CUSTOMER STORIES
See how teams are preparing their data for AI with Encord
From cutting edge start-ups to enterprise and international research organisations, our tools and infrastructure are accelerating the development of cutting-edge AI applications

20%
increase in model performance
Boosting Last-mile Model Performance

$600K
saved per year
How Standard AI Powers Retail Intelligence At Scale
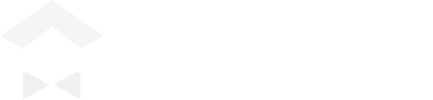
60%
increase in labeling speed
Improving Efficiency in the Construction Industry with Encord
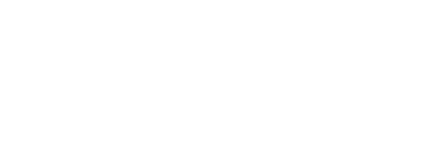
30%
improvement in annotation accuracy
How Pickle Robot Is Using Encord To Build Physical AI For Warehouse Automation

40+
annotators trained using Encord's QA and annotation review features
Building Multimodal AI Systems for Insurance with Tractable
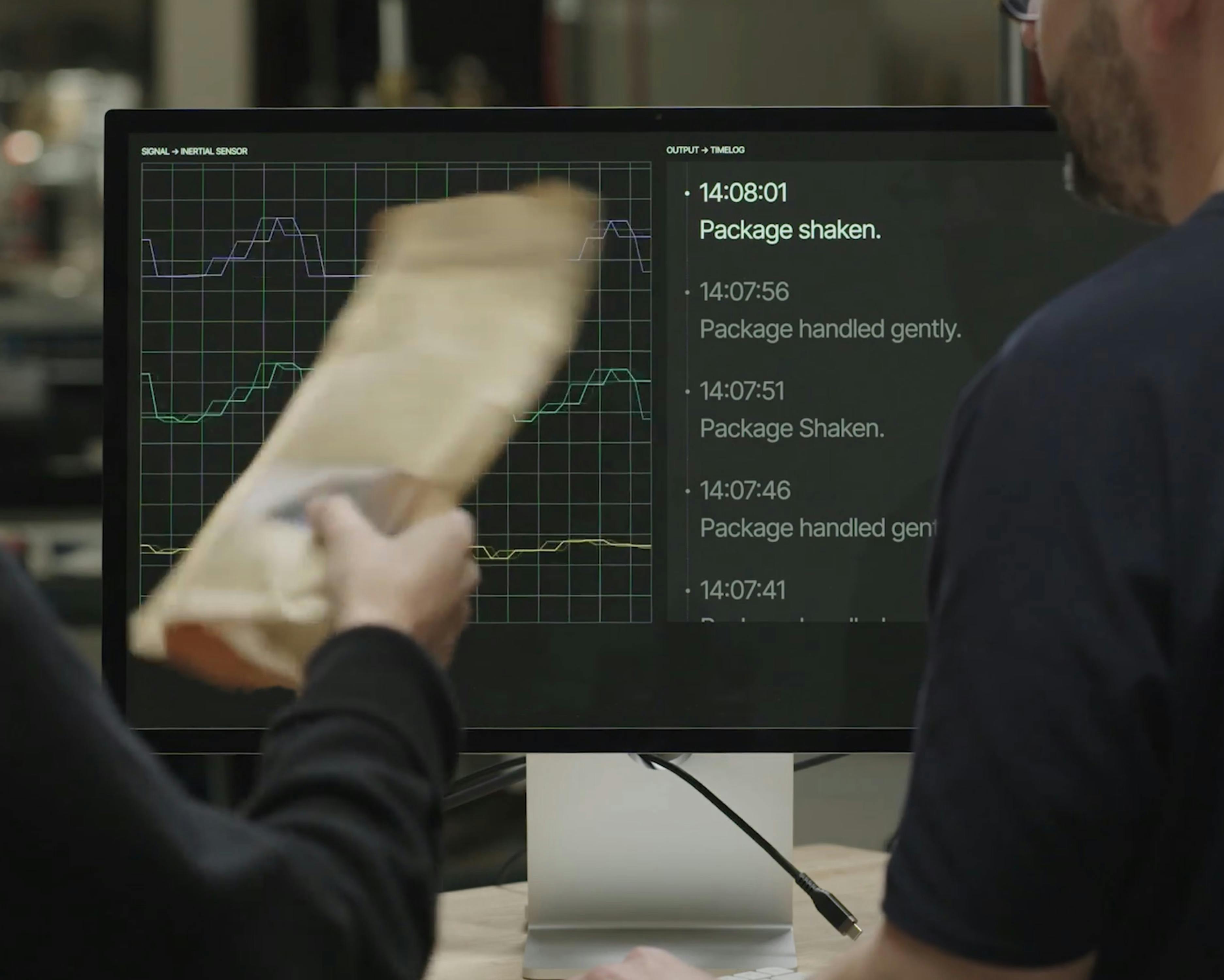

How Encord Made Archetype AI’s Team 70% More Efficient
Introducing Customer: Archetype AI Archetype AI is building the first developer platform for Physical AI — powered by Newton, a foundation model trained on real-world sensor data. The platform enables enterprises to build and deploy custom AI applications for the physical world through simple APIs and no-code tools. With deep multimodal sensor fusion, Newton delivers rich, accurate insights across industries — from predictive maintenance to human behavior understanding. They're working with global leaders like Mercedes-Benz, NTT Data, and SLB, and backed by Venrock, Amazon, and Bezos Expeditions. Before Encord Prior to adopting Encord, Archetype AI managed data curation and annotation through a combination of internally developed tools and third-party platforms. A key limitation was the lack of native video support within their primary annotation tool. Without the ability to annotate directly on video, annotators were forced to label frame by frame — an inefficient and labor-intensive process that significantly hindered throughput. Object tracking capabilities were similarly constrained, leading to inconsistent performance and difficulty in maintaining continuity across frames. As the scale and complexity of the data increased, maintaining label accuracy was difficult due to limited visibility in the annotation interface, and the overall user experience lacked the flexibility required for managing high-volume video datasets. These inefficiencies created friction throughout the pipeline, delaying project timelines and impacting the quality of data used for model development. Recognizing the need for a more robust and purpose-built solution, the team began evaluating platforms capable of supporting scalable, high-performance video and multimodal annotation workflows. The Encord Solution <comment>What stood out to us wasn’t just one feature — it was how Encord brought together scalability, video-native annotation, clear label visibility, and the flexibility to support other modalities in a single, cohesive platform. It was the completeness of the solution, not just its individual parts, that aligned perfectly with our evolving needs.</comment> <image>https://images.prismic.io/encord/aDg96ydWJ-7kSrIr_nickgillian_1.jpeg?auto=format,compress</image> <author>Nick Gillian, Founder, Head of AI & SW.</author> Since adopting Encord, the team has achieved a 70% increase in overall productivity. Most significantly, annotation speed has doubled, allowing the team to work far more efficiently than with previous tooling. This improvement is driven by Encord’s fast and intuitive annotation tools, powerful features like trackers and bulk operations, and most importantly, the flexibility of Encord's SDK, which enabled the team to build custom ETL pipelines for seamless data ingestion and annotation retrieval. Tasks that previously required manual upload/download steps and browser-heavy interactions are now fully streamlined. Together, these capabilities have drastically streamlined Archetype AI’s end-to-end labeling workflow. Encord’s intuitive interface has streamlined project setup and management. It’s now easier for them to design workflows, assign and review tasks, and maintain full visibility across active projects. Labeling accuracy has also improved, leading to tangible gains in model performance, highlighting the impact of having the right data labeled correctly from the start. The platform made it easy for the team to experiment with new annotation methodologies and iterate quickly, without being constrained by tooling limitations. This flexibility introduced a new level of agility, enabling the team to adapt and innovate faster across their data pipeline. Collectively, these benefits have resulted in substantial time savings, improved model outcomes, and a more scalable and adaptable approach to handling complex multimodal data.
Physical AI
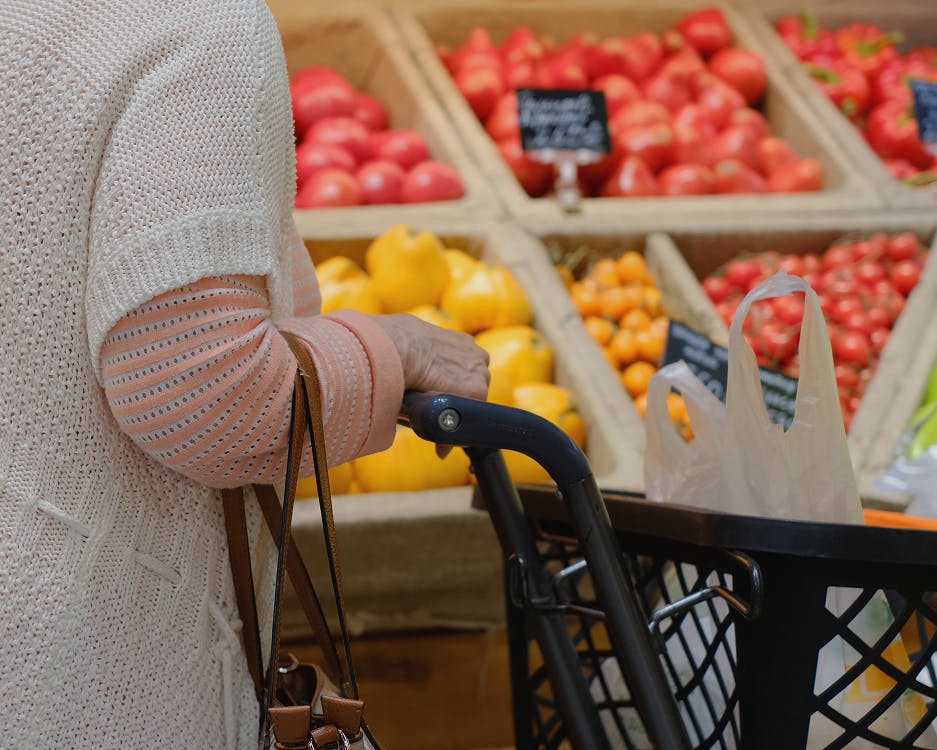

How Standard AI Powers Retail Intelligence at Scale With Encord
Introducing Customer: Standard AI Retailers today face some of the toughest challenges in physical stores — from tracking shopper behavior to optimizing promotions and in-store media. Standard AI solves these challenges by equipping retailers and CPG brands with AI-powered computer vision technology that unlocks real-time insights on promotions, media impact, new product launches, and more. The VISION platform turns data into actionable insights, helping retailers drive sales, improve store performance, and gain deeper visibility into the shopper journey. Standard AI is equipping brick-and-mortar stores and their suppliers with real-time in-store analytics, all while maintaining a privacy-first approach. Helmed by an executive team that spans firms like Mars, Sara Lee, Oracle, NASA, and Adobe, Standard AI is at the forefront of applying artificial intelligence and computer vision to physical retail spaces. The data challenge: lower quality, longer time to value At its core, Standard AI’s business is based on its ability to extract meaningful insights from millions of hours of video footage from store’s security cameras. For the ML team, access to high-quality and enriched video data was an extremely cumbersome, time-consuming process that relied on multiple internal and agency hand-off points, lengthy quality control review cycles, and fragmented point solutions. In several instances, custom scripts were needed to glue these workflows together. The ML team spent more time managing fragile data pipelines than focusing on model performance. The product and ML teams also wanted to find a way to streamline the data curation and annotation processes and spend more time evaluating and fine-tuning their models to continue improving performance. This wasn’t possible due to limitations of Standard AI’s existing tooling, which offered limited debugging, quality control, and statistical insights. From a business perspective, Standard AI understood that the way of managing their AI data pipeline wouldn’t scale or support the rapid innovation needed to meet customer demands. Standard AI’s Product and ML team defined strict requirements for their updated approach: Native support for large-scale video processing (including fish eye lens) with support for other modalities A unified platform for curation, labeling, and evaluation Robust API and SDK support Ability to automate labeling tasks with HITL A simplified user experience for non-technical users The solution: easy access to pixel-perfect video data to build production-grade AI <comment>With Encord, we went from spending multiple weeks labeling large volumes of retail video, having to download all the data, process it, and curate the data, to our present state, where the Product and the ML team iterates in days visually, collaboratively, and seamlessly.</comment> <image> https://images.prismic.io/encord/aDWIHSdWJ-7kSka9_Image-1-.png?auto=format,compress</image> <author>Bruno Abbate, Head of Machine Learning.</author> Native video processing — With prior approaches, the Standard AI team spent long cycles manually uploading videos to other platforms and faced significant conversion issues. With Encord’s native video support and API, the Standard AI team is now able to upload and process millions of video files 5x faster. Unified data management platform for AI — Standard AI shifted from relying on outsourced labelers, open-source tooling, and niche labeling platforms to Encord, a unified platform that streamlines data curation and annotation at enterprise scale. Robust API and SDK support — The API integration for data upload is intuitive, enabling rapid implementation. and programmatic data annotation at speed with the Encord SDK. Granular ontologies — Standard AI was able to rapidly refine complex hierarchical ontologies in response to evolving project insights, continuously enhancing data labeling precision. AI-assisted labeling with HITL — Standard AI benefited from automated labeling functions paired with human-in-the-loop reviews to ensure label accuracy whilst speeding up labeling pipelines. Seamlessly scalable across the organization — The ML team has democratized access, enabling all team members to add, annotate, and utilize enriched data for their specific tasks. This distributed approach accelerates company-wide progress by eliminating bottlenecks previously created by specialized tooling gatekeepers. <comment>Encord transformed how we manage AI data at scale. Instead of fragmented workflows, we now seamlessly ingest and label millions of video frames—iterating in hours rather than weeks. This shift lets us focus on advancing AI-driven insights across video, behavior, and spatial signals—not just annotation.</comment> <image> https://images.prismic.io/encord/aDWIfCdWJ-7kSkbd_Image.png?auto=format,compress</image> <author>Ryan Cook, Senior Technical Product Manager</author> Benefits: Standard AI built production-ready models faster and at lower cost <comment>With Encord, our mindset has shifted from what we can do within the limits of our tooling to what is possible with Encord to delight our customers further</comment> <image>https://images.prismic.io/encord/aDWIvydWJ-7kSkbx_Image-2-.png?auto=format,compress</image> <author>Jeremie Morfin, Director of Product</author> Since moving to Encord, Standard AI has seen a drastic improvement in productivity across its product and ML teams, significant cost savings, improved data quality, and faster time-to-model improvement. Key benefits: Saved $600k a year Process millions of video files 5x faster with Encord’s native video platform 99.4% faster project initiation
Physical AI
Retail & E-Commerce
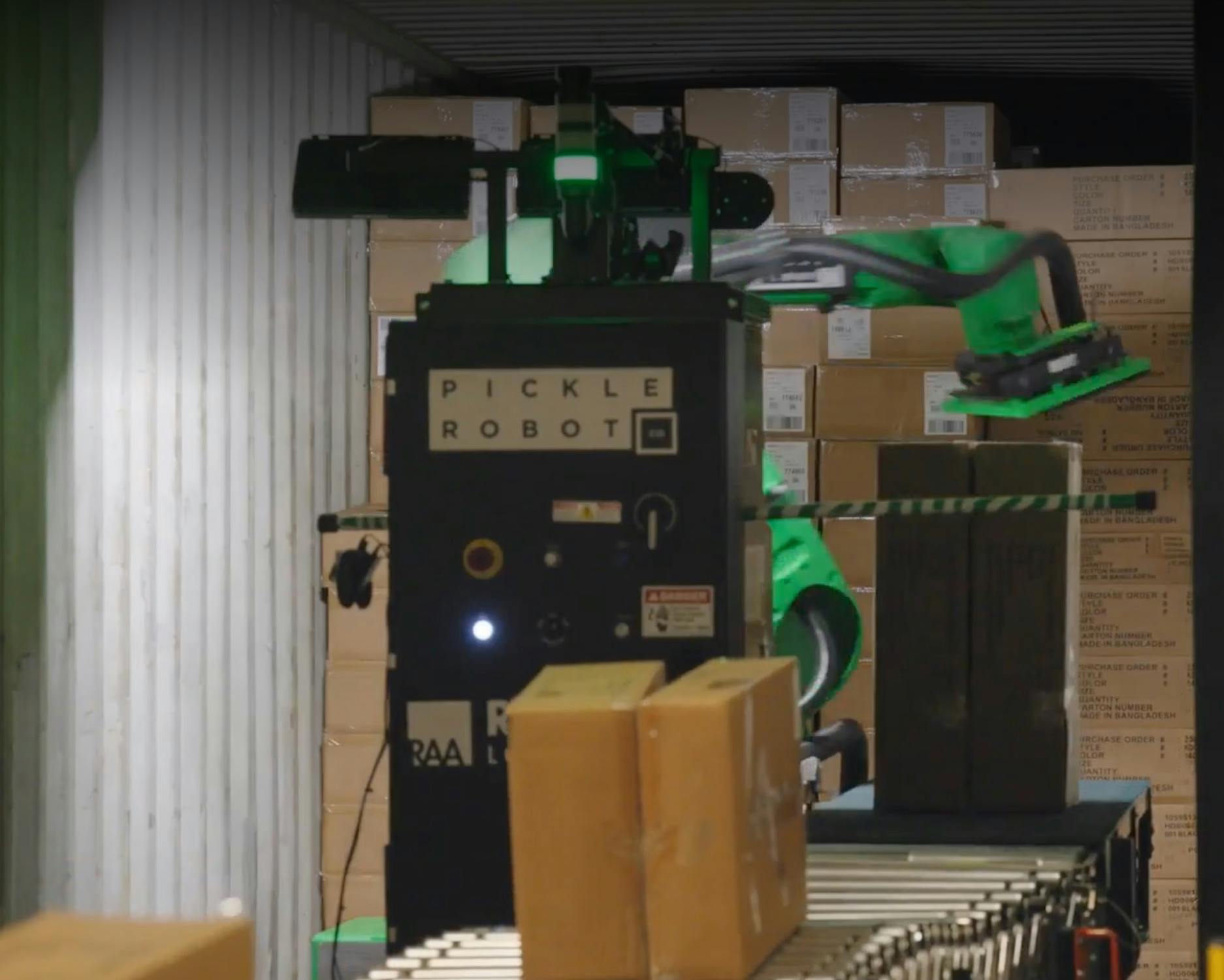
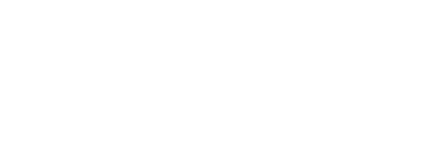
How Pickle Robot Is Using Encord To Build Physical AI For Warehouse Automation
Introducing: Pickle Robot Pickle Robot, a Cambridge, MA-based Physical AI innovator, is revolutionizing the logistics industry with cutting-edge applications of hardware, AI, and data. Focused first on optimizing truck unloading and loading, Pickle Robot streamlines one of the most physically demanding tasks in warehousing. Historically, the process of unloading non-palletized goods from trailers relied heavily on manual labor, resulting in fatigue and inefficiencies and prone to high worker safety risks. To address these challenges, a team of MIT graduates came together to form Pickle Robot. They developed a sophisticated solution that uses advanced AI algorithms trained on highly accurate images and videos to automate the unloading of up to 1,500 packages per hour with its green mobile manipulation robots. The purpose-built solution combines custom AI/ML, highly contextualized data, and off-the-shelf hardware and sensors, significantly reducing the time, effort, and risk in an often overlooked part of the supply chain that warehouses spend over $100 Billion a year to do. Today, they are helping their customers like UPS, Ryobi Tools, and Randa Apparel & Accessories unload millions of pounds of packages monthly. The data challenge To build fast and precise robotic systems, Pickle Robot needed to think about the end-to-end experience while facing hurdles across hardware and AI models. Specifically on the model front, Pickle Robot recognized the need for a robust AI system capable of handling diverse cargo. This led the team to implement a unique combination of sensors and machine learning models to identify different types of packages and manipulate various goods accurately. The integration of these technologies enhanced operational efficiency and minimized errors and downtime. Achieving success required a robust data engine and rich images with precise annotations. Prior to Encord, Pickle Robot used data annotation services from other providers. It relied on an outsourced labeling team to conduct the labeling within the software's limitations and the skills of the outsourced labelers. Challenges included: Poor labels—overlapping polygons, or, more often than not, a significant number of packages were submitted with incomplete labels. Excessive auditing cycles—the legacy approach was error-prone. The lean team of AI and ML engineers spent up to 20+ minutes on auditing tasks per cycle, with high rejection rates. Complex semantic segmentation ontologies were infeasible, which inhibited the robot's ability to accurately understand its operating environment. Platform unreliability limited the efficacy of automated workflows and reduced the time available for model development. Accuracy in training data is critical when your business depends on the accuracy and efficiency of the robotics system's performance. Utilising Encord for consolidated data curation & annotation To address these challenges, Pickle Robot made a strategic decision to partner with Encord. With Encord, Pickle Robot gained a platform that does data curation, annotation, and provides robust analytics and model evaluation functionality, with full integration to Pickle Robot's Google Cloud Platform based data engine infrastructure. The Encord platform enables data management and discoverability capabilities, granular annotation features (bounding boxes, polylines, key points, bitmasks, and polygons), nested ontologies, collaborative workflows, AI-assisted labeling with HITL, and comprehensive data curation functionality required to run efficient data pipelines. "For our AI initiatives, rapid automation is critical. Encord and our ML infrastructure allow us to prototype learning tasks efficiently. The composability of Encord enables us to merge diverse data sources, facilitating extensive experimentation. With a well-integrated SDK, it's a matter of a few lines of code to achieve seamless integration and functionality." - Matt Pearce, Applied ML, Pickle Robot Benefits: Pickle Robot increased precision by 15% and iterated models 60% faster Since Pickle Robot partnered with Encord, Pickle Robot has seen a drastic improvement in the AI and ML engineers’ productivity, improved precision in task execution, and faster time-to-model improvement. Key benefits: Achieving reliable data pipelines for model training and evaluation 60% faster 30% improvement in annotation accuracy Faster and more comprehensive audit and review cycles Increased observability of real-time data distributions, allowing for rapid domain drift corrections. 15% Improvement in robotic grasping accuracy with better training data
Physical AI
Logistics
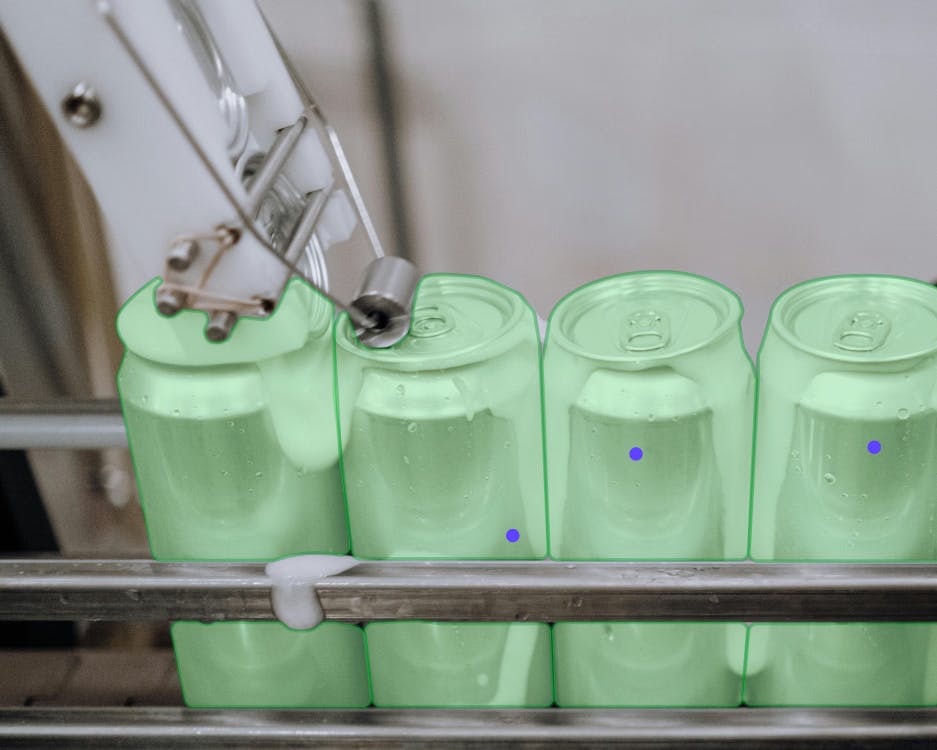
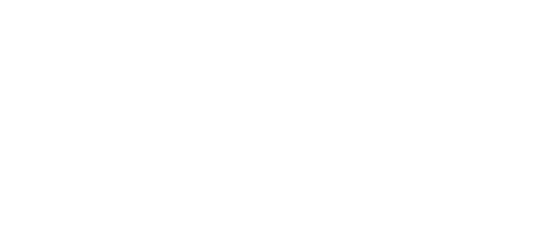
Slashing Data Management Overhead By 90%: How Plainsight Streamlined Vision Data Pipelines with Encord
Introducing Customer: Plainsight Technologies Plainsight Technologies is the enterprise vision data company that makes your cameras count. Plainsight dramatically reduces the cost and complexity of AI-powered computer vision across many verticals and enterprise use cases, including manufacturing, retail, food service, theft, and more. As companies automate their operations with AI and Agents, Plainsight brings vision capabilities to watch and analyze events in the real world and generate structured data for analytics and reporting. Problem: Struggles with in-house built data pipelines Plainsight's mission to provide an application framework for computer vision was hindered by challenges in their data management and annotation processes. Initially, they invested in building their own end-to-end management and annotation pipelines. However, their homegrown solutions fell short of their desired standard and required significant resources to maintain and update, distracting the team from their core objective. Additionally, Plainsight needed a solution that respected data privacy and could handle the granular segmentation of customer data, as their customer's training data is proprietary and highly sensitive. Solution: Utilising Encord for an end-to-end data development platform To address these challenges, Plainsight made a strategic decision to discontinue their internal management and annotation tooling. They sought an external solution that could meet their specific needs. After evaluating several options, they chose Encord as their provider. Encord offered a comprehensive, industry-leading data development platform that integrated seamlessly with Plainsight's existing data pipelines. The platform enabled high-level data management and discoverability capabilities, granular annotation features, and strong data curation functionality required to run efficient data pipelines. Kit (CEO, Plainsight) says, “Before using Encord, it was challenging to see all the data, projects, and annotations in one place. I constantly had to ask questions to understand what was going on. Now, with Encord I feel like we have a much clearer understanding of everything that's happening.” The Plainsight team specifically mentioned the automated annotation tooling, notably the SAM 2 model, as a key improvement over their previous set-up. By partnering with Encord, Plainsight was able to focus on its core mission without the challenges of managing in-house data pipelines. Results: Reduced data management complexity by 90% and annotation costs by 50%. The adoption of Encord led to significant improvements for Plainsight and their customers: Streamlined Cloud Bucket Management: Reduced from 14 buckets per customer to 1 bucket per customer by using Encord folder structures, cutting bucket management complexity by over 90%. Faster Project Setup and Workflow Efficiency: Reduced labeling project setup time from 1 day to 1-2 hours and simplified data export processes. Increased Annotation Efficiency and Cost Savings: Encord’s Automated Labeling Suite, including tools like SAM 2 assisted labeling, boosted annotator productivity, reduced manual annotation time and costs by approximately 50%. Direct Export to Model Training: Direct export of labeled data to their model training programs remove the need for intermediary storage. Plainsight is now able to focus on their strategic priorities instead of struggling with their underperforming and complex in-house tools. The leadership team has full oversight of all their data and is empowered to build optimal data pipelines and models for their customers, accelerating the process of bringing structured data to cameras worldwide.
