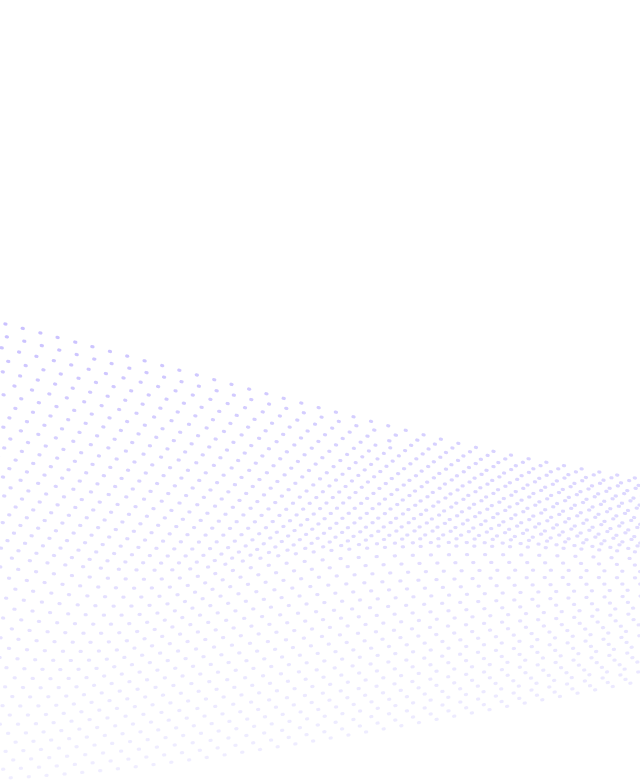
Encord Blog
Immerse yourself in vision
Trends, Tech, and beyond
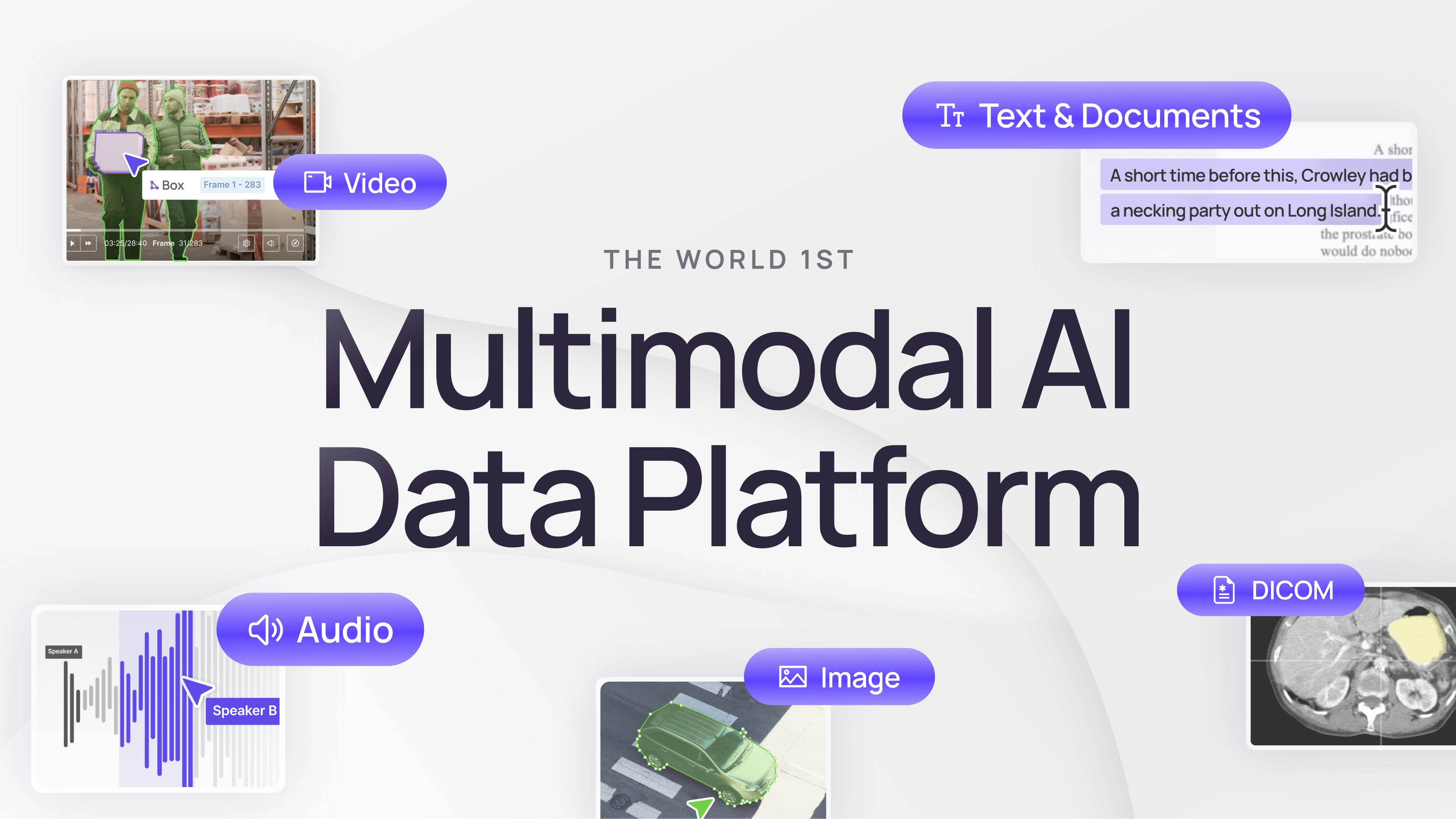
Encord is the world’s first fully multimodal AI data platform
Encord is the world’s first fully multimodal AI data platform Today we are expanding our established computer vision and medical data development platform to support document, text, and audio data management and curation, whilst continuing to push the boundaries of multimodal annotation with the release of the world's first multimodal data annotation editor. Encord’s core mission is to be the last AI data platform teams will need to efficiently prepare high-quality datasets for training and fine-tuning AI models at scale. With recently released robust platform support for document and audio data, as well as the multimodal annotation editor, we believe we are one step closer to achieving this goal for our customers. Key highlights: Introducing new platform capabilities to curate and annotate document and audio files alongside vision and medical data. Launching multimodal annotation, a fully customizable interface to analyze and annotate multiple images, videos, audio, text and DICOM files all in one view. Enabling RLHF flows and seamless data annotation to prepare high-quality data for training and fine-tuning extremely complex AI models such as Generative Video and Audio AI. Index, Encord’s streamlined data management and curation solution, enables teams to consolidate data development pipelines to one platform and gain crucial data visibility throughout model development lifecycles. {{light_callout_start}} 📌 Transform your multimodal data with Encord. Get a demo today. {{light_callout_end}} Multimodal Data Curation & Annotation AI teams everywhere currently use 8-10 separate tools to manage, curate, annotate and evaluate AI data for training and fine-tuning AI multimodal models. It is time-consuming and often impossible for teams to gain visibility into large scale datasets throughout model development due to a lack of integration and consistent interface to unify these siloed tools. As AI models become more complex, with more data modalities introduced into the project scope, the challenge of preparing high-quality training data becomes unfeasible. Teams waste countless hours and days in data wrangling tasks, using disconnected open source tools which do not adhere to enterprise-level data security standards and are incapable of handling the scale of data required for building production-grade AI. To facilitate a new realm of multimodal AI projects, Encord is expanding the existing computer vision and medical data management, curation and annotation platform to support two new data modalities: audio and documents, to become the world’s only multimodal AI data development platform. Offering native functionality for managing and labeling large complex multimodal datasets on one platform means that Encord is the last data platform that teams need to invest in to future-proof model development and experimentation in any direction. Launching Document And Text Data Curation & Annotation AI teams building LLMs to unlock productivity gains and business process automation find themselves spending hours annotating just a few blocks of content and text. Although text-heavy, the vast majority of proprietary business datasets are inherently multimodal; examples include images, videos, graphs and more within insurance case files, financial reports, legal materials, customer service queries, retail and e-commerce listings and internal knowledge systems. To effectively and efficiently prepare document datasets for any use case, teams need the ability to leverage multimodal context when orchestrating data curation and annotation workflows. With Encord, teams can centralize multiple fragmented multinomial data sources and annotate documents and text files alongside images, videos, DICOM files and audio files all in one interface. Uniting Data Science and Machine Learning Teams Unparalleled visibility into very large document datasets using embeddings based natural language search and metadata filters allows AI teams to explore and curate the right data to be labeled. Teams can then set up highly customized data annotation workflows to perform labeling on the curated datasets all on the same platform. This significantly speeds up data development workflows by reducing the time wasted in migrating data between multiple separate AI data management, curation and annotation tools to complete different siloed actions. Encord’s annotation tooling is built to effectively support any document and text annotation use case, including Named Entity Recognition, Sentiment Analysis, Text Classification, Translation, Summarization and more. Intuitive text highlighting, pagination navigation, customizable hotkeys and bounding boxes as well as free text labels are core annotation features designed to facilitate the most efficient and flexible labeling experience possible. Teams can also achieve multimodal annotation of more than one document, text file or any other data modality at the same time. PDF reports and text files can be viewed side by side for OCR based text extraction quality verification. {{light_callout_start}} 📌 Book a demo to get started with document annotation on Encord today {{light_callout_end}} Launching Audio Data Curation & Annotation Accurately annotated data forms the backbone of high-quality audio and multimodal AI models such as speech recognition systems, sound event classification and emotion detection as well as video and audio based GenAI models. We are excited to introduce Encord’s new audio data curation and annotation capability, specifically designed to enable effective annotation workflows for AI teams working with any type and size of audio dataset. Within the Encord annotation interface, teams can accurately classify multiple attributes within the same audio file with extreme precision down to the millisecond using customizable hotkeys or the intuitive user interface. Whether teams are building models for speech recognition, sound classification, or sentiment analysis, Encord provides a flexible, user-friendly platform to accommodate any audio and multimodal AI project regardless of complexity or size. Launching Multimodal Data Annotation Encord is the first AI data platform to support native multimodal data annotation. Using the customizable multimodal annotation interface, teams can now view, analyze and annotate multimodal files in one interface. This unlocks a variety of use cases which previously were only possible through cumbersome workarounds, including: Analyzing PDF reports alongside images, videos or DICOM files to improve the accuracy and efficiency of annotation workflows by empowering labelers with extreme context. Orchestrating RLHF workflows to compare and rank GenAI model outputs such as video, audio and text content. Annotate multiple videos or images showing different views of the same event. Customers would otherwise spend hours manually Customers with early access have already saved hours by eliminating the process of manually stitching video and image data together for same-scenario analysis. Instead, they now use Encord’s multimodal annotation interface to automatically achieve the correct layout required for multi-video or image annotation in one view. AI Data Platform: Consolidating Data Management, Curation and Annotation Workflows Over the past few years, we have been working with some of the world’s leading AI teams such as Synthesia, Philips, and Tractable to provide world-class infrastructure for data-centric AI development. In conversations with many of our customers, we discovered a common pattern: teams have petabytes of data scattered across multiple cloud and on-premise data storages, leading to poor data management and curation. Introducing Index: Our purpose-built data management and curation solution Index enables AI teams to unify large scale datasets across countless fragmented sources to securely manage and visualize billions of data files on one single platform. By simply connecting cloud or on prem data storages via our API or using our SDK, teams can instantly manage and visualize all of your data on Index. This view is dynamic, and includes any new data which organizations continue to accumulate following initial setup. Teams can leverage granular data exploration functionality within to discover, visualize and organize the full spectrum of real world data and range of edge cases: Embeddings plots to visualize and understand large scale datasets in seconds and curate the right data for downstream data workflows. Automatic error detection helps surface duplicates or corrupt files to automate data cleansing. Powerful natural language search capabilities empower data teams to automatically find the right data in seconds, eliminating the need to manually sort through folders of irrelevant data. Metadata filtering allows teams to find the data that they already know is going to be the most valuable addition to your datasets. As a result, our customers have achieved on average, a 35% reduction in dataset size by curating the best data, seeing upwards of 20% improvement in model performance, and saving hundreds of thousands of dollars in compute and human annotation costs. Encord: The Final Frontier of Data Development Encord is designed to enable teams to future-proof their data pipelines for growth in any direction - whether teams are advancing laterally from unimodal to multimodal model development, or looking for a secure platform to handle immense scale rapidly evolving and increasing datasets. Encord unites AI, data science and machine learning teams with a consolidated platform everywhere to search, curate and label unstructured data including images, videos, audio files, documents and DICOM files, into the high quality data needed to drive improved model performance and productionize AI models faster.
