position in the affected frames. Step 5: Validate Annotations & Use Video Quality Metrics Use the video quality metrics to identify potential issues that could affect tracking accuracy. These metrics allow annotators to assess the quality of video frames and address issues proactively, ensuring accurate tracking over the entire sequence. Resolution: Verify the resolution of the video to ensure clarity, especially for small or distant objects. Low-resolution videos can lead to blurred objects and poor tracking results. Frame Rate: By checking the frame rate, you can ensure that video frames are captured at a sufficient frequency to track fast-moving objects. A low frame rate may result in skipped or inconsistent frames, affecting tracking accuracy. Lighting & Contrast: You can identify the areas with poor lighting or low contrast that can make objects harder to detect or distinguish. By monitoring these conditions in the video content, annotators can adjust the video to ensure that objects are clearly visible throughout the tracking process. Motion Consistency: Inconsistent or erratic object motion is flagged, helping to identify tracking issues such as object occlusion or misalignment. This metric ensures that objects are tracked consistently across frames. These metrics help in pre-emptively identifying issues with the video, enabling you to correct errors and optimize the annotation process before exporting the training data for building machine learning models. Step 6: Export & Integrate with ML Pipelines Export your annotation work in formats like COCO, YOLO, or in a JSON schema. Integrate directly with machine learning pipelines for model training and iterative improvements. Conclusion Multi-object tracking annotation is a crucial yet complex task in computer vision, requiring precision, consistency, and efficiency. Encord simplifies this process through AI-assisted tracking, smart interpolation, and powerful quality metrics, ensuring high-quality annotations while reducing manual effort. By following best practices and leveraging Encord’s tools, you can create accurate, reliable datasets that drive better model performance, ultimately improving the capabilities of object tracking systems across various applications. ⚙️ Automate video annotations without frame rate errors with Encord's AI-assisted video annotation tool.
Feb 05 2025
5 M
Introducing: Upgraded Project Analytics
Encord Upgrades Project Analytics: Granular Data Insights for AI Data Annotation Projects & Teams Today, we are excited to announce an upgraded experience for analyzing project analytics and team performance within the Encord platform. The improved Project Analytics experience gives admins and team managers increased visibility into team performance and project health and progress, with extreme precision, to the label-level. We have redesigned the Project Analytics interface to improve how Human-in-the-loop (HITL) AI data workflows are analysed, managed, and optimised. The upgraded Project Analytics experience includes: Workflow and Agent-native performance metrics to track efficiency & quality Advanced filtering for targeted analysis and comparisons Upgraded CSV exports for seamless external reporting and analysis With this release, customers can now track all of their task, labels, and timer metrics and trends across projects, enabling informed decisions directly from the Encord platform insights. All analytics data is exportable as CSV files, with SDK access coming soon. Early adopters of Encord’s Project Analytics are already seeing a major impact on their workflows - “Project Analytics gives us real-time tracking with clear data visualizations, making it easy to see how every stage of our workflow is performing. We can compare team output, efficiency, and quality at a glance, with advanced filters for deeper analysis.” With this powerful upgrade, Encord is redefining how teams analyze, manage, and optimize their AI data workflows. Whether you're looking to streamline annotation projects, enhance team performance, or make data-driven decisions with ease, our enhanced Project Analytics dashboard provides the precision and flexibility you need. Ready to experience the difference? Book a demo today and see how Encord can help you unlock deeper insights, improve efficiency, and take full control of your data workflows.