CV Platform
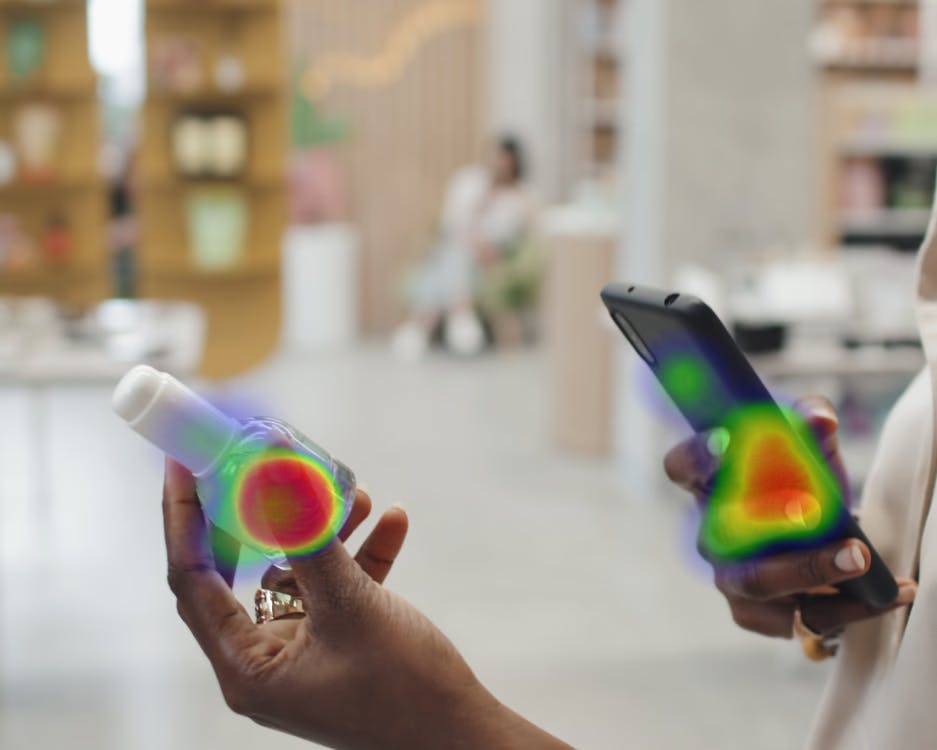
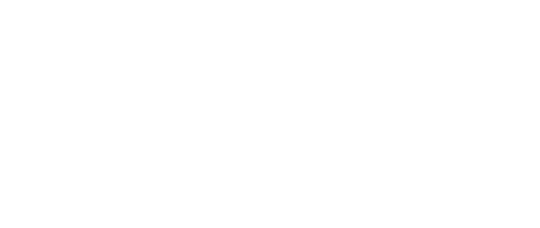
Accelerating the Prediction of Consumer Behaviour with Encord
Introducing Customer: Neurons Neurons has been a leading company in the field of neuroscience marketing for more than 10 years. With their expertise in understanding how humans react to content, they have developed AI-powered solutions to enable companies to create predictable marketing content at scale. Their mission is to eliminate biases and guesswork from marketing strategies. As a pioneer in this domain, Neurons faced scalability challenges in their data annotation process due to the sheer volume of label categories and the growing number of assets requiring annotation. We sat down with Dennis Green-Lieber (Director of Product and Engineering) and Konstantina Kaisheva (AI Product Manager) to discuss how Encord alleviated these challenges. Problem: Challenges with in-house solution Neurons, historically, have relied on manual annotation by their internal team using their in-house annotation platform. With a growing volume of assets requiring annotation, they found it increasingly challenging to scale their operations using their in-house solutions. They also encountered difficulties in ensuring precision and consistency when labeling large volumes of data, resulting in delays in getting their models to production. Solution: Scaling with Encord's end-to-end platform Seeking a solution to their data annotation challenges, Neurons turned to Encord’s platform. They recognized the need for manual and automated labeling capabilities, so they were drawn to Encord’s comprehensive offering. With Encord, Neurons found a user-friendly platform that facilitated a precise and efficient annotation process. They appreciated the ability to define labeling instructions/ontologies tailored to their specific requirements, ensuring accuracy in their data sets. Encord's platform offered features for quality assurance, workflow management, and data categorization streamlining Neurons’ annotation workflows. They were particularly impressed by Encord's flexibility, which allowed them to adapt labeling criteria for different content types. Konstantina, AI Product Manager, noted her happiness with the function of model evaluations. Recognizing its potential as an invaluable tool for the future, she highlighted its ability to provide insights into the performance and accuracy of its AI models. "It's a very easy platform to operate. What I really liked was the whole offering of Encord for both automated labeling and manual work, as precision is incredibly important to us."- Konstantina Kaisheva Result: Efficient and fast annotation With Encord’s platform, Neurons achieved marked improvements in their data annotation process. With the appropriate workflows and quality assurance, they were able to maintain precision and consistency even when they were handling larger volumes of data. This led to building a higher-quality dataset for their AI models. Neuron’s newly launched Copilot, provides actionable insights for marketing decision makers. This is run by AI models which require large volumes of precisely annotated datasets, which Encord enabled them to achieve. By eliminating guesswork and biases, they empowered their clients to make informed decisions and optimize their marketing strategies effectively. Overall, Encord’s platform proved to be a significant asset for Neurons, enabling them to overcome their data annotation challenges. With a newfound ability to make data-driven decisions, the company saw a tangible improvement in its ability to achieve their desired model outcomes.
Consumer
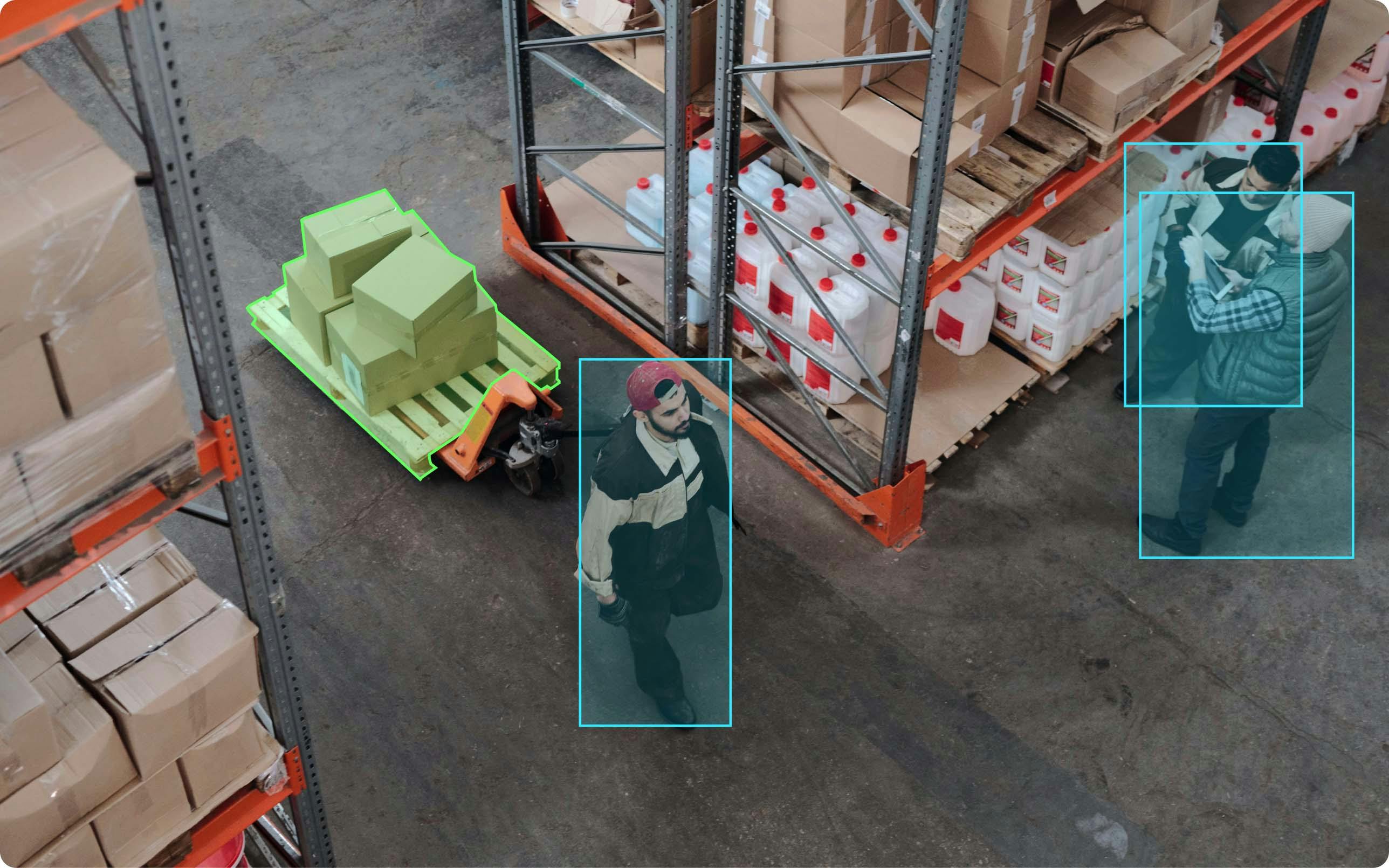
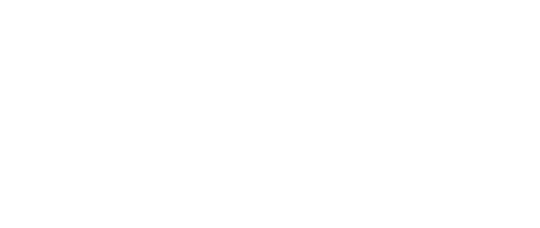
Finding a Reliable Ecosystem to Scale Model Development
Introducing Customer: Voxel Voxel is a global leader in workplace safety, empowering worksites by providing them with the data they need to protect workers and gain insight into workplace activities. Their mission is to protect the people who power our world. We spoke with Anurag Kanungo, the co-founder and CTO, about why he decided to transition to Encord to manage their machine learning pipeline and computer vision projects. Problem: Operational Challenges in Data Accessibility and Model Scalability As Voxel grew, they encountered several challenges that hampered their ability to deliver on their mission effectively. The initial approach to data gathering and analysis wasn't sufficient for scale, leading to difficulties in finding relevant data and a lack of dataset diversity. The frequent changes in work environments, such as uniform updates, posed challenges in accurately updating models with new, unseen data. Also, addressing model edge cases and efficiently scaling the data labeling and analysis process became a prominent issue. Initially, Voxel trained pipelines using open-source tooling for object detection in videos. While sufficient on a small scale, as Voxel grew and required more complexity, the limitations of these tools became evident. Among others, they faced challenges with the user interface, backend data management, interpolation issues, and label exports. Despite being a good starting point, these tools proved inadequate for scaling operations effectively. “…as we started growing and adding more customers and more people using the tool there were certainly a bunch of challenges that came in, like our previous OS labeling tool kept running out of disk, so we had to start doing maintenance ourselves. We had to start editing the code and diverging from the main branch, which we really didn’t want to do…because we wanted to focus on our product.” - Anurag Kanungo As Voxel scaled, they sought a more robust solution that had critical features such as video support and image classification. Solution: Transitioning to Encord for Scalable and Efficient Video Analysis The decision to transition to Encord marked a significant turning point for Voxel. Encord's video-first approach addressed their need for robust video support, while its innovative features, such as image group classification, stood out. Moreover, Encord's exceptional support and technical design resonated with Voxel's needs, offering a seamless and efficient solution that aligned perfectly with their vision for enhancing workplace safety. "We went through a bunch of vendors and one of the things that stood out about Encord was the video first support, which other vendors do not have. Specifically understanding how the video works behind the scenes: the encoding, the frame indexes and square pixel ratios."- Anurag Kanungo Results: Impact of Encord on Voxel’s Operations One of the key requirements for Voxel was the ability to integrate their existing data pipelines into a new solution, which Encord was able to provide seamlessly. This enabled their team to continue to focus on their end solution without being preoccupied with the handover. Voxel were impressed by the robustness of the platform, enabling them to utilise many of the advanced features enabling them to address the safety issues and ergonomic concerns more effectively, aligning with their overarching mission to reduce workplace risks and ensure a safer environment for all workers Overall, the adoption of Encord has significantly aided Voxel's approach to workplace safety and efficiency. The platform's integration and its capabilities have empowered Voxel to address safety concerns and optimize operations effectively. With Encord's ongoing support, Voxel is well-equipped to navigate future challenges and drive innovation in workplace safety, setting new standards for operational excellence.
Physical AI
Logistics
Human safety
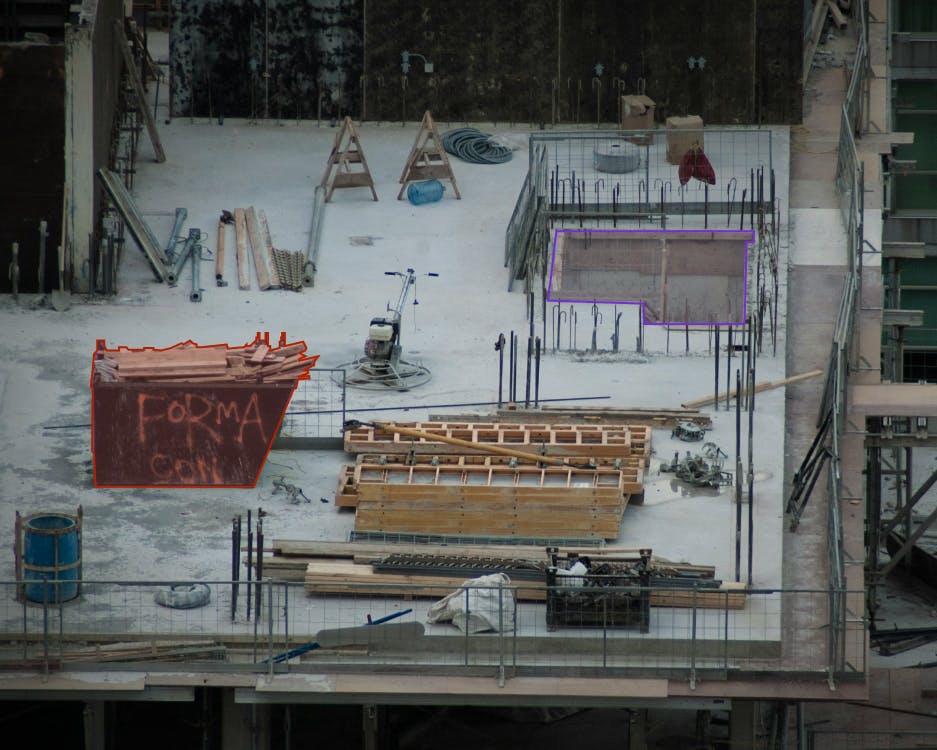
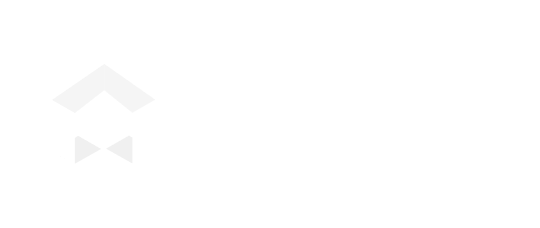
Improving Efficiency in the Construction Industry with Encord
CONXAI is building an AI platform for the Architecture, Engineering and Construction industries to contextualise different data and transform them into actionable insights. CONXAI, however, encountered challenges with optimizing datasets, reviewing labels, and managing large volumes of data with their in-house data annotation solution. This is where Encord came in - CONXAI was looking for an end-to-end solution for data management and curation, annotation, and evaluation. Introducing Customer: CONXAI CONXAI’s goal is to help AEC teams perform better by organizing and making sense of the vast amount of data generated during different stages of construction projects. They specialize in making data more useful, especially since a lot of project data often goes unused. Their ultimate aim is to help AEC professionals use AI effectively to improve efficiency and tackle challenges in their projects. We sat down with Markus Kittel, AI Product Development Manager at CONXAI, to discuss his work overseeing the product roadmap, and their exciting plans ahead for the business. Problem: Challenges in Data Curation and Management CONXAI's approach involves working with large unstructured datasets, which leads to challenges in effectively managing and curating project data. Their initial reliance on their in-house solution for data annotation proved to be problematic as the volume of data increased. Like many in-house tools, it was prone to frequent malfunctions, obscured the data it processed, and lacked mechanisms for reviewing annotations. Additionally, scalability was a major concern, as the in-house tool struggled to handle the increasing volume and complexity of project data. Without a reliable and scalable data management system in place, they faced difficulties in optimizing datasets and analyzing data effectively. As a result, CONXAI recognized the pressing need for a comprehensive solution that could streamline its data curation and management processes, enabling it to unlock the full potential of AI-driven insights within the AEC industry. CONXAI were also in need of a solution where data security took precedence, enabling data to remain within CONXAI servers and be accessed via an API or SDK. Solution: Encord Provides a Unified Platform for Data Curation and Management “With other labeling tools, we needed to integrate another tool for data management and exploration capabilities, but Encord combined the two needs and provided a single comprehensive solution, along with excellent customer care and support,” Markus says. To address these challenges, CONXAI explored various annotation tools. They were searching for a single platform that could handle data curation and management seamlessly. Encord's Annotate and Encord Active emerged as the ideal solution, offering a comprehensive platform to streamline CONXAI’s operations. As Markus says “We connect Encord Active with our large dataset and then use metrics to prioritize building a collection of images. This collection is then sent to Encord Annotate for labeling images in preparation for training. And all this without the data leaving our server.” Result: 60% Increase in Labeling Speed With the adoption of Encord into the data pipeline, CONXAI witnessed significant improvements in its data management processes. Encord facilitated the transformation of unstructured data into labeled, training-ready, datasets. The intuitive interface of Encord's Annotate tool simplified the annotation process for CONXAI's team, while also providing robust label review capabilities. Moreover, Encord's Active platform allowed CONXAI to efficiently curate and evaluate their datasets. “The labeling speed of the annotation team improved to almost 60% compared to when using their in-house tool.” - Markus Kittel CONXAI was able to curate over 40k images with Encord Active. They were then able to efficiently evaluate and prioritize these images based on metrics, facilitating streamlined data management and enhanced decision-making processes within their operations. CONXAI were able to contribute to Encord’s product roadmap by identifying that mapping relationships between labels in their ontology would enhance model performance. The Encord team were able to deploy this functionality, resulting in an improved user experience for CONXAI. Overall, using Encord led to enhanced robustness, simplified data pipelines, and a remarkable 60% increase in labeling speed compared to CONXAI's previous in-house tool. This demonstrates how adopting an end-to-end platform with annotation, curation, and evaluation capabilities provides the best solution for computer vision teams.
Logistics
Human safety
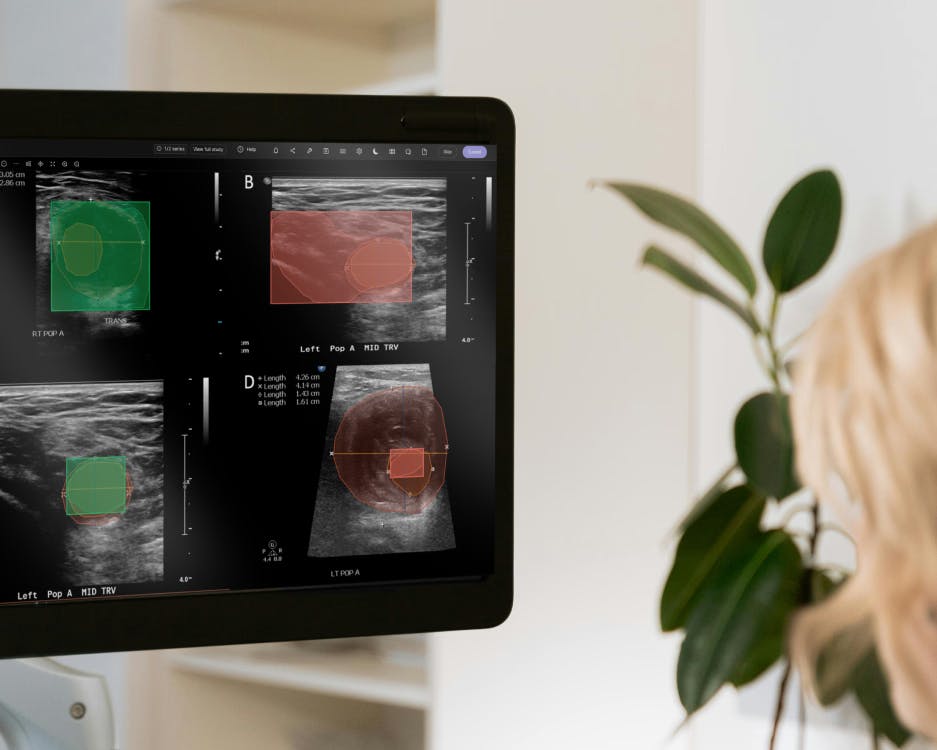
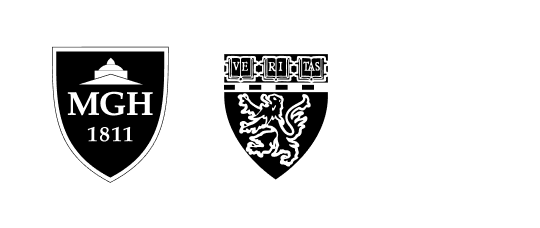
How Harvard Medical School and MGH Cut Down Annotation Time and Model Errors with Encord
Introduction A new paper published in MDPI (Multidisciplinary Digital Publishing Institute) demonstrates how, using the Encord platform, researchers at Harvard Medical School, Massachusetts General Hospital, and Brigham and Women’s Hospital were able to reduce vascular ultrasound annotation time from days to minutes and run automated analyses of their datasets. Using Encord, the team was able to: Create their first segmentation models by labeling only a handful of images Cut annotation time through segmentation models by an order of magnitude Visually explore their dataset and identify problematic areas - in their case, the impact of blur on their dataset Evaluate the performance of their segmentation models in the Encord platform Problem: DUS Image Annotation is Resource-Intensive and Prone to Human Error Medical imaging, particularly Arterial Duplex Ultrasound (DUS), plays a crucial role in diagnosing and managing vascular diseases like Popliteal Artery Aneurysms (PAAs). The traditional method of analyzing DUS images relies heavily on manual annotation by skilled medical professionals. This process is fraught with challenges: Time-consuming—especially with the growing volume of medical imaging data. Prone to human error. Heavily dependent on expertise and experience - furthering how resource-intensive the process becomes The subjective nature of manual annotations can lead to inconsistent measurements and interpretations due to inter- and intra-observer variability during annotation. This raises concerns about the reliability and reproducibility of the results and could impact the accuracy of diagnoses and treatment plans for patients. The primary issue in this research paper lies in precisely annotating the inner and outer lumens of the artery in images - a critical step for accurate measurement and subsequent treatment planning. Solution: Encord Annotate to Auto-Label DUS Images and Encord Active to Validate Model Performance The study tested the feasibility of the Encord platform to create an automated model that segments the inner and outer lumen within PAA DUS. Using image segmentation to find the largest diameter and thrombus area within PAAs helped standardize DUS measurements that are important for making decisions about surgery. Using Encord Annotate for Automated Annotation The researchers collected and prepared (deidentification and extraction) a dataset comprising DUS images of PAAs for upload to Encord before annotating a few images to serve as ground truth for the annotation models using Encord Annotate. Using Encord Annotate’s automated labeling feature, they could generate segmentation masks for unlabeled images. This reduced the time and effort required for DUS image analysis while minimizing the potential for human error. Using Encord Active to Select the Best-Performing Model They trained three models and validated them with Encord Active on the annotated images (20, 60, and 80 sets). Encord Active enabled the researchers to understand the performance metrics that helped them select the best model for segmenting the inner and outer lumens of the popliteal artery with high precision. After training models on image subsets, we tested them within the Encord platform. We selected the desired tests in the analysis tab of the project, and after a runtime period, the platform presented calculations of true positives, false negatives, mAP, IoU, and blur. The report referenced Encord’s ability to seamlessly integrate into clinical processes with a user-friendly interface, simple onboarding, and rapid annotation workflows as crucial to the study's success. For healthcare practitioners who use the platform, this improves their diagnostic process without disrupting established procedures. Results Encord Reduced Annotation Time from Days to Minutes Where manual annotation could take several minutes per image, the researchers accomplished the task in a fraction of the time using Encord. Their workflow went from relying on RPVI-certified physicians manually annotating DUS images, a process that took several days, to using Encord to annotate a few images, train models, and auto-label unlabeled images in minutes. This efficiency proves crucial in clinical settings, where timely diagnosis and treatment decisions can significantly impact patient outcomes. Figure 1. AI segmentation classifications on duplex ultrasound images. (A) Outer polygon true-positive classification, where the color green indicates a correct segmentation. (B) Outer polygon false-positive classification, where red indicates an incorrect segmentation. (C) Inner polygon true-positive classification, where the color green indicates a correct segmentation. (D) Inner polygon false-positive classification, where red indicates an incorrect segmentation. Better Evaluation and Observability of Model Performance with Encord Active The researchers quantitatively assessed the performance of the three models with Encord Active providing analytics on the following metrics: mean Average Precision (mAP). Intersection over Union (IoU). True Positive Rate (TPR). Encord Active calculated the outer polygon's mAP to be 0.85 for the 20-image model, 0.06 for the 60-image model, and 0 for the 80-image model. The mAP of the inner polygon was 0.23 for the 20-image, 60-image, and 80-image models. The true-positive rate (TPR) for the inner polygon remained at 0.23. See the full results in the table below: “With regard to the models for outer and inner polygons, the outer polygon model outperformed the inner polygon model on every metric. The outer polygon demonstrated almost equal precision and recall at 0.85. The mAP for the outer polygon model was 0.85 with a true-positive rate of 0.86, which is comparable to other clinically used high-performing models for US segmentation.” With Encord Active automatically providing model evaluation analytics, the team instantly discovered the model's strengths and weaknesses. For every model they trained, Active provided breakdowns and graphs on its performance, including the ability to visually explore the regions the model incorrectly segmented vs. the ground truth. Encord Active Uncovered Blurry DUS Images that Could Degrade Annotation Model Performance The researchers used Encord Active to explore the model's performance depending on the blur level, allowing them to visually interact with varying levels of blur in their dataset to understand how this impacted model performance. The paper states, “Intuitively, our analysis found that as the images became blurrier, the model precision declined, and false-negative rates increased... Removing blur from—or augmenting—blur in images can be important for training accurate AI models.” Conclusion In summary, the platform’s intuitive navigation, complemented by tutorials for both model training and analysis, allowed for straightforward operationalization of the model training system among members of the research team. The results were displayed in an understandable format and interpreted within the following discussion. The findings have far-reaching consequences for medical imaging and diagnosis. The researchers greatly improved the accuracy, reliability, and efficiency of DUS image analysis by auto-annotating images with Encord Annotate and validating annotation models with Encord Active. This could result in potentially better patient care, treatment planning, and diagnostic procedures. At Encord, we are committed to continually providing healthcare practitioners and physicians with the data-centric AI platform they need to improve their medical imaging and analysis workflows. We’re proud of the work the researchers were able to accomplish and how Encord is paving the way for broader applications of AI in various aspects of medical diagnostics. 📑 Read the full paper on MDPI (Multidisciplinary Digital Publishing Institute).