Nov 14 2024
m
Trending Articles
1
The Step-by-Step Guide to Getting Your AI Models Through FDA Approval
2
Introducing: Upgraded Project Analytics
3
18 Best Image Annotation Tools for Computer Vision [Updated 2025]
4
Top 8 Use Cases of Computer Vision in Manufacturing
5
YOLO Object Detection Explained: Evolution, Algorithm, and Applications
6
Active Learning in Machine Learning: Guide & Strategies [2025]
7
Training, Validation, Test Split for Machine Learning Datasets
Explore our...
What is Speaker Diarization?
Imagine you are listening to the recording of an important team meeting that you missed. The conversation flows naturally and different voices chime in, ideas bounce back and forth, questions are asked and answered. But as the minutes tick by you find yourself frustrated and you may question yourself, “Who’s talking right now? Was that John’s suggestion or James’s?” “Wait, was it the client or the product manager who raised that concern?” Without knowing who said what, it’s just a sea of words. Now imagine if the recording could automatically tell you: Suddenly, the conversation has structure, clarity, and meaning. That is the speaker diarization, a technology that teaches machines to separate and label voices in an audio stream, just like your brain does in real life. Speaker diarization is the process of partitioning an audio stream into homogeneous segments according to the identity of the speaker. In simple terms we can say that it answers the question, "Who spoke when?" Speaker diarization (Source) This technology is important for analyzing multi-speaker audio recordings, such as meetings, phone calls, interviews, podcasts, and even surveillance audio. Speaker diarization involves the segmentation and clustering of an audio signal into distinct parts where each part is associated with a unique speaker. It does not require prior knowledge about the number of speakers or their identities. The typical output of a speaker diarization system looks like following, It essentially adds structure to unstructured audio, providing metadata that can be used for further analysis, indexing, or transcription. Why Speaker Diarization Matters in Audio and AI In our increasingly audio-driven world, (i.e. from smart assistants and call centers to podcasts and meetings) it is not enough for machines to just hear what is being said. They need to understand who is speaking. Speaker diarization adds this critical layer of intelligence to audio to make it easier to understand, organize, and work with audio in smart ways across numerous real-world applications. Following are some important points why it matters. Enhances Speech Recognition: In Automatic Speech Recognition (ASR), speaker diarization improves transcription accuracy by associating text with individual speakers. This makes the transcript more readable and contextually meaningful, especially in overlapping conversations. Boosts Conversational AI Systems: Conversational AI (like virtual assistants or call center bots) get benefits from diarization by better understanding user intent in multi-speaker conversations. It helps systems differentiate between users and agents and respond more appropriately. Critical in Meeting Summarization: Speaker diarization is essential for generating intelligent meeting notes. It enables systems to group speech by speaker which is important for action-item tracking, sentiment analysis, and speaker-specific summaries. Privacy and Security: Speaker diarization helps isolate speakers for identity verification, anomaly detection, or behavior analysis without always needing to know who the speaker is in surveillance and legal audio analysis. Content Indexing and Search: Speaker diarization enables better indexing and retrieval of audio content for media houses, podcasts, and broadcasting companies. Users can search based on speaker turns or speaker-specific quotes. Speaker Identification vs Diarization Although both speaker identification and speaker diarization deal with analyzing who is speaking in an audio clip. However, both solve different problems and are used in different scenarios. Let’s understand the difference between the two. What is Speaker Identification? In speaker identification, the voice of a person in an audio is recognized and real identity is assigned to it. In other words, it answers the question “Who is speaking right now?”. Speaker Identification (Source) Speaker identification is a supervised task in which a pre-enrolled list of known speakers with voice samples is required. The system matches the speaker’s voice against the list and identifies them. Speaker identification systems typically work by extracting voice features and comparing them to stored voice profiles. The system knows the possible speakers ahead of time. For example, imagine a voice-controlled security system at home. When a user says, “Unlock the door,” the system not only recognizes the command but also checks who said it. If it matches the voice to an authorized user, the door unlocks. Here, the system is identifying the user's voice by comparing it to known voices it has in its database. What is Speaker Diarization? In Speaker Diarization, different voices in an audio are separated and labelled without necessarily knowing who the speakers are. It answers the question “Who spoke when?”. Speaker Diarization (Source) Speaker diarization is an unsupervised task which does not need prior enrollment of speaker speaker data. It simply separates the audio into segments and assigns labels like "Speaker 1", "Speaker 2", etc. Therefore, the system doesn’t know who the speakers are. For example, suppose you have a recording of a team meeting for which you want to create a transcript. You do not care about matching voices to specific names, you just want to understand the flow of conversation and know when one speaker stopped and another started. So the system outputs: You can now read the transcript with clear speaker turns, even if you don’t know who the actual speakers are. Speaker identification is used when there is a need to verify or recognize who is speaking, such as in voice-based login systems, forensic voice matching, or personalizing voice assistants etc. Speaker Diarization on the other hand is used when there is a need to analyze conversations with multiple people, such as transcribing meetings, analyzing group discussions or organizing podcast interviews etc. In real-world applications, these two techniques are often used together. For example, in a customer service call, speaker diarization can first separate the customer and agent voices. Then, speaker identification can confirm which agent handled the call, allowing for quality review and personalization. Applications of Speaker Diarization Speaker diarization plays an important role in audio understanding by breaking down conversations into “who spoke when”. even when the speakers are not known in advance. Following are key applications of speaker diarization in real-world use cases. Meeting Transcription and Summarization In corporate settings, meetings often involve multiple people contributing ideas, sharing updates, and making decisions. Speaker diarization helps separate speaker voices, making transcriptions clearer and summaries more meaningful. For example, a team uses a meeting transcription tool like Otter.ai or Microsoft Teams that applies speaker diarization to tag each speaker’s contribution. This allows team members to see who said what. It automatically generates action items per speaker which provides easy review of discussions for absent participants. Otter.ai transcription (Source) Call Center Analytics Customer service calls often involve two speakers i.e. the agent and the customer. Speaker diarization helps monitor conversations, measuring things like agent performance, customer satisfaction, and detecting service issues by separating who is talking. For example, in a customer service center of a telecom company the recordings of support calls are diarized. The system analyzes if the agent followed the troubleshooting script, if the customer sounded frustrated (detected through emotional analysis on customer segments), and how much time the agent vs. customer spoke. This helps improve customer service quality. Observe.AI uses diarization in customer-agent calls to measure agent speaking time, detect interruptions, track emotional tone per speaker, and improve coaching for call center agents based on how well they interact with customers. Observe AI speaker diarization (Source) Broadcast Media Processing News broadcasts, interviews, and talk shows involve multiple speakers. Diarization is used to automatically label and separate speech segments for archiving, searching, subtitling, or content moderation. For example, during a TV political debate, speaker diarization automatically segments speech between Candidate A, Candidate B and Moderator. Later, when a journalist searches for "closing statement by Candidate A" the system quickly retrieves it because it knows who spoke when. Veritone Media applies speaker diarization on radio talk shows and TV interviews to archive and search by speaker. Podcast and Audiobook Indexing Podcasts and audiobooks often feature multiple hosts, guests, or characters. Speaker diarization helps in indexing content by speaker. This makes it easy to