Feb 05 2025
5 M
Key Challenges in Video Annotation for Machine Learning
“Did you know? A 10-minute video at 30 frames per second has 18,000 frames, and each one needs careful labeling for AI training!” Video annotation is essential for training AI models to recognize objects, track movements, and understand actions in videos. But it’s not easy as it presents many challenges. This article explores these challenges and explains how tools like Encord help in annotating video data faster and more accurately. What is Video Annotation? Video annotation is the process of annotating objects, actions, or events in video data to help machine learning (ML) models understand and recognize these objects when exposed to new video data. This annotation process involves identifying and marking objects of interest across multiple frames with the help of annotation tools and different annotation types. This labeled (annotated) data serves as the foundation for training ML models to recognize, track, and understand patterns in video data. It’s like giving a guidebook to machines so that they can use this guide to understand what is in the video data. Video Annotation in Encord (Source) ⚙️ Automate video annotations without frame rate errors with Encord's AI-assisted video annotation tool. Types of Video Annotations Video annotation can take different forms depending on the specific use case. Following are the different types of methods used to annotate objects in a video frame. Types of Annotations in Encord Bounding Boxes Annotation Bounding box annotation is a method of annotating an object using rectangular boxes. This type of annotation helps to identify and locate objects within an image or video. Bounding box annotation is used for tasks like object detection to recognize and locate multiple objects in a scene. It assists in training computer vision models to detect and track objects. Bounding Box Annotation in Encord (Source) Polygon Annotation Polygon annotation is a method of annotating objects by drawing detailed and custom-shaped boundaries around them. Unlike bounding boxes, polygons can closely follow the contours of objects that have complex shapes. This type of annotation is particularly useful for tasks like detecting and segmenting objects with complex shapes, such as trees, buildings, or animals, in computer vision models. Polygon annotation in Encord (Source) Keypoints Annotation Keypoints annotation is the method of marking specific points of interest on an object such as the joints of a human body. These points help in identifying and tracking features of the object. Keypoint annotation is used to build computer vision applications like activity recognition, pose estimation, or facial expression analysis. Keypoint Annotation in Encord (Source) Polyline Annotations Polyline annotations are used to draw lines along objects like roads, lanes, or the paths of moving objects. Polyline annotation is used when we want to annotate non-closed shapes. This method is helpful for tasks such as lane detection in autonomous driving or tracking the movement of objects over time in video data. Polyline Annotation in Encord Segmentation Mask Annotation Semantic mask annotation or bitmask annotation is a method where each pixel in an image or video is labeled with a specific class, such as "car," "road," or "person." This pixel-level annotation provides a detailed understanding of the objects in an image or a video frame. This annotation method is used to build applications for scene understanding, medical imaging, or environmental monitoring. Segmentation Mask (Bitmask) Annotation in Encord Understanding the Complexity of Video Data Video data is more complex than image or textual data because it has multiple dimensions, including spatial, temporal, and contextual information. This complexity makes it a challenging but essential component for artificial intelligence (AI) applications. High Data Volume Videos consist of sequences of frames (images) which are captured at high frame rates such as 30 or 60 frames per second. This generates a large amount of data even for short video clips. For example, a 10-second video at 30 fps may have 300 frames to process and annotate. Higher resolutions, such a 4K, increases the data size and computational requirements. Videos require high storage capacity due to their size and annotating such video for building machine learning models requires advanced tools to handle these large datasets efficiently. Temporal Dimension Unlike images, videos capture sequences of events over time. This adds a temporal layer of complexity. While annotating such video the relationships between successive frames must be understood to make sense of movements, interactions, or changes. For example, tracking a person while he is walking involves understanding his motion across multiple frames. In applications like action recognition, temporal understanding is important for recognizing actions or events. For example, detecting a vehicle slowing down or identifying two persons having handshakes in surveillance footage. Richness of Content Videos often contain multiple objects interacting simultaneously. For example, in a traffic video you may see multiple pedestrians, vehicles, and cyclists. Annotating and understanding these interactions is complex but it is important for applications like autonomous vehicles. Real-world videos also have dynamic and unpredictable scenarios such as a change in lighting or weather conditions, or even change in appearances of objects. This makes it challenging to maintain consistent annotations. Object Motion and Tracking Fast-moving objects in video can appear blurry in frames which makes it difficult to accurately detect and track them. For example, sports videos often have fast moving objects such as a ball traveling at high speed. Objects may also be partially or completely obscured by other objects which leads to complexity in detection or tracking. For example, a pedestrian walking behind a vehicle may be partially visible in certain frames. The video data is complex as we have discussed in the above points. Handling such data is challenging and requires advanced