Contents
What is Computer Vision?
How Computer Vision Works in Agriculture?
Benefits of Computer Vision in Agriculture
Challenges of Computer Vision Applications in Agriculture
What is the Future of Smart Farming?
Computer Vision in Agriculture: Key Takeaways
Encord Blog
Computer Vision in Agriculture: The Age of Agricultural Automation through Smart Farming
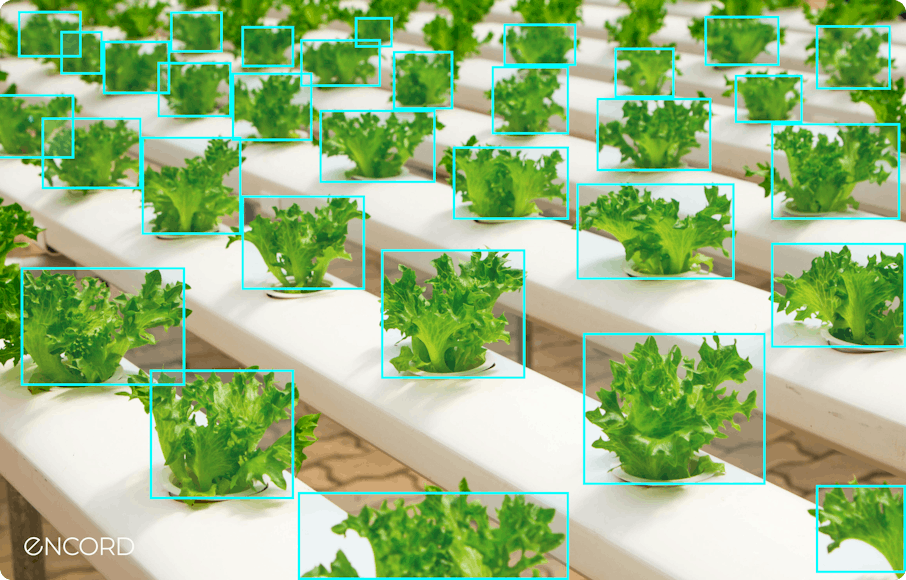
Agriculture accounts for 4% of the global gross domestic product (GDP) and 25% of GDP in the least developed countries. The global population's dependence on agriculture makes it a crucial part of human life. However, agricultural errors such as poor crop and livestock management, climate change, and farming mistakes can reduce yields and increase costs, affecting the global population.
Smart farming uses computer vision (CV) systems to minimize human errors and optimize agricultural processes. Computer vision involves capturing and analyzing digital images to extract meaningful information.
In agriculture, CV systems can detect early signs of crop stress, disease, or pest infestations, enabling precise decision-making. Additionally, CV automates tasks like weed control and fruit sorting, reducing the need for human labor and increasing production efficiency.
In this article, we will explore the various applications, benefits, and future computer vision trends in agricultural automation, highlighting its role in enabling smart farming practices and transforming the agriculture industry.
What is Computer Vision?
Computer Vision (CV) enables machines to interpret visual information in the real world. The area involves developing deep learning algorithms, which are advanced machine learning (ML) techniques that can automatically learn and improve from experience, and architectures to analyze visual data, such as images and videos, and extract relevant patterns to understand the content present in the data.
Common CV techniques include image classification, object detection, instance and semantic segmentation, and video object tracking. These techniques require data annotation, which labels data to provide meaningful information about its content. Clear tags allow users to train and test machine vision systems efficiently.
Computer vision models mostly use convolutional neural networks (CNNs) to break images into smaller chunks. They recognize properties such as shapes, corners, colors, textures, and edges to understand what the image contains and assign relevant labels.
In recent years, improvements in computer power have made it possible to process huge amounts of visual data and CV algorithms. As a result, computer vision is being used increasingly in many areas, such as autonomous driving, facial recognition, retail, manufacturing, healthcare, agriculture, and robotics.
Here, we will focus on agricultural applications in which CV technology allows nations to boost agricultural productivity and yields to satisfy increasing food demand.
How Computer Vision Works in Agriculture?
CV systems enable farmers and other agricultural workers to automate workflows by analyzing agricultural fields using real-time algorithms. These techniques rely heavily on deep learning technologies to perform video analytics.
Additionally, edge computing is used, where devices such as cameras, scanners, unmanned aerial vehicles (UAVs), and other Internet-of-Things (IoT) devices have built-in CV algorithms to analyze live data feeds instantly.
Edge computing allows faster processing and reduced latency, as the data is processed locally on the device rather than sent to a central server.
Automated Sorting
Grading and sorting is a quality assurance farming practice that categorizes yield according to multiple attributes and quality parameters, such as ripeness, weight, color, shape, and size.
Performing the process manually is time-consuming and error-prone. With CV algorithms, farmers can quickly grade thousands of product categories in minimal time with high accuracy.
For instance, an image classification system can quickly detect anomalies in particular plants, vegetables, and fruits and label them as fresh, diseased, rotting, etc., to indicate their quality level.