search and navigate long audio recordings for required information. For example, a podcast episode features three hosts discussing technology. Speaker diarization allows listeners to jump directly to Host 2's thoughts on AI, and view a timeline showing when each speaker talks. This makes podcasts more interactive and searchable, like chapters in a book. Descript applies speaker diarization to podcasts so that users can edit episodes easily such as remove filler words or edit a specific guest’s section without disturbing the flow. Courtroom Proceedings and Legal Documentation In legal settings, accurate attribution of who spoke is critical. Speaker diarization enables transcripts to properly record testimony, objections, and judicial rulings without manual effort. For example, during a court trial, speaker diarization can help distinguish between instructions by a Judge, arguments by a defense attorney, and testimonies by a witness. It produces a clean transcript necessary for official legal records and appeals, ensuring fairness and accountability. Verbit specializes in legal transcription. It uses speaker diarization to separate attorneys, judges, witnesses in court recordings automatically, helping produce official court transcripts with clear speaker attribution. Health and Therapy Session Monitoring In mental health counseling and therapy, speaker diarization can help therapists analyze sessions, track patient participation, and even assess changes in patient speech patterns over time. For example, a psychologist records therapy sessions with consent. Speaker diarization can show that the patient spoke 60% of the time answering open-ended questions by the therapist. Over months, analysis reveals the patient started speaking longer and more confidently which is a sign of progress. Eleos Health records therapy sessions (with client consent) and diarizes who is speaking, therapist or client. It analyses engagement metrics like speaking ratios, pauses, emotional markers, helping therapists understand client progress over time. Eleos Health records therapy sessions (Source) Speaker diarization can be used in many other applications across various domains. It has become a critical enabler for making audio and voice-driven systems more intelligent, personal, and practical. From automating meeting notes and customer service analytics to powering smarter healthcare systems and legal services, speaker diarization plays a foundational role wherever "who is speaking" matters. Criteria to evaluate Speaker Diarization Once the speaker diarization system is built, it should be evaluated how well it performs to get the best speaker diarization. When evaluating speaker diarization, you are basically checking how accurately the system splits and labels speech into different speakers over time. There are three popular metrics to evaluate the speaker diarization. Diarization Error Rate (DER) The Diarization Error Rate (DER) is the traditional and most widely used metric for evaluating the performance of speaker diarization systems. DER measures the proportion of the total recording time that is incorrectly labeled by the system. It is computed as the sum of three different types of errors, False alarms (FA) - speech detected when none exists) Missed speech - speech present but not detected Speaker confusion - speech correctly detected but attributed to the wrong speaker The formula for DER is: To ensure fair speaker label matching between the system output and the ground truth, the Hungarian algorithm is used to find the best one-to-one mapping between hypothesis speakers and reference speakers. Additionally, the evaluation allows for a 0.25-second "no-score collar" around reference segment boundaries to account for annotation inconsistencies and timing errors by human annotators. This collar means that slight boundary mismatches are not penalized. While DER is widely accepted, it has some limitations. DER can exceed 100% if the system makes severe errors, and dominant speakers may disproportionately affect the score. Therefore, while DER is highly correlated with overall system performance, it sometimes fails to reflect fairness across all speakers. Jaccard Error Rate (JER) The Jaccard Error Rate (JER) was proposed in the DIHARD II evaluation, to overcome some of the shortcomings of DER. JER aims to equalize the contribution of each speaker to the overall error, treating all speakers fairly regardless of how much they talk. Instead of calculating a global error over all time segments, JER first calculates per-speaker error rates and then averages them across the number of reference speakers. For each speaker, JER is computed by summing the speaker’s false alarm and missed speech errors, and dividing by the total speaking time of that speaker. It is mathematically expressed by: Where N is the number of reference speakers. Importantly, speaker confusion errors that appear in DER are reflected in the false alarm component in JER calculation. Unlike DER, JER is bounded between 0% and 100%, making it more interpretable. However, if a subset of speakers dominates the conversation, JER gives higher error rates than DER. JER provides a balanced and speaker-centric evaluation method that complements DER. Word-Level Diarization Error Rate (WDER) WDER is a metric designed to evaluate the performance of systems that jointly perform Automatic Speech Recognition (ASR) and Speaker Diarization (SD). Unlike traditional metrics that assess errors based on time segments, WDER focuses on the accuracy of speaker labels assigned to each word in the transcript. This word-level evaluation is particularly relevant for applications where both the content of speech and the identity of the speaker are crucial, such as in medical consultations or legal proceedings. Where SIS (Substitutions with Incorrect Speaker tokens): The number of words where the ASR system incorrectly transcribed the word and assigned it to the wrong speaker. CIS (Correct words with Incorrect Speaker tokens): The number of words correctly transcribed by the ASR system but assigned to the wrong speaker. S (Substitutions): The total number of words where the ASR system incorrectly transcribed the word. C (Correct words): The total number of words correctly transcribed by the ASR system. This metric specifically evaluates the accuracy of speaker assignments for words that were either correctly or incorrectly recognized by the ASR system. However, it does not account for insertions or deletions, as these errors do not have corresponding reference words to compare against. Therefore, WDER should be considered alongside the traditional Word Error Rate (WER) to obtain a comprehensive understanding of system performance. How Encord is Used for Speaker Diarization Encord is a comprehensive multimodal AI data platform that facilitates efficient management, curation, and annotation of large-scale unstructured datasets, including audio files. Its audio annotation tool is particularly adept at handling complex tasks like speaker diarization, which involves segmenting audio recordings to identify and label individual speakers. Following are the features of Encord in annotating data for speaker diarization. Encord Audio Annotation (Source) Precise Temporal Annotation Encord allows annotators to label audio segments with millisecond-level precision. This is important for accurately marking the start and end times of each speaker's voices. Support for Overlapping Speech In real-world scenarios like meetings or interviews, speakers often talk over each other. Encord platform supports overlapping annotations, enabling annotators to label multiple speakers speaking simultaneously. This feature ensures that models trained on such data can handle crosstalk and interruptions effectively. Layered Annotations Beyond identifying who spoke when, Encord allows for layered annotations, where additional information such as speaker emotion, language, or background noise can be tagged alongside speaker labels. AI-Assisted Pre-Labeling Encord integrates with state-of-the-art models like OpenAI's Whisper and Google's AudioLM. These models can generate preliminary transcriptions and speaker labels, which annotators can then review, refine and use thus reducing manual effort. Collaborative Annotation Environment Encord platform is designed for team collaboration that allows multiple annotators and reviewers to work on the same project simultaneously. Features like real-time progress tracking, change logs, and review workflows ensure consistency and quality across large annotation projects. Scalability and Integration Encord supports various audio formats, including WAV, MP3, FLAC, and EAC3, and integrates with cloud storage solutions like AWS, GCP, and Azure. This flexibility allows organizations to scale their annotation efforts efficiently and integrate Encord into their existing data pipelines. Key Takeaways Speaker diarization separates an audio recording into segments based on who is speaking, answering "Who spoke when?" without needing to know their identities. Speaker diarization adds structure to audio, improves transcription accuracy, enhances conversational AI. Speaker identification matches a voice to a known person, while diarization only separates and labels speakers without requiring pre-known identities. Speaker diarization used in meetings, call centers, podcasts, legal transcription, media broadcasting, and healthcare monitoring to organize and analyze conversations. Speaker diarization systems are evaluated using metrics like DER, JER, and WDER Encord helps streamline audio annotation for building speaker diarization models.