Healthcare
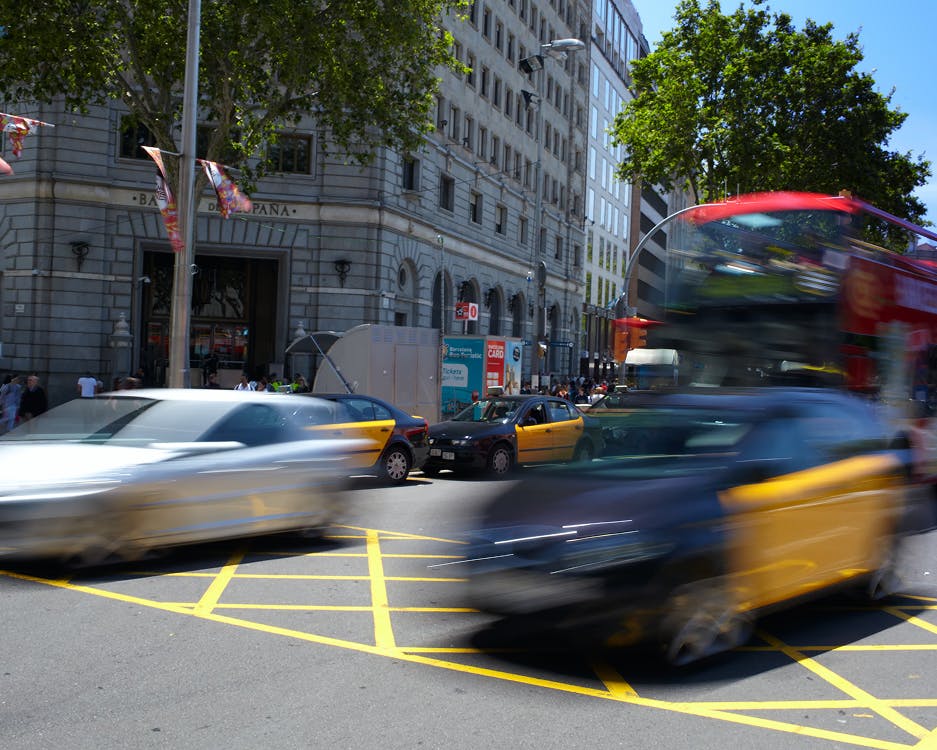
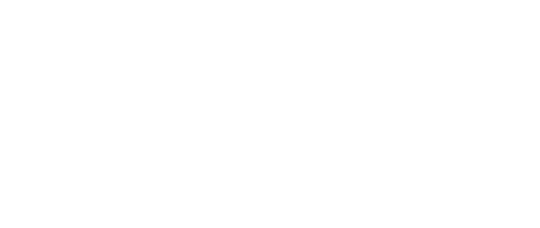
Boosting Last-mile Model Performance: Increase mAP by 20% while reducing your dataset size by 25% with intelligent visual data curation
Introducing Customer: Automotus Automotus is leading the advancement of parking and traffic management solutions through the use of AI and computer vision technology. The platform serves cities and airports to enhance street safety, sustainability, and efficiency by automating curbside operations, including parking, loading zones, zero-emission areas, bike lanes, and more. Problem: Large volume of data, expensive to label Automotus faced challenges in managing their entire data pipeline for model development, including automating data curation, ensuring efficient AI-assisted labeling, and streamlining model evaluation processes. After first establishing a continuous annotation pipeline, the team faced a critical question: Among all the collected data, how could they ensure they were labeling the most relevant data? And, how would they identify such data? The large volumes of de-identified images captured from hundreds of cameras presented an abundance of data. Labeling all of it would not necessarily improve model performance and would be prohibitively expensive. Solution: Use Encord to manage data pipeline Upon evaluating other tools, the Automotus team partnered with Encord to manage their entire data pipeline for model development, from automated data curation to AI-assisted labeling to model evaluation. Here's a look at their entire workflow: One of the team’s first priorities was converting the vast amount of de-identified images into labeled training data. Results: 35% reduction in the datasets' size for annotation Leveraging Encord, the team was able to visually inspect, query, and sort their datasets to eliminate undesirable low-quality data in just a few clicks. This process resulted in a 35% reduction in the datasets' size for annotation. Consequently, the team achieved over a 33% reduction in their labeling costs. The high model accuracy enabled Automotus to better serve and grow with their customers – presenting more accurate data to their clients: “From the modality distribution that happens at the street-level, to more accurate representations of the dwell times [and a few other metrics that we supply to our clients] – these base models are the ones driving these analytics.” How does Encord compare to other tools on the market? The team evaluated several other tools on the market but ultimately decided to partner with Encord for several reasons: Flexible Ontology Structure: Encord facilitated efficient object classification and tracking, allowing for straightforward ontology management and seamless training of both detection and classification models within a single project. Quality Control Capabilities: Encord's human-in-the-loop feedback mechanisms significantly enhanced annotation performance, particularly in identifying small objects within frames. Automated Labeling Features: The ability to label a few frames and use assisted labeling to speed up the process, reducing the need for manual labeling of every frame, was highly advantageous. The Automotus AI team notes: “For example, a shortcoming with other tools was the quality of the labels: we’d occasionally realize bounding boxes would be tighter or too wide around the objects they were identifying, or objects wouldn’t be classified correctly within frames. Now, we can select the sampling rate of frames that we want to move towards a review process, and share real-time context with annotators so that they can also power our model performance. This human-in-the-loop approach means we can use Encord to help our annotators perform annotations better, which in turn speeds up how quickly we can improve our model performance. We are able to localize objects better and increase accuracy.”
Logistics
Smart cities
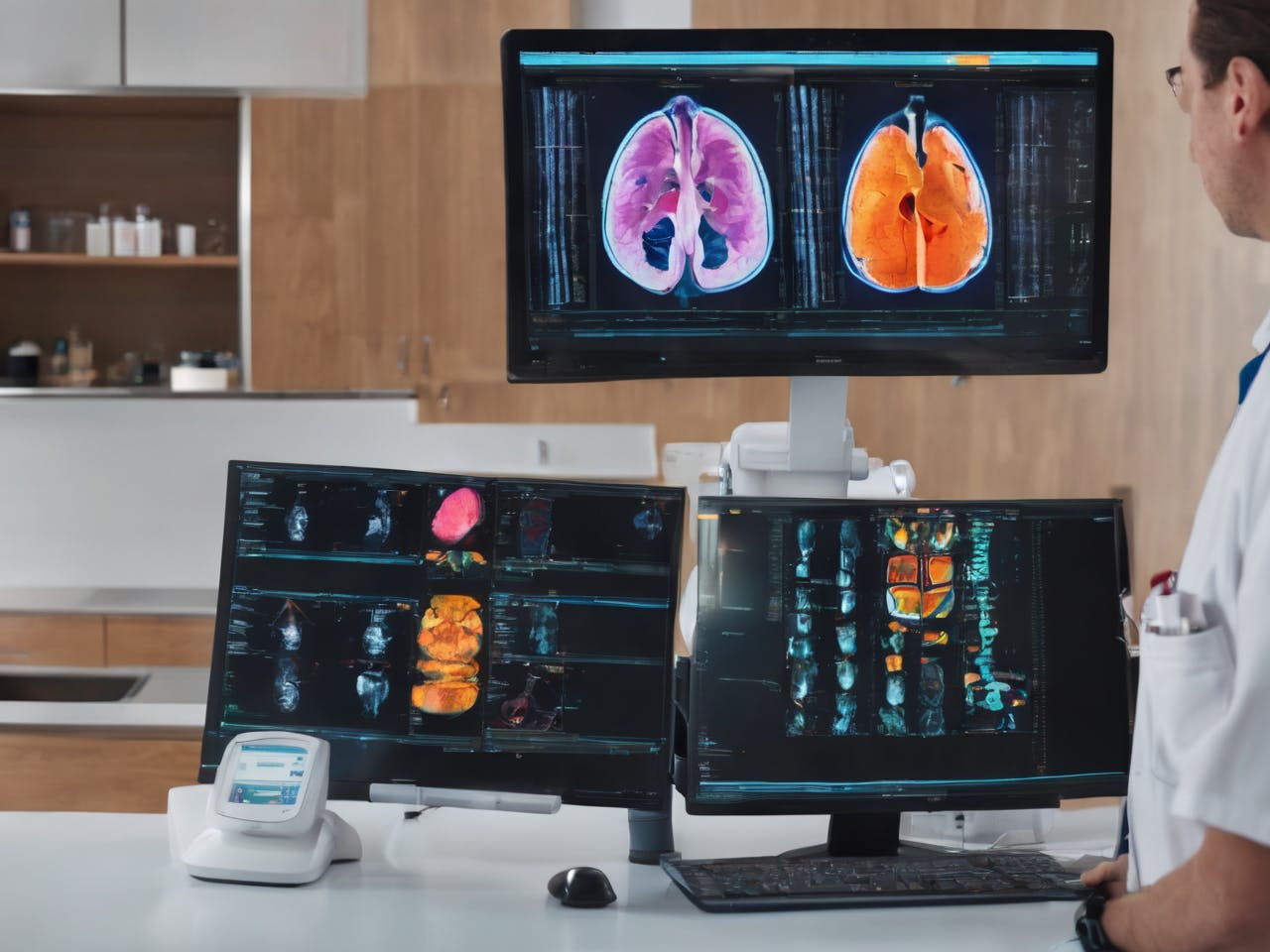
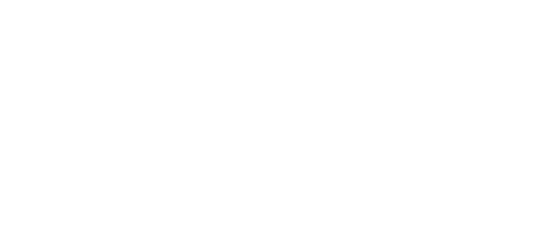
Accelerating Diagnosis to Treatment with Encord
Viz.ai’s revolutionary technology empowers medical teams to save lives through early detection and care coordination. The award-winning health tech company leverages Encord to accelerate processes, improve accuracy of diagnosis, and streamline collaboration among clinical AI teams. Encord’s medical training data platform and services have leveled up Viz.ai’s capabilities. They are now able to analyze data quickly and enter new areas of medical research. Introducing Customer: Viz.ai Viz.ai uses artificial intelligence to speed up care and improve patient outcomes. The San Francisco-based health tech company uses its software to ingest patient data and images in real-time and use regulatory-approved AI algorithms to detect disease and notify the appropriate medical team. This allows early detection of large vessel occlusion strokes, aneurysms, pulmonary embolism, and other critical diseases. It alerts doctors and nurses, who can see the images on their phones, saving hours of the time it would otherwise take to get that patient to diagnosis and treatment. Their slogan “time is brain” speaks to the heart of their mission. Every minute that passes during a stroke, 2 billion brain cells die, so early detection and accurate diagnosis are crucial. Viz.ai’s success in aiding medical teams to rapidly detect diseases has allowed them to help enhance and preserve the quality of patients’ lives. And, with more success, comes more responsibility. The healthcare tech company has been rapidly growing and is constantly developing more solutions to support clinical teams worldwide. Viz.ai is now expanding into cardiovascular diseases with a recent approval by the FDA of AI-powered software for hypertrophic cardiomyopathy, an often missed but potentially deadly condition. Problem: Time-consuming data comparison & need for team collaboration Early detection of a stroke, for instance, is possible thanks to Viz.ai’s abundance of data. By accessing and comparing scans with their multiple medical databases, the accuracy of their products is top of the range. However, for their teams, this has also translated into a lot of manpower and time consumed. Previously, the clinical AI teams at Viz.ai relied on an in-house annotation platform to analyze and label medical data obtained from outside resources. “However, it lacked several features to enhance and streamline team collaboration,” explains Maayan Gerbi, Clicinal AI Specialist. “We desired more automated annotation features to track progress amongst teams and lower maintenance.” It was time to search for a new solution that could support Viz.ai’s ambitious and fast-growing teams. Solution: Encord providing management & tracking for large volumes of data After considering multiple annotation solutions, it was clear that Encord was the best fit for their needs. What stood out for Viz.ai was Encord’s highly responsive team, top-notch annotation features, as well as the user-friendly interface of the platform. “Encord’s robust support system has been remarkable. Whenever questions or issues come up, they are always supportive and helpful. This ensures that our workflows remain uninterrupted,” said Maayan. Encord’s helpful and reliable team also facilitated a smooth integration. “The annotation platform is well designed for compatibility and interpretability. We were able to effectively align it with the current systems,” added Data Manager Sarit Meshesha. From project management, tracking, and monitoring to organizing large volumes of data in a user-friendly manner, Encord’s capabilities empower teams to annotate more speedily and accurately. Viz.ai particularly highlights the interpolation tool. “Encord constantly impresses us with the ability to think outside the box,” says Maayan. “The interpolation tool is a sophisticated, semi-automatic labeling tool that has proven to be a significant time saver for our team by reducing labeling time and improving precision.” Results: Less manual work & higher-quality data Encord is now an integral part of Viz.ai’s workflow. The platform’s range of innovative features has allowed teams to take on more projects while staying organized. Additionally, the accuracy of the automatic and semi-automatic annotation features means less manual work and higher-quality data. The clinical AI teams can now expedite processes and review medical imaging more quickly. The collaborative features have also proven beneficial. Viz.ai now experiences more effective communication amongst teams and better project management end-to-end. Maayan explains, “The division of tasks and clear role assignment ensures organized workflows and the ability for team members to consult certain cases with experts along the way.” Thanks to the benefits that Viz.ai has witnessed with Encord, they now envision the platform as key to their growth and ever-challenging needs. “We plan on leveraging Encord to expand the range of use cases and include more medical conditions in our solutions… We are excited about our potential with Encord and look forward to seeing how we will evolve together in the future.”
Healthcare
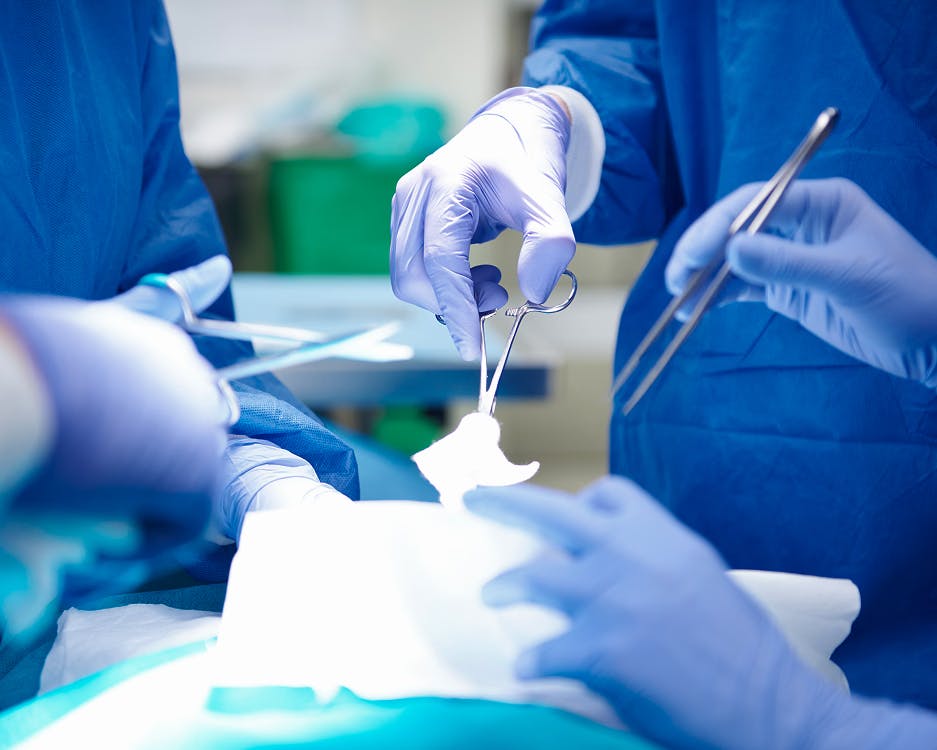
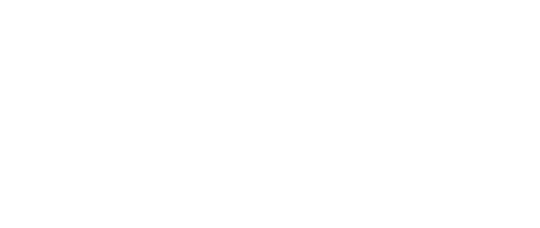
How Surgical Data Science Collective (SDSC) Conducted Video Annotation 10x Faster
Surgical Data Science Collective (SDSC) is a data platform that provides surgeons with access to data and quantitative insights about procedures to expedite the training process and democratize access to safe surgery. Working with Encord, SDSC has increased the speed of annotations by 10x while simultaneously improving precision and accuracy. Introducing Customer: SDSC SDSC is a non-profit organization dedicated to transforming surgery from an art to a science. With five core products, SDSC provides essential metrics on various procedures once videos are uploaded to the platform. For instance, the Kinematics model captures the movement of specific tools during a surgical procedure. As Director of Machine Learning (ML), Margaux Masson-Forsythe is responsible for leading the ML roadmap at SDSC, defining the strategy of generating high-quality training data, managing the data pipeline, and overseeing the ML team. Problem: Vast amount of video data A vast amount of video data requires technical knowledge for annotation and a need to connect their training data platform to a wider pipeline. Before switching to Encord, the SDSC team faced three common problems: quantity of data, poor quality of annotations, and a lack of customizability and integrations. Firstly, they faced a challenge in dealing with the vast amount of video data that required annotation. With each procedure split into 20 clips and each clip lasting approximately 15 minutes, the team had several Terabytes of data to annotate. Their previous tool suffered from a lot of latency issues when rendering videos, which hindered the labelers’ ability to effectively annotate at speed. Secondly, the team discovered that around 20% of the annotations they had previously conducted were incorrect, with most of these coming in the form of inconsistent naming conventions on the same objects. Using Encord Active’s automated label error detection feature, the team could identify these errors that they attributed to: i) the absence of a robust annotation toolkit and ii) the requirement for a high level of technical knowledge and expertise to conduct and review annotations. Lastly, the team had difficulty programmatically interacting with their training data platform and integrating it into their wider model production pipeline. They needed a working and usable Python SDK to create automated training data pipelines. Solution: Training Data Platform allows for video annotations Leveraging Encord’s comprehensive Training Data Platform to conduct video annotations with state-of-the-art tools and unparalleled support. After reviewing several solutions, the SDSC team chose to integrate Encord into their data pipelines. On the onboarding process, Margaux noted “Getting started with Encord and integrating it into our workflow was really fast. The thing that I find the most valuable is the flexibility of how we can integrate the Encord pipeline into our own pipeline, we use the Python SDK a lot”. By natively rendering videos in the Encord platform, SDSC’s team was able to speed up annotation while increasing precision. Margaux praised the platform's support for video annotation, noting “How smooth [Encord Annotate] was and all of the different tools that come with labeling videos” and that “[Encord] definitely was the best platform we’ve seen and we were looking around different platforms”. In order to solve their issues with incorporating expert review into their annotation workflows, the team used Encord Annotate's workflows to automate review by their labeling manager. Margaux explained that with Encord “We have a better reviewing system [...] that is the key component to having better quality datasets that we were missing before”. This allowed the annotators to get up to speed with complex annotations a lot quicker, without requiring experts to conduct annotations themselves. Margaux praised Encord's analytics capabilities, noting that “Now I have this whole system where I get analytics from Encord and we’re going to populate that into a dashboard so we can see how the annotation is going up”. She also appreciated how quick Encord was to incorporate Meta’s Segment Anything Model (SAM) into the platform, stating “One feature that made me go with Encord was the integration of SAM in the [Encord Annotate] platform which was done really quickly after the model was released so I knew when there was a new computer vision model released it will be integrated into the platform quite fast - which is something that was also a really good point”. Results: 10x faster annotation 10x faster annotation whilst moving towards 0% annotation errors (previously 20%) After integrating Encord into their wider data pipelines, SDSC was able to produce high-quality training data with quick annotations. With the help of Encord Active, the team identified that approximately 20% of the annotations completed on the previous tool were incorrect. The team is now “aiming to have 0% bad annotations” with the use of Encord’s platform. Margaux discussed an upcoming project where SDSC would be annotating 100 hours of procedures (20 procedures at 5 hours per procedure) in four months and she expressed confidence in their ability to complete the task with Encord, in conjunction with their wider Active Learning pipeline. According to Margaux,“... we know we can do that now with Encord because of the whole process that we have, which compared to what we had before, it would be maybe one procedure every two months even, much slower”. This represents a 10x increase in efficiency, as SDSC would have previously been able to annotate only around 10 hours (2 procedures) in the same time frame. As SDSC continues to grow and increase model production, they will further scale their use of Encord Annotate in addition to building out more mature Active Learning pipelines using Encord Active, given their initial success with the automated label error detection feature.
Healthcare
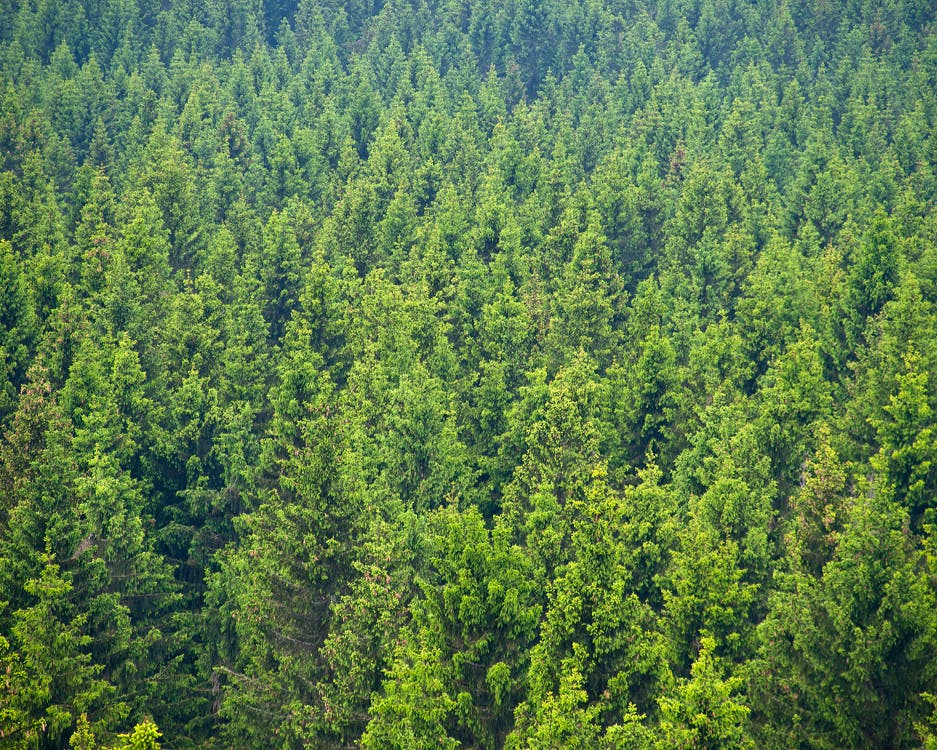
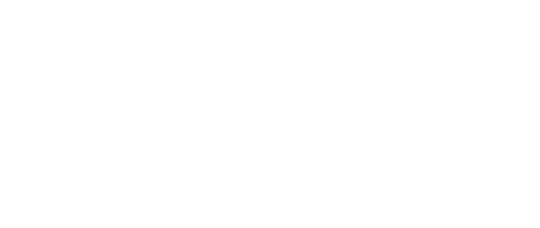
Accurately Measuring Carbon Content in Forests
Treeconomy uses Encord to collect reliable, granular data on trees. This is used to more accurately measure the carbon content in forests. More reliable carbon credit data bolsters Treeconomy’s reputation, allows the company to charge 50-150% more for carbon credits, and enables them to redirect more funds to reforestation efforts. Introducing Customer: Treecomony Treeconomy, an Earth Tech company, was created to better incentivize the planting of trees. It does so by accurately quantifying carbon captured and stored by trees (using sophisticated technology), packaging them as carbon offsets, and selling these high-quality carbon offsets to industries. Profits generated benefit landowners and incentivize them to preserve forests and plant trees. What sets Treeconomy apart from competitors is its use of world-class remote sensing, machine learning, and monitoring tools. This enables Treeconomy to more accurately measure carbon offsets and detect changes to a project location that could alter carbon levels. Doing so helps to assure investors that their nature-based carbon credits are real, that trees are really growing, and that the project is delivering on its impact claims. Problem: Time-consuming & error-prone to measure carbon forests Accurately measuring the amount of carbon in a forest is extremely important for carbon offsetting. Errors can lead to greenwashing and a loss of trust in the carbon market. As Treeconomy Co-Founder Robert Godfrey notes “Right now there are a number of companies being called out and caught with bad credits on their books, where projects have maybe grossly overestimated their climate impact.” Yet measuring carbon in forests is difficult. Traditionally, this was done by meticulously going from tree to tree with a tape measure and making broad extrapolations based on these measurements. The process was time-consuming and error-prone. Monitoring changes in the forest - like growth or deforestation - was similarly laborious. To address these challenges, Treeconomy explored open-source data and satellite imagery but found they had limitations. For example, satellite imagery still required them to manually count trees, a time-consuming and inefficient process. They created a computer vision algorithm to detect tree crowns from high-resolution drone and satellite data imagery but determined that larger and more relevant data sets were required to produce more accurate results. Solution: Encord provided labeling to train computer vision mdoel to count trees Treeconomy turned to Encord as an intuitive and flexible data annotation tool capable of labeling hundreds of images at once. The tool has enabled Treeconomy to train its computer vision algorithm to accurately count the number of trees in a given location. One of the big advantages of Encord for Treeconomy is its compatibility with Microsoft Azure and ease of use. This enabled the team to seamlessly integrate their existing data sets, thereby saving time and effort. Using Encord resulted in a positive discovery for Treeconomy: The tool could facilitate their future plans of labeling specific tree species - not just counting trees - and remove the need to hire staff for the task. Results: Sell offsets at premiums, from 50% to 150% above alternative options Encord has helped Treeconomy gather more accurate data and save time. As Godfrey explains, “Encord helps us to offer a ‘best in class’ capability for counting trees. It has helped us to improve the computer vision algorithm that allows us to delineate individual tree tops. [We were able] to count the entire forest in two minutes as opposed to two weeks.” By accurately quantifying trees in a forest, Treeconomy has been able to create more reliable carbon offsets. According to Godfrey, this has allowed the company to package and sell these offsets at premiums ranging from 50% to 150% above those of alternative options. This increased revenue is not only good for the company’s bottom line but is channeled back into the communities, benefiting landowners and supporting reforestation efforts. Ultimately, these actions reinforce Treeconomy's dedication to transforming sustainable land use into an economically rewarding and competitive venture.
