Contents
Top Picks for Computer Vision Papers You Should See
Want to get hands-on? Check Out These Computer Vision Tutorials
Developer Resources You’d Find Useful
Practical Computer Vision Use Cases
Top 3 Resources by Encord in January
Our Power Tip of the Month
Encord Blog
Encord Monthly Wrap: January Industry Newsletter
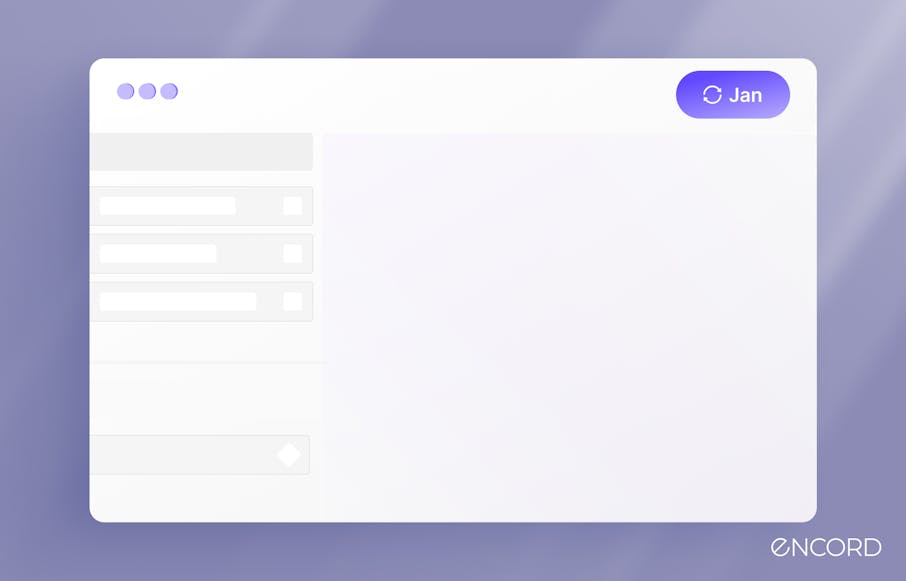
Written by

Stephen Oladele
View more postsWelcome to the January 2024 edition of Encord's Monthly Wrap.
It’s also our chance to wish you a belated happy new year!
Here’s what you should expect:
- Two interesting computer vision papers we reckon you check out.
- Hands-on tutorials you can work on during weekends.
- Developer resources you should bookmark, including Colab Notebooks.
- Computer vision use cases in manufacturing and robotics.
- Power tip for computer vision data explorers.
Let’s dive in!
Top Picks for Computer Vision Papers You Should See
Segment Anything in Medical Images (MedSAM)
This paper presents MedSAM, a novel adaptation of the Segment Anything Model (SAM) specifically for medical images.
What’s impressive? 🤯
- It introduces a large-scale medical image dataset with over 200,000 masks across 11 modalities and utilizes a fine-tuning method to adapt SAM for general medical image segmentation.
- It demonstrates superior performance over the original SAM, significantly improving the Dice Similarity Coefficient on 3D and 2D segmentation tasks.
There’s also an accompanying repository with a shoutout to one of our pieces on fine-tuning SAM 😉.
CLIP in Medical Imaging: A Comprehensive Survey
This survey explores the Contrastive Language-Image Pre-Training (CLIP) application in the medical imaging domain. It delves into the adaptation of CLIP for image-text alignment and its implementation in various clinical tasks.
What’s impressive? 👀
- It provides an in-depth analysis of CLIP's utility in medical imaging, covering the challenges of adapting it to the specific requirements of medical images.
- It shows how well CLIP generalizes tasks like 2D and 3D medical image Fsegmentation, medical visual question answering (MedVQA), and generating medical reports.
Illustration of CLIP’s generalizability via domain identification
Medical professionals use Encord’s DICOM & NIfTI Editor to quickly label large training datasets across modalities such as CT, X-ray, ultrasound, mammography, and MRI.
- How Harvard Medical School and MGH Cut Down Annotation Time and Model Errors with Encord
- Stanford Medicine reduced experiment times by 80%.
- Floy reduced label times by 50% for CT and 20% for MRI scans.
Want to get hands-on? Check Out These Computer Vision Tutorials
- [COLAB NOTEBOOK] How to Use the Depth Anything Model → The Depth Anything model is trained on 1.5 million labeled images and 62 million+ unlabeled images jointly and provides the most capable Monocular Depth Estimation (MDE) foundation models. This notebook shows you how to use the pipeline API to perform inference with any of the models. Here is the original paper (the image was adapted).
- How to Detect Data Quality Issues in Torchvision Dataset using Encord Active → This article shows you how to use Encord Active to explore images you have preloaded with Torchvision, identify and visualize potential issues, and take the next steps to rectify low-quality images.
- How to Use OpenCV With Tesseract for Real-Time Text Detection → This is a code walkthrough guide on building an app to perform real-time text detection from a webcam feed.