May 12 2025
5 M
Audio Segmentation for AI: Techniques and Applications
Imagine your voice assistant flawlessly transcribing every word in a noisy meeting or a security system instantly detecting the sound of a potential threat like a gunshot. Audio segmentation is the crucial element that is turning such ideas into reality, leveraging artificial intelligence (AI) to process different sound types. This technology is driving significant advancements in the audio AI industry, fuelling the demand for several audio AI solutions. For instance, according to MarketsandMarkets, the current global speech and voice recognition market is projected to reach USD 73.49 billion by 2030. The core concept behind audio segmentation is to split audio recordings into distinct, homogeneous segments. It enables AI to interpret between various audio components, such as speech, music, and environmental sounds. While it may sound straightforward in principle, audio segmentation presents several challenges, such as overlapping sounds, poor audio quality, and the need for carefully annotated datasets. In this post, we will explore audio segmentation and its techniques, applications, and challenges. We will also see how tools like Encord can help developers segment audio to build scalable audio AI systems. Audio Segmentation - A Brief Overview Audio segmentation divides an audio signal into contiguous segments for AI to process. It identifies parts of the audio where the sound stays relatively consistent, like speech, music, or silence. Each segment should ideally contain a single type of sound event or acoustic characteristic. For example, in a conversation recording, segmentation can identify speech segments from different speakers, periods of silence, or any background noise present. Audio segmentation relies on several key concepts: Segments: These are the audio units resulting from segmentation, each representing a specific part of the recording. Boundaries: These are the temporal points that mark the start and end of a segment, defining where one acoustic event ends and another begins. Labels/Categories: After identifying a segment, it is usually given a label or category that describes its content. This might include the speaker's name, the nature of the sound event (e.g., "dog bark," "car horn"), or a description of the acoustic environment (e.g., "office," "park"). Boundaries segments Types of Audio Segmentation Audio segmentation categorizes audio signals into distinct types for targeted AI processing. Below are some key types: Speaker Diarization: This type focuses on answering "Who spoke when?". It includes segmenting an audio stream to identify individual speakers and determine the time intervals each person speaks. This is useful in meetings, interviews, and multi-party conversations for indexing and understanding the flow of dialogue. Environmental Sound Event Detection: The goal is to identify and label specific audio events occurring within an audio signal. Examples include detecting the sound of a car horn, a dog barking, or glass breaking. Effective sound event detection depends on algorithms that distinguish these events from general background noise within the audio files. Music Structure Analysis: This includes segmenting a piece of music into its constituent structural elements, including the intro, verse, chorus, bridge, and outro sections. Music information retrieval uses this type of audio segmentation to understand the composition and organization of musical pieces by analyzing patterns in the waveform and other features of the audio data. Speech Segmentation: This type is fundamental to automatic speech recognition (ASR) and aims to divide spoken language into smaller, linguistically meaningful units. These units range from individual phonemes (the smallest sound units) to words or even entire sentences. Acoustic Scene Classification: This type of audio classification focuses on identifying the overall acoustic environment of an audio recording. Algorithms analyze the characteristics of the audio stream to classify the recording as taking place in an office, a park, a restaurant, or another defined acoustic scene. This has important applications in context-aware systems and multimedia analysis. Learn how speech-to-text AI works How Audio Segmentation Works The process of audio segmentation involves several stages. It begins with the pre-processing step, which cleans up the audio signal by reducing noise and normalizing the audio levels. This enhances the quality of the audio data and prepares it for subsequent analysis. Next, feature extraction techniques are applied to the preprocessed audio stream. The goal here is to extract relevant information from the waveform that can be used to differentiate between different acoustic events or segments. Acoustic waveform characteristics Common feature extraction methods include Mel-Frequency Cepstral Coefficients (MFCCs), which represent the short-term power spectrum of sound. Another method is spectrograms, which visually depict the audio signal's frequency content over time. These extracted features are represented as vectors, which are numerical representations of an audio signal. These vectors distill complex audio data into manageable forms that ML algorithms process and analyze effectively. Illustration of spectrograms After feature extraction, segmentation methods identify the boundaries between different segments based on some criteria. Audio segmentation methods can be classified into two primary approaches: supervised and unsupervised methods. Below, we’ll explore each approach and the techniques within them. Supervised Methods Supervised methods rely on labeled training data, where each segment is annotated with its class or boundary information. These methods use this data to train algorithms and to predict segment boundaries in new audio streams. While effective, they require significant resources to create large, annotated datasets. Within supervised learning, several techniques are used: ML-Based Techniques: Hidden Markov Models (HMMs): These model the statistical properties of audio sequences, learning transitions between segments. They’re widely used in tasks like speaker diarization. Gaussian Mixture Models (GMMs): These treat observed data as a mix of Gaussian distributions, each representing a cluster in feature space, aiding in segment classification. Deep Learning Approaches: Convolutional Neural Networks (CNNs): These analyze spectrograms for pattern recognition, excelling in tasks like acoustic event detection. Recurrent Neural Networks (RNNs): Including Long Short-Term Memory (LSTM) units, RNNs capture temporal dependencies in audio signals. For example, a study at the IEEE International Conference on Acoustics, Speech, and Signal Processing (ICASSP) demonstrated Bidirectional LSTMs with Attention mechanisms effectively segmenting heart sounds. Advanced Deep Learning Methods: Mamba-Based Segmentation Models: The Mamba architecture space model with attention-like capabilities processes long audio sequences with reduced memory requirements. This makes it suitable for identifying speaker turns in extended recordings. You Only Hear Once (YOHO) Algorithm: YOHO treats audio segmentation as a regression problem, predicting the presence and boundaries of audio classes directly. This approach improves speed and accuracy over traditional frame-based classification methods. Audio Spectrogram Transformer (AST): AST applies transformer models to audio spectrograms for classification tasks. Due to their self-attention mechanisms, ASTs are computationally intensive. Audio spectrogram transformer (AST) architecture Unsupervised Methods Unsupervised methods don’t use labeled data. Instead, they identify segment boundaries by detecting patterns or changes in the audio signal, often through clustering or similarity analysis. While they’re valuable when labeled data is unavailable, they may lack the precision of supervised methods due to the absence of training guidance. Common techniques include: Threshold-Based Segmentation: This compares feature values against predefined thresholds or metrics (e.g., similarity between adjacent windows) to detect changes, with local maxima indicating segment boundaries. Clustering Algorithms: Methods like K-means or hierarchical clustering group similar audio frames based on feature similarity, revealing natural transitions. These are often applied in music structure analysis or environmental sound detection. Applications of Audio Segmentation Across Industries Audio segmentation drives multiple industries by helping analyze and interpret audio data. Its applications cover various sectors, improving functionality and user experience. Speech Technology Audio segmentation helps various speech-based technologies. Transcription services depend on segmenting audio files into smaller units to convert speech to text. Voice assistants use it to isolate and process user commands from background noise. Call centers use audio segmentation for analytics, such as identifying periods of silence, speaker changes, and key phrases within customer interactions. Speaker diarization Security and Surveillance In security systems, audio segmentation helps detect specific sound events that may indicate anomalies or threats. For instance, algorithms can be trained to identify the distinct waveform of a gunshot or the sound of breaking glass within an audio recording, triggering alerts for real-time response. Media and Entertainment Audio segmentation benefits the media and entertainment industry significantly. It powers automated music information retrieval systems that can analyze and categorize vast music libraries based on their structure, identifying intros and choruses. Similarly, sound event detection through segmentation methods allows for efficient indexing and retrieval of specific sound effects in multimedia content. Healthcare Healthcare professionals are using audio segmentation for various analytical purposes. They can identify patterns indicative of certain medical conditions by segmenting patient vocalizations. Another growing application is monitoring respiratory sounds, such as coughs or wheezes, through audio stream analysis. Education Educational platforms can use audio segmentation capabilities to enhance learning experiences. Analyzing student participation in online discussions by segmenting individual contributions can provide insights into engagement levels. Furthermore, automated feedback on pronunciation can be facilitated by segmenting spoken words into phonemes and comparing them against a reference, often in conjunction with ASR technology. Challenges in Audio Segmentation Audio segmentation faces several challenges that impact its accuracy and effectiveness: Overlapping Sounds: In real-world environments, multiple audio sources can overlap, making it difficult to distinguish individual sound events. For example, sounds such as doorbells, alarms, and conversations can overlap in a home setting, complicating the segmentation process. Variability in Audio Quality: Differences in recording devices, environments, and conditions lead to inconsistencies in audio quality. Factors such as background noise, echo, and distortion can degrade the performance of segmentation algorithms, especially those relying on subtle audio features. Need for High-Quality Annotated Datasets: Training effective audio segmentation models requires large datasets with precise annotations. However, creating these datasets is labor-intensive and time-consuming. The lack of standardized, high-quality annotated data hampers the development and evaluation of robust segmentation systems. How Encord is Used for Audio Segmentation An advanced annotation tool like Encord can help overcome the challenges mentioned above. Encord is a data curation, annotation and evaluation platform for AI. It’s audio annotation feature segments audio files for speaker recognition and sound event detection applications. Its capabilities enable the precise classification of audio attributes and accurate temporal annotations. Comprehensive Audio File Format Support The platform supports various audio formats, including .mp3, .wav, .flac, and .eac3, allowing seamless integration with existing data workflows. You can upload audio files through Encord's interface or SDK, connecting to cloud storage solutions like AWS, GCP, Azure, or OTC for efficient data management. Precision Labeling