Agriculture
Generative AI
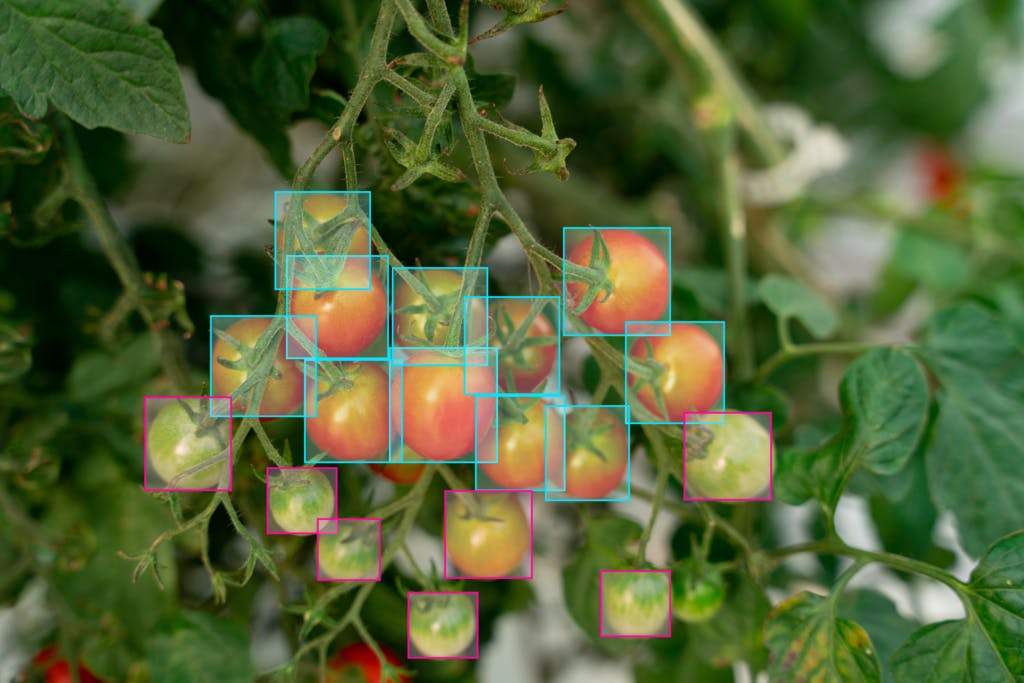
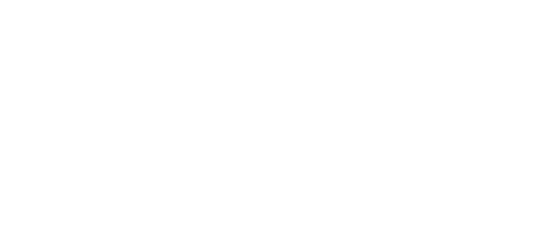
Transforming Fruit and Vegetable Harvesting & Analytics with Computer Vision
Automated harvesting and analytics company Four Growers uses Encord’s platform and annotators to help build its training datasets from scratch labeling millions of instances of greenhouses and plants. So far, Encord has halved the time it takes Four Growers to get its datasets in working order. Customer: Meet Four Growers Four Growers was founded to provide healthy, affordable, local produce by reducing the production costs of greenhouse growers through autonomous harvesting robots. The GR-100 robot has a robotic arm, calibrated by four stereo cameras that allow it to precisely detect and harvest produce using computer vision, focusing on tomatoes initially. Now in its second iteration, the GR-100 also offers heat mapping and yield forecasting abilities, as well as patented packing technology. By automating the harvesting process, Four Growers hopes to minimize food waste, increase quality, and contribute to making produce more affordable for everyone. Problem: Insufficient Access to Annotated Datasets Hinders Development of Automated Harvesting Models Currently, commercial greenhouses are expansive and it is impossible for manual pickers to get all of the ripe fruits on time. Over time, this leads to a large quantity of fruit just rotting in the greenhouse. Hoping to expedite this process, Four Growers set about developing their harvesting and analytics robot. But when it came to training its deep learning models for automated harvesting, Four Growers found that while there is a lot of awareness around growing in greenhouses, there was a distinct lack of publicly available data sets to draw from. This meant that the company had to build its own datasets from scratch, initially employing a number of freelance contractors to manually annotate all of the data, which consisted mostly of images of greenhouses and tomato plants. This process was incredibly time-consuming and manual. By having multiple external annotators using various tools, the Four Growers team had to often deal with multiple different annotation platforms at once, consolidating the data into one succinct database at a later stage. For Mira Murali, Computer Vision Engineer at Four Growers, this manual approach was eating into her schedule every week taking as long as 10 hours to manually review and condense data from multiple annotators that came through in various formats. However, despite the huge amount of time, it was taking to have the data manually labeled and then verified by the team at Four Growers, it felt like a necessary task given the importance of having clean accurate data to begin with. “You can tweak your models all you want but if your data at the beginning is wrong, then no matter how good your model is, you won’t get the end resource that you want,” Mira says. Solution: Encord Annotate for automated labeling and a team of annotators The team at Four Growers needed a data annotation tool that could handle the labeling of millions of object instances at the same time and this is where Encord came into the mix. Initially, the team was attracted to Encord because not only did the platform offer automatic annotating, but it also allowed Four Growers to work with an in-house team of human annotators. So rather than having different freelance contractors using different systems that had to be later consolidated, this streamlined approach instantly gave the Four Growers team time back in their days to focus on innovating their product and growing the business. “The fact that Encord was able to offer both an automatic labeling tool as well as a team of annotators that we could use was very attractive. We need that flexibility at this stage in our journey,” says Murali. Encord Active is another tool beginning to be utilized by the Four Growers team. It is an open-source toolkit that can be used for testing, validating, and evaluating your models. This allows Four Growers to further segment their datasets and build on their predictive model, making the harvesting even more precise. Results: Significant Time Reduction and Streamlined Annotation Process Achieved with Encord's Platform For Four Growers, a fast-growing and lean business, the biggest win with using Encord has been the time saved. Now, instead of spending hours upon hours dealing with contractors and re-verifying all of that work, the team can simply upload their images to Encord’s platform, wait for a ping to say the annotation work is complete, and focus on their core jobs. Speaking about the experience with Encord’s platform, Murali says: “Using Encord has more than halved the time it takes us to provide feedback on imagery. What could have previously taken us 2 hours now takes approximately 30 minutes.” Four Growers currently works with a number of large commercial greenhouses located in the US, Canada, and the Netherlands. As the company continues to grow its business and expand into more regions and crops, the company plans to continue its journey with Encord, leaning into the data analysis aspect of the platform to further understand how the team can improve its model, further streamline harvesting and help reduce food waste.
Physical AI
Agriculture
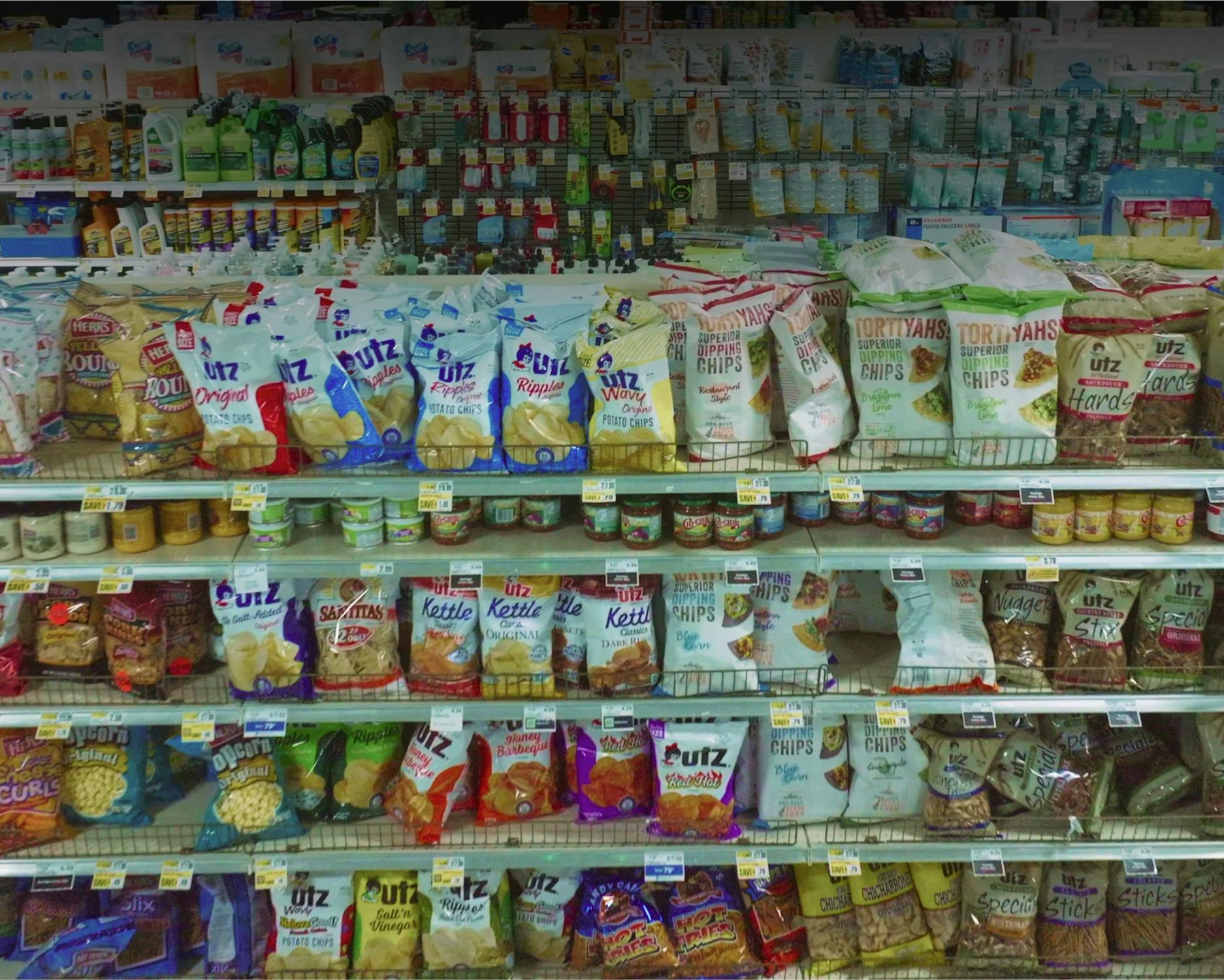
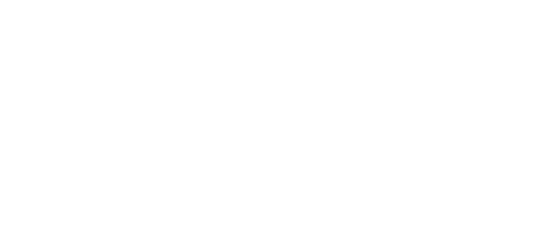
Improving Synthetic Data Generation with Encord Active
This is a guest report fully written by Neurolabs. You can read it to discover how Neurolabs is changing retail performance with synthetic computer vision and how Encord Active enhances its data generation efforts to improve model performance. Introducing Customer: Neurolabs At Neurolabs, we’re on a mission to revolutionize the world of in-store retail performance with our cutting-edge image recognition technology, ZIA. Our advanced technology empowers Consumer Packaged Goods (CPG) brands, Field Marketing Agencies, and Sales Force Automation companies to optimize retail execution, elevate the customer experience, and boost revenue growth. By building the most comprehensive 3D asset library for product recognition in the CPG industry, we are at the forefront of innovation. Our state-of-the-art synthetic image recognition technology sets new standards and drives remarkable results. With a steadfast commitment to a data-driven approach, we provide our customers with invaluable insights, enabling them to make informed decisions and outpace the competition. Problem: Training models with synthetic data to solve real-world problems Transferring knowledge trained on synthetic data to the real world remains a major challenge in computer vision. When evaluating our models using synthetic data, we noticed certain classes were underperforming compared to real-world data, so we set out to analyze the source of this discrepancy. By understanding the root cause, we hoped to improve our synthetic data generation process. Solution: Encord Active Quality Metrics and model failure modes By leveraging Encord Active and its Quality metrics, we gained a better understanding of our data and identified areas for improvement. In practice, we addressed issues for synthetic images of individual classes such as 3D object scaling, pose, image brightness, and blur. This methodical data-centric approach can be used to generate better synthetic data and improve model performance across various use-cases. Result: Improved overall last-mile performance by 2% and performance of edge-case class by 67% We started with implementing the recommendations for 1 of 6 low-performing classes we got based on Encord Active insights into our synthetic data generation pipeline. With this, we improved the per-class performance from 0% to 67% (AP50) and 0% to 100% (P1). The next steps are to implement changes for all low-performing classes in our dataset based on our insights. In this guest post, we will: Analyze our current model performance and identify gaps Analyze our training and test dataset using Encord Active Perform targeted improvements to our synthetic data to improve overall model performance and class-based performance. Let’s dive in! Analysing Current Model Performance This analysis was prompted by having a closer look at the per-class performance plots obtained when first creating benchmarks between our Unity renderer setup and our more recent UE5 data generation pipeline. When evaluating our models on real-world data, it revealed large performance drops for certain classes that performed well when using Unity. The overall performance plot, such as the ones below for AP50 and P@1, provides an insight into how a particular set of references perform. Transition unity to UE 💡Tip: You can read all about computer vision model performance and how to evaluate them here. If we inspect the per-class performance between two of our first use-case, we notice that there are classes with low performance. An example is the “Ariel + Revita Black” class Class example “Ariel + Revita Black” When we isolated the class performance we got an AP50 and P@1 of 0.0. With Encord Active’s functionality to analyze model performance based on quality metrics and its data visualization features, we can gain a better understanding of what causes this issue. AP50 when using UE5 References achieves 0.0 P@1 when using UE5 References achieves 0.0 Analysing Data Inputs The data we use during our evaluation stage can have a significant impact on our decisions regarding data generation and model improvements in the future. Gaining insight into the data in greater detail is essential for us as a team. We want to be well-informed about our experiments' findings, and why they may be the way they are so we can successfully implement improvements. By having a better comprehension of the data, we can move towards bettering our results with confidence. When it comes to data analysis, we leveraged Encord Active’s per-class performance plots. This enabled us to pinpoint areas of strength and areas for improvement. If we tackle the lowest-class performers in our dataset, we should be able to push the overall performance up. To do this, we should take a look at the individual data inputs to the evaluation stage to get a better understanding of what we are working with. Encord Active is a powerful tool, offering many features. In this article, we won’t provide an exhaustive list of all the features we utilized; instead, we will highlight a few of the most useful features and quality metrics that offered us beneficial information and actionable insights into our data. {light_callout_start}}💡Tip: read all about Quality Metrics and how to use them. Real-World Test Set — “Query set” We started our investigation by examining our real-world query set. This is the type of data we typically use to evaluate how well our model is performing when using some set of references — either synthetic, real, or mixed. The data here plays a vital role in the evaluation phase, and we need to pay close attention to some key points: Do we have a balanced test set? Do we have outliers in our test set? Do we have issues with the annotations? Do we have duplicate samples? Using Encord Active, we got a better understanding of these points and more. To begin with, we generated a new project in Encord Active, using the test set for our first use case. Using the Data Quality feature, we visualized our data and interpreted classes of interest. First, we investigated the distribution of samples per class. Here, we identified six under-sampled classes, as illustrated in the image above. Gaining this insight enabled us to understand better whether lower performance on a per-class basis is related to fewer data samples for those particular classes. This would be a good indication for us to investigate ways to increase the number of samples for those particular classes in our test set that are underrepresented. At the top end of the scale, we can see that we are performing well in situations where we have a lot of samples, as it’s more forgiving when we make an incorrect prediction. In addition to finding the lowest-class performers, keeping an eye out for under-sampled classes is a good way to isolate problems. Overview of the query dataset from the Data Quality page. The Data quality summary can be accessed by going to Data Quality → Summary on the left pane. In the above figure, we can see several issues being raised in our query set. Our test set contains several outliers across different quality metrics. Image singularity, blur, and sharpness are the ones with the most severe outliers. Our test dataset contains a variety of different image resolutions ranging from 50x50 to 600x400. Next, we will explain how we analyzed the outliers from one of the quality metrics, Image Singularity: Image Singularity Outliers The Image Singularity quality metric enabled us to accurately spot unique images and duplicates in our query set. This was highly advantageous in the identification of low-quality data in our test set. To illustrate, the figure below displays several samples flagged as being unique in our test set. Unique samples In the figure above, we can see that several samples in our test set are cut off, occluded, blurry, and rotated in a more challenging way to predict. This was an unexpected benefit of our analysis, and we noted down and tagged these cases to investigate further how they could impact our performance. Ultimately, we aim to tackle these challenging scenarios to make our models more robust. However, if this is a frequent occurrence, it frames the problem in a manner that may reshape how we approach generating the data for particular classes of interest. To ensure accuracy, it was important to analyze other metrics, and Encord Active was a great tool for tagging and keeping track of any issues we encountered during our evaluation. This allowed us to gain a better understanding of the impact of using these datasets. Real-World Training Set — References We performed a data quality analysis similar to the above on the training set to understand why we may be getting much higher performance on real data compared to using synthetic data. Here, we visualized the Object Annotation Quality metric and took a closer look at samples from the training set that was classified as low quality. The Object Annotation Quality metric transforms polygons into bounding boxes, and an embedding for each bounding box is extracted. Then, these embeddings are compared with their neighbors. If the neighbors are annotated differently, then a low score is given to it. This analysis helped us better identify which particular classes have close associations with each other. Notice that the low-quality samples are classes of interest, and there may be a chance that these classes have similar-looking samples in the test set that wouldn’t appear in a synthetically trained model. An example of this can be seen below, where the image on the left is a sample from the real-world training set, and the sample on the right is from the test set. Notice how both objects of interest contain some string occluding them. This situation would not occur when using our current synthetic data. This means that for this particular sample, we may be underperforming on synthetic data compared to real, as we do not model the two similarly. The more samples of this kind that we include in our real-world training set, the more pronounced the impact will be. This divergence in performance between synthetic and real data is due to differences between the types of data used as references. UE5 Synthetic Data — References Referring back to this figure that shows a particular class of synthetic data underperforming relative to our Unity rendering pipeline, we aim to get a better understanding of the potential reasons behind this. AP50 when using UE5 References achieves 0.0 P@1 when using UE5 References achieves 0.0 💡Tip: This can be repeated for other classes that are performing worse than expected. Using Encord Active to isolate this particular class, we inspected the images using various quality metrics and noted several differences between the real samples and synthetic ones. Small synthetic images that do not match real-world images Rotated synthetic images that deviate from real-world images Dark synthetic images that do not match real-world images At first, we discovered a significant number of synthetic images that were really small in scale and did not resemble their real-world counterparts in terms of size. Object scaling is much smaller for this particular class compared to the real data. Secondly, we included rotations that deviate quite far from reality. These samples may be sub-optimal to include in the training set, as shown below, as they are unlikely to be encountered in the real world for our current use case. Rotations Lastly, we identified several samples with a much darker tone when compared to the real data. This can make it quite challenging to distinguish the object of interest from the background. Brightness metric Brightness, rotation, and scaling issues for this ariel_+_revita_black_allin1_pods_22_buc getting an AP50 of 0 and P@1 of 0. After addressing the above issues and re-generating the data for that class, we ended up with samples that looked much closer to the real-world data. We re-generated the data by looking at samples from the real-world references (training set) to avoid introducing additional bias in targeting performance specific to the real-world test set. The images on the left are synthetic with the changes, and the right is real-world samples. Left side synthetic examples with changes, right side real-world samples. As shown in the P@1 plot below, the blue bar shows the performance of using the updated samples for that class resulting in P@1 jumping from 0.85 to 0.891 and AP50 from 0.604 to 0.622. We also found that the previously problematic class that obtained a P@1 and AP50 of 0.0 subsequently increased. AP50 when using updated UE5 References results in 0.676 compared to 0.0 previously. P@1 when using updated UE5 results in a P@1 of 1.0 compared to 0.0 previously. Takeaways By taking a data-centric methodical approach and analyzing the data closely, we gained a better understanding of what type of synthetic data we needed to generate to achieve the highest performance from our models. Diving deep into the performance on a class-by-class basis allowed us to tackle some of the worst-performing classes and improve their performance based on insights from analysis of the datasets and models in Encord Active. Appendix We can extend the ideas above to better approach data generation on a different use case. For example, a second use case contains a proportion of the training and test set with blurry image samples for our classes of interest. By inspecting object scaling, poses, image brightness, and image blur, we can better generate synthetic data for the use-case. The updated synthetic data outperforms our models trained on real data. Left side Synthetic Data and right side Real-world data After blurring the images to the same degree as the real-world data and retraining our model, performance surpassed the same model trained on real-world data.