Developer Resources You’d Find Useful
- How to Pre-Label Data at Speed with Bulk Classifications → If you're working with large unlabeled datasets and want to quickly classify and curate for labeling, you’ll find our tutorial on pre-labeling data at warping speed with bulk classification useful.
- Best Image Annotation Tools for Computer Vision [Updated 2024] → Choosing the right image annotation tool is a critical decision that can significantly impact the quality and efficiency of the annotation process. To make an informed choice, this article considers several factors and evaluates suitable image annotation tools for your business needs.
- Generate Synthetic Data for Deep Object Pose Estimation Training with NVIDIA Isaac ROS → NVIDIA developed Deep Object Pose Estimation (DOPE) to find the six degrees of freedom (DOF) poses of an object. In this article, they illustrated how to generate synthetic data to train a DOPE model for an object.
- Best Computer Vision Projects With Source Code And Dataset → An article with 16 ideas for computer vision projects for beginners and start building.
Practical Computer Vision Use Cases
- Top 8 Use Cases of Computer Vision in Manufacturing → This article discusses the diverse applications of computer vision across various manufacturing industries, detailing their benefits and challenges, from product design and prototyping to operational safety and security.
- Top 8 Applications of Computer Vision in Robotics → This article explores computer vision applications in the robotics domain and mentions key challenges the industry faces today, from autonomous navigation and mapping to agricultural robotics.
Top 3 Resources by Encord in January
- How to Adopt a Data-Centric AI → For data teams to succeed in the long term, they must use high-quality data to build successful AI applications. But what is the crucial sauce for building successful and sustainable AI based on high-quality data? A data-centric AI approach! We released this whitepaper to guide you on how to develop an effective data-centric AI strategy.
- Top 15 DICOM Viewers for Medical Imaging → In the market for a DICOM viewer? We published a comparison article that discusses what to look for in an ideal viewer and the options in the market so you can make the optimal choice.
- Instance Segmentation in Computer Vision: A Comprehensive Guide → We published an all-you-need-to-know guide on instance segmentation, including details on techniques like single-shot instance segmentation and transformer- and detection-based methods. We also cover the U-Net and Mask R-CNN architectures, practical applications of instance segmentation in medical imaging, and the challenges.
Our Power Tip of the Month
If you are trying to become a computer vision data power user, I’ve got a tip to help supercharge your exploration gauntlet (I see you, Thanos 😉).
Within Encord Active, you can see the metric distribution of your data to identify potential data gaps that could influence model performance on outliers or edge cases. Here’s how to do it in 3 steps on the platform: Analytics >> Scroll down to Metric Distribution >> Choose a pre-built or custom Metric, and observe!
Good stuff 🤩. I hope you find it useful. Here are other quick finds if you 💓 Encord and computer vision data stuff ⚡:
- Data-centric computer vision blog
- Join the Encord Community to discuss the resources
- GitHub repo
- The Docs
Till next month, have a super-sparkly time!
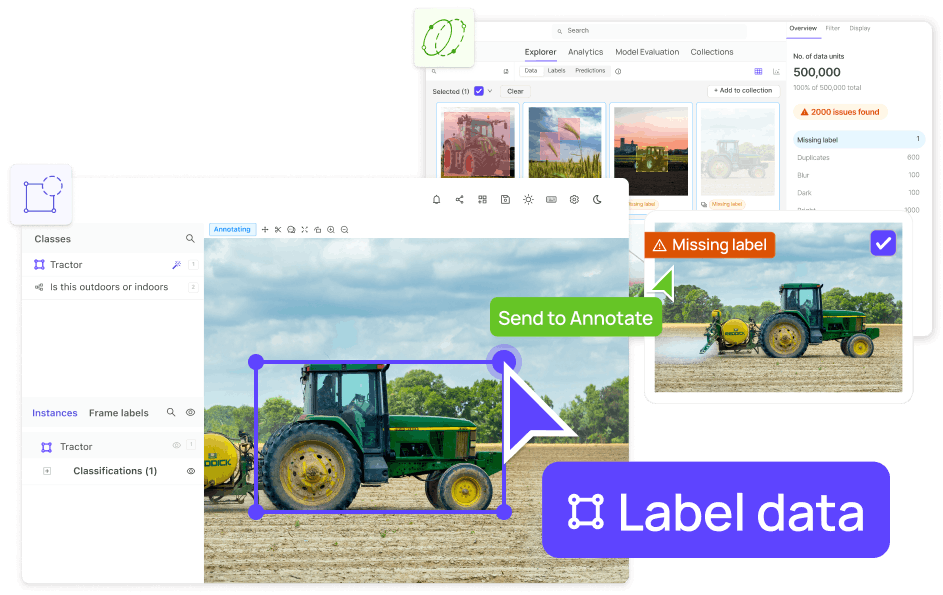
Build better ML models with Encord
Get started todayWritten by

Stephen Oladele
View more posts