and Layered Annotations Encord's label editor supports detailed classification with millisecond-level precision, allowing annotators to accurately label sound events, emotional tone in speech, languages, and speaker identities. Its ability to handle layered and overlapping annotations is particularly effective for applications involving complex audio streams, such as audio classification tasks where multiple events may co-occur. This functionality supports advanced use cases in multimedia indexing, sound event detection, and speech segmentation. Temporal Classification for AI Training Another key feature is temporal classifications, which allow annotators to label specific time segments corresponding to individual speakers or sound events. This helps enhance AI training and model optimization in applications like transcription services, virtual assistants, and security systems. AI-Assisted Annotation for Efficiency Encord also offers AI-assisted annotation tools that automate parts of the labeling process, increasing efficiency and accuracy. These tools can pre-label audio data, identifying spoken words, pauses, and speaker identities, thereby reducing manual effort. Foundational models, such as OpenAI’s Whisper and Google’s AudioLM, can achieve breakthrough performance in several actions to accelerate audio curation and annotation workflows. Label complex audio using Flixble Ontologies AI teams can use Encord Agents to integrate with new models as well as their own, orchestrating automated audio transcription, pre-labeling, and quality control to significantly enhance the efficiency and quality of their audio data pipelines. Collaborative Annotation and Quality Control Integrated collaboration tools within Encord facilitate team-based projects by providing features like real-time progress tracking, change logs, and review workflows. This ensures teams can work together effectively, maintaining high-quality annotations across complex audio datasets. Audio Applications of Encord Encord's platform provides a robust environment for annotating audio data, directly supporting the development and enhancement of various audio-centric AI applications. Development of Voice Assistants and Chatbots Encord can help create high-performing voice assistants and chatbots by enabling the accurate annotation of speech audio data. Its precise temporal labeling features help label spoken words and phrases, crucial for training automatic speech recognition (ASR) models. Encord helps build more context-aware and personalized conversational AI agents by enabling detailed annotation of who is speaking and when (speaker diarization). Furthermore, the ability to annotate various audio attributes helps developers train models that can understand not just the content of the speech but also its nuances and characteristics. Speaker Annotation Enhancing Emotion Recognition Systems Encord can significantly improve the accuracy of emotion recognition systems. This detailed annotation of sentiment and emotion in audio files provides the high-quality training set required for deep learning models. These models can accurately identify and classify a wide range of emotions from audio data. Handling overlapping annotations is particularly valuable in emotion recognition, where multiple emotions or intensities might be present simultaneously in an audio stream. Sentiment or Emotion Annotation Want to accurately curate and label audio data? Explore Encord's Audio modality. Key Takeaways Audio segmentation transforms industries through AI to process audio signals accurately. It powers transcription, security, and healthcare with precise segment labeling. Best Use Cases for Audio Segmentation: It excels in speaker recognition for voice assistants, sound event detection for surveillance, and emotion analysis in call centers. Challenges in Audio Segmentation: Overlapping sounds, poor audio quality, and dataset annotation demands hinder performance. Encord for Audio Segmentation: Encord’s tools enhance audio data quality with AI-assisted annotation and temporal precision. It streamlines datasets for deep learning, ensuring scalable, high-performing audio AI systems.
May 06 2025
5 M
Webinar Recap: Building Physical AI
AI systems are increasingly moving beyond text and images into the physical world like robots, autonomous vehicles, and embodied agents. This makes the need for robust multimodal reasoning at scale critical. Last week’s webinar, Building Physical AI: How to Enable Multimodal Reasoning at Scale, brought together experts from Encord and Archetype AI. The session explored what it really takes to build AI systems that can perceive, reason, and act in complex real-world environments. Here’s a recap, in case you missed it. What is Physical AI? Physical AI refers to AI systems that can operate in and reason about the physical world. These systems not only process images or pre-processed text, they also interpret sensory inputs from multiple sources like video, audio, and LiDAR. This combination of AI with physical systems makes decisions in real time in order to navigate real environments. For more information, read the blog What is Physical AI At the core of Physical AI is the ability to combine visual, auditory, spatial, and temporal data to understand what’s happening and what actions should follow. That is multimodal reasoning. Multimodal reasoning requires more than just robust AI models. It needs new data infrastructure, scalable annotation workflows and evaluation pipelines that mimic real world environments, not just benchmark accuracy. Why It’s Hard to Build Physical AI Systems Building AI systems deployed in the real world adds a new layer of complexity. Here are few gaps: High-dimensional, unstructured input: You’re not dealing with curated datasets anymore. You’re working with noisy sensor streams, long video sequences, and time-synced data from multiple sources. No clear ground truth: Especially for downstream tasks like navigation, the “correct” label is often contextual, ambiguous, or learned through feedback. Fragmented workflows: Most annotation tools, model training frameworks, and evaluation platforms aren’t built to handle multimodal input in a unified way. Key Takeaways from the Webinar Encord provides tools built for multimodal annotation and model development. Our latest webinar “Building Physical AI: Enabling Multimodal Reasoning at Scale”, was in collaboration with Archetype AI, “a physical AI company helping humanity make sense of the world”. They have developed Newton, an AI model that can understand the physical world using multimodal sensor data. In this webinar, we outline key challenges and show how to build Physical AI systems that use rich multimodal context for multi-step reasoning. The aim is to build AI models which are safer and provide reliable real-world performance. Here are the key takeaways for teams scaling multimodal AI. Multimodal Annotation The first challenge in Physical AI is aligning and labeling data across modalities. The annotation pipelines must handle: Video-native interfaces: Unlike static images, video requires temporal awareness. Annotators need to reason about motion, persistence, and cause-effect relationships between frames. Ontologies that reflect the real world: Events, interactions, and object properties need structured representation. For example, knowing that “Person A hands object to Person B” involves spatial and temporal coordination, not just bounding boxes. Multifile support: A key Encord feature highlighted was the ability to annotate and synchronize multiple data streams such as RGB video, depth maps, and sensor logs within a single interface. This enables richer context without switching tools. Scalable Automated Labeling Once a strong ontology is in place, it becomes possible to automate large parts of the annotation process. The Encord team outlined a two-step loop: Use ontologies for consistent labeling: The structure enforces what can be labeled, how relationships are defined, and what types of attributes are expected. This reduces ambiguity and improves inter-annotator agreement. Add model-in-the-loop tools: After initial manual labeling, models can be trained to pre-label data in the future as well. This cuts the annotation time dramatically. Annotators then shift to verification and correction, speeding up throughput. This hybrid approach balances the need for quality via human input with the need for scale via automation. It’s particularly useful in domains with long-tail edge cases like industrial robotics or medical imaging, where manual annotation alone doesn’t scale. Agentic Workflows Traditional ML workflows are often rigid. They collect data, train a model, evaluate, repeat. But the real-world environment is dynamic. In the webinar, the speakers introduce the idea of agentic workflows. The pipelines are modular that can: React to new data in real time Orchestrate multiple model components Include humans in the loop during key stages Be reused across tasks, domains, or hardware setups Encord’s agentic workflow builder is designed for this kind of modularity. It lets teams compose workflows using building blocks like models, data sources, annotation tools, evaluation criteria and run them in structured loops. This helps AI systems that can not only perceive but also act to evaluate their own performance. They can also trigger re-labeling or retraining when needed. Evaluation Metrics Most existing ML benchmarks fall short when applied to Physical AI. Accuracy, mAP, and F1 scores don’t always correlate with real-world performance especially when the task is “did the robot successfully hand over the object?” or “did the system respond to the sound cue correctly?” “Evaluation is no longer just a number. It’s whether your robot does what it’s supposed to do.” - Frederik H. What’s needed instead are: Behavioral benchmarks: To measure whether the system can accomplish end-to-end tasks in physical environments. Continuous evaluation: Instead of one-time test sets, build systems that constantly monitor themselves and flag drift or errors. Task-aware success criteria: For example, a model that misses 3% of bounding boxes might still succeed in object tracking but fail miserably in manipulation if it loses track of a key object for just a moment. The teams building physical AI systems should think beyond classic metrics and focus on holistic evaluation that spans perception, reasoning, and action. The webinar also included a product walkthrough showing how Encord supports: Video-native annotation tools with time-based tracking, object permanence, and multimodal synchronization Multifile annotation interfaces where audio, LiDAR, or sensor data can be aligned with video Reusable workflows that integrate models into the annotation process and kick off retraining pipelines Agentic workflows for tasks like active learning, error correction, and feedback loops For teams used to stitching together open-source tools, spreadsheets, and Python scripts, this kind of unified, GUI-driven interface could dramatically simplify experimentation and iteration. What This Means for Teams Building Physical AI If you’re building AI systems for the physical world whether that’s drones, manufacturing bots, self-driving cars, or AR agents, here’s what you should take away: Start with a strong data ontology that reflects the semantics of your task Choose annotation tools that support multimodal data natively and avoid fragmented workflows Use model-in-the-loop setups to scale annotation cost-effectively Design workflows that are modular, composable, and agent-driven Define success using task-based or behavioral metrics, not just classification scores Physical AI isn’t just a new application domain. It’s a fundamental shift in how we collect, train, and evaluate AI systems. Want to go deeper? You can watch the full webinar. Building Physical AI: How to Enable Multimodal Reasoning at Scale Conclusion Physical AI represents the next frontier in machine learning, where models aren’t just answering questions, but interacting with the world. But to reach that future, we need more than smarter models. We need better data workflows, richer annotations, and tools that can keep up with the complexity of real-world signals. The teams that win in this space won’t just be good at training models. They’ll be the ones who know how to structure, label, and scale the messy multimodal data that Physical AI depends on.