Model Evaluation
Retail & E-Commerce
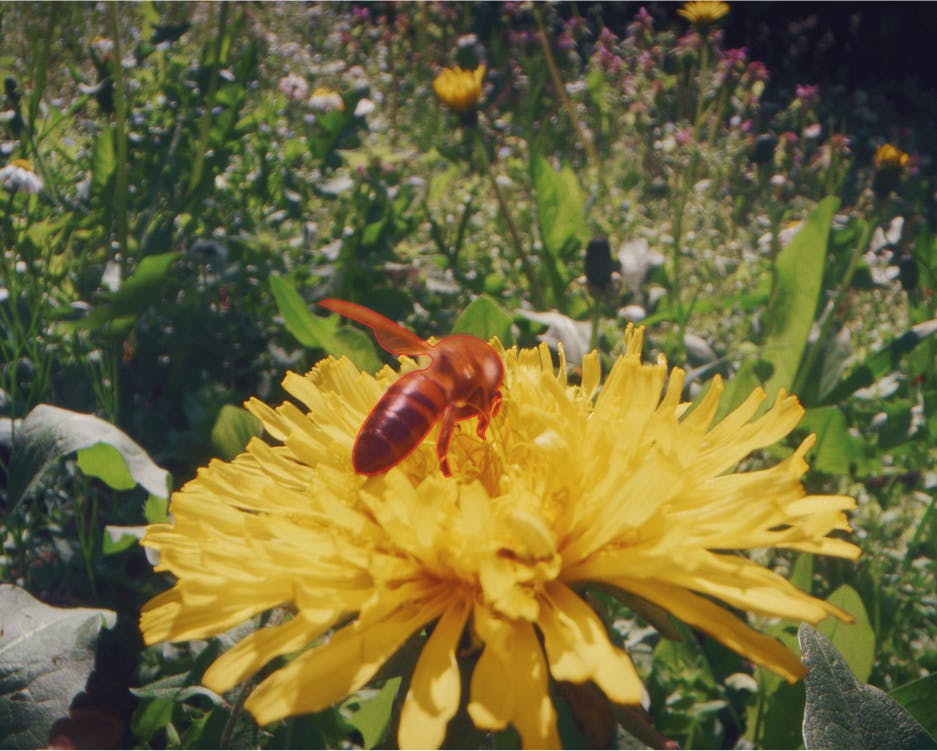
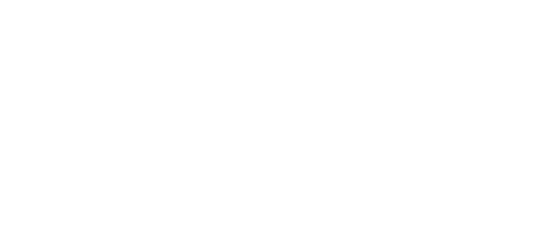
Saving the Honey Bees with Computer Vision
The Far Out Thinking Company, a UK based environmental R&D company that uses AI to combat pollinator decline, needed an accessible, affordable annotation tool to enable all members of their team to label images at speed. Using Encord’s platform, the team quickly annotated 4500 images of the European Hornet, Asian Hornet, and the Common Wasp and built a computer model to help distinguish between these different species and to confirm and detect the presence of Asian Hornets, an invasive species to the UK. Introducing Customer: Pollenize In late 2018, Pollenize, a U.K.-based pollinator conservation community interest company, launched its R&D arm The Far Out Thinking Company. The Far Out Thinking Company develops technology and AI solutions that help protect pollinators and preserve biodiversity. Pollinators, and in particular bees, play a crucial role in the viability of our plant and animal ecosystems. Bees are responsible for pollinating a large percentage of crop species. According to the Food and Agriculture Organization of the United Nations, bees pollinate around 75% of the world's food crops. However, bees are disappearing at a terrifying rate: 40 percent of insect pollinator species are facing extinction, with eight species of bees having been declared endangered. Working in tandem with Pollenize, The Far Out Thinking Company is developing technology that helps to better understand pollinator populations and their resourcing needs. By devising devices such as AI beehives, hornet identification boxes, and moth detectors, the Far Out Thinking Company helps determine gaps in flora, pinpoint resource-stressed areas, inform public rewilding strategies, identify invasive predators, and more. Problem: An Invasive Species Threatens the U.K.’s Honey Bee Population In 2003, the Asian Hornet arrived in Europe. An invasive species, the hornet has now spread throughout Europe, taking up the resources of natural pollinators and decimating the honey bee population. Asian hornets attack honey bees outside of beehives, a behavior known as “hawking,” biting off their heads and abdomens and taking their thoraxes back to their nests to feed their larva. Killing individual Asian Hornets won’t solve the problem. Local authorities need to destroy the nests, each of which can house from several hundred to several thousand individual hornets. Asian hornets haven’t yet naturalized in the U.K.; however, every year, they make their way into the country via shipping crates and the caravans of returning holidaymakers. Asian Hornets create their nests in April, and in October each nest produces an average of 14 reproductive individuals, so nests must be destroyed before October. Otherwise, the Asian Hornets will multiply rapidly. Since 2016, nine nests have been destroyed throughout the U.K.. However, local residents aren’t familiar with the look of this invasive species, and locating and destroying nests without a system for identifying hornets creates many challenges. Solution: Training Computer Vision Models to Identify Invasive Species and Protect Local Pollinators The Far Out Thinking Company developed the Asian Hornet ID Device. It uses computer vision to distinguish between local pollinators and Asian Hornets. With a network of these devices, port cities and areas of entry into the U.K. would no longer have to rely on individuals to identify Asian Hornets. Instead, the devices will provide an automated early detection system and prevent the hornets from naturalizing in the U.K. The Asian Hornet ID Device is situated in a box that contains a small reservoir of hornet attractant. After an Asian Hornet lands on the attractant, a camera located above the attractant observes it, and the computer vision model uses object detection to determine whether it is a common wasp, Asian Hornet, or European Hornet. If the AI system detects an Asian Hornet, it sends an alert to the Far Out Thinking Company, which contacts the authorities who put a radio tag on it. They then track the hornet back to its nest and destroy it. To build its computer vision model, The Far Out Thinking Company needed access to a data annotation tool that enabled multiple team members to label hundreds of images of pollinators at the same time. Only one member of the team has a computer science and computer vision background. The other members of the team found the open-source annotation tools alienating. Without a foundation in computer science or a background in data labeling, they couldn’t easily use the tools to assist in annotation despite having valuable expertise in entomology. An Encord employee familiar with the company’s work reached out, asking if they’d like to demo the product. “The platform was so intuitive. It provides a lot of different levels of control and team management. Everyone on the team could label and review images easily,” says Mathew Elmes, Director of Pollenize and The Far Out Thinking Company. “We could also do computation both on the cloud and locally on our deep learning machine. All those factors made it really advantageous for us, and I saw the opportunity to save a lot of time and costs by using Encord to speed up our model development.” Although Encord’s platform was initially designed for video annotation, the Encord team helped The Far Out Thinking Company string together a number of still images so that they could use the product to meet their specific annotation needs. “After they helped us put the images together, we pressed a couple of buttons, and the platform made a model for us,” says Elmes. “It was so easy.” Results: Accessible Annotating, New Projects, Pollinator Protection The Far Out Thinking Company’s team culled its training data iNaturalists, a crowdsourced image database. All of the images have been second certified to research grades. Using Encord, two members of the AI Hornet Device team annotated 1500 images of each pollinator class (4500 total) in just three hours. “We built separate pipelines for the three sets of images and labeled them accordingly. Then we trained and tested this small model. We got really good results, and it took more time to pull the images off the internet than it did to label them and build the model,” says Elmes. The Far Out Thinking Company has installed the first two of its Asian Hornet ID Devices on the Isle of Scilly, off the coast of Cornwall. They’re now working with Associated British Ports to build and install a device in Plymouth Port. The University of Plymouth has also helped them build an image scraper to speed up the process of gathering images, and the company is now building a larger model for its Asian Hornet ID Devices, training it on 1500 images of each pollinator class. “With Encord, myself and other team members can label the data ourselves. We have numerous projects that we want to achieve this year, and I think having an easy to use platform where we can all work together is going to help us move them forward quickly and successfully,” says Elmes. Beyond the Asian Hornet ID Device, The Far Out Thinking Company is using Encord in three other projects – one to determine the plant biodiversity in local fields, one to identify local moth diversity, and one to decode the honey bees’ waggle dances. All of these projects will help local pollinators survive by providing valuable information about the resourcing and health of the environment.
Agriculture
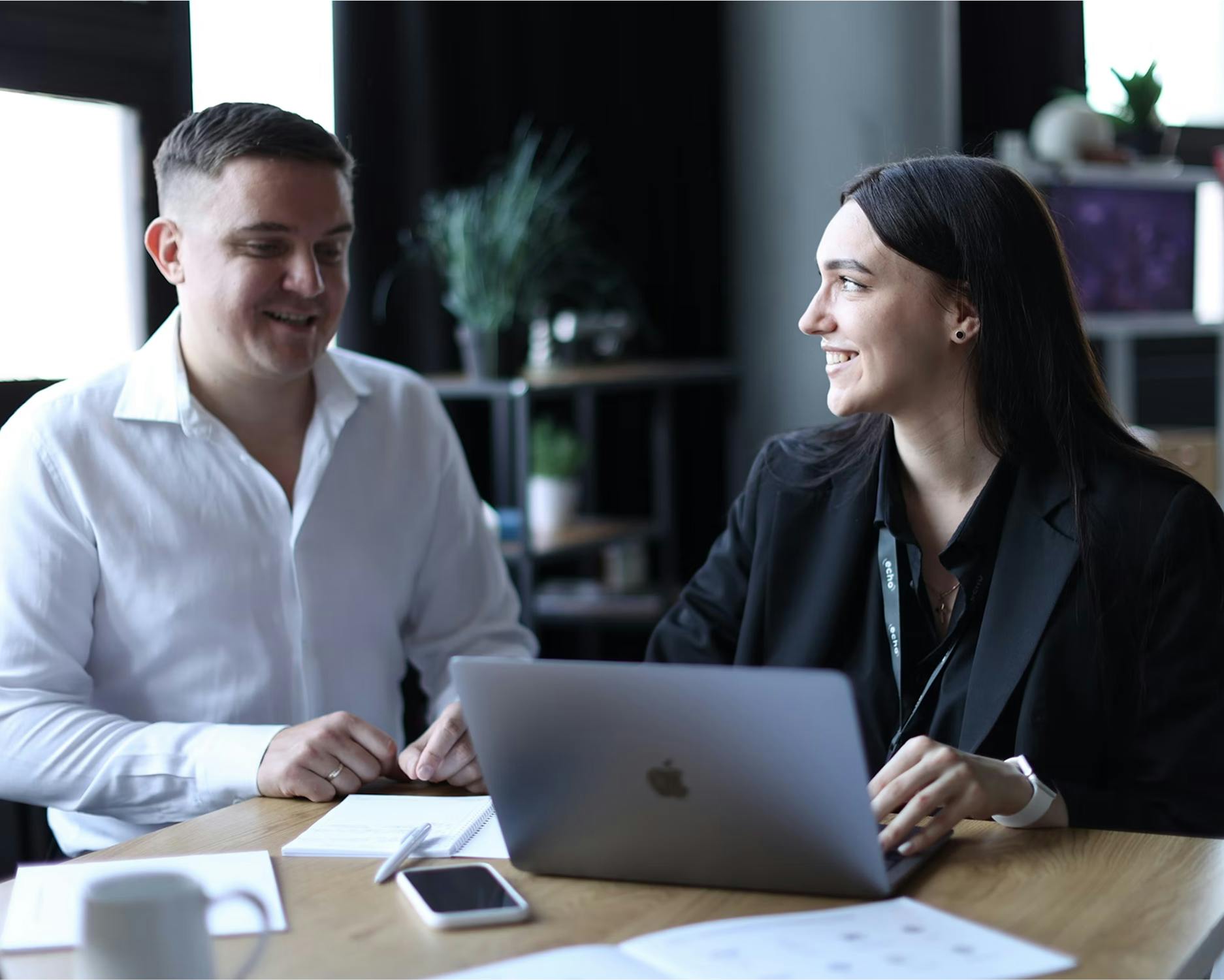
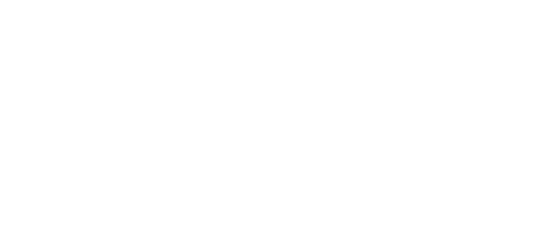
Building Multimodal AI Systems for Insurance with Tractable
Founded in 2014, Tractable uses AI technology to assist insurance providers in performing visual assessments of damaged assets. The company designs customizable machine learning models to serve insurance providers around the globe. As the complexity of data annotation projects grew, Tractable turned to Encord for a user-friendly platform that allowed for complex ontologies, annotator monitoring, and quality assurance. Introducing Customer: Tractable By analyzing customer images, Tractable quickly facilitates accurate damage appraisal, making the recovery from accidents 10 times faster and enabling people to move through the claims process quickly and efficiently after they’ve had an automobile accident. Tractable also offers products for property assessment, providing the same high-quality experience for incidents that involve property damage and thereby helping people to recover faster after a disaster strikes their home. Beyond accident recovery, Tractable’s technology can assist people throughout the entire lifecycle of owning a car or property. With Tractable, they can perform visual inspections before selling an asset, determine which parts of an asset to salvage or replace, obtain a condition report on a leased asset, and more. By using Tractable, insurance providers can free up employee time for high-value tasks, improve customer experiences, accelerate repairs, and increase recycling– all of which has a positive impact on people and the world they live in. Problem: Performing Quality Assurance When Scaling Up Projects with Complex Ontologies When building their first models for image classification, Tractable’s team designed and used internal tools for data annotation. However, as Tractable grew their product offerings, the annotation tasks became more complicated, and the team needed a training data platform that supported segmentation. Building one in-house would be costly and time consuming. Tractable’s team tried a few third-party platforms, but as the projects scaled and grew in sophistication– moving towards more pixel-level annotations with more complex ontologies– they found that many of these platforms had limitations, especially when it came to quality assurance (QA) functionality. As Tractable began building its property assessment models, the remote annotation team began to grow rapidly and the need for improved data annotation workflows became more urgent. Ensuring the quality of both the data and its labels became increasingly important as annotation tasks grew in complexity and size. “As our ambition grew, we realized that the functionality of many of the tools we were using was quite limited,” says Camilla Gilchrist, Head of Operations at Tractable. “We had a lot of steps in our annotation workflows, with many different pipelines, and the platforms couldn’t handle that complexity. We also had a bottleneck around quality assurance. With image segmentation, you can’t do a quick agreement rate analysis and doing a manual quality assurance check on each piece of data isn’t feasible. We needed a far more efficient way to assess quality.” Tractable needed a training data platform that could incorporate the feedback of expert decision makers in the annotation process and provide QA functionality. Solution: A User-Friendly Training Data Platform with Quality Assurance and Annotator Review Functionality When annotating data for its models, Tractable needs to take into account expertise beyond visual observations. “Think about how motor engineers assess damage on a vehicle,” explains Camilla. “They don’t just look at the car: they open and close the doors, feel the body work, and much more. As much as possible, we incorporate that expertise into our models, which requires determining specific annotation criteria that’s often quite complex. We need an accessible training data platform that allows annotators to break these complex labeling tasks into smaller parts and provides quality assurance and annotation review functionality. Otherwise, annotation tasks quickly become overwhelming, and the risk of mistakes increases.” The higher the granularity of an annotation task, the greater the amount of detailed features that must be labeled in each piece of data. This level of detail increases the need for annotator monitoring and quality assurance. Unlike Tractable’s legacy training data platforms, Encord built out the QA functionality that the company needed, enabling Tractable to unlock the power of using granular annotation techniques at scale. At the same time, Encord’s user-friendly platform provided a level of control that allowed Tractable’s operations professionals to build out ontologies quickly. The other platforms that Tractable had used allowed for custom mixtures of segmentation and frame-level annotation, but they required users to implement these customizations programmatically. SageMaker, for instance, allows for users to create custom UIs and ontologies, but to do so Tractable’s engineering and research teams had to put in a lot of time and effort into delivering the tooling the company needed. Results: Improved Quality Control, Data Governance, and Annotator Training With Encord’s QA and annotation review features, Tractable has been able to train annotators efficiently, monitoring their performance and providing feedback along the way. Their remote annotation team has grown to over 40 people. “Managing QA workflows efficiently and having an overview of the entire annotation process has been so important for our success. The more manual that QA process is, the more the amount of work explodes as the annotation workforce scales,” explains Camilla. “It’s always about balancing speed and quality. A lot of platforms prioritize speed over quality or quality over speed. Encord speeds up annotation while still allowing for strong quality control.” Encord’s API also works with S3 servers as a primary integration, so Tractable can keep customer data on its own servers. Because Tractable operates globally, it has to adhere to different data governance rules depending on the region in which a customer is located. The other tools that Tractable tried didn’t prioritize S3 integrations, so when the API broke, Tractable had to wait for and nudge those platforms to fix it– a less-than-ideal situation for a global company. “Encord’s team has been very hands-on in delivering what we need to succeed,” says Camilla. “A lot of companies promise that level of commitment, but Encord actually delivers it. We receive excellent support. They build out new features quickly based on our feedback. With other platforms, we’ve had to troubleshoot problems on our own– even to the extent that we basically needed to build our own offline tooling to process the data we labeled using them! Encord has worked with us to find a solution for every challenge."
Insurance
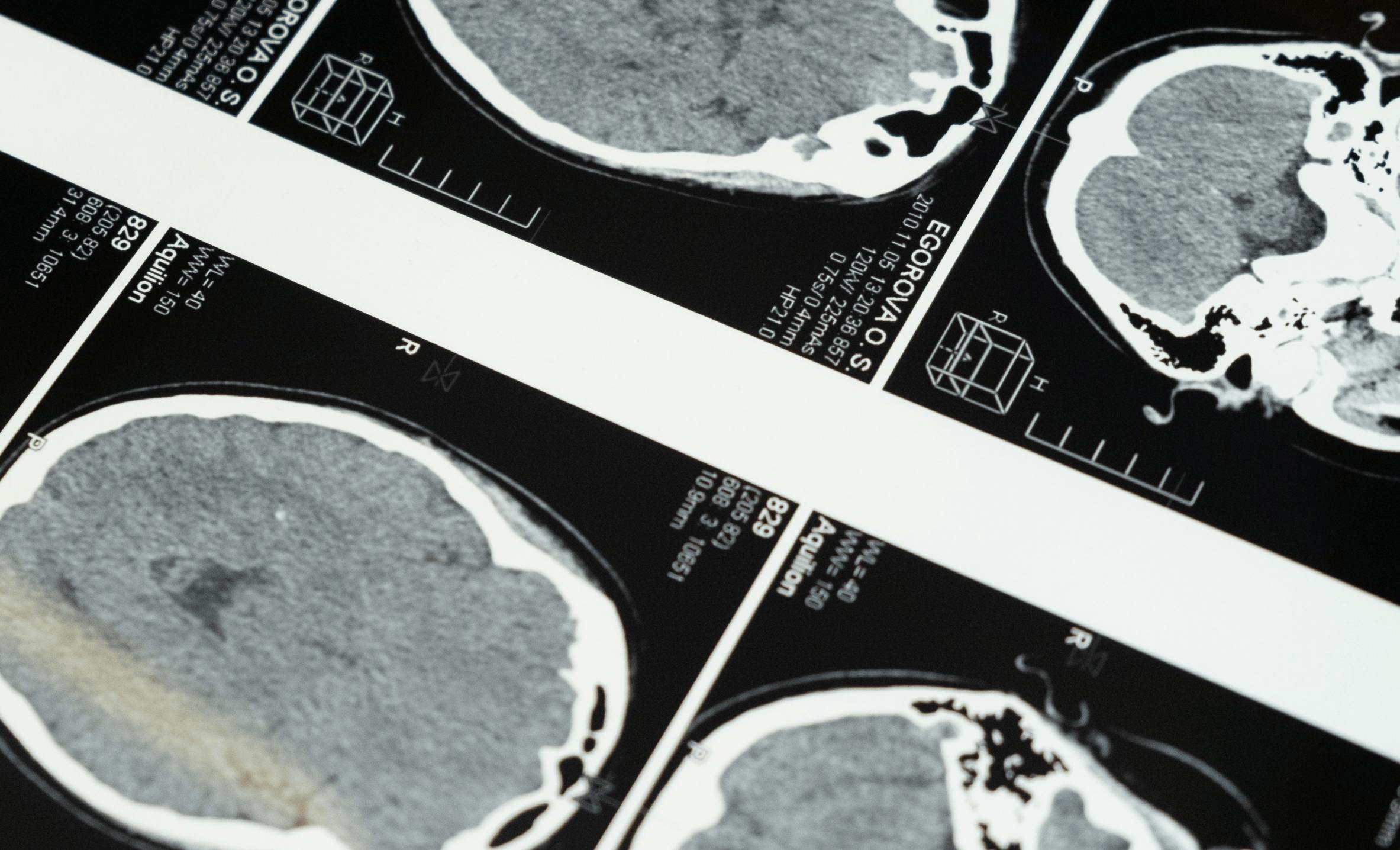
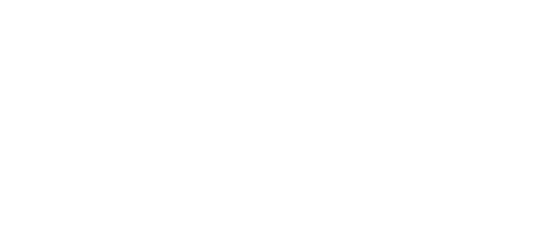
Reducing MRI and CT Annotation Time by 70% for Rapid AI
RapidAI develops AI that helps medical professionals identify and provide treatment for stroke victims. The company works with multiple annotators to label vast datasets of medical imaging. When managing the annotation and review process for large projects became unwieldy, the company turned to Encord’s platform for a seamless, centralized annotation experience. Introducing Customer: RapidAI For decades, doctors believed that only patients who sought care within a few hours of suffering a stroke would benefit from treatment. However, Dr. Greg Albers and Dr. Roland Bammer thought that with better technology, the window for care could be extended and more people with cerebrovascular disorders could be saved. When diagnosing stroke patients, doctors use perfusion and diffusion imaging. However, perfusion imaging is a complicated process: obtaining the results is time-consuming, and perfusion scans provide a lot of information that doctors must compile and analyze quickly. If doctors had more immediate access to a summary image that provided meaningful information, they could quickly identify patients who would benefit from care, extending the time frame for treatment. In 2012, Albers and Bammer founded RapidAI. Building on the technology they’d developed at Stanford University; the company provides brain imaging software tools designed to help doctors extract greater meaning from patient data. By creating high-quality images from diffusion and perfusion data, RapidAI’s technology assists neurovascular and vascular teams in making faster, more accurate diagnoses and treatment decisions. In support of the founding mission, RapidAI has analyzed more than 7 million scans. The company is the exclusive advanced neuroimaging partner for the World Stroke Organization, and today, more than 2,000 hospitals in 100-plus countries use the Rapid platform to diagnose cerebrovascular disorders, such as strokes and aneurysms. Problem: Managing Annotations for High-Volume Data Projects To train a model, RapidAI’s machine learning teams need thousands of labeled CT, CTA, MR, and MRA images. Each training project consists of about 500 cases, with between 30 and 200 CT image slices per case. If the case contains CTA scans, that number is closer to 1000 slices. RapidAI’s annotation team consists of many highly trained annotators – with several board-certified diagnostic radiologists and subspecialists acting as reviewers. Initially, open-source labeling tools were used. As the project grew in complexity and size, the need for a robust management system and a seamless export process became essential so the company began to look for an online platform that could help them manage the annotation and review process more efficiently. Solution: A Centralized Platform with Annotation Review and Interpolation Features RapidAI tried out several online annotation vendors when looking for an online platform to manage and label training data. “We went through the process of trying out each platform– uploading a test case and labeling a particular pathology,” says Dr. Ryan Mason, a neuroradiologist overseeing annotations at RapidAI. “Encord made it very easy to centrally keep track of annotations, including who had made them and who had reviewed them. It also had this great interpolation tool which was especially useful for the projects we were working on.” For particular diseases, the shape of the pathology changes substantially in the peripheral slices of a case. In the middle slices, however, it maintains a uniform shape. With Encord’s interpolation tool, when RapidAI’s annotators reach the middle slices, they only have to label one out of every five images. Encord’s DICOM annotation tool captures the shape of the pathology, interpolating the label on the slices the annotators skipped. In a case with 100 slices, annotators only have to label about 30 images. Encord’s reviewer function also helped RapidAI improve the efficiency of its annotation process. Using this feature, neuroradiologists can leave notes for annotators, correcting mistakes and providing guidance for improvement. They can also reject a case and label it directly. That way, annotators can then compare their original work against a reviewer’s to identify and learn from their makes. This feature is especially useful because as the team is gathering the labeled data they need, they are simultaneously teaching annotators to improve their labeling skills. Results: More Efficient Data Labeling Processes With Encord’s interpolation tool, Rapid AI’s team has increased the speed at which they can label data resulting in as much as a 30-minute reduction in annotation time per case. Encord and RapidAI’s team communicate directly on Slack, and should RapidAI encounter a bug or issue, Encord responds immediately and fixes it within a few hours. Encord’s team has also worked with them to customize the product to meet their annotation needs. “One of my favorite things about using Encord’s platform is how involved their team is with their customers,” says Mason. “For instance, when we started this project, I told them we liked to use Wacom tablets for drawing our annotations. Encord’s platform wasn’t set up for that, but their team asked to hop on a call so they could learn more. Within about two days, they released an update that made their platform compatible with the pads.” Rapid AI is in the process of expanding its technology to additional disease states, such as pulmonary embolisms. As they expand, the company will continue to build out a pipeline of projects that each require annotating thousands of MRI and CT scans. By working with a centralized platform that effectively manages the annotation process and speeds up labeling, the company can increase the speed at which its AI enters production and, ultimately, saves lives.
Healthcare
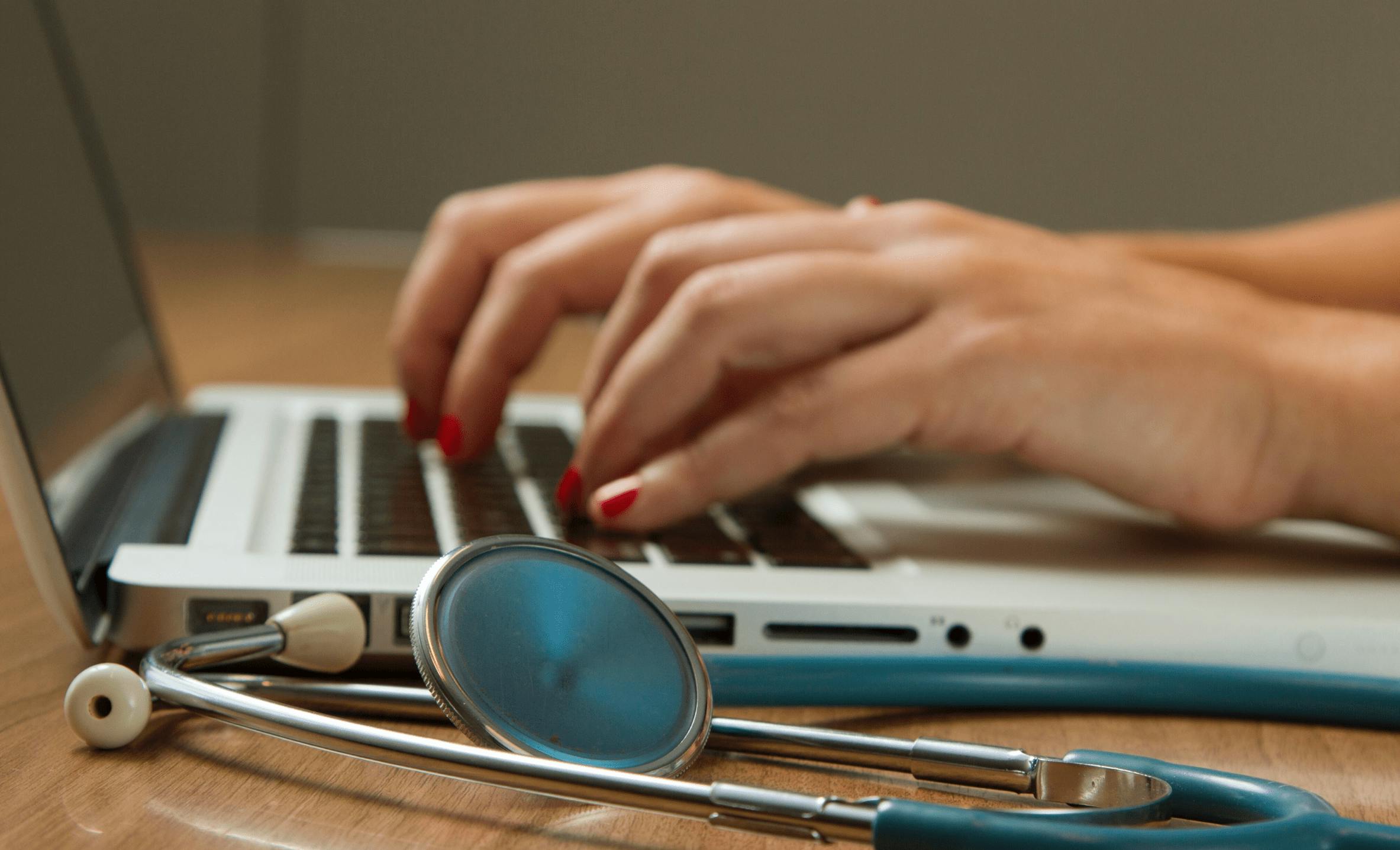
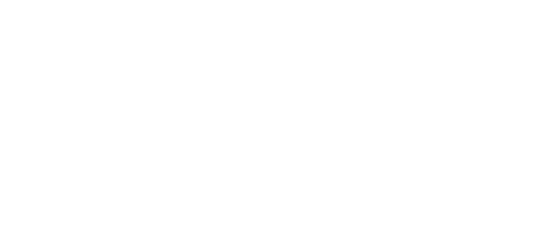
How King's College London used Encord to annotate videos 6.4x faster
Employing clinicians to label medical data is extremely expensive. Speed and accuracy are paramount. By leveraging Encord, the most senior (and thus, most expensive) clinician at KCL saw a 16x improvement in labeling efficiency, cutting model development time from 1 year to 2 months. In a published study, “Novel artificial intelligence-driven software significantly shortens the time required for annotation in computer vision projects,” researchers at King’s College London annotated endoscopy videos of polyps 6.4x faster on Encord’s platform. For researchers in the field, the accurate labeling of data remains “painstaking, cost-inefficient, [and] time-consuming.” Employing clinicians to label videos of pre-cancerous polyps is excessively expensive and thus inhibits the creation of large datasets. Encord vs. CVAT The study compared Encord, an enterprise-grade solution, with CVAT, an open-source tool, to analyze the speed and accuracy of labeling on each platform. Using a sample of polyp videos from the Hyper-Kvasir dataset, annotators leveraged the functionality offered on each platform. On the Encord platform, annotators employed embedded intelligence features, including object tracking algorithms and functionality to train CNNs to annotate the data. After labeling a small number of frames, annotators built a micro-model that predicted the annotations for the remaining frames. With CVAT, the annotators drew bounding boxes & propagated them across frames using linear interpolation of box coordinates. “With the model assistance, we found a much higher increase in efficiency within Encord simply because most labels were produced by a trained model and did not require correction.” The increased efficiency and accuracy decreases the time investment required by clinicians to annotate data and frees up their time for more productive activities.