Automated Harvesting
Harvesting involves picking mature crops, rice, animals, or fish that people can consume. It requires farmers to ensure they harvest at the right time when the produce is healthy.
While traditional machines like combine harvesters improve the harvesting process, they still require manual input. The farmer must predict the appropriate harvesting season, crop yield, variety, and maturity period before using the harvester to pick the produce.
CV systems use robotics with advanced detection functionality to analyze and predict harvesting seasons for crops such as wheat, corn, or soybeans. They also use mechanical grasping systems to pick mature crops, fruits, or vegetables from the field.
The CV system can consider multiple variables, such as plant growth stage, weather conditions, and soil moisture, to calculate the precise harvesting period, ensuring minimal waste and better food security. It integrates with robotic arms equipped with cutting and grasping tools to handle produce gently.
Weed Detection
Weeds are unwanted plants that interfere with the growth of other desirable crops for human consumption.
Detecting and eradicating weeds is a complex and tedious process. An efficient weed management system is required to ensure crop health and prevent pest infestations in the produce.
With CV imaging methods, farmers can optimize the weed detection process by using UAVs, RGB cameras, robots, and satellites to automatically identify weed-infected areas.
The CV algorithms can distinguish between normal crops and unwanted weeds while providing information on the exact weed species.
The method can help farmers plan and surgically apply relevant herbicides and pesticides in infected areas without damaging nearby plants.
Plant Disease Detection
Computer vision-based models allow farmers to implement precision agriculture for proactive crop monitoring fields and help collect relevant data and metrics to detect issues.
For instance, CV models can perform advanced image analysis for plant phenotyping using satellite images and remote-sensing devices to capture high-resolution images. The technique helps farmers detect signs of illness early in the plant growth process.
It also significantly improves crop production, as farmers can quickly apply treatments to ensure plants remain healthy. It also prevents crop diseases from spreading to other nearby plants, ensuring the long-term sustainability of the produce.
Livestock Monitoring
CV models’ capabilities extend beyond crop management to livestock farming, where advanced vision systems help monitor animals such as sheep, fish, cattle, etc.
The models help precision farming by using machine learning models to collect relevant data regarding animal species, their counts, and health status. The information helps with resource optimization as farmers can accurately estimate the feed and area required to grow their livestock.
Using advanced image processing, CV systems also help farmers track animal movements and assess behavior to detect anomalies and potential diseases.
Yield Prediction
Yield estimation is a critical pre-harvest practice that requires high precision to avoid additional costs associated with over or underestimation. Overly optimistic estimates cause resource wastage as farmers inaccurately allocate excessive resources for the harvesting period. Similarly, underestimation leads to crop wastage as the farmer fails to harvest all the consumable crops.
To accurately estimate yield potential, automated CV systems analyze multiple variables, including plant nutrients, pest infestations, fruit ripeness, temperature, rainfall, and soil type.
By combining data from satellite imagery, weather stations, and soil sensors, CV algorithms can create predictive models that estimate crop yields based on these factors. This helps farmers optimize resource allocation and more effectively plan for the harvesting season.
Soil Analysis
Monitoring and assessing soil health is crucial for maintaining healthy plant growth. However, analyzing different soil types manually is tedious and requires significant expertise to judge which soil type is suitable for which crop.
Edge computing is a valuable technique that uses drones, ground sensors, and satellite imagery to judge soil conditions. The devices have in-built CV models that capture soil images and detect soil suitability based on color.
The analysis provides information on acidity and soil moisture levels to help farmers build automated irrigation management systems to ensure minimal water wastage.
Benefits of Computer Vision in Agriculture
The above applications highlight the significance of CV models in modern agriculture. With remote sensing and edge computing functionalities, CV systems can help farmers implement an end-to-end smart agriculture pipeline that minimizes manual labor and costly errors.
The following benefits demonstrate how computer vision is revolutionizing the agricultural sector:
Enhanced Decision-making
CV models provide farmers with accurate and timely information regarding crop growth, crop health, environmental conditions, pest infestations, potential diseases, soil analysis, and yield predictions.
The data helps farmers efficiently allocate resources, determine the required amount and type of fertilizers, irrigation systems, and pesticides, and plan better for harvesting periods.
Enhanced Efficiency
Automated systems powered by computer vision enable precision farming practices. This involves timely detection and removal of weeds and surgically supplying water and pesticides to areas that need them the most.
By optimizing resource allocation and proactively responding to potential issues such as dwindling plant health, potential diseases, and rising soil acidity, farmers can save time and money while ensuring optimal crop growth.
Enhanced Sustainability
With CV models helping farmers detect pest infestations, soil erosions, and other pollutants, the system helps preserve biodiversity.
Further, the targeted supply of pesticides using remote sensing and satellite imaging prevents run-offs to rivers and lakes that can harm nearby plant and insect species.
Lastly, CV systems help with optimal land management by allowing farmers to analyze soil health and suitable plantation areas.