annotation tools and techniques as well as powerful computational resources to build machine learning models capable of capturing both spatial and temporal information. Video data is a key data source for AI applications therefore understanding and addressing complexity of such data is important for building effective AI solutions. Key Challenges in Video Annotation Video annotation is very important for training machine learning and computer vision models. However, the process of annotating video is too complex because the video data is dynamic and multi-dimensional. Following are the key challenges in video annotation. Scalability Annotating video data requires labeling thousands to millions of frames of videos because it has high-resolution and sometimes high-frame-rates. This process of data annotation is time-consuming and resource-intensive. For example, annotating a 10-minute video at 30 frames per second (fps) generates 18,000 frames. Each frame must be labeled for objects, actions, or events, which could take days or weeks for a team of annotators. The manual annotation for such large-scale data becomes impractical without automated annotation tools and as a result it leads to delay in project timelines or error in data annotation. Consistency Across Frames Ensuring consistency in video data annotations across successive frames is difficult because the objects may change in appearance, size, or position. For example, a car driving into the frame may be labeled differently in terms of boundary size or position across multiple frames which may result in inconsistencies in annotations. This is a common issue when different annotators are working on the same project. Inconsistent annotations can result in poorly trained models with unreliable predictions. Temporal Understanding Temporal understanding in video annotation refers to the ability to analyze and interpret how objects, actions, or events change and move over time in a video. Unlike images, videos capture motion and sequences, so temporal understanding focuses on tracking these changes frame by frame. Annotating this temporal aspect is much more complex than labeling the static image data. For example, in a surveillance video, identifying "a person picking up an object" requires annotators to mark the entire sequence of frames where the action occurs and not just the key moments. If annotators mislabel actions or fail to annotate the entire sequence, it will reduce the ability of ML models to understand and recognize events. Handling Occlusions Sometimes objects to be tracked become partially or fully occluded by other objects in video. This makes it hard to track and annotate such objects accurately. For example, in the image (center image) below the person on the right is partially occluded by the one on the left, making part of his body less visible. Annotators must predict his position in such cases. Incorrect labeling of occluded objects leads to incomplete data and reduces the ability of trained models to track objects in real-world scenarios. Object Tracking during and after Occlusion (Source) Motion Blur and Poor Visibility Objects in video that move fast may sometimes appear blurred. This makes it hard to define the boundaries or track such fast moving objects. For example, a fast moving ball in sports videos may appear as a streak which makes it challenging to annotate its exact position in a frame. Annotated data for such objects may lack precision which can affect the accuracy of models. Fast moving Train causing a Motion Blur (Source) Annotation Tools Limitations Many existing annotation tools are not optimized for handling large-scale, complex video datasets as well as advanced automated annotation features. If an annotation tool does not support automated annotation , it will force annotators to manually label objects in every frame of a video which increases the workload. Inefficient tools slow down the annotation process and increase costs. Cost and Expertise Annotating video data is labor-intensive and requires skilled annotators. For example, annotating medical videos that consist of events such as surgical procedures requires domain-specific expertise to label tools, anatomy, and actions correctly. High costs and the need for specialized skills make video annotation less accessible for smaller projects or research groups. Quality Assurance Ensuring the accuracy of annotation across thousands of frames is a challenging task. The quality assurance becomes more difficult when there are multiple annotators of different skill sets are involved. FOr example, two annotators may label the same object differently in the same video which may lead to inconsistencies in the annotation. Poorly annotated data reduces the accuracy of ML models. So it is important to have strong quality control measures. Real-Time Requirements In some annotation tasks such as annotating data for autonomous driving or security surveillance applications, the real-time annotations for quick decision-making is required. For example, annotating video data for autonomous cars requires labeling of objects like pedestrians and traffic signals in milliseconds because these objects move with speed or happen very quickly across video frames. The real-time annotation of such events requires advanced annotation tools. How Encord helps in Video Annotations Encord helps to achieve high quality video annotation with granular tooling, customizable workflows and automated pipelines. Encord helps solve your most complex computer vision annotation tasks. Following are some important features of Encord platform which helps in high quality annotation tasks. AI-Assisted Annotation Tools Encord uses AI assisted annotation to simplify and speed up the annotation process. Encord has an automated object tracking feature which tracks objects across frames and also maintains consistency of annotation. The platform also integrates the Segment Anything Model (SAM), which automatically segments objects in video frames. Encord allows users to use model-assisted tools to make the labeling process faster and more efficient. Encord’s AI-assisted labeling feature allows the use of