Healthcare
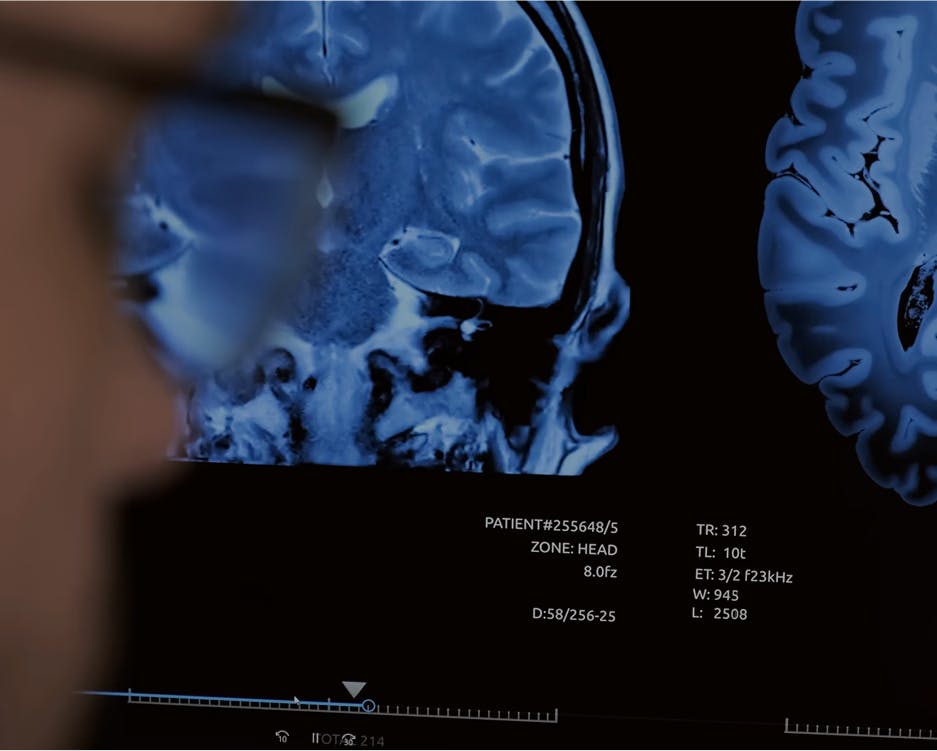
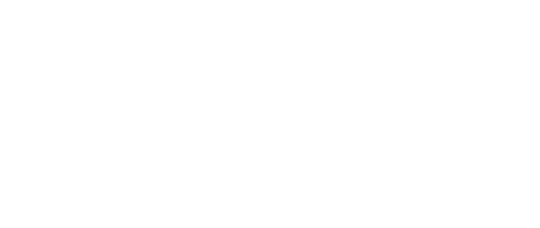
Floy Reduced CT & MRI Annotation Time with AI-Assisted Labeling
Floy develops AI that helps radiologists detect critical incidental findings in medical images. Floy partners with radiologists who use their medical expertise to annotate data used for training diagnostic models. When other annotation tools didn’t provide the flexibility or speed that radiologists need, Floy turned to Encord to improve its medical-imaging annotation process. Introducing Customer: Floy Floy’s technology scans radiological images to detect abnormalities, providing a second pair of eyes for radiologists. Radiologists have to interpret and make diagnoses from complex images, such as X-rays, CT scans, and MRIs. They are highly skilled medical professionals, but they’re also human, and their diagnoses are vulnerable to human error. Radiologists can make perceptual errors, in which they overlook abnormality, and interpretive errors in which they identify an abnormality but draw the wrong conclusions. Because radiologists have a tremendous workload – sometimes hundreds of images a day – perceptual errors account for 80 percent of diagnostic radiology errors. Floy’s AI helps solve the perceptual error problem for inpatient and outpatient radiologists. After radiologists complete reading of scans, they use Floy’s product to screen for abnormalities – such as tumors or metastasis – in spinal scans. The AI will alert the doctors to abnormal patterns that may have been overlooked by the human eye, requesting that they double check the scan before making a declarative diagnosis. Problem: Unreliable and Inflexible Tools for Radiologists Developing high-quality medical AI products depends on having high-quality training data. Having processes in place for obtaining consistent, high-quality annotations is of great importance. Floy tried working with open source labeling tools for medical images, but they didn’t have an option for building efficient workflows or sharing data across a team of radiologists. Floy’s radiologists had to download images locally, annotate them using the open source tool, and re-uploaded the image segmentations back to Floy. This unscalable, cumbersome process wasted radiologists’ precious time, decreasing productivity and increasing the likelihood of errors. The off-the-shelf annotation platforms Floy tried contained cloud-based features and allowed users to structure workflows, but they didn’t have specialized annotation features when labeling DICOM images. Radiologists couldn’t annotate seamlessly in these platforms without encountering issues, and some of these tools had bugs which caused image labels to shift location, meaning the radiologists had to redo hours of work. Floy needed a training data tool that enabled high-quality labeling as well as efficient workflow structuring and labeling. The company also needed a tool that made key employees happy. “Radiologists are highly specialized, well educated doctors. Radiological annotators have a separate and rare skill: the ability to interpret medical images and perform accurate segmentation,” explains Leander Märkisch, Co-Founder and Managing Director of Floy. “This skillset makes them hard to find. With inefficient data labeling processes, we have to find and employ more of them to develop our product. With cumbersome products that cause frustration, we run the risk of losing talented annotators.” Image annotation is also the most time consuming and expensive part of product development for medical AI, so labeling efficiency is key for keeping costs down. Solution: DICOM Image Specialization and Quality Control Features Looking for a tool that enabled DICOM image annotation and improved workflows for radiologists, Floy turned to Encord’s collaborative data labeling platform. Encord’s DICOM annotation tool can render 20,000+ pixel intensities as opposed to existing tools that manage just 256. Created specifically for medical image labeling, the technology perfectly fits Floy’s annotation needs. Annotating DICOM images in Encord with windowing pre-set set to 'bone' For data privacy reasons, Floy needed to work with a German cloud provider. Encord built out integrations with Open Telekom so that Floy could store patient data on local servers while still streaming it quickly into Encord’s platform for annotation. Encord’s user-friendly platform enables radiologists to move quickly from image slice to image slice, and it creates a workflow that prioritizes images by order of importance. It also allows reviewers and annotators to collaborate so that annotators can leave notes for reviewers, and reviewers can provide annotators with modified labels and feedback, helping them to improve. Using Encord’s quality assessment tool, Floy’s team has visibility into the entire annotation and review process. Not only does this tool help ensure that labels are of the highest possible quality, it also provides Floy’s team with insights about the annotators themselves. For instance, if one annotator produces consistently low-quality labels, Floy’s team can have them retrained. They can also see whether an annotator performs better on certain scan types and then adjust their annotation tasks according to their strengths. Annotator management dashboard in Encord Results: Higher-quality Data in Less Labeling Time With Encord, Floy has built a scalable workflow process that results in high-quality annotated data that feeds back into its models. Using Encord’s platform, Floy’s annotation team has labeled 5,100 scans, each containing between about 16 to 200 images, or a total of approximately 60,000 images. Equally important, Floy’s annotators are very happy when using the product. The time it takes for them to label images has reduced by approximately 50 percent for CT scans and 20 percent for MRI scans. Encord’s platform also facilitates new types of collaboration beyond those between annotators and reviewers. “Our ML engineers and product managers also use the platform,” says Märkisch. “Because everyone has visibility into the training data pipeline, our conversations have changed. We no longer have to ask annotators for general updates about the labeling process. We can instead use our insights about the annotation process to have discussions about product development timeline and make better business decisions.” Floy and Encord’s teams have benefited from close collaboration. The two companies solve problems together in real time. When Floy has provided feedback about the product from radiologists or data engineers, Encord’s team implemented features and improvements based on that feedback. Working with Encord, Floy can be certain that it’s training its models on high-quality data so that it can build great products that serve patients. As the company expands and builds technology that works with new modalities and different body parts, Encord’s platform can help them ramp up their training data processes to develop new products quickly.
Healthcare
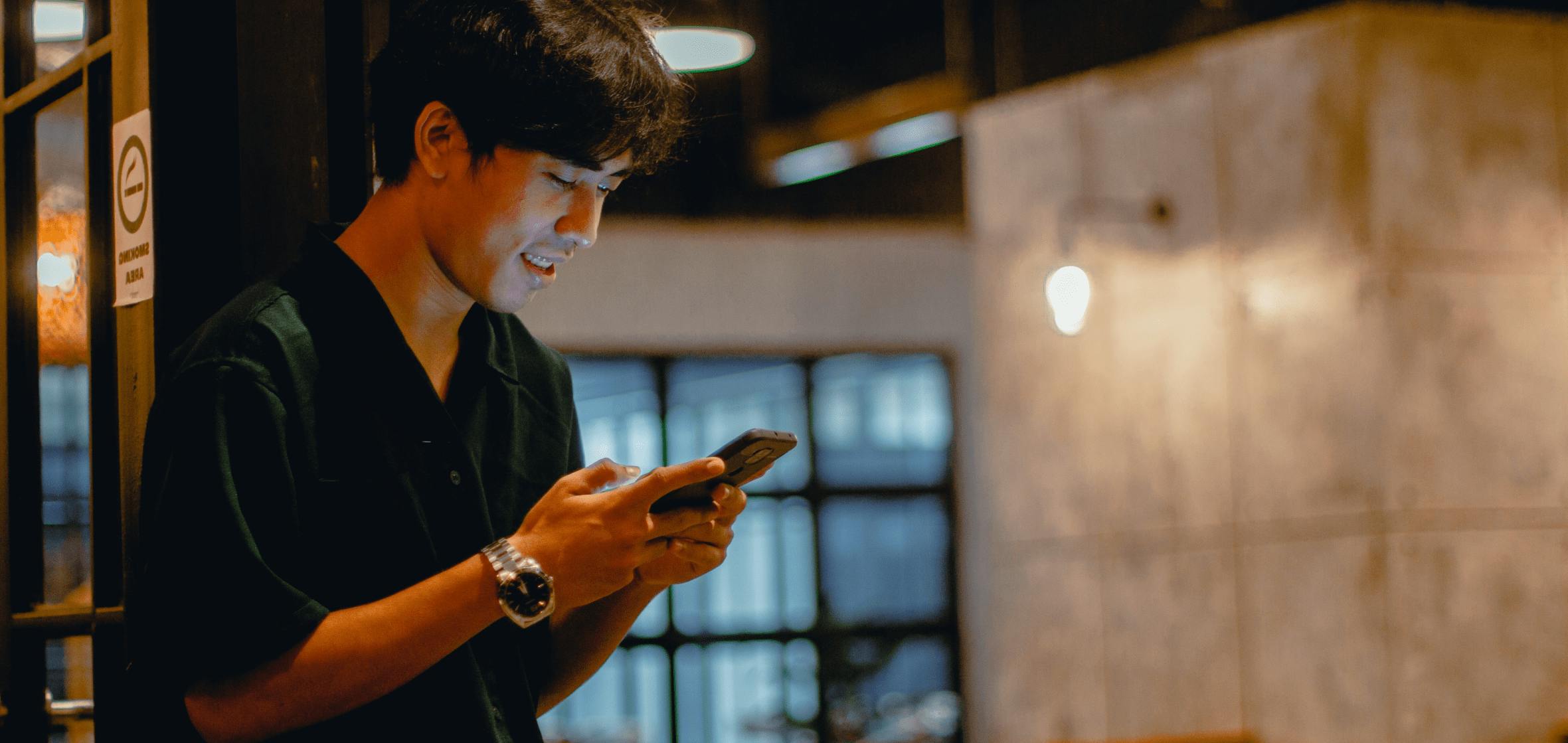
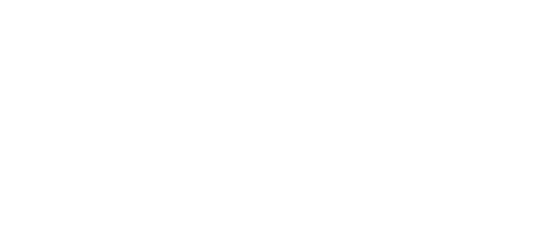
Reducing Model False Positive Rate from 6% to 1% with Vida ID
Vida, a full-service verified digital identity platform, serves customers throughout Southeast Asia. While facial recognition is a mature technology, most open-source facial recognition datasets aren’t reflective of the region’s populations. Models trained on these datasets perform poorly, so Vida needs to build and manage new own datasets. Using Encord’s platform, Vida can oversee a large labeling team and annotate tens of thousands of images quickly. Introducing Customer: Vida Vida uses optical character recognition and computer vision technology to provide a full-service verified digital identity platform. Digital verification empowers people to participate in the economy. For instance, financial institutions require identity verification to reduce fraud and ensure that assets arrive in the correct accounts, and ride-hailing services require drivers to verify their identity. Operating mainly in Indonesia, Vida’s services enable banks, fintechs, online trading platforms, ride-hailing companies, and other companies to verify the identity of users online. Indonesia is the biggest archipelago in the world, and traveling long distances can be challenging. With digital verification, customers no longer have to spend time waiting or traveling to get their identities verified. Vida users take a photo of themselves and a photo of their identification document. Vida’s technology then confirms the authenticity of the document, and compares the document to the photo and an authoritative source to verify the user's identity. Throughout Indonesia, a digital signature must be accompanied by identity verification. Being able to sign documents and open bank accounts online is incredibly beneficial, especially for micro-entrepreneurs and SMEs in rural areas. Vida’s platform reduces barriers for these populations when accessing financial products like loans and savings accounts. Problem: Large Datasets, Large Labeling Teams Vida trains its computer vision models to predict the liveness of an image. The models learn to determine whether the image contains a physically present person or whether the image contains a fake representation of a person, such as a pre-taken photo or a 2D mask. Although facial recognition is a mature technology, most of the open-source facial verification datasets contain faces from the Western Hemisphere or East Asia. When models train on these datasets, they don’t perform well on Southeast Asian demographics. Indonesia is also a majority Muslim country, so many women wear a hijab, an attribute rarely encountered by models that train on these open-source datasets. To improve model performance, Vida began collecting and annotating new datasets– ones reflective of Southeast Asian populations. The company needed a platform that could help them label and manage the tens of thousands of new images collected. Vida’s team tried using some open source tools, but none of them allowed for managing a labeling team. Furthermore, facial verification data contains sensitive Personally Identifiable Information (PII), and Vida struggled to find a tool that gave them strong access control and the ability to keep customer data on their own servers. Solution: Flexible Platform, Iterative Process With Encord’s platform, Vida could easily set up a system for managing their 20-person labeling team. They have key managers who oversee the other annotators as well as reviewers. When a new annotator comes on board, Vida uses Encord’s tools to evaluate the new annotations and ensure that all labels are high quality. Vida’s work requires managing a lot of images – about 60,000 in a project. At first, Encord’s interface was showing all 60,000 at once, which created challenges around speed. However, after Vida gave Encord’s team feedback, they quickly changed the UI so that Vida could scale up the amount of images in each project. “I’ve been very impressed with how Encord iterates on the SDK, listens to feedback, and constantly improves the product,” says Jeffrey Siaw, VP of Data Science at Vida. With Encord, Vida can keep the data in their own Amazon S3 buckets, alleviating data privacy concerns about access and storage. Rather than require that data be stored on its own servers, Encord’s platform facilitates the use of a signed URL allowing it to access and retrieve the data from a customer’s preferred storage facility without storing data locally. Results: Increased Labeling Speed, Decreased False Acceptance Rate In the first month of using Encord, Vida’s team labeled 70,000 images at a rate much faster than they expected. When trained on the old datasets, Vida’s previous models had a false acceptance rate– they predicted that an image was of a physically present person when it was not– of six percent. False acceptances can have serious implications for Vida’s customers. For banks, a false verification could result in the opening of a fraudulent account. In ride hailing companies, it can increase the chance of robbery because a driver with a criminal record is onboarded using a false identity. With Encord, Vida improved the quality of their datasets, and the new models had a false acceptance rate of only one percent. “Using Encord’s platform, we were able to train our new models on much better datasets with much higher quality labels, reducing our false acceptance rate to only one percent” says Jeffrey Siaw, VP of Data Science. As Vida continues to grow, the data science team will begin taking a more granular approach in their data management and labeling. They’ll try labeling faces differently and label attributes such as religious headdresses to better track how their models perform across more specific demographic features. Using Encord, they can label these datasets at speed while managing multiple projects with different types of labels and ontologies, all in one platform. Ready to automate and improve the quality of your data labeling? AI-assisted labeling, model training & diagnostics, find & fix dataset errors and biases, all in one collaborative active learning platform, to get to production AI faster. Try Encord for Free Today.
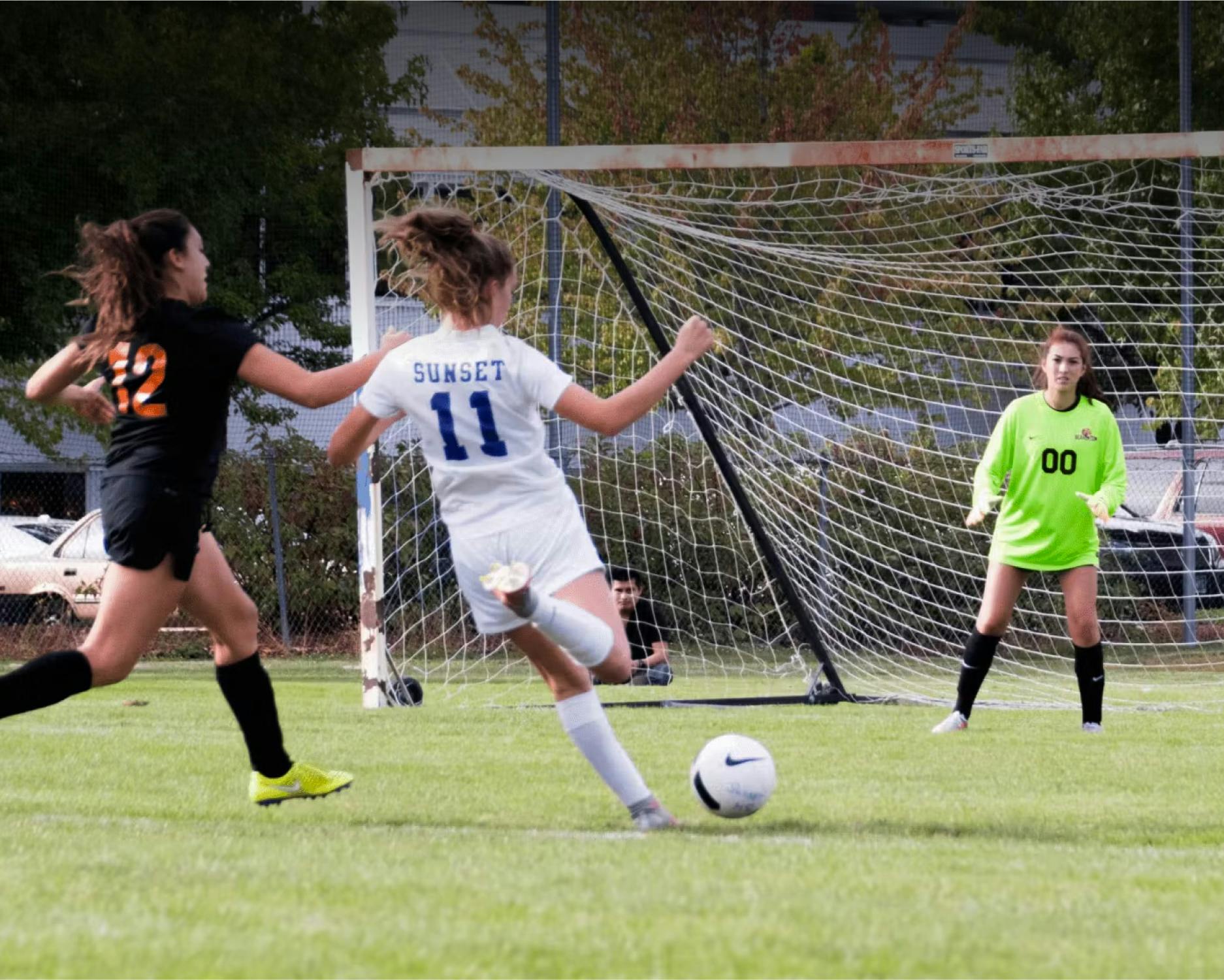
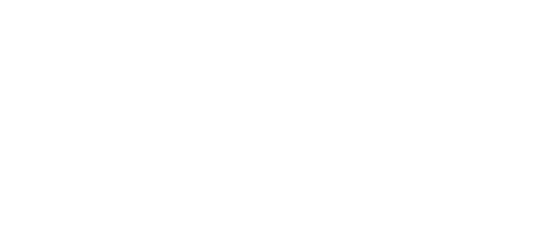
Rapid Annotation & Flexible Ontology for a Sports Tech Startup
A leading sports analytics company needed to label large amounts of video footage to train its models to identify and analyze key moments in matches. Labeling data quickly is key, but when the company’s rapid in-house annotation tool limited its ML team’s ability to iterate and develop new features, they turned to Encord to annotate at speed and build new ontologies quickly. Use Case: Sports Analytics This leading sports analytics company’s computer vision technology allows teams to record, live stream, and analyze professional quality videos of their matches. It detects key events, such as free kicks and goals, and player positions. Players and coaches can interact with the recording, look at stats, and review critical moments from the match to improve their skills. Problem: Scaling In-house Tools When labeling video datasets, the company’s annotation team must click within each frame to mark players and objects. At first, the company built its own in-house tool and interface for data annotation. While the tool worked well for labeling at speed, it had limitations: it took months to build and refine, and the result was a single-purpose tool. Having been built for a specific annotation task, the tool could only label certain objects, which restricted the machine learning team from trying new models, working on new problems, and building out new products and features. Spending months building an in-house tool for each specific annotation task wasn’t a feasible, sustainable, or scalable strategy. Solution: Using API Access and Object Detection The company’s annotation team has a workload that consists of approximately two million clicks, so speed matters. They needed a data labeling tool that allowed them to label objects rapidly as they moved quickly from frame to frame. Before finding Encord, the team tried a couple of high-profile off-the-shelf annotation tools, but these products didn’t have a multi-frame object detection feature. Most of them focused on fancy annotation features without prioritizing the importance of annotating at speed. For this sports analytics company, spending several seconds to click and select an object – as opposed to spending only one second to click and select an object – significantly and negatively affected the rate at which they could train and develop models. Speed matters most to the company because if the annotation team can label 10 times faster, then they can annotate 10 times as many frames. In other products, it wasn’t possible to quickly track an object across frames with a single click per frame, but Encord built out a feature to do exactly what the company needed. The team could easily add action classifications to the labeled data, providing the ML team with important information about cameras and frame timestamps. Another benefit of working with Encord was that its API allowed the company to take the datasets already annotated with the in-house tool and upload them into the platform. That meant that the company’s previous annotation effort was not wasted because the ML team could continue to use and expand upon the old data using Encord. Because Encord’s user-friendly interface allows for multiple annotators to use the platform at the same time, the company could also continue to use the external annotation team with whom they had worked and built trust for years. Annotating a video of a soccer match in Encord Results: Start Collecting Data Immediately While building a new annotation-specific tool in-house takes months, Encord can build out a new ontology in a matter of minutes. Having this flexible ontology enables this company’s ML teams to try out different ontologies, work with new data types, and explore different ways of annotating to learn what works best. Before using Encord, the ML team had to take the safe route because of the high cost of pursuing new ideas that failed. With a multi-purpose, cost-effective annotation tool, they can now iterate on ideas and be more adventurous in developing new products and features. As the company continues to build analysis features for additional sports, using Encord’s flexible, multi-purpose annotation tools will help them move with speed to create new training datasets for identifying key sports events such as jump balls, faceoffs, and touchdowns. By harnessing the power of data-centric AI, this computer vision technology is on the path to giving players around the world the AI-powered tools they need to improve their games.