Challenges of Computer Vision Applications in Agriculture
Despite computer vision's immense potential in agriculture, implementing CV systems presents its own set of challenges.
The following issues may hinder the adoption of AI-powered solutions in agricultural workflows:
Initial Investment in Hardware and Software
Implementing end-to-end CV systems requires a significant financial investment in the necessary infrastructure, such as remote sensing devices, drones, cameras, and relevant software.
Small farms situated in rural areas may face additional challenges due to limited internet connectivity and inadequate power supply.
While the high upfront costs may be a barrier, governments can support farmers through grants, subsidies, tax exemptions, and the provision of basic infrastructure. These initial investments can lead to long-term savings and increased productivity.
Data Privacy
Smart farming relies heavily on quality data regarding crops, soil, livestock, and environmental conditions. Appropriate data protection policies must be in place to prevent misuse and exploitation.
Compliance with national and international regulatory laws can be challenging, and farmers must ensure that their technology providers have clear data protection guidelines to prevent data breaches.
Establishing comprehensive agreements with providers and implementing robust data governance frameworks are crucial to ensuring data privacy and regulatory compliance. Data breaches can have severe consequences, such as financial losses, reputational damage, and legal repercussions, underscoring the importance of data protection.
Technical Training
Installing and operating CV systems requires specialized training to use the hardware and software components for optimal results. Additionally, data collection and analysis requires data experts who understand how to store, manage, and visualize key metrics for a smart management system.
The farming workforce may find it challenging to acquire these skills and may need to hire external staff to manage the technical workload. However, hiring additional workers will increase operational costs.
Again, the government can play a relevant role by offering low-cost training programs to farmers to close the skills gap. Farmers can also select technology providers offering continuous technical support to streamline learning.
What is the Future of Smart Farming?
Despite the challenges, smart farming is the future of agriculture, as it offers great benefits over traditional farming practices.
Below are a few ways emerging technologies will shape how smart farming works in the coming years:
- Enhanced Recommendation Systems: With advanced multimodal frameworks (e.g., Meta-Transformer), AI will offer better recommendations for plantation, irrigation, pest control, and harvesting. It will comprehend visual, text, and audio data in more detail, emulating human cognitive abilities.
- Spatial Intelligence: Spatial intelligence involves deriving greater insights from visual data using 3D spatial models to simulate infinite environments. The technology will allow farmers and researchers to understand how plant species grow in different environments and select the best conditions for growing a particular species. It will also allow researchers to build smarter robots that can quickly detect diseases and recommend treatments for better results.
- IoT and Connectivity: IoT devices will be central in smart farming, with better ground sensors to detect changes in soil and environmental conditions. Deploying 5G networks will ensure robust connectivity and integration between multiple IoT devices. This trend will allow farmers to collect more detailed data (visual, satellite, temperature, timeseries) and connect with multimodal systems to derive greater insights in real-time.
- Genetic Engineering: As CV frameworks mature to better understand plant and animal phenotypes, researchers can integrate these systems with sequential models to process genetic data. The insights will allow researchers to engineer plant species robust to multiple diseases and pests. The trend will lead to higher yields for farmers and better food security.
Computer Vision in Agriculture: Key Takeaways
As CV algorithms become more advanced, their use in agriculture will rapidly increase, automating a significant portion of the agricultural process.
Below are a few key points to remember regarding CV applications in agriculture:
- Primary CV Use Cases in Agriculture: Automated sorting, harvesting, weed detection, plant disease detection, livestock monitoring, soil analysis, and yield estimation are significant ways CV improves the agricultural process.
- Benefits of CV in Agriculture: By automating tedious agricultural workflows, CV reduces costly errors, increases yield, and ensures better food security.
- Challenges of Implementing CV in Agriculture: High upfront costs, data privacy issues, and a technical skills gap are a few challenges farmers face when implementing CV systems for smart farming. However, with adequate government support, farmers can overcome these problems.
Better Data, Better AI
Enhance your AI with automated data curation, annotation, and validation.
Try it todayWritten by
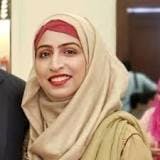
Haziqa Sajid
- Smart farming involves automating agricultural processes using advanced technology, such as artificial intelligence, the Internet of Things (IoT), and data analytics.
- Farmers use computer vision to automate agricultural workflows by proactively monitoring fields and detecting signs of diseases and pests.
- Image classification and object detection help detect pest infestations by analyzing images for signs of potentially harmful insects.
- Computer vision systems are adaptable to different climates and environmental conditions. Farmers can train CV models on region-specific data to detect particular species of plants, crops, and insects in a specific environment.
- Farmers must invest in high-resolution cameras, drones, data collection and storage solutions, data management systems, and analytics tools to derive valuable insights.
- Computer vision applications in agriculture include automated harvesting and sorting, soil analysis, yield prediction, livestock monitoring, and plant disease detection.
- Computer vision helps farmers improve decision-making, reduce labor costs, increase sustainability, and boost food security and production.
Explore our products