Apr 30 2025
5 M
Encord at ICLR 2025
Apr 26 2025
5 M
Meet Alex - Account Executive at Encord
Another day, another episode of "Behind the Enc-urtain", where we go behind the scenes with the Encord team and learn more about their life and work! Today we sit down with Alex Winstone, Account Executive here at Encord. As one of our first AEs, Alex has worn many hats in these first 6 months — he's brought onboard some of the leading AI research labs, F500 organizations and fast growing scale-ups, run industry roundtables in 5+ countries, helped onboard 4 new members of the UK Sales team... and somehow also managed to almost never miss an Encord Thursday bar! PS. We are hiring! We are looking for AEs to join our London and San Francisco teams - you can find more about the London AE role here and SF AE role here. Let's start with a quick introduction — can you share a bit about your background and how you found your way to Encord? I joined Encord after spending 4 years at an AI scale-up, joining as the first Sales hire and seeing their growth from seed to a $45M Series A and beyond. It was an incredible journey. As I was thinking about what was next for me, I had some key criteria in mind. I was firstly looking for a great sales team I could learn from and develop with. From the outset, I was impressed by Leo and the team, and was certain this was an environment within which I could continue developing. Having been first on the ground previously, I wanted to ensure I could take ownership and have a tangible impact on the company's outcomes. Secondly, I wanted to find a deeply interesting problem-area with huge growth potential. I was looking for a company with product market fit (or strong signs of it). This was potentially the hardest criteria to fulfil, as often companies where you can have meaningful impact are yet to achieve PMF. I was convinced through my conversations that Encord was delivering true value to their clients and have now seen this first-hand in these first 6 months! What does a 'day in the life' of Alex look like? Each day is quite different! Around two thirds of my day is usually spent running demos, presentations and 1:1 meetings with companies who are exploring Encord. I get to work with firms at the cutting-edge of AI, consultatively showcasing the Encord platform and working with the Solutions Engineering team to solve their pains around data curation, annotation, RLHF and model evaluation. Internally, I also work very closely with our Commercial Associate team — who identify companies where Encord can really move the needle and solve MLOps bottlenecks — and the Product team, sharing feedback and ideas from customers, and seeing them turn into reality. What kinds of companies or personas make the right Encord buyers? We typically work with ML and AI teams — from engineers and data scientists, to CTOs and Heads of AI. Encord is industry-agnostic, so I might work with healthcare or logistics companies in the morning, and sports analytics and robotics teams in the afternoon! We also work with organizations of various sizes, from large Fortune 500 orgs to big tech companies and fast-growing scale-ups. No two conversations are ever identical, but it's interesting to see so many similar pain points. What advice would you give to someone who wants to join Encord as our next AE? Reach out to me on LinkedIn (..we have a referral bonus! 😉) Jokes aside, I'd say be prepared to get stuck in, learn quickly, and be a team-player. I'd join as many calls as you can in your early weeks and really absorb everything. If you’re at a point in your career where you are looking for a sales team to grow in, a fast-paced environment and strong signs of PMF, I'd wholeheartedly recommend Encord. And now for a rapid fire round... What 3 words would you use to describe the Encord culture? Focused, collaborative & transparent. Which fictional character would make the best Encord hire and why? Mystery Incorporated (Scooby-Doo and the gang). You’ll always be getting to the bottom of mysteries (bottlenecks in MLOps and data pipelines) and it’s a dog friendly office. What is one thing you found surprising or different about Encord when you joined? How customer-focused the team is. Every idea or bit of feedback that could improve a client's 'life' is meaningfully fed back to the product and engineering team, where it's considered, discussed and often implemented. It’s also great to see the founders regularly share their vision for the platform (as well as historic views) and see how these materialize in real time. You can find Alex on Linkedin here. See you at the next episode of “Behind the Enc-urtain” 👋
Apr 14 2025
5 M
What is a Digital Twin? Definition, Types & Examples
Imagine a busy factory, where all the machines are running and sensors are tracking every detail of how they run. The key technology of this factory is a digital twin, a virtual copy of the whole factory. Meet Alex, the plant manager, who starts his day by checking the digital twin of the factory on his tablet. In this virtual model, every conveyor belt, robotic arm, and assembly station is shown in real time. This digital replica is not just a static image. It is a dynamic, live model that replicates exactly what is happening within the factory. Earlier in the week, a small vibration anomaly was detected on one of the robotic arms. In the digital twin, Alex saw the warning signals and quickly zoomed in on the problem area. By comparing the current data with historical trends stored in the model, the system predicted that the robotic arm might experience a minor malfunction in the next few days if not serviced. Alex then called a meeting with the maintenance team using the insights from the digital twin. The team planned a repair to ensure minimal disruption to production. The digital twin not only helped predict the issue but also allowed the team to simulate different repair scenarios and choose the most efficient one without stopping the production line. As production increases, the digital twin continues to act as a silent guardian monitoring energy use, optimizing machine settings, and suggesting improvements to reduce waste. It is like having a virtual copy of the factory in the cloud that constantly learns and adapts to make the physical world more efficient. Digital Twin in Factory (Source) What is a Digital Twin? A Digital Twin is a virtual representation of a physical object, system, or process that reflects its real-world version in real-time or near-real-time. It uses data from sensors, IoT devices, or other sources to simulate, monitor, and analyze the behavior, performance, or condition of the physical entity. This concept is widely used in industries like manufacturing, healthcare, urban planning, and more to help improve decision-making, predictive maintenance, and optimization. Digital twin fundamental technologies (Source) A Digital Twin is a dynamic, digital copy that grows and changes along with its physical counterpart. It combines data (whether from the past, in real-time, or predictive) with advanced technologies like AI, machine learning, and simulation tools. This allows it to provide insights, predict outcomes, or test scenarios without the need to directly interact with the physical object or system. A Digital Twin arrangement in automotive industry (Source) Types of Digital Twins Digital twins can be categorized into different types based on the scope, complexity of what they represent and application it can perform. Here are four primary types. Component Twins Component twins are digital replicas of individual parts or components of a larger system. They focus on the specific characteristics and performance metrics of a single element. For example, imagine a jet engine where each turbine blade is modeled as a component twin. By tracking stress, temperature, and wear in real time, engineers can predict when a blade might fail and schedule maintenance before a critical issue occurs. Asset Twins Asset twins represent entire machines or physical assets. They integrate data from multiple components to provide a collective view of an asset's performance, condition, and operational history. Consider an industrial robot on a production line. Its digital twin includes data from all its moving parts, sensors, and control systems. This asset twin helps the maintenance team monitor the robot’s overall health, optimize its performance, and schedule repairs to avoid downtime. System Twins System twins extend beyond individual assets to represent a collection of machines or subsystems that interact with one another. They are used to analyze complex interactions and optimize performance at a broader scale. In a smart factory, a system twin might represent the entire production line. It integrates data from various machines, such as conveyors, robots, and quality control systems. This comprehensive model enables managers to optimize workflow, balance loads, and reduce bottlenecks throughout the entire manufacturing process. Process Twins Process twins model entire workflows or operational processes. They capture not just physical assets but also the sequence of operations, decision points, and external variables affecting the process. A supply chain process twin could represent the journey of a product from raw material sourcing to final delivery. By simulating logistics, inventory levels, and transportation routes, businesses can identify potential disruptions, optimize delivery schedules, and enhance overall supply chain efficiency. Levels of Digital Twins Digital twins evolve over time as they incorporate more data, analysis, and autonomous capabilities. Here are the 5 Levels of Digital Twins. Descriptive Digital Twin A descriptive digital twin is a basic digital replica that mirrors the current state of a physical asset. It represents real-time data and static properties without much analysis. The example of a descriptive digital twin is a digital model of a hospital MRI machine that displays its operating status, temperature, and usage statistics. It shows the current condition but does not analyze trends or predict future issues. Diagnostic Digital Twin This level enhances the descriptive twin by adding diagnostic capabilities. It analyzes data to identify deviations, errors, or early signs of malfunction. For example, consider the same MRI machine that now includes sensors and analytics that detect if its cooling system is underperforming. Alerts are generated when operating parameters deviate from normal ranges to enable identification of the issue early. Predictive Digital Twin At this stage, the digital twin uses historical and real-time data to forecast future conditions. Predictive analytics help anticipate failures or performance drops before they occur. For a surgical robot, the predictive digital twin analyzes past performance data to predict when a component might fail. This allows maintenance to be scheduled proactively which reduces the risk of unexpected downtime during critical operations. Prescriptive Digital Twin It is a more advanced twin that goes beyond prediction to recommend specific actions or solutions, often with “what-if” scenario testing. It combines predictive insights with recommendations or automated adjustments. A digital twin of a hospital’s intensive