Sports
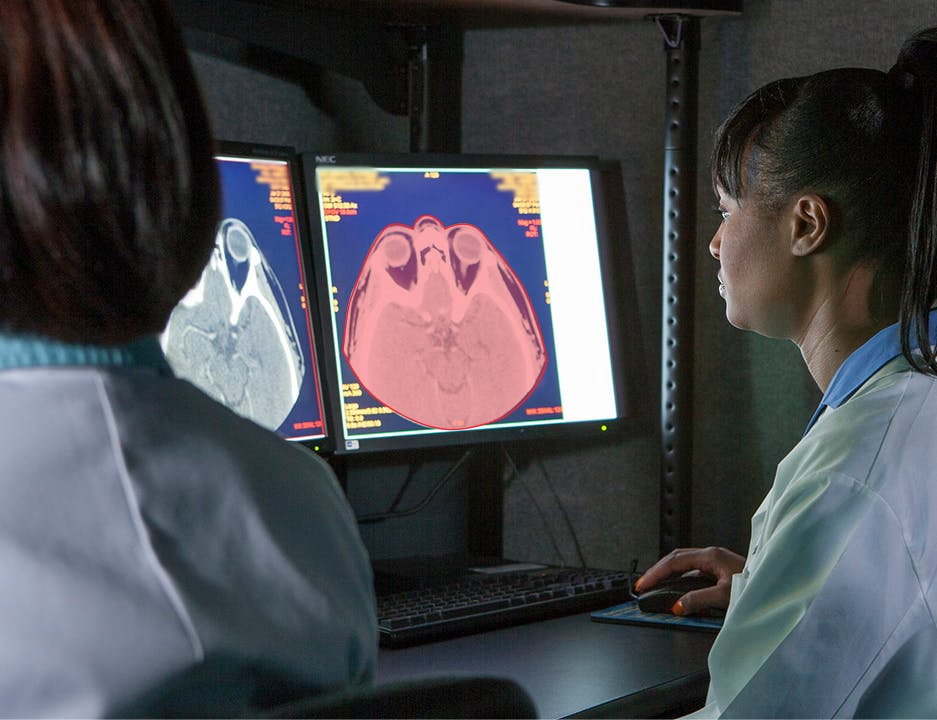
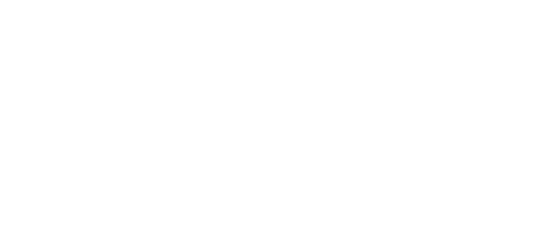
Customer Interview: Overcoming The Mental Torture of Manual Data Labelling
King’s College Hospital’s Director for Gastroenterology and Endoscopy Dr. Bu Hayee is– to put it mildly– a busy man. As a practising physician and assistant professor, he has a number of competing interests: academic, strategic, research, and clinical. In addition to supervising students and treating patients, he works closely with NHS England on their digital pathways and transformations, and he has a deep interest in how emerging technologies such as artificial intelligence and computer vision can increase the efficiency of patient care. In 2020, Dr. Hayee assisted in supervising a collaboration between King’s College London and Encord– the platform for data-centric computer vision– which aimed to increase the speed at which doctors could annotate polyps in colonoscopy videos (training data for computer vision models detecting precancerous polyps). Polyp detection in action We recently sat down with Dr. Bu Hayee to hear more about his thoughts on the role of deep learning in medical diagnosis, his collaboration with Encord, and the changing landscape of medical AI. The following interview has been edited for clarity and length. How has medical AI evolved over the course of your career? Dr. Hayee: It’s very new. We only began to see the practical application of medical AI in my speciality about three years ago. Endoscopy lends itself extremely well to computer vision because it's a very image oriented speciality. The diagnosis and evaluation of patients and the conditions they might have relies on image analysis, so computer vision is gaining a lot of traction in the field. Although I have had a longstanding interest in machine learning and how it can be leveraged to provide better patient care, unfortunately, until very recently, these kinds of machine learning projects have been slow to deliver because training a model requires millions and millions of data points. Preparing that data requires laborious image annotation and time-intensive quality control. These barriers make it difficult to move a model out of the research stage and into production. These roadblocks also mean that this technology has mostly been the domain of large industries as opposed to other, smaller actors that have been dissuaded from pursuing deep learning because of the challenges associated with training data curation and model development. You mentioned data annotation as a laborious process. Could you describe what it’s like to annotate these images manually? Dr. Hayee: It’s mental torture. Annotation is simply one of the most painful tasks that we have to perform in image based research. Labelling data is time consuming, and it requires the attention of someone who understands the subject well enough to annotate the images. Reliable and highly accurate annotations are the bedrock of the machine learning process, so labelling has to be done properly. One option is to outsource the images to a company that provides annotation services, but since these annotators have no background in medicine, clinicians must educate them about the data which is in itself a labour intensive effort. Alternatively, a hospital can pay a highly trained medical professional with two postgraduate degrees to sit in front of a screen and click a mouse for hours, which is not an efficient or cost effective use of time. How did Encord’s tools change the image annotation experience for you? Dr. Hayee: The built-in tools allowed us to rapidly annotate large numbers of data points. Before, using clinicians to annotate precancerous polyp videos had prohibitively high costs because of the large number of datasets needed to train the model. With Encord, we annotated the data over six times faster than using traditional methods. That’s a fantastic result because at those rates we can still deploy highly qualified medical professionals to annotate data, but we don’t take up a huge amount of their time. Much of the task shifts to quality assurance, which is much quicker than annotating, because Encord’s micromodels automatically generated 97 percent of the labels. Rather than acting as annotators, we become the humans-in-the-loop who review the machine’s output, in this case labels, for accuracy. Compared to other label assistance tools that we’ve worked with, Encord’s user experience is much smoother. The interface is intuitive, so it requires very little training to become a sort of “expert” at using all the functionality. That’s what you want, right? The mark of a great product is in many ways its useability. For instance, you plug in your smartphone, it works, and you don’t need much training to operate it. That’s the kind of UX we’ve come to expect in the 21st century. When it comes to implementing medical AI, we’ll need that kind of experience because doctors aren’t programmers. We’re end users, and we don’t want to have to learn to code to use the product effectively. We want a product that compliments our medical expertise and enhances our medical practice without requiring us to become computer science experts. Encord's tools in action What’s the greatest benefit that AI in general, and computer vision in particular, can bring to your field? Dr. Hayee: In short, time– time for both patients and clinicians. We’ll be able to give patients real-time evolutions of what we’re seeing on the screen. We’ll be able to detect abnormalities visually with greater reliability and greater speed rather than having to wait for a microscopic confirmation. When it comes to patient care, physicians will have more time to dedicate to a care plan because we won’t have to depend on the current pathways that we use for diagnosis, such as waiting for a histology on a biopsy we’ve taken to come back from the lab, before we make a care management plan. AI will be incredibly useful in decision support. It’s not a decision replacement. The machine won’t replace the doctor and diagnose the patient itself, but it will provide a second pair of eyes, so to speak, for an already educated clinician. The machine can confirm the doctor's diagnosis, increasing the physician’s confidence so that they can make a management plan and treat the patient straight away. Alternatively, if the machine detects something different from what the doctor had thought, the doctor can take a bit more care, be a bit more cautious, and investigate a bit more thoroughly before making a diagnosis and devising a management plan. Both of those scenarios are positive in my book. How do you think patients and medical professionals view adoption of AI for medical treatment? Dr. Hayee: I think there’s some confusion around the technology’s role. Even calling this technology AI unnecessarily raises some hackles because a lot of people associate AI with The Terminator and iRobot– two of the worst movies to have brought AI into the common consciousness because they perpetuate such a negative, unrealistic view of the technology and what it does. When I talk to people about AI, I say: ‘Listen, these aren’t thinking machines or all-seeing eyes. They aren’t even as smart as a three-year-old child putting blocks into a hole. They’re not going to replace doctors or nurses.’ Medical professionals will become comfortable working daily with AI. In time, computer vision will become what our Texas Instrument calculators are to us now. It’ll make us better and faster at our jobs. I can do simple addition without my calculator, but the calculator augments what I do and improves my performance. That’s what AI is going to do for healthcare. The clinician will always be the person who decides, but the AI will give us additional information to make that decision, similar to how we make decisions about treatment based on blood test results. You mentioned earlier that time was the greatest benefit that AI could provide to patients. Can you see AI eventually making a profound difference in early diagnosis for diseases? Dr. Hayee: One would hope so. Not catching a disease before it’s too late– diagnosing a cancer at a late stage when an early diagnosis could have saved a life– those are the kinds of things that keep physicians up at night. They’re horrendous, and unfortunately, most of us have personal experiences of them. Physicians constantly wonder how they can keep such things from happening. Right now, we don’t have enough proof to say with certainty that AI will be able to do that, but if you look logically at what AI purports to do, then hopefully it will only be a matter of time before we can definitively prove that AI is saving lives In healthcare, one of the biggest strengths and biggest weaknesses is the large amount of data that we generate. If we knew how to tap into it, I’m sure it could help us solve many of these problems. It could be a strategic asset that enables us to help our patients rather than just numbers on a page Products like Encord are going to help us do that because they’re allowing us to unlock the potential of that data faster and in a more democratising way. Not having to be a highly trained programmer to get started with an AI project or proposal opens all kinds of opportunities for innovation and new ideas from new actors. That’s got to be the way forward. If we're going to make progress, we can’t forever concentrate these efforts in big silos and big companies. We need to have more grassroots involvement, and products like Encord are going to help make that participation possible.
Healthcare
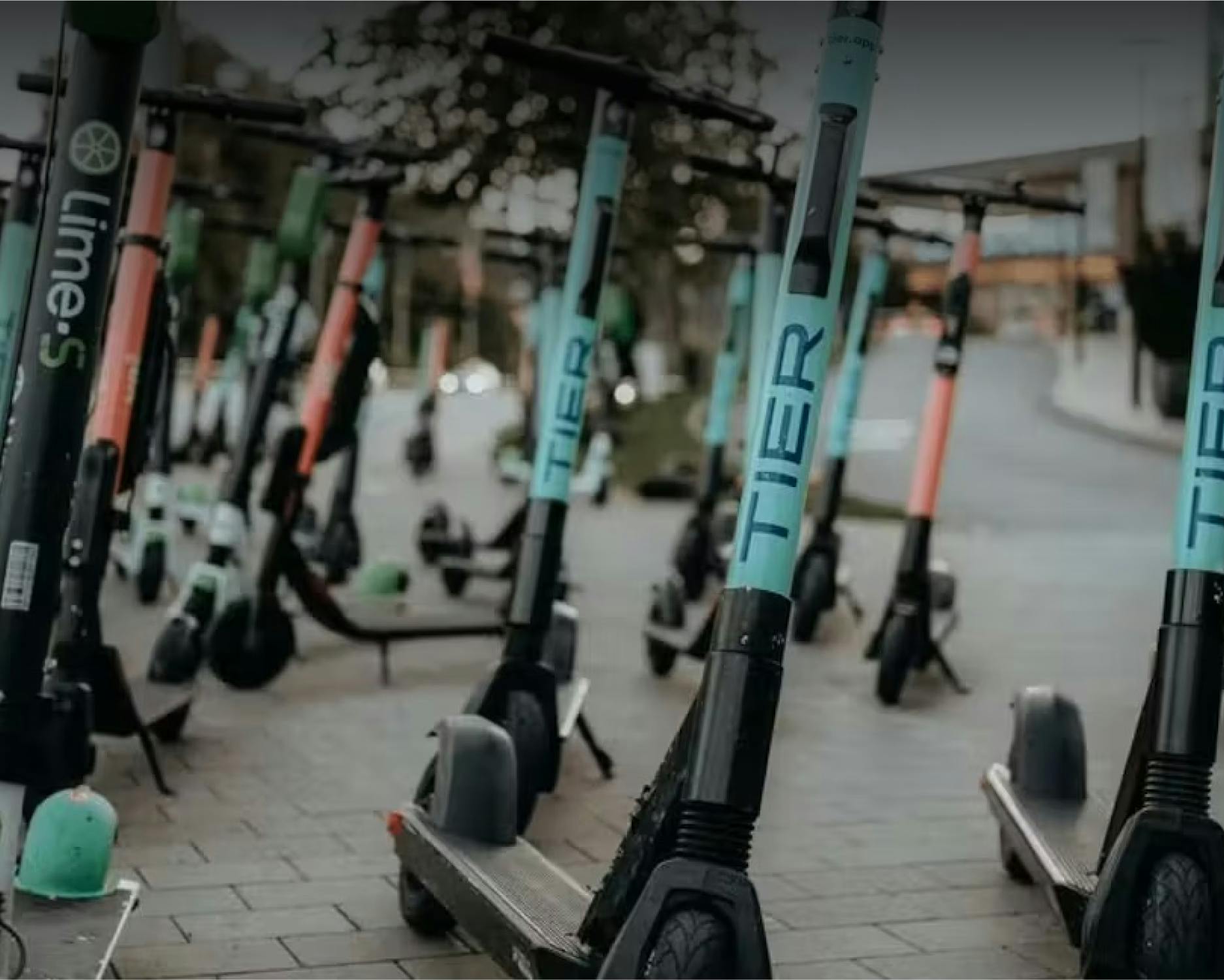
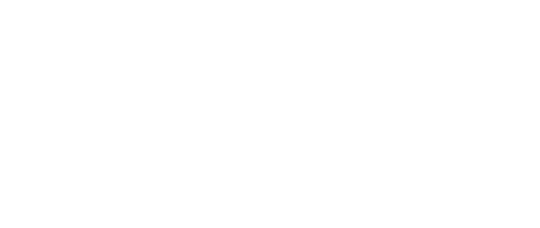
Training Computer Vision Models to Track Rented Assets
How can AI help solve real-world operations problems for businesses and their on-the-ground employees? That’s the question that drove Charlotte Bax to start Captur. Founded in 2020, Captur uses computer vision to capture images of and detect damage to physical assets. Over the past two years, Captur has worked with major global players in micro-mobility and last-mile delivery, including Tier, Zapp, and Buzzbike, helping these companies access real-time data about their operations. Like most start-up founders, Bax wears many hats. Every day, she is responsible for making a variety of decisions. One day, in early 2021, Bax made the decision to use Encord to help build out Captur’s data pipeline infrastructure. “When you’re running a startup, anything that’s core to your product, you build it. In any case, where you can, you buy tools so that you can move faster,” says Bax. “Machine learning is core to our technology, but the reason for buying Encord was that it could help us improve the quality of the data going into our models, and that would help us speed up our go-to-market measurably.” We recently caught up with Bax to hear more about the power of computer vision to assess the condition of remote assets, her experience using Encord, and her mission to bring applied AI to long-tail industries like operations. The following interview has been edited for clarity and length. Could you talk a little about the problem Captur is trying to solve? Charlotte Bax: The problem we’re trying to solve is basically: how can companies track assets and resolve issues remotely? Ultimately making scaling logistics easier and more efficient. Most people are familiar with the experience of renting a car. Before you leave the parking lot, you walk around the vehicle with a representative from the rental car company. Together, you mark down any visible signs of damage. This inspection usually only lasts a few minutes. But what happens if something isn’t caught by either party? Who’s to say if the scratch on the passenger’s side door was there before the driver rented the car? It’s a lose-lose situation for both parties. Computer vision can help companies and users track that type of damage, removing the likelihood of human error. That example is just the tip of the iceberg. Property, vehicles, construction equipment– there are multiple different verticals that could benefit from computer vision providing real-time operations information about physical assets. As the on-demand business model grows, more and more companies will look to computer vision technologies because on-demand businesses–the ones where customers rent items on-demand–need new operating tools, ones that can enable them to scale operations that manage rented and shared physical items efficiently. It might surprise you to learn that most companies with physical operations still rely on hand-built spreadsheets for asset tracking. This method lends itself to errors and disputes. Also, spreadsheets don’t provide any real-time data or visibility about operations, so companies don’t have access to the data needed to develop basic metrics for making decisions about their business. Computer vision can not only help companies track the condition of their assets remotely, but it can also provide them with the data needed to automate their frontline decision-making using AI. Let’s talk about some of Captur’s customers and how they’re using this technology. Bax: We’ve been working a lot with micro-mobility companies, such as e-scooters and e-bikes. We’re starting to move into some other asset classes, such as larger vehicles like vans and cargo bikes, and eventually, we aim to offer our service for any mobile asset. Our product is API first, so for B2B customers, we’re embedded in their apps. With e-scooters, for instance, riders take a photo of the scooter at the end of their trip and upload those images into the company’s app. Our technology automatically verifies these photos, and ultimately, Captur’s models provide an output in the form of a suggested action based on that visual information. For instance, ‘Yes, that’s good parking,’ or ‘No that’s bad parking’. ‘Yes, the scooter is in good condition,’ or ‘No it’s been damaged’. The goal is to provide the end user real-time feedback on their device and instantly prevent safety issues. How valuable are data sets and training data for the future of your business? Bax: Incredibly valuable. Our approach as a business has always been a data-centric approach that lets us build models in a transparent and collaborative way. Think of it as building personalized machine learning for companies. We build up data sets that are very specialized– certain types of assets that have certain types of issues, damages, and conditions. These use cases are very specific. We can do that in a scalable way across multiple customers because we have a solid data review process and pipeline, which has been critical for both building models and providing our customers with transparency and confidence. Why and how do you use Encord? Bax: When we came across Encord, we were thinking about how we were going to scale our data annotation pipeline and data quality assurance. We wanted to be checking subsets of our models’ decisions at any given time to evaluate their performance. That’s something we could have spent a solid nine months alone building in-house, but we really liked what Encord could do. We have essentially built out a critical part of our own pipeline infrastructure in tandem with them. Their team has been a real product development partner to us. We use Encord as a part of our training and evaluation pipeline. Both annotating new datasets and evaluating the results from live models. We use expert-in-the-loop feedback to improve the model’s performance as quickly as possible. In the beginning, we used a few different open-source tools to annotate images for training models. Still, once we got the scale of moving beyond research projects and really needing a production-ready system, we moved towards using Encord because of the additional features in the platform– like having a robust system to do quality control and a method for measuring the confidence scores of how much labelers agree– were ideal for scaling. We needed a platform that enabled various different types of labeling because we have different types of machine learning challenges. Our goal is to diagnose the condition of an object, which requires several steps that include individual issue detection– e.g. damage to a scooter frame– and the condition of where something is placed, like where a scooter is parked. We have six people who actively work on Encord daily, and the Encord team has been a great partner in helping us solve labeling challenges within the platform and also in helping us think about where this platform sits in the larger infrastructure we’re building. Overall, Encord’s vision for automating data labeling and training data management really aligns with our mission of bringing computer vision to this long-tail of industries like operations. Many industries just haven't been able to take advantage of AI or computer vision automation because the data set doesn't exist or the tools that enable them to do so don’t exist. Encord is really the start of the tool that will enable many startups like us, who are focused on bringing applied AI to every industry, not just healthcare and autonomous vehicles.
Logistics
Retail & E-Commerce
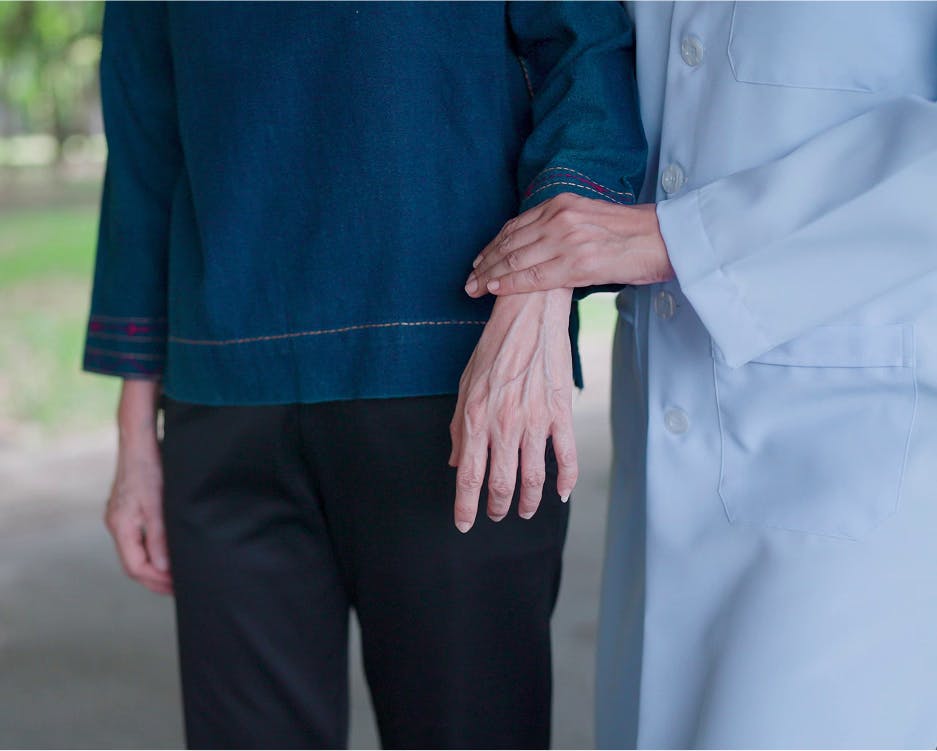
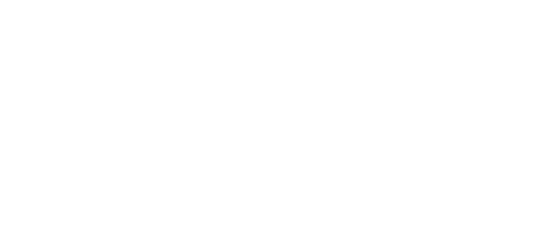
Using Computer Vision to Prevent Falls in Care Homes and Hospitals
In the United States, approximately 36 million senior adults experience falls every year. These falls result in about three million emergency room visits and 32,000 deaths. In Western Europe, approximately 8.4 million individuals aged 70 or older suffered a fall-related injury in 2017. Falling has a huge impact on the quality of life of senior adults. Severe falls can cause mobility reduction, mental confusion, and – in extreme cases – death. For senior adults residing in care homes, the likelihood of falling is three times greater than that of their peers living elsewhere. Countries that are home to aging populations are at risk of an epidemic of elderly falling. Such an epidemic will not only lead to an immense amount of individual suffering, but it will also consume a considerable amount of healthcare resources. However, if we knew more about the conditions that led to falls, perhaps we could prevent them. That’s the thinking behind Teton AI, a computer vision company designing fall-prevention tools for healthcare workers in hospitals and care homes throughout Denmark. We recently got together with Teton’s Chief Technology Officer Esben Thorius to learn more about the role of computer vision in fall prevention and how Encord’s training data tooling has provided Teton with the flexibility needed to label and manage training data in the ways that result in the best model performance possible. The following interview has been edited for clarity and length. Tell me a little bit about how Teton uses computer vision to help prevent falls. Esben Thorius: We are building tools focused on capturing and conveying relevant and timely information to healthcare staff when they need it. At the moment, we are deployed in one hospital across three wards and in one care home. Our main focus is on understanding patient behavior so that we can prevent adverse events even when care staff is not in the room. This concept is rooted in two core pillars: context and continuity. As patient care is currently practiced at night and in the evenings, medical staff only check on patients every couple of hours, so they don’t really know what’s going on during the time between. A patient can easily have fallen or injured themself in the meantime. Teton aims to be the constant observer that can bring continuous and contextual information to the staff while they are doing other work. With our technology, staff gain insight into what the patient is doing. Our monitoring system provides information about the patient's behavior. Using deep learning, such as object detection and pose estimation, our models identify movements, and our system alerts care staff of actions that put the patient at risk of falling. For instance, it lets the staff know if a patient has moved their legs off the side of the bed. The information about that position lets staff know that they need to react and go help the patient out of bed to prevent a fall. Because the staff knows that the other patients are lying calm in their beds, they can focus their full attention on helping the one patient currently in need of assistance. How does having access to this information help prevent falls? Thorius: At the hospital wards where we’re working, risk prevention is about detecting the pre-stages of a fall. The patients are neurological, geriatric patients, so the staff has time to react to these pre-stage poses because the patients move slowly when getting out of beds or chairs. We categorise these pre-fall stages into general movement which is when a patient has started to move a lot in the bed, sitting up, moving legs out of the bed, sitting on the edge of the bed, and starting to stand up. Care staff can determine at what stage they want to receive a notification for each individual patient. For instance, because the staff knows the patients’ conditions and histories, they know which patients have the highest risk of falling. The nurse can set an alert in Teton’s system so that if one of those patients begins to sit up in the bed, they receive a notification saying, “A high-risk patient is sitting up.” Memory care homes are a newer market for us, and the use case is a bit different there. We’re still focused on how to prevent falls, but we are taking different factors into account beyond the pre-fall stages. For instance, maybe the patient fell because their walking aid wasn’t placed near enough to their bed. We’re using AI to figure out why these falls are happening in the first place in addition to preventing them. We are currently implementing methods that allow staff to conduct fully remote and privacy-sensitive check-ups on residents. Such check-ups would provide staff with only the necessary information they need to ensure the resident is safe and remove the hassle and risk of waking residents during the night. Does the technology have any other use cases? Thorius: I think some of its greatest potentials actually rests in its ability to automate documentation for healthcare workers. At the moment, nurses and care workers spend 30 percent of their time on documentation. Understanding long and complex sequences would unlock the ability to precisely document the entire patient journey. Documenting this information automatically would save the staff a lot of time that could be spent on patient care rather than administrative tasks. What sort of hardware does Teton require? Thorius: At the moment, we have one camera in the room, infrared lights for nighttime visibility, and a small computer. If there’s something a nurse needs to be notified about, the computer sends the data out in a digitised version, and the nurse receives an alert. Unlike CCTV footage, our product doesn’t require someone to monitor it constantly, and it doesn’t save video data. Once the model is running, it’s a fully enclosed system. We don’t need to save the data, and we’re not actually collecting data because the only thing we are sending out is a fully anonymised and digitised version of the events in the room. What are the greatest barriers you encounter when trying to implement this technology? Thorius: Obtaining the proper consent for data and privacy laws can be challenging. We need consent from all the patients and care staff. There’s often an initial fear among care staff that we are filming them. Sometimes, they worry our product is a surveillance tool designed to watch them perform their jobs, so we have to communicate our purpose and technology clearly while also obtaining the data in a compliant and legal manner. Also, as with all deep learning, we need a lot of data to train the model to perform well, and we need to annotate that data. Figuring out how we should annotate different features in the data based on the outcomes we want to achieve has been a challenge. At first, we tried using normal bounding box detection, overlapping, and heuristic algorithms. We realized that this wasn’t going to solve our problems, so we moved away from the bounding box idea. Our system worked a lot better if we let the network directly predict different classes or states of patients, for instance, by asking the model: Is this a person? Is it medical staff standing on the floor, or is it a patient in bed? Is it a patient in bed with their legs over the edge? The flexibility of the Encord platform helped tremendously because it was easy to change to this type of annotation strategy. As we’ve been developing this technology, we’ve had to be adaptive. The Encord platform is capable of being flexible and merging with our progression along the way as we’ve made changes and pivoted. Tell me a little more about how Teton uses Encord. Thorius: We started using Encord because they had begun building a pose estimation labeling tool which was an interesting option for us. We mostly use the flexibility of creating and quickly setting up a project in the platform. There’s a nice feature where you can use the same data for different projects and then quickly set up a new way of labeling things in the data. Using Encord’s SDK, we’ve built some internal pre-labeling tools to take that same labeled data and train a much larger model on a more specific task than what is in production. We then use the SDK to upload those labels and data directly, and we have some annotators who can quickly review and correct the labels. After that, we can easily download the labeled data through the SDK and continue to train our models with it. We really value that loop with the SDK and having the flexibility to set up new projects and have unique labeling tools that don’t just limit us to bounding boxes. In the wake of the Covid-19 pandemic, as hospitals and care homes struggle with medical staff shortages, Teton AI is leveraging cutting-edge technology to provide medical staff with timely information, remove unnecessary pressures from their daily routine, and increase job satisfaction. Encord is proud to work with Teton AI as they support medical staff in providing patients with the best possible care.