state-of-the-Art (SOTA) foundation models as well as your own custom models to automate video annotation. These models can pre-label data which means that it automatically suggests annotations for objects, actions, or events in your videos. This saves time and effort by reducing the manual work needed for labeling. By integrating these models directly into the workflow, you can speed up the annotation process and focus on refining the results. The AI assisted annotation makes video annotation tasks faster and more efficient. AI-assisted labeling with SOTA foundation models (Source) Comprehensive Annotation Capabilities Encord offers a collection of annotation tools for video annotation to meet the needs of all types of video annotation projects. It provides bounding box annotation to annotate objects for object detection tasks by enclosing them in rectangular frames. To annotate irregularly shaped objects it provides a polygon annotations tool. For pose estimation projects it provides a keypoint annotation tool. The dynamic attributes in the Encord platform helps ensure that the annotations capture temporal changes to accurately detect the objects that appear over time in a video frame. For example, if you’re annotating a video of a car, you can track its attributes like its speed, color, or direction, and update them as they appear across multiple frames. This is especially useful for videos where objects or their characteristics are not static but change dynamically. By capturing these changes, Encord helps create more detailed and accurate datasets which is important to train advanced ML models for various real-time applications such as autonomous driving, activity recognition, or surveillance systems. The following image shows annotation of a moving hen using dynamic attribute. Working with Dynamic Attributes in Encord (Source) This powerful capability makes Encord a powerful tool for complex annotation needs. Scalability for Large Video Datasets Encord is built to handle large video datasets with ease and efficiency. It allows annotators to work on multiple videos at the same time which saves time and effort. Automated workflows make it simple to manage data by organizing tasks like importing, annotating, and exporting videos. The performance analytics feature offers clear insights into the progress and quality of annotations which helps teams to manage large-scale projects effectively. Collaboration and Quality Assurance Encord makes teamwork and quality control easy by offering features that help teams to work together and ensure high quality annotations.Encord allows teams to create custom workflows according to their specific needs which may include steps for annotating, reviewing, and approving data. Multiple team members can work on the same project at the same time with real-time updates which keeps all members updated. Encord helps teams to review and check annotations systematically. It helps team members make sure that the annotations are accurate and consistent. Advanced Features for Temporal Data Encord is a great tool to work with video data in which objects in video changes over time. Encord provides a frame synchronization feature to make sure that the annotations are consistent across multiple frames even when annotated objects are moving or the background is changing for the objects. Encord also helps in time-series annotation which means that you can annotate events or actions that happen over time. It also supports action segmentation by breaking down continuous actions into small sub-segments. These features provided by Encord help annotators to get the most out of video data by focusing on how things change and evolve over time. Video annotation using Encord (Source) The image shows an annotation of a warehouse video using Encord. In this annotation Autonomous Mobile Robots (AMRs) and inventory are labeled with color-coded overlays for object detection and tracking. The timeline highlights active frames for each annotation, with options for automated labeling and manual adjustments. Integration with Machine Learning Pipelines Encord provides APIs and SDKs that make it simple to create workflow scripts. This helps to quickly develop effective data strategies. You can set up advanced pipelines and integrations in just minutes to save time and effort. Encord also provides various data export options and supports various formats for easy integration into training pipelines which are compatible with different ML frameworks. Encord SDK (Source) In summary, Encord provides all the necessary tools to tackle all the challenges in video annotation. Encord thus helps in building advanced ML models that can recognize objects, actions, or events in video data. Key Takeaways Video annotation is crucial for training machine learning (ML) models to detect and track objects, actions, and events in videos. It powers applications like self-driving cars, surveillance systems, and activity recognition by providing well-labeled data. However, it comes with its own set of challenges. Why Video Annotation Matters: Video annotation helps ML models understand and analyze video content to make it possible to recognize patterns, track movements, and detect events over time. Challenges in Video Annotation: There are many challenges in video annotation such as: Videos contain a lot of frames which makes annotation time-consuming. Keeping annotations accurate and consistent across frames is hard. Issues like occlusions (objects blocking each other), motion blur, and understanding how things change over time is difficult. Handling large datasets efficiently can be challenging. Not all tools can handle advanced annotation needs or real-time requirements. How Encord Helps: Encord simplifies video annotation with AI-assisted tools, automated object tracking, and a variety of annotation options. It supports large datasets, allows easy integration with ML pipelines, and ensures high-quality results through workflow automation. This makes the process faster, more accurate, and scalable. Accelerate labeling projects and build production-ready models faster with Encord Annotate.
Jan 31 2025
5 M
Explore our products