Contents
Is It Built To Scale?
Cost Grows with Complexity
I/O Is Key to Success
Starting from Scratch
Doesn’t Get Smarter With Time
Conclusion
Encord Blog
Why You Should Ditch Your In-House Training Data Tools
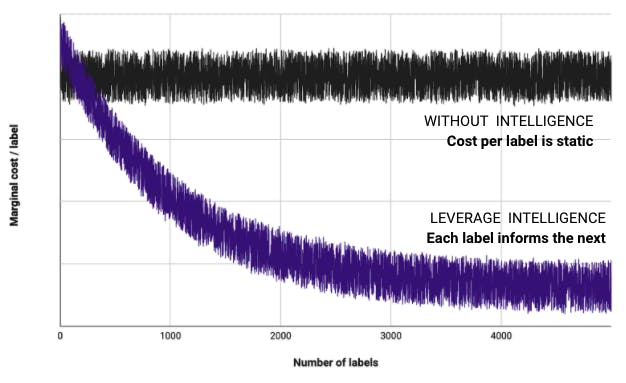
At Encord, we’ve spent weeks interviewing data scientists, product owners, and distributed workforce providers. Below are some of our key learnings and takeaways for successfully establishing and scaling a training data pipeline.
If you’ve ever dabbled in anything related to machine learning, chances are you’ve used labeled training data. And probably lots of it. You might even have gone through the trouble of labeling training data yourself. As you have most likely discovered, spending time creating and managing training data sucks — and it sucks even more if you can’t find an open-source tool that fits your specific use case and workflow.
Building custom tools might seem like the obvious choice, but making the first iteration is typically just the tip of the iceberg. More start- and scale-ups than we can count end up spending an insurmountable amount of time and resource building and maintaining internal tools. Making tools is rarely core to their business of building high-quality machine learning applications.
Here are things to consider when establishing your training data pipeline and when you might want to ditch your in-house tools.
Is It Built To Scale?
You’ve produced the first couple of thousand labels, trained a model, and put it into production. You begin to discover that your model does poorly in specific scenarios. It could be that your food model infers a tomato as an orange in dim lighting conditions, for example. You decide to double or even triple your workforce to keep up with your model’s insatiable appetite for data to help solve these edge cases. If your tool is built on top of CVAT — like most of the machine vision teams we’ve worked with — it quickly starts to succumb to the increased workload and comes down crashing faster than you can say Melvin Capital.
Cost Grows with Complexity
Machine learning is an arms race. Keeping up with the latest and greatest models require you to re-evaluate and update your training data. That typically means that the complexity of your label structure (ontology) and data grows, requiring you to add new features to your in-house tools continuously. New features take time to build and will be around to maintain long after, eating up precious resources from your engineering team and dragging down your expensive workforce’s productivity. This cost is not immediately apparent when you are first building out a pipeline but can become a considerable drag on your team as your application grows.
I/O Is Key to Success
A robust pipeline should give you a complete overview of all of your training data assets and make it easy to pipe them between different stakeholders (annotators, data scientists, product owners, and so on). Adequate piping necessitates that the data resides in a centralized repository and that there is only a single source of truth to keep everyone synced. Building a series of well-defined APIs that allows for effective pushing and pulling data is no small feat. Additionally, making a good API is often complicated by attempting to mould training labels produced by open-source tools into queryable data assets.
Label I/O should be as simple as calling a function
Starting from Scratch
When establishing a training data pipeline, the perennial mistake teams make when they spend money on a workforce is starting the annotation process from scratch. There are enough pre-trained pedestrian and car models to cut initial annotation costs drastically.
Even if you are working on something more complex, using transfer learning on a pre-trained model fed with a few custom labels can get you far. An additional benefit is that it allows you to understand where a model might struggle down the line and immediately kickstart the data science process before sinking any money into an expensive workforce. At Encord, we applied this exact method in our collaboration with the gastroenterology team at King’s College London, helping them speed up their labeling efficiency by 16x, which you can read more about here.
Labeling Pre-Cancerous Polyps Case Study: Marginal cost per label with and without utilizing pre-trained models & data algorithms
Doesn’t Get Smarter With Time
In addition to using pre-trained models, intelligently combining heuristics and other statistical methods (what we like to call ‘data algorithms’) to label, sample, review, and augment your data can drastically increase the ROI on human-produced labels. Existing software doesn’t apply these intelligent ‘tricks’, which means that the marginal cost per produced label remains constant. It shouldn’t. It should fall, even collapse, as your operation scales.
We’ve seen teams attempt baking in some of these methods in their existing pipelines. However, each data algorithm can take days, if not weeks, to implement and often lead to nasty dependency headaches. The latter can be a substantial time suck — we know first-hand how frustrating it can be to line up the exact version of CUDA matching with PyTorch, matching with torchvision, matching with the correct Linux distribution… you get the idea.
Conclusion
If any of the above points resonate with you, it might be time to start looking for a training data software vendor. While the upfront cost of buying or switching might seem steep relative to building on top of an open-source tool, the long-term benefits most often outweigh the costs by orders of magnitude. Purpose-built training data software ensures that all of your stakeholders’ needs are satisfied, helping you cut time to market and increase ROI. If you’re a specialist AI company or a company investing in AI, training data is at the core of your business and forms a vital part of your IP. It is best to make the most of it.
Power your AI models with the right data
Automate your data curation, annotation and label validation workflows.
Get startedWritten by

Ulrik Stig Hansen
Explore our products