Healthcare
Trusted by 200+ of the world’s top AI teams
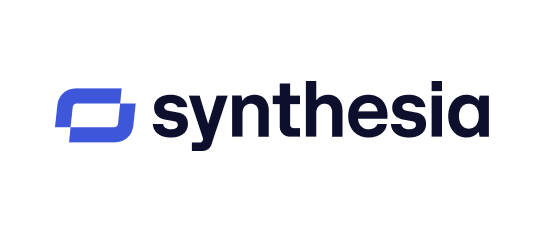
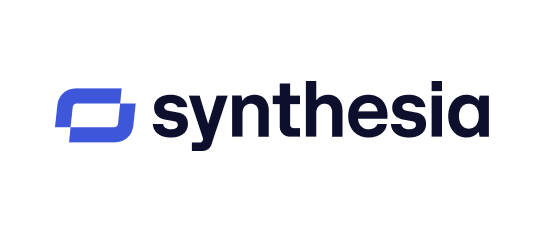
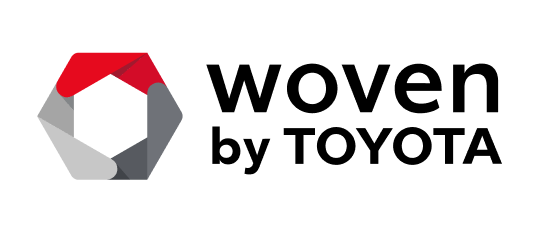
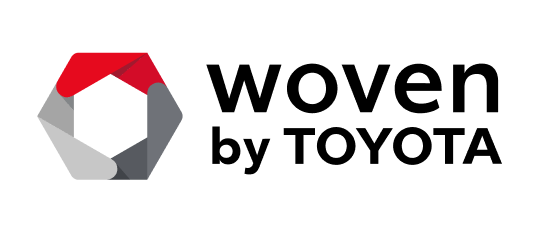
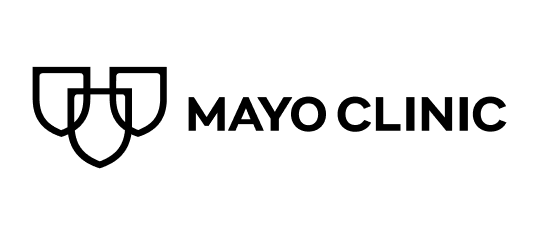
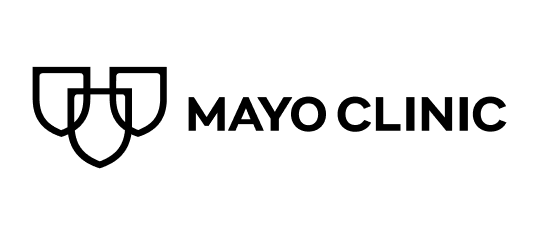
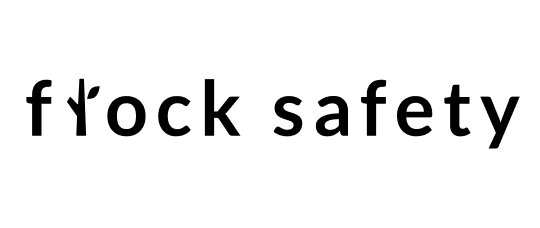
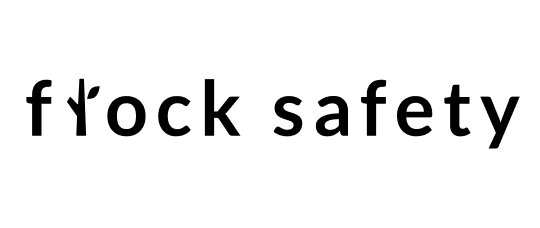
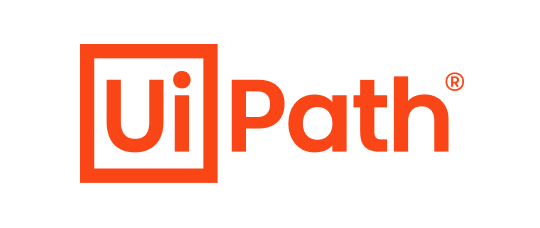
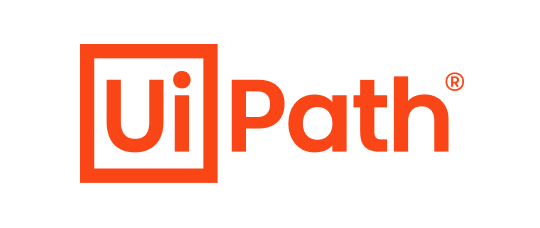
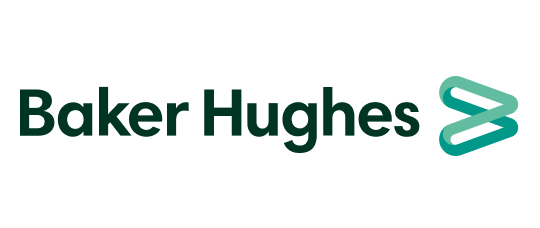
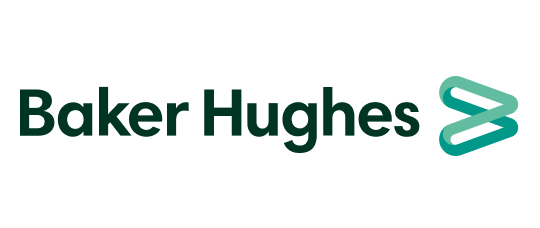
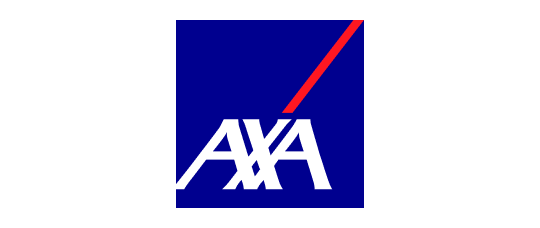
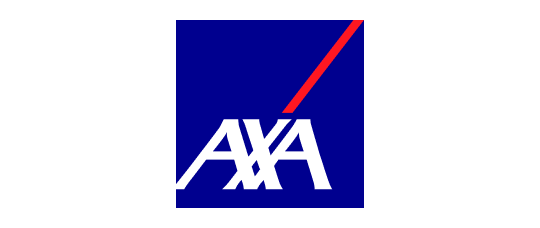
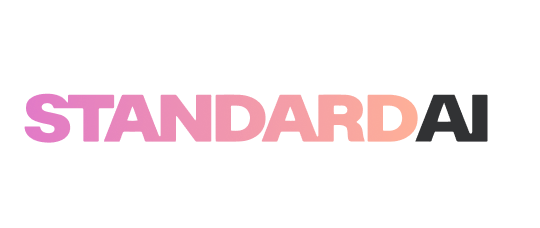
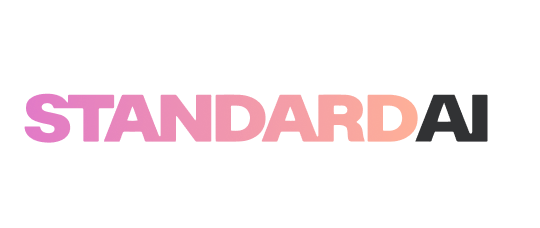
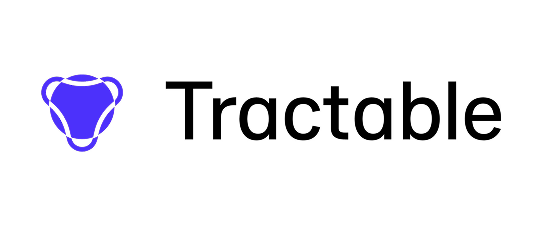
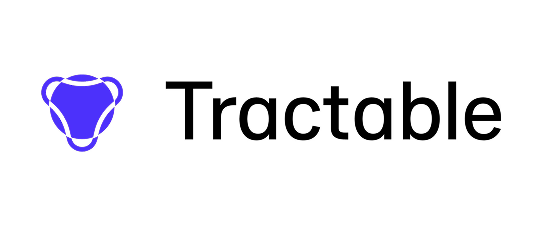
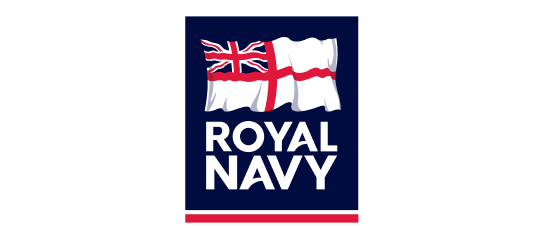
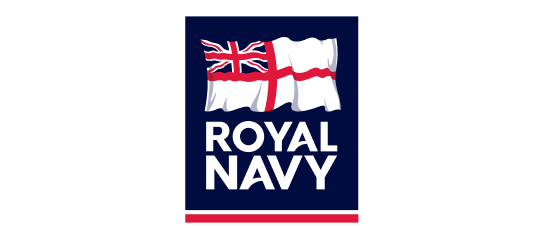
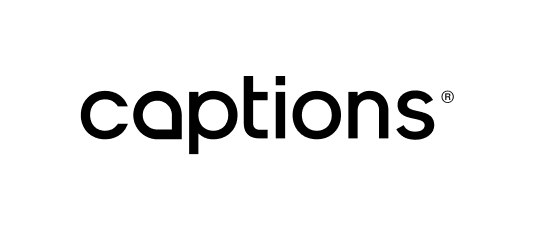
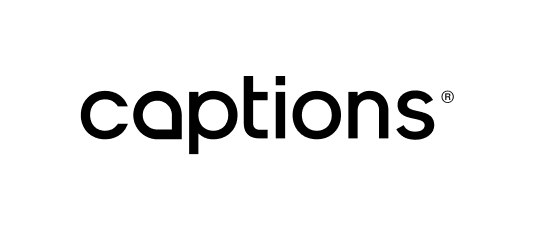
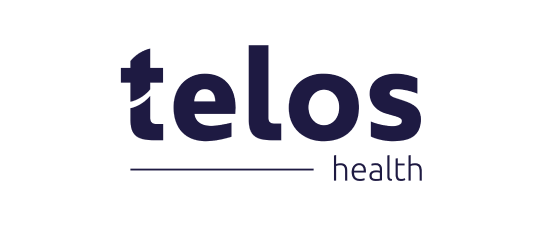
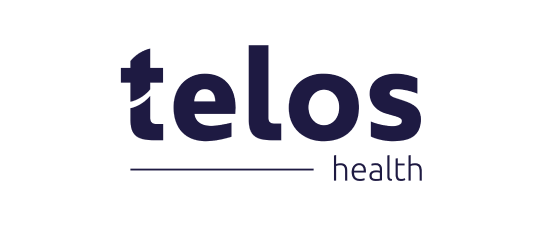
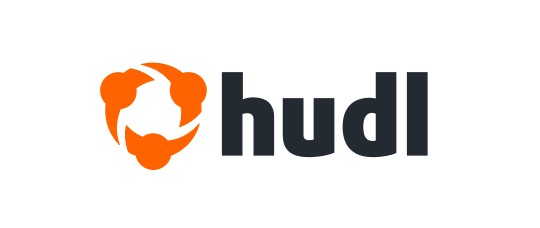
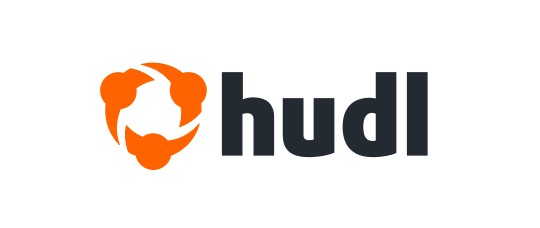
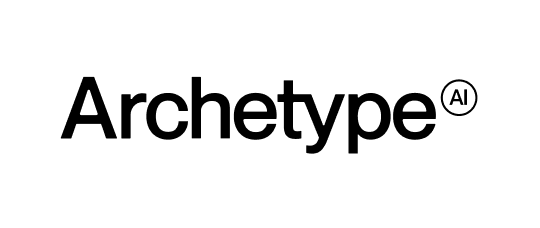
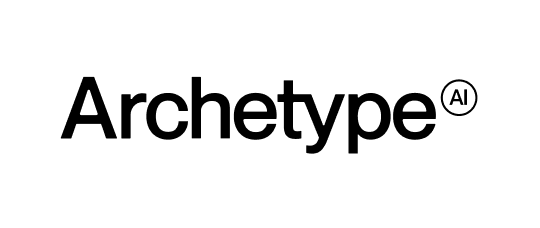
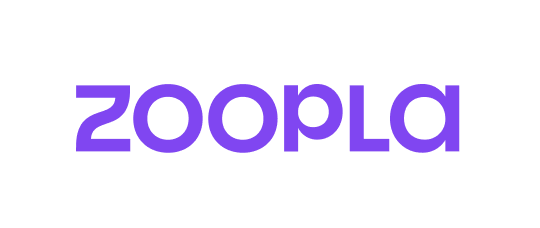
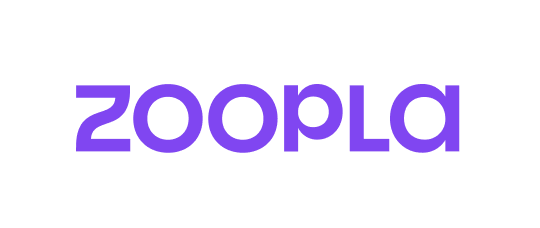
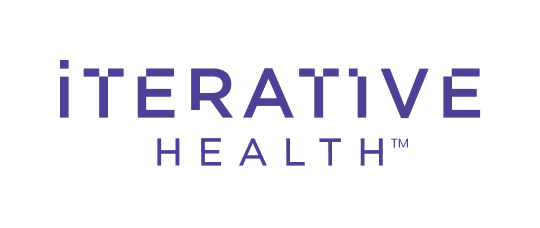
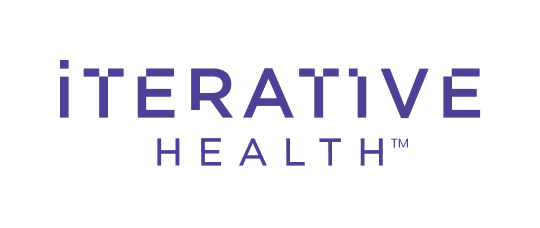
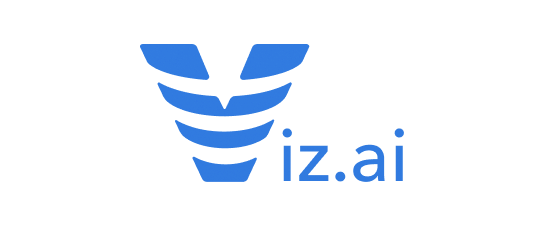
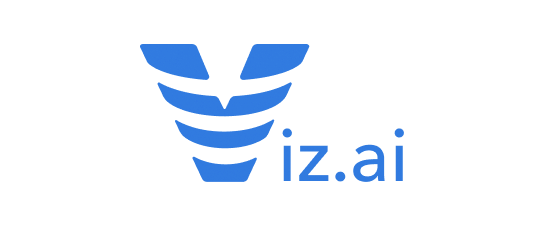
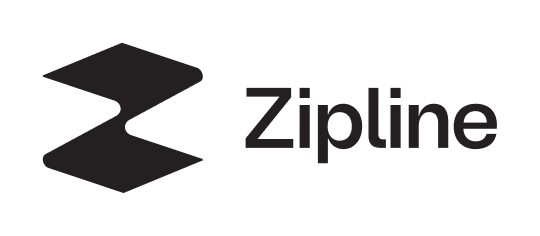
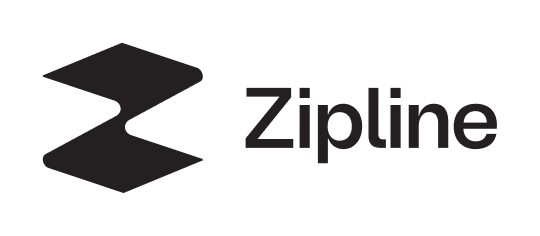
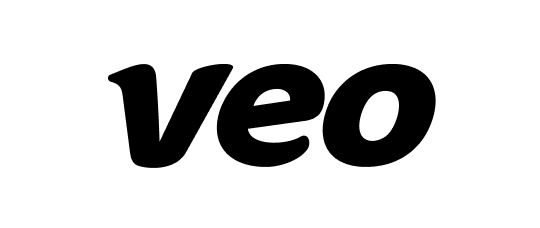
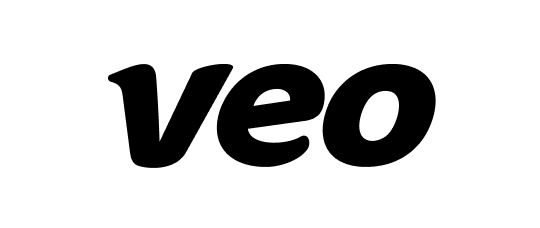
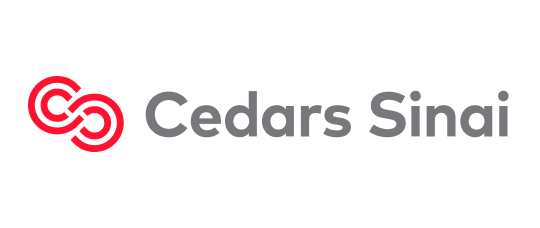
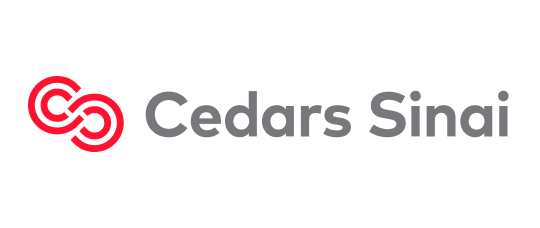
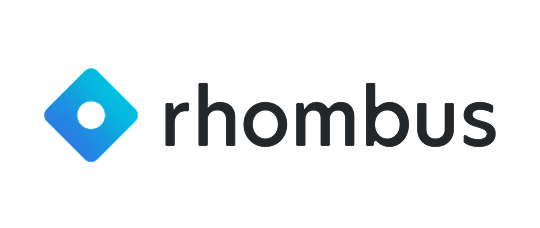
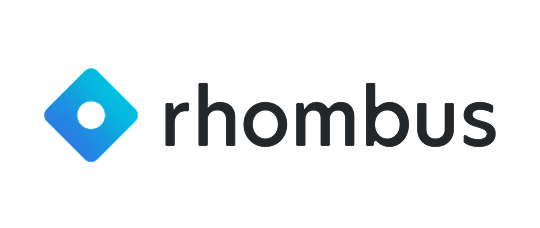
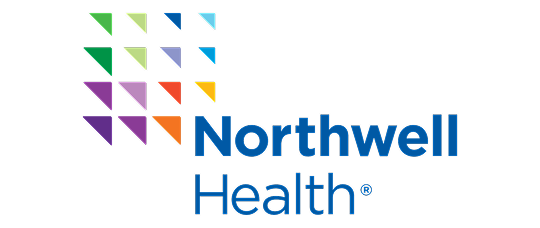
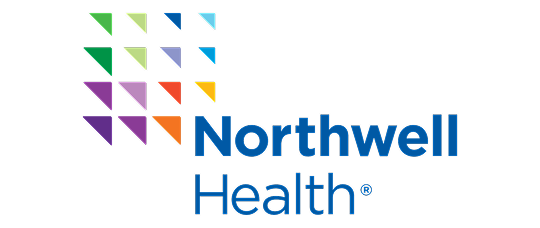
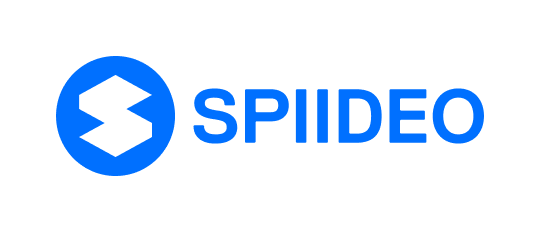
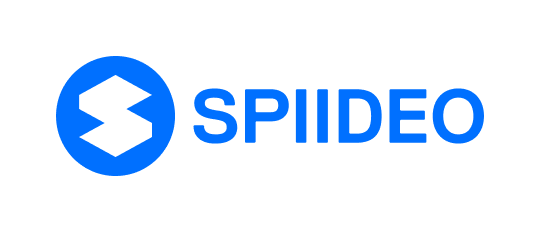
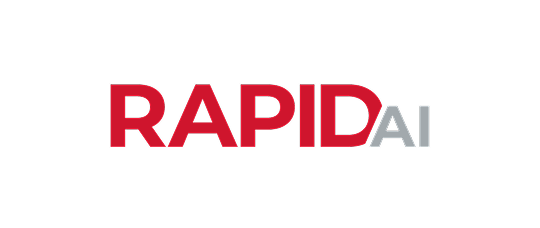
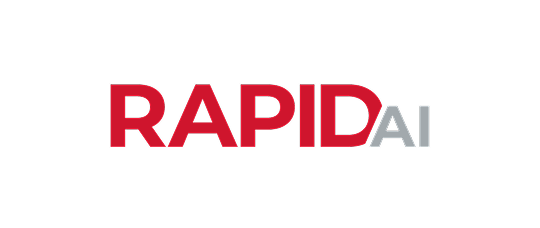
Take control of your ML pipeline with Encord
Forget fragmented workflows, annotation tools, and Notebooks for building AI applications. Encord's Data Development platform accelerates every step of taking your model into production.
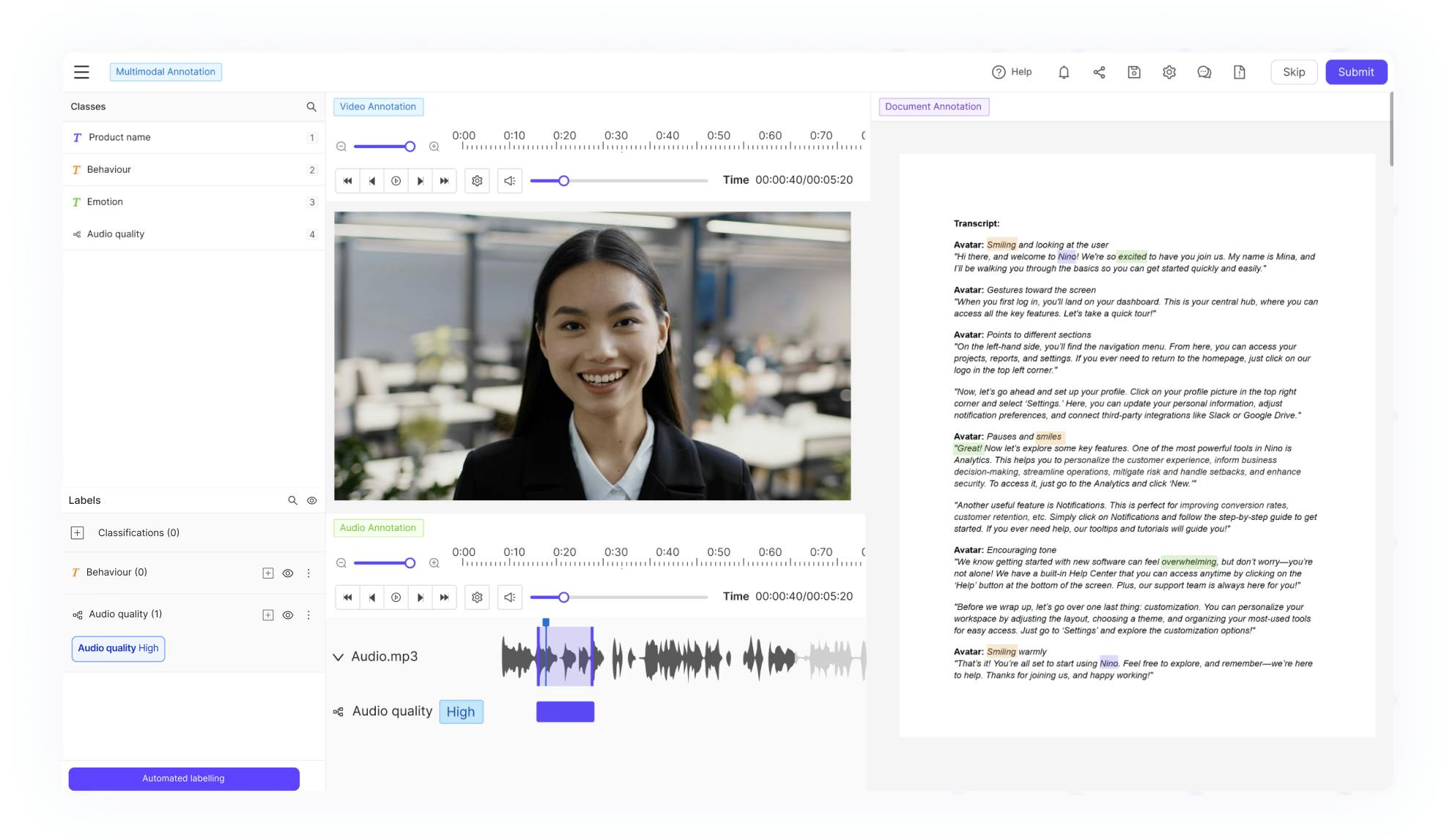