care unit (ICU) monitors various devices and patient parameters. If the twin predicts a rise in patient load, it might suggest reallocating resources or adjusting ventilator settings to optimize care which ensures the unit runs smoothly during peak times. Autonomous Digital Twin It is the most advanced level of digital twins. An autonomous digital twin not only predicts and prescribes actions but can execute them automatically in real time. It uses AI and machine learning to adapt continuously without human intervention. For example, in a fully automated pharmacy system this digital twin monitors medication dispensing, inventory levels, and patient prescriptions. When it detects discrepancies or low stock, it autonomously reorders supplies and adjusts dispensing algorithms to ensure optimal service without waiting for manual input. Do Digital Twins Use AI? Digital twins often integrate AI to transform raw data into actionable insights, optimize performance, and automate operations. The following points describe how AI enhances digital twin models: Predictive Insights AI algorithms analyze historical and real-time data gathered by the digital twin to identify patterns and trends. For example, machine learning models can predict when a critical component in a manufacturing line might fail which enables maintenance to be scheduled proactively. By continuously monitoring performance metrics, AI can detect anomalies before they rise into major issues. This early detection helps prevent costly downtime and improves overall reliability. Advanced Analytics AI can analyze huge amounts of data from sensors to find hidden patterns and insights that traditional methods might miss. This deep analysis helps create more accurate models of how physical systems work. Advanced algorithms can also simulate different operating situations to let decision-makers test possible changes in a virtual setting. This is especially useful for improving system performance without causing real-world problems. Automation Using AI, digital twins can not only suggest corrective actions but also execute them automatically. For example, if a digital twin identifies that a machine is overheating, it might automatically adjust operating parameters or shut the machine down to prevent damage. AI models embedded within digital twins continuously learn from new data. This adaptability means that the system improves its predictive and diagnostic accuracy over time and becomes more effective in managing complex operations. Imagine a virtual copy of a factory production line. AI tools built into this virtual copy keep an eye on how well the machines are working. If the AI notices a small sign that an important part is wearing out, it can predict that the part might fail soon. The system then changes the workflow to reduce any problems, plans a maintenance check, and gives the repair team detailed information about what’s wrong. By using digital twin technology with AI, industries can move from reactive to proactive management and transform how they maintain systems, predict issues, and optimize operations. Digital Twins Examples Digital twins have many use cases in different domains. Let’s discuss some example of digital twins. Digital Twin in Spinal Surgery A digital twin in spinal surgery is a detailed virtual replica of a real surgical operation. It captures both the static setup (like the operating room and patient anatomy) and the dynamic actions (like the surgeon’s movements and tool tracking) in one coherent 3D model. A digital twin is a virtual simulation that mirrors an actual surgery, created by merging data from various sensors and imaging methods. Digital photograph of a spinal surgery (left) and rendering of its digital twin (right) (Source) Following are the main components of this digital twin system. Reference Frame: A high-precision 3D map of the operating room is built using multiple laser scans. Markers are placed in the room to fuse these scans into one common coordinate system. Static Models: The operating room, equipment, and patient anatomy are modeled using photogrammetry (detailed photos) and 3D modeling software. This produces realistic textures and accurate dimensions. Dynamic Elements: Multiple ceiling-mounted RGB-D cameras capture the surgeon’s movements. An infrared stereo camera tracks the surgical instruments with marker-based tracking. Data Fusion and Integration: All captured data is registered into the same reference frame, ensuring that every element—from the static room to dynamic tools, is accurately aligned. The system is built in a modular and explicit manner, where each component is separate yet integrated. Use of AI: AI techniques enhance dynamic pose estimation (e.g., using models like SMPL-H) and help in processing the sensor data. The detailed digital twin data also provides a rich source for training machine learning models to improve surgical planning and even automate certain tasks. Comparison of the rendered digital twin with the real camera images (Source) This digital twin can help in the following tasks: Training & Education: Surgeons and students can practice procedures in a risk-free, realistic environment. Surgical Planning: Doctors can simulate and plan complex surgeries ahead of time. Automation & AI: The rich, detailed data can train AI systems to assist with surgical navigation, process optimization, and even automate some tasks. The digital twin for spinal surgery is a comprehensive 3D virtual model that integrates high-precision laser scans, photogrammetry, multiple RGB-D cameras, and marker-based tracking. This system captures the entire surgical scene and aligns them within a common reference frame. AI plays a role in enhancing dynamic data capture and processing, and the detailed model serves as a powerful tool for training, surgical planning, and automation. Digital twin in Autonomous Driving This paper on digital twins in virtual reality describes a digital twin built in a virtual reality setting to study human-vehicle interactions at a crosswalk. The digital twin recreates a real-world crosswalk and an autonomous vehicle using georeferenced maps and the CARLA simulator. Real pedestrians interact with this virtual environment through a VR interface, where an external HMI (GRAIL) on the vehicle provides explicit communication (e.g., changing colors to signal stopping). The system tests different braking profiles (gentle versus aggressive) to observe their impact on pedestrian confidence and crossing behavior. The setup uses questionnaires and sensor-based measurements to collect data, and it hints at leveraging AI for data processing and analysis. Overall, this approach offers a controlled, safe, and realistic way to evaluate and improve communication strategies for autonomous vehicles, potentially enhancing road safety. Following are the components of the system. Digital twin for human-vehicle interaction in autonomous driving. Virtual (left) and real (right) setting (Source) Digital Twin Environment: The virtual crosswalk is digitally recreated using map data to ensure it matches the real-world layout. Experiments run in CARLA, an open-source simulator that creates realistic traffic scenarios. Human-Vehicle Interaction Interface: A colored bar on the vehicle indicates if the car is about to stop or yield. Two braking styles are tested which are gentle (slow deceleration) and aggressive (sudden deceleration). Virtual Reality Setup: Participants use a VR headset and motion capture to see and interact with the virtual world. Their movements are synchronized with the simulation for accurate feedback. Data Collection & Analysis: Participants share their feelings about safety and the vehicle's actions. The system records objective data like distance, speed, and time-to-collision. Role of AI: AI analyzes both subjective feedback and sensor data to model behavior and refine communication. AI helps integrate data so the simulation responds realistically to both the vehicle and pedestrians. This digital twin system helps in following: Enhances Safety: Clear communication through the digital twin helps pedestrians understand vehicle intentions, reducing uncertainty and potential accidents. Improves Training: It offers a realistic simulation for both pedestrians and autonomous vehicles, enabling safer, hands-on training and evaluation. Informs Design: By collecting both subjective feedback and objective measurements, designers can refine vehicle behavior and HMI features for better user interaction. Supports Data-Driven Decisions: The system’s real-time data and AI processing allow for continuous improvements in autonomous driving and pedestrian safety strategies. How Encord Enhances Digital Twin Models Encord, a data management and annotation platform which can be used in digital twin applications. It is used to annotate, curate, and monitor large-scale datasets to train machine learning models for digital twin creation and optimization. Following are the important points how Encord helps in creating and enhancing Digital Twins. Encord provides tools for preparing the data needed to train machine learning models that can power digital twins. Encord allows users to annotate and curate large datasets, ensuring the data is clean, accurate, and suitable for training machine learning models that will be used in digital twin applications. Encord platform enables users to monitor the performance of their machine learning models and datasets, allowing for continuous improvement and optimization of the digital twin. By using high-quality, well-curated datasets, machine learning models can achieve higher accuracy and reliability. Encord platform can accelerate the development of digital twins by streamlining the data preparation and model training process. Digital twins powered by machine learning models can provide valuable insights into the performance of physical systems, enabling better decision-making. Key Takeaways Digital twin technology revolutionizes industrial operations by creating a dynamic virtual replica of physical systems. This technology not only mirrors real-time activities in environments like factories and hospitals but also uses historical data and AI to predict issues, simulate repairs, and optimize processes across various industries. Real-Time Monitoring & Visualization: Digital twins provide live, interactive models that replicate every detail of a physical system that allows to quickly identify anomalies and monitor system performance continuously. Predictive Maintenance: Digital twin helps in analyzing historical and real-time data which can be used to forecast potential equipment failures and enables proactive maintenance. Enhanced Decision-Making Through Simulation: Digital twins allow to simulate repair scenarios and operational adjustments in a virtual space which ensures the most efficient solutions are chosen. Cross-Industry Applications: From factory production lines to surgical procedures and autonomous driving, digital twins are transforming how industries plan, train, and optimize their systems. AI Driven Insights: The integration of AI and machine learning empowers digital twins to offer advanced analytics, automate corrective actions, and continuously learn from new data to improve accuracy over time.
Apr 11 2025
5 M
Meet Jen - ML Solutions Engineer at Encord
Another day, another episode of "Behind the Enc-urtain", where we go behind the scenes with the Encord team and learn more about their life and work! Today we sit down with Jen Ding, ML Solutions Engineer here at Encord. Our Solutions team plays a huge role in our commercial and technical progress as a company — they uncover new interesting use cases for us to support, build a strong feedback loop between our technical and commercial teams, and help empower leading AI teams across our customer base as they build cutting-edge AI applications in their F500 organizations, AI research labs and fast growing AI scale-ups. PS. We are hiring! We are looking for Solutions Engineers to join our London and San Francisco teams - you can find more about the London SE role here and SF SE role here. Let's start with a quick introduction — can you share a bit about your background and how you found your way to Encord? I come to Encord from a research institute, where I was part of a team building real-world applications of AI research. I focused on topics like open source and participatory practices for AI, stewarding a UK Choral AI dataset and co-founding a public data festival called London Data Week with the Mayor of London. Before that, I was part of a couple of applied ML startups as a Data Scientist and Solutions Engineer. Joining Encord’s Solutions team is a nice return to the startup world! How is Solutions Engineering at Encord different from Solutions Engineering in other environments? It’s a great moment to be an ML Solutions Engineer. In my first Solutions role years ago, Computer Vision was still an emerging research topic. My work focused more on demonstrating the potential of CV to customers by building custom models on their data to prove that it was a technology worth investing in. Now that more organisations are actively adopting and building products with AI, the work at a startup like Encord can focus more on enabling the frontier of AI applications. It’s been exciting to learn about the different AI dreams of our customers from radiology and robotics to vertical farming and video generation, and to create custom solutions with Encord’s suite of data products to help make these dreams come true. What is one exciting customer project you've worked on this month? The first few that come to mind are engagements with robotics companies that are building robots to perform tasks like tidying a room, cleaning windows, or delivering drinks. It’s been exciting to learn more about this dynamic problem space, and create demos to showcase how Encord can help improve and scale key datasets for this field. I’ve worked with colleagues from Encord’s Solutions and ML teams to build data agents that support expanding the linguistic breadth of Vision Language Action (VLA) datasets for robot tasks. I didn’t realise how challenging this problem space is, and the importance of collecting a semantically diverse set of instructions to capture the many ways different people may describe the same task. The Exact Instructions Challenge video captures how hard this problem can be for humans — let alone robots, which have a much more limited model of the world. I’m excited to see where our work in this space goes. What’s a misconception one might have about our product space from the ‘outside’? Data and data annotation may not be the “sexiest” of topics in the AI space. This can lead to the misconception that data work is not as important or requires less attention than training models or obtaining more compute. In fact, data quality remains one of the most important factors in model performance and efficient use of compute and is often the biggest competitive advantage for organizations adopting and applying AI within their domains. I’ve actually been working on a side initiative in this area — a video series called “AI Data Chats”. The plan would be to invite AI researchers and practitioners to get their “AI Data Hot Take” (paired with a drink of their choice!), to create more airtime for these key data topics that are currently underrepresented in the news and research. Now onto a rapid fire round... What 3 words would you use to describe the Encord culture? Dynamic, Dedicated and Customer-driven. Which fictional character would make the best Encord hire and why? Naomi Nagata from The Expanse would be an amazing member of the Solutions team! Ready for any challenge — engineering, negotiation, extraterrestrial life form — that comes her way and ready to implement a creative solution in T-10 seconds. Every second counts on the Rocinante (and a customer demo!) If you could time-travel to 2030 – what’s one thing you hope hasn’t changed about working at Encord? The Nugget Challenge! (iykyk) You can find Jen on Linkedin here and subscribe to AI Data Chats (which just launched this April!) here. See you at the next episode of “Behind the Enc-urtain” 👋
Apr 11 2025
5 M
Meet Rad - Head of Engineering at Encord
At Encord, we believe in empowering employees to shape their own careers. The company fosters a culture of ‘trust and autonomy’, which encourages people to think creatively and outside the box when approaching challenges. We strongly believe that people are the pillars of the company. With employees from over 20 nationalities, we are committed to building a culture that supports and celebrates diversity. For us, we want our people to be their authentic self at work and be driven to take Encord's mission forward. Rad Ploshtakov was the first employee at Encord and is a testament to how quickly you can progress in a startup. He joined as a Founding Engineer after working as a Software Engineer in the finance industry, and is now our Head of Engineering. Hi Rad! Tell us about yourself, how you ended up in Encord, and what you’re doing. I was born and raised in Bulgaria. I moved to the UK to study a masters in Computing (Artificial Intelligence and Machine Learning) at Imperial College London. I am also a former competitive mathematician and worked in trading as a developer, building systems that operate in single digit microseconds. Then I joined Encord (or Cord, which is how we were known at the time!) as the first hire - I thought the space was really exciting, and Eric and Ulrik are an exceptional duo. I started off as a Founding Engineer and, as our team grew, transitioned to Head of Engineering about a year later. I am responsible for ensuring that as an engineering team we're working on what matters most for our current and future customers - I work closely with everyone to set the overall direction and incorporate values for the team. Nowadays, a lot of my time is also spent on hiring, and on helping build and maintain an environment in which everyone can do their best work. What does a normal day at work look like for you? Working in a startup means no two days are the same! Generally, I would say that my day revolves around translating the co-founders' goals into actionable items for our team to work on - communicating and providing guidance are two important aspects of my role. A typical day includes meeting with customers and prospects, code reviewing, and supporting across different initiatives. Another big part is collaborating with other teams to understand what we want to build and how we are going to build it. Can you tell us a bit about a project that you are currently working on? Broadly speaking, a lot of my last few weeks has been supporting our teams as they set out and execute on their roadmaps. 2023 will be a huge year for us at Encord, and we're moving at a very fast pace, so a lot of my focus recently has been helping us be set up for success. As for specific projects, I'm very excited about all the work our team is doing for our customers. For example, our DICOM annotation tool has recently been named the leading medical imaging annotation tool on the market - which is a huge testament to the work our team has poured into it over the last year. I remember hacking together a first version of our DICOM annotation tool in my first (admittedly mostly sleepless!) weeks at Encord, and seeing how far it's come in just a few months has been one of the most rewarding parts of my last year. What stood out to you about the Encord team when you joined? Many things. When I first met the co-founders (Eric & Ulrik), I was impressed by their unique insights into the challenges that lay ahead for computer vision teams - they can simultaneously visualize strikingly clearly what the next decade will look like, while also being able to execute at mind-boggling speed in the moment, in that direction. I was impressed also by how smart, resourceful and driven they were. By the time I joined, they had been able to build a revenue generating business with dozens of customers - getting to understand deeply the problems that teams were facing and then iterating quickly to build solutions that not even they had thought about. What is it like to work at Encord now? It's a very exciting time to be at Encord. Our customer base has been scaling rapidly, and the feedback loop on the engineering cycle is very short, so we get to see the impact of our work at a very quick pace which is exciting - often going from building specs for a feature, to shipping it, showing it to our customers, and seeing them starting to use it all happen in a span of just a few weeks. A big part of working at Encord is focusing a lot on our customer's success - we always seek out feedback, listen, and apply first principles to the challenges our customers are facing (as well as getting ahead with ones we know they'll be facing soon that they might not be thinking about yet!). Then work on making the product better and better each day. How would you describe the team at Encord now? The best at what they do - also hardworking, very collaborative and always helping and motivating each other. One of our core values is having a growth mentality, and each member of our team has come into the company and built things from the ground up. Everyone has a willingness to roll up their sleeves and make things happen to grow the company. A resulting factor of this is also that it's okay to make mistakes - we are constantly iterating and trying to get 1% better each day. We have big plans for 2023 & are hiring across all teams! ➡️ Click here to see our open positions
3